- 1 Experimental Psychology, University of Groningen, Groningen, Netherlands
- 2 Centre for Child and Family Studies, University of Leiden, Leiden, Netherlands
- 3 Leiden Institute for Brain and Cognition, Leiden University Medical Center, Leiden, Netherlands
- 4 Donders Institute for Brain, Cognition and Behavior, Radboud University, Nijmegen, Netherlands
- 5 Rotterdam School of Management, Erasmus University, Rotterdam, Netherlands
Medial prefrontal cortical (mPFC) functions may be aspects of ventral or dorsal control pathways, depending on the position along a rostral–ventral to caudal–dorsal gradient within medial cortex that may mirror the pattern of interconnections between cortex and striatum. Rostral–ventral mPFC is connected to ventral striatum and posterior cingulate cortex/precuneus are connected with dorsal striatum. Reentrant ventral (limbic), central (associative), and dorsal (motor) corticostriatal loops pass information from ventral-to-dorsal striatum, shifting hedonic processing toward habitual action. Splitting up unexpected occurrences (positive surprise) from non-occurrences (negative surprise) instead of splitting according to valence mirrors the importance of negative surprise in dorsal habitual control which is insensitive to the valence of outcomes. The importance of positive surprise and valence increases toward the rostral–ventral end of the gradient in mPFC and ventrolateral prefrontal cortex. We discuss paradigms that may help to disentangle positive from negative surprise. Moreover, we think that the framework of the functional gradient may help giving various functions in mPFC their place in a larger scheme.
In a recent issue of Nature Neuroscience, Alexander and Brown (2011) presented a computational model that suggests that seemingly diverse medial prefrontal cortex (mPFC) or dorsal anterior cingulate cortex responses may be explained by a single construct, “negative surprise,” which occurs when actions do not produce the expected outcome. The simulation results demonstrated that a single term, not reflecting the valence of the outcome, but reflecting the surprise related to the non-occurrence of a predicted event, can capture a broad range of cognitive control, and performance monitoring effects from various research methodologies. The negative surprise signals consist of rich and context-specific predictions and evaluations.
The model of Alexander and Brown seems compatible with a theory we recently proposed (Tops et al., 2010; Tops and Boksem, 2011). In short, we proposed that two control pathways developed during evolution. The dorsal pathway, including mPFC, dorsolateral prefrontal cortex, posterior cingulate cortex, and precuneus, was adapted to control learning and behavior in high-predictable and stable environments. These systems control behavior in a prospective, feedforward fashion, guided by context models; models that are formed in long term memory by the predictability of the environment/context and kept stable by slow learning. The ventral pathway, including ventrolateral prefrontal cortex [inferior frontal gyrus (IFG) and anterior insula], was adapted to learning and behavior in low-predictable environments. In low-predictable environments, effective context models can not be formed nor used to control behavior in adaptive ways. Instead, behavior is guided reactively by momentary feedback control by environmental stimuli. Ventral corticolimbic control pathways incorporate fast associative learning that is adaptive in low-predictable environments.
Our theory seems related to the well-known hypothesized distinction between ventral and dorsal posterior visual processing streams specialized respectively in processing of “what” and “how” information (Goodale and Milner, 1992). According to Goodale and Milner, the dorsal pathway extracts visual signals relevant for driving automatic or feedforward motor behavior (perception for action), whereas the ventral pathway extracts information relevant for identification and other forms of semantic knowledge. It has been suggested that these processing streams are extended toward frontal ventral and dorsal areas that feed back to implement cognitive control (Sakagami et al., 2006; O’Reilly, 2010). Ventrolateral prefrontal cortex (IFG) mediates active maintenance of stimulus information, and this produces a top-down biasing effect to drive selection and retrieval dynamics in posterior cortex. On the other hand, one should expect to see dorsolateral prefrontal cortex activation whenever the dorsal parietal cortex requires extra cognitive control (such as working memory and top-down biasing) to carry out the processing of sensory information to guide action outputs (O’Reilly, 2010; cf. Sakagami et al., 2006). Although similar, compared to this account our model seems more integrative, associating ventral and dorsal systems with behavioral programs that include motivation, emotion, viscerosensation, and memory (Tops et al., 2010; Tops and Boksem, 2011).
In many situations and for many tasks, dorsal and ventral systems will collaborate and interact in the control of behavior. Alexander and Brown (2011) suggest that negative surprise signals may provide an important reactive control signal to other brain regions to drive a change in strategy when the current behavioral strategy is no longer appropriate. Interestingly, in the same issue of Nature Neuroscience, Thiebaut de Schotten et al. (2011) reported evidence for connections between the parietal component of the ventral attentional network and the prefrontal component of the dorsal network, especially in the right hemisphere. This and other findings (Sridharan et al., 2008) suggest that inputs from right IFG may modulate dorsal networks, redirecting goal-directed, or internally oriented attention mediated by dorsal networks to events identified as salient by the ventral network. Additionally, support has been found for interactions between IFG and dorsal frontal areas, where IFG implements reactive immediate action according to the information conveyed by concomitant input signals, while dorsal frontal areas implement episodic motivation control: sustained control over behavioral episodes according to information conveyed by temporally remote contextual events (Kouneiher et al., 2009).
Functions of mPFC areas may be aspects of ventral or dorsal control, depending on the position along a rostral–ventral to caudal–dorsal gradient within medial cortex. A rostral–ventral to caudal–dorsal gradient was recently found in mPFC, displaying a functional shift from responding to events (errors) of a kind that may trigger the need for “manual” momentary feedback guided control and learning, via feedforward control learning to action selection aspects of more automated action control (Nee et al., 2011). This gradient may mirror the pattern of interconnections between cortex and striatum, as IFG and rostral–ventral mPFC are connected to ventral striatum and posterior cingulate cortex/precuneus are connected with dorsal striatum. Reentrant loops through the ventral striatum terminate in regions of prefrontal cortex that are more dorsal than where they begin, forming ventral (limbic), central (associative), and dorsal (motor) corticostriatal loops through which information can pass from ventral striatum forward into dorsal striatum, and this shift from ventral-to-dorsal striatum is associated with a shift from hedonic processing toward automated, non-hedonic habitual action control (see Figure 1; Alcaro and Panksepp, 2011). High density electroencephalographic source modeling suggests that this shift is also seen in the slow wave during sleep, which propagates from IFG through mPFC to posterior cingulate cortex and precuneus (Murphy et al., 2009).
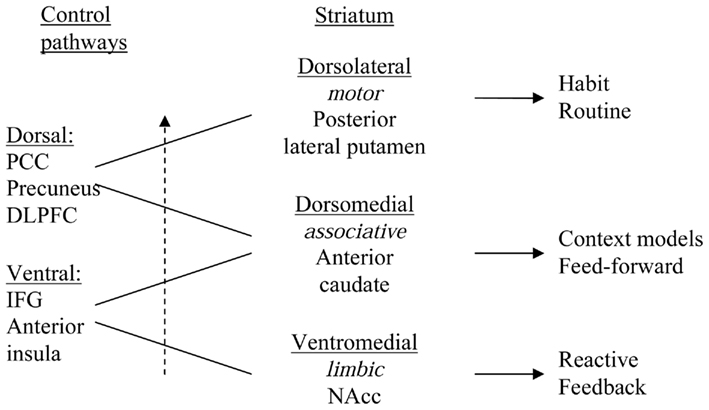
Figure 1. Schematic representation of an interface between ventromedial (limbic), central/dorsomedial (associative), and dorsolateral (motor) striatal regions, via ventrolateral corticolimbic and dorsomedial corticostriatal control pathways and via the midbrain dopamine (DA) cells (ventral tegmental area and substantia nigra, not shown), that forms an ascending spiral between regions. The ventromedial striatum influences the dorsomedial striatum and the dorsomedial striatum influences the dorsolateral striatum. IFG, inferior frontal gyrus (ventrolateral prefrontal cortex); PCC, posterior cingulate cortex; DLPFC, dorsolateral prefrontal cortex; NAcc, nucleus accumbens/ventral striatum.
Information about the integration between striatal regions is obtained from examination of results from multiple retrograde and anterograde tracing experiments, which demonstrate an interface between ventromedial (limbic), central (associative), and dorsolateral (motor) striatal regions via the midbrain dopamine cells (ventral tegmental area and substantia nigra) as well as via cortical areas, that forms an ascending spiral between regions (Haber et al., 2000; cf. Joel and Weiner, 2000). The ventromedial striatum influences the central striatum, and the central striatum influences the dorsolateral striatum. This anatomical arrangement creates a hierarchy of information flow and provides an anatomical basis for the limbic/cognitive/motor interface via the midbrain and cortex (Haber et al., 2000; Joel and Weiner, 2000). Functional connectivity between the cortex and the striatum in a meta-analysis of 126 published functional neuroimaging studies in humans confirmed this pattern (Postuma and Dagher, 2006), as did recent studies (Di Martino et al., 2008; Zhang and Li, 2012).
A similar functional subdivision has previously been proposed between emotional rostral–ventral and cognitive caudal–dorsal (midcingulate) mPFC (Devinsky et al., 1995; Bush et al., 2000). Overviewing the whole cingulate cortex and its striking dichotomy in structure and function, it has been suggested to participate in two distinct parts of the limbic system. A rostral–ventral part engaged in executive functions including those associated with affect and a caudal part involved in visuospatial and memory functions. In this context the rostral limbic system includes the anterior cingulate cortex and ventral striatum, and further the amygdale and septum, anterior insula, IFG, and orbitofrontal cortex. The caudal limbic system includes the posterior cingulate cortex and dorsal striatum, and the hippocampus, and posterior parietal, posterior parahippocampal cortices. Midcingulate cortex would be a transitional region in this conceptualization of two limbic systems (Devinsky et al., 1995).
Functionally, depending on the nature of the events within the learning task, the ventral-to-dorsal shift is seen when learning proceeds from manual, environmental feedback control to more feedforward control and eventually habitual control. Ventral controls “assume” unpredictability (Tanaka et al., 2006) and do not predict temporally distant outcomes. Dorsal controls “assume” predictability and initiate outcome prediction learning. At the same time, monitoring of outcome prediction learning enables estimation of the predictability of the outcome (instrumental contingency), and this calculation of instrumental contingency may be the basis of the subjective experience of control and of selection of more or less automatization for optimal control (Liljeholm et al., 2011). Relatedly, individuals with the greater effect of volatility of predictor–outcome relationships in the mPFC had a higher mean learning rate, and therefore gave more weight to the most recent piece of information (Behrens et al., 2007). The central position of mPFC between reactive ventral control and automated or context model-guided dorsal control suggests a pivotal role in control of learning and behavior, as an interface between the ventral and dorsal systems. Similarly, Luu et al. (2011) suggest that framing the role of the dorsal anterior cingulate cortex as early context-formation to temporarily guide actions, permitting current context to guide learning of new responses in similar situations while supporting the gradual context-updating process that must occur to support skilled performance, may serve as a generic theoretical model that subsumes more specific contemporary theories of mPFC function.
Our theory may explain why, according to the model of Alexander and Brown (Egner, 2011), surprise signals are split up as a function of whether they correspond to unexpected occurrences (positive surprise) or non-occurrences (negative surprise) of action outcomes. Alexander and Brown (2011) concede that positive surprise signals may also be seen in mPFC, but it does not follow from their model why population activity in this region should nevertheless be dominated by negative surprise signals (Egner, 2011). Our theory suggests an explanation. In unpredictable environments, potential threats and rewards are detected through unexpected salient, positive surprise stimuli (“What’s that?”). In contrast, in predictable environments, cognitive control of feedforward, habitual, and/or context model-guided action involves detecting when actions do not produce the predicted outcome (“What went wrong?”). Feedforward action control may further be facilitated by context-specific “predictions and evaluations of multiple likely outcomes that provide a basis for evaluating candidate actions and decisions before execution” (Alexander and Brown, 2011).
The present analysis predicts that Alexander and Brown’s (2011) suggestion of special importance of negative surprise may be true for dorsal control areas where negative surprise reflects the monitoring of habitual action control. Indeed, splitting up of action outcomes according to whether they are unexpected occurrences (positive surprise) or non-occurrences (negative surprise) instead of according to valence, may reflect the importance of negative surprise in dorsal habitual control, as this control is insensitive to the valence of the outcome (Alcaro and Panksepp, 2011). The importance and occurrence of positive surprise as well as valence signals may increase toward the rostral–ventral end of the gradient in mPFC function and toward the IFG. However, negative surprise and positive surprise are correlated – if a strongly predicted event fails to occur (high negative surprise), it follows that the event that did occur will also be very surprising (high positive surprise). We will discuss a few paradigms, such as contingency and instrumental contingency learning (Liljeholm et al., 2011), that may help in disentangling positive from negative surprise.
Our theory suggests that different brain areas should control behavior in future- vs. present moment-focused ways depending on the stability and predictability of the environment. There is some support from human fMRI studies. A study of reward prediction at different time scales showed graded maps of time scale within the right IFG–insula and the striatum: ventroanterior regions were involved in predicting immediate rewards and dorsoposterior striatal regions (and dorsolateral prefrontal cortex, posterior cingulate cortex) were involved in predicting future rewards (Tanaka et al., 2004). A follow-up study showed that the different learning systems in corticostriatal loops are sensitive to the predictability of the environment: the IFG–ventral striatum loop is involved in action learning based on the present state, while the dorsolateral prefrontal cortex–dorsal striatum loop is involved in action learning based on predictable future states (Tanaka et al., 2006).
Another study in monkeys suggests dissociation between prefrontal cortical areas, in which orbitofrontal neurons dynamically evaluate current choices relative to recent choice values, whereas mPFC neurons encode choice predictions and prediction errors using a common valuation currency reflecting the integration of multiple decision parameters (Kennerley et al., 2011). Notice that the evaluation of current choices relative to recent choice values facilitates the detection of stimuli that are motivationally important when action is controlled by a system using only recent information, i.e., proximate to the present moment. The deviation from recent values can be regarded a positive surprise stimulus.
The subjective experience of control or predictability varies with the level of instrumental contingency (Liljeholm et al., 2011), which is the number of contingent outcomes (expected minus negative surprise outcomes) minus the number of non-contingent (positive surprise) outcomes. Liljeholm et al. (2011) found that positive surprise was associated with right IFG and dorsomedial striatum activation. In contrast, contingent outcomes were associated with mPFC and dorsomedial striatum activation. The composite measure of instrumental contingency appeared to be associated with mPFC, middle frontal gyrus, and inferior parietal lobe activation. Notice that in this study the inverse of negative surprise (the proportion of action-following outcomes that were contingent) was actually associated with mPFC activation.
We describe the posterior cingulate cortex and precuneus, which is connected to the dorsolateral striatum and dorsolateral prefrontal cortex, as the dorsal endpoint of the rostral–ventral to caudal–dorsal gradient within medial cortex, and mediators of dorsal context model-guided control. This may seem at odds with proposals that these areas are important in self-reflection and central parts of the default mode network that is active at rest. However, it may be important to realize that self-reflection may be possible only at rest, and when performing habitual actions, as in both states attention is not involved in other processes. Self-reflection may involve activation of memories of the self in contexts (context models). Although during self-reflection retrieval and action control may partly dissociate, true context model-guided control may involve activation of currently relevant context models that facilitate behavioral control guided and informed by previous experiences, involving automated and habitual behaviors. The default mode network has been proposed to support an ability to perform internal mentation by providing a platform for putting together dynamic mental models and scenarios that are largely detached from the specific or current external world (Buckner and Carroll, 2007). Typically, these scenarios would contain elements of auto-biographical episodic memory and self-related prospective thoughts. Further, it has been suggested that the purpose for a continuously on-going internal mentation process is to act as a simulator and predictor of future events that builds upon previous experiences.
Negative surprise is a different concept than negative prediction error in the classical reinforcement learning approach that has previously been applied to understand mPFC function. In our approach as well as in the model of Alexander and Brown (2011), positive and negative surprise are unrelated to valence and can be positively correlated. In contrast, positive and negative reward prediction error in the classical reinforcement learning approach are negatively correlated and form a dimension of valence (Matsumoto et al., 2007; Kennerley et al., 2011). Recently, a valence-based model has been proposed that contains units coding for the value of cues (stimuli or actions) and units coding for the differences between such values and the actual reward (prediction errors; Silvetti et al., 2011). The model reproduced the mPFC behavior of previous single-unit, EEG, and fMRI studies on reward processing, error processing, conflict monitoring, error-likelihood estimation, and volatility estimation, unifying the interpretations of the role performed by the mPFC in some aspects of cognition. It will have to be determined whether this model relates to our theory and how it compares to the model of Alexander and Brown. Moreover, we think that the framework of dorsal and ventral controls and the functional gradient associated with it, may help in giving various functions in mPFC their place in a larger scheme.
Conflict of Interest Statement
The authors declare that the research was conducted in the absence of any commercial or financial relationships that could be construed as a potential conflict of interest.
Acknowledgments
This research was supported by a Veni grant of the Netherlands Organization for Scientific Research (NWO; 451-07-013).
References
Alcaro, A., and Panksepp, J. (2011). The seeking mind: primal neuro-affective substrates for appetitive incentive states and their pathological dynamics in addictions and depression. Neurosci. Biobehav. Rev. 35, 1805–1820.
Alexander, W. H., and Brown, J. W. (2011). Medial prefrontal cortex as an action-outcome predictor. Nat. Neurosci. 14, 1338–1344.
Behrens, T. E., Woolrich, M. W., Walton, M. E., and Rushworth, M. F. (2007). Learning the value of information in an uncertain world. Nat. Neurosci. 10, 1214–1221.
Buckner, R. L., and Carroll, D. C. (2007). Self-projection and the brain. Trends Cogn. Sci. (Regul. Ed.) 11, 49–57.
Bush, G., Luu, P., and Posner, M. I. (2000). Cognitive and emotional influences in anterior cingulate cortex. Trends Cogn. Sci. (Regul. Ed.) 4, 215–222.
Devinsky, O., Morrell, M. J., and Vogt, B. A. (1995). Contributions of anterior cingulate cortex to behaviour. Brain 118, 279–306.
Di Martino, A., Scheres, A., Margulies, D. S., Kelly, A. M., Uddin, L. Q., Shehzad, Z., Biswal, B., Walters, J. R., Castellanos, F. X., and Milham, M. P. (2008). Functional connectivity of human striatum: a resting state FMRI study. Cereb. Cortex 18, 2735–2747.
Egner, T. (2011). Surprise! A unifying model of dorsal anterior cingulate function? Nat. Neurosci. 14, 1219–1220.
Goodale, M. A., and Milner, A. D. (1992). Separate visual pathways for perception and action. Trends Neurosci. 15, 20–25.
Haber, S. N., Fudge, J. L., and McFarland, N. R. (2000). Striatonigrostriatal pathways in primates form an ascending spiral from the shell to the dorsolateral striatum. J. Neurosci. 20, 2369–2382.
Joel, D., and Weiner, I. (2000). The connections of the dopaminergic system with the striatum in rats and primates: an analysis with respect to the functional and compartmental organization of the striatum. Neuroscience 96, 451–474.
Kennerley, S. W., Behrens, T. E., and Wallis, J. D. (2011). Double dissociation of value computations in orbitofrontal and anterior cingulate neurons. Nat. Neurosci. 14, 1581–1589.
Kouneiher, F., Charron, S., and Koechlin, E. (2009). Motivation and cognitive control in the human prefrontal cortex. Nat. Neurosci. 12, 939–945.
Liljeholm, M., Tricomi, E., O’Doherty, J. P., and Balleine, B. W. (2011). Neural correlates of instrumental contingency learning: differential effects of action-reward conjunction and disjunction. J. Neurosci. 31, 2474–2480.
Luu, P., Jiang, Z., Poulsen, C., Mattson, C., Smith, A., and Tucker, D. M. (2011). Learning and the development of contexts for action. Front. Hum. Neurosci. 5:159. doi:10.3389/fnhum.2011.00159
Matsumoto, M., Matsumoto, K., Abe, H., and Tanaka, K. (2007). Medial prefrontal cell activity signaling prediction errors of action values. Nat. Neurosci. 10, 647–656.
Murphy, M., Riedner, B. A., Huber, R., Massimini, M., Ferrarelli, F., and Tononi, G. (2009). Source modeling sleep slow waves. Proc. Natl. Acad. Sci. U.S.A. 106, 1608–1613.
Nee, D. E., Kastner, S., and Brown, J. W. (2011). Functional heterogeneity of conflict, error, task-switching, and unexpectedness effects within medial prefrontal cortex. Neuroimage 54, 528–540.
O’Reilly, R. C. (2010). The what and how of prefrontal cortical organization. Trends Neurosci. 33, 355–361.
Postuma, R. B., and Dagher, A. (2006). Basal ganglia functional connectivity based on a meta-analysis of 126 positron emission tomography and functional magnetic resonance imaging publications. Cereb. Cortex 16, 1508–1521.
Sakagami, M., Pan, X., and Uttl, B. (2006). Behavioral inhibition and prefrontal cortex in decision-making. Neural. Netw. 19, 1255–1265.
Silvetti, M., Seurinck, R., and Verguts, T. (2011). Value and prediction error in medial frontal cortex: integrating the single-unit and systems levels of analysis. Front. Hum. Neurosci. 5:75. doi:10.3389/fnhum.2011.00075
Sridharan, D., Levitin, D. J., and Menon, V. (2008). A critical role for the right fronto-insular cortex in switching between central-executive and default-mode networks. Proc. Natl. Acad. Sci. U.S.A. 105, 12569–12574.
Tanaka, S. C., Doya, K., Okada, G., Ueda, K., Okamoto, Y., and Yamawaki, S. (2004). Prediction of immediate and future rewards differentially recruits cortico-basal ganglia loops. Nat. Neurosci. 7, 887–893.
Tanaka, S. C., Samejima, K., Okada, G., Ueda, K., Okamoto, Y., Yamawaki, S., and Doya, K. (2006). Brain mechanism of reward prediction under predictable and unpredictable environmental dynamics. Neural Netw. 19, 1233–1241.
Thiebaut de Schotten, M. T., Dell’Acqua, F., Forkel, S. J., Simmons, A., Vergani, F., Murphy, D. G., and Catani, M. (2011). A lateralized brain network for visuospatial attention. Nat. Neurosci. 14, 1245–1246.
Tops, M., and Boksem, M. A. S. (2011). A potential role of the inferior frontal gyrus and anterior insula in cognitive control, brain rhythms and event-related potentials. Front. Psychol. 2:330. doi: 10.3389/fpsyg.2011.00330
Tops, M., Boksem, M. A. S., Luu, P., and Tucker, D. M. (2010). Brain substrates of behavioral programs associated with self-regulation. Front. Psychol. 1:152. doi: 10.3389/fpsyg.2010.00152
Keywords: cognitive control, predictability, anterior cingulate cortex, inferior frontal gyrus, prediction error
Citation: Tops M and Boksem MAS (2012) “What’s that?” “What went wrong?” Positive and negative surprise and the rostral–ventral to caudal–dorsal functional gradient in the brain. Front. Psychology 3:21. doi: 10.3389/fpsyg.2012.00021
Received: 26 October 2011;
Accepted: 17 January 2012;
Published online: 03 February 2012.
Edited by:
Tom Verguts, Ghent University, BelgiumReviewed by:
Birte U. Forstmann, University of Amsterdam, NetherlandsMassimo Silvetti, Ghent University, Belgium
Copyright: © 2012 Tops and Boksem. This is an open-access article distributed under the terms of the Creative Commons Attribution Non Commercial License, which permits non-commercial use, distribution, and reproduction in other forums, provided the original authors and source are credited.
*Correspondence: Mattie Tops, Centre for Child and Family Studies, University of Leiden, Post Box 9555, 2300 RB Leiden, Netherlands. e-mail: topsm@fsw.leidenuniv.nl