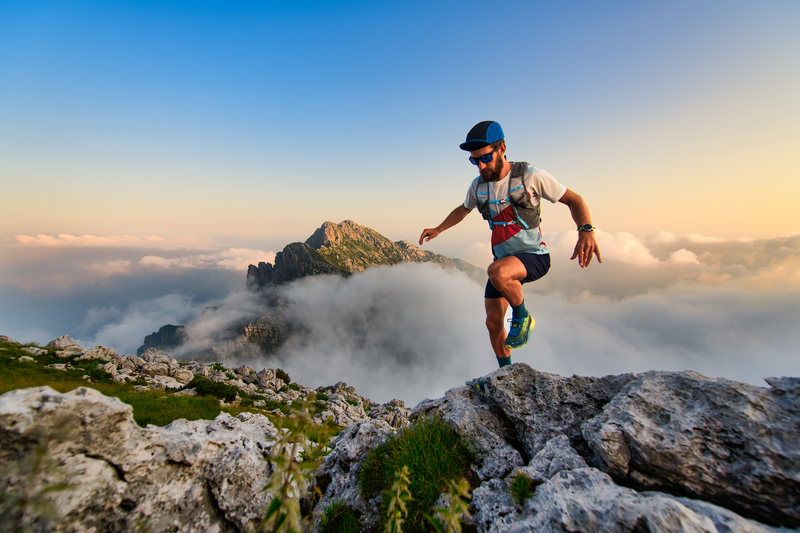
94% of researchers rate our articles as excellent or good
Learn more about the work of our research integrity team to safeguard the quality of each article we publish.
Find out more
HYPOTHESIS AND THEORY article
Front. Psychol. , 10 January 2012
Sec. Consciousness Research
volume 2 - 2011 | https://doi.org/10.3389/fpsyg.2011.00395
We describe a theoretical model of the neurocognitive mechanisms underlying conscious presence and its disturbances. The model is based on interoceptive prediction error and is informed by predictive models of agency, general models of hierarchical predictive coding and dopaminergic signaling in cortex, the role of the anterior insular cortex (AIC) in interoception and emotion, and cognitive neuroscience evidence from studies of virtual reality and of psychiatric disorders of presence, specifically depersonalization/derealization disorder. The model associates presence with successful suppression by top-down predictions of informative interoceptive signals evoked by autonomic control signals and, indirectly, by visceral responses to afferent sensory signals. The model connects presence to agency by allowing that predicted interoceptive signals will depend on whether afferent sensory signals are determined, by a parallel predictive-coding mechanism, to be self-generated or externally caused. Anatomically, we identify the AIC as the likely locus of key neural comparator mechanisms. Our model integrates a broad range of previously disparate evidence, makes predictions for conjoint manipulations of agency and presence, offers a new view of emotion as interoceptive inference, and represents a step toward a mechanistic account of a fundamental phenomenological property of consciousness.
In consciousness science, psychiatry, and virtual reality (VR), the concept of presence is used to refer to the subjective sense of reality of the world and of the self within the world (Metzinger, 2003; Sanchez-Vives and Slater, 2005). Presence is a characteristic of most normal healthy conscious experience. However, theoretical models of the neural mechanisms responsible for presence, and its disorders, are still lacking (Sanchez-Vives and Slater, 2005).
Selective disturbances of conscious presence are manifest in dissociative psychiatric disorders such as depersonalization (loss of subjective sense of reality of the self) and derealization (loss of subjective sense of reality of the world). Depersonalization disorder (DPD), characterized by the chronic circumscribed expression of these symptoms (Phillips et al., 2001; Sierra et al., 2005; Simeon et al., 2009; Sierra and David, 2011), can therefore provide a useful model for understanding presence. In VR, presence is used in a subjective–phenomenal sense to refer to the sense of now being in a virtual environment (VE) rather than in the actual physical environment (Sanchez-Vives and Slater, 2005). These perspectives are complementary: While studies of DPD can help identify candidate neural mechanisms underlying presence in normal conscious experience, studies of VR can help identify how presence can be generated even in situations where it would normally be lacking. Here, we aim to integrate insights into presence from these different perspectives within a single theoretical framework and model.
Our framework is based on interoceptive predictive coding within the anterior insular cortex (AIC) and associated brain regions. Interoception refers to the perception of the physiological condition of the body, a process associated with the autonomic nervous system and with the generation of subjective feeling states (James, 1890; Critchley et al., 2004; Craig, 2009). Interoception can be contrasted with exteroception which refers to (i) perception of the environment via the classical sensory modalities, and (ii) proprioception and kinesthesia reflecting the position and movement of the body in space (Sherrington, 1906; Craig, 2003; Critchley et al., 2004; Blanke and Metzinger, 2009). Predictive coding is a powerful framework for conceiving of the neural mechanisms underlying perception, cognition, and action (Rao and Ballard, 1999; Bubic et al., 2010; Friston, 2010). Simply put, predictive coding models describe counter flowing top-down prediction/expectation signals and bottom-up prediction error signals. Successful perception, cognition and action are associated with successful suppression (“explaining away”) of prediction error. Applied to interoception, predictive coding implies that subjective feeling states are determined by predictions about the interoceptive state of the body, extending the James–Lange, and Schachter–Singer theories of emotion (James, 1890; Schachter and Singer, 1962). Predictive coding models have previously been applied to the sense of agency (the sense that a person’s action is the consequence of his or her intention). Such models propose that disturbances of sensed agency, for example in schizophrenia, arise from imprecise predictions about the sensory consequences of actions (Frith, 1987; Blakemore et al., 2000; Synofzik et al., 2010; Voss et al., 2010). In one line of previous work, Verschure et al. (2003) proposed that presence in a VE is associated with good matches between expected and actual sensorimotor signals, leveraging a prediction-based model of behavior (“distributed adaptive control”; Bernardet et al., 2011). However, to our knowledge, computationally explicit predictive coding models have not been formally applied to presence, nor to interoceptive perceptions. Anatomically, we focus on the AIC because this region has been strongly implicated in interoceptive representation and in the associated generation of subjective feeling states (interoceptive awareness; Critchley et al., 2004; Craig, 2009; Harrison et al., 2010); moreover, AIC activity in DPD is abnormally low (Phillips et al., 2001).
In brief, our model proposes that presence is the result of successful suppression by top-down predictions of informative interoceptive signals evoked (directly) by autonomic control signals and (indirectly) by bodily responses to afferent sensory signals. According to the model, disorders of presence (as in DPD) follow from pathologically imprecise interoceptive predictive signals. The model integrates presence and agency while proposing that they are neither necessary nor sufficient for each other, offers a novel view of emotion as “interoceptive inference,” and is relevant to emerging models of selfhood based on proprioception and multisensory integration. Importantly, the model is testable via novel combinations of VR, neuroimaging, and manipulation of physiological feedback.
The model is motivated by several lines of theory and evidence, including: (i) general models of hierarchically organized predictive coding in cortex, following principles of Bayesian inference (Neal and Hinton, 1998; Lee and Mumford, 2003; Friston, 2009; Bubic et al., 2010); (ii) the importance of insular cortex (particularly the AIC) in integrating interoceptive and exteroceptive signals, and in generating subjective feeling states (Critchley et al., 2002, 2004; Craig, 2009; Harrison et al., 2010); (iii) suggestions and observations of prediction errors in insular cortex (Paulus and Stein, 2006; Gray et al., 2007; Preuschoff et al., 2008; Singer et al., 2009; Bossaerts, 2010); (iv) evidence of abnormal insula activation in DPD (Phillips et al., 2001; Sierra and David, 2011); (v) models of the subjective sense of “agency” (and its disturbance in schizophrenia) framed in terms of predicting the sensory consequences of self-generated actions (Frith, 1987, 2011; Synofzik et al., 2010; Voss et al., 2010); and (vi) theory and evidence regarding the role of dopamine in signaling prediction errors and in optimizing their precision (Schultz and Dickinson, 2000; Fiorillo et al., 2003; Friston et al., 2006; Fletcher and Frith, 2009).
In the remainder of this paper, we first define the concept of presence in greater detail. We then introduce the theoretical model before justifying its components with reference to each of the areas just described. We finish by extracting from the model some testable predictions, discussing related modeling work, and noting some potential challenges.
The concept of presence has emerged semi-independently in different fields (VR, psychiatry, consciousness science, philosophy) concerned with understanding basic features of normal and abnormal conscious experience. The concepts from each field partially overlap. In VR, presence has both subjective–phenomenal and objective–functional interpretations. In the former, presence is understood as the sense of now being in a VE while transiently unaware of one’s real location and of the technology delivering the sensory input and recording the motor output (Jancke et al., 2009); a more compact definition is simply “the sense of being there” (Lombard and Ditton, 1997) or “being now there” (Metzinger, 2003). The objective interpretation is based on establishing a behavioral/functional equivalence between virtual and real environments: “the key to the approach is that the sense of “being there” in a VE is grounded on the ability to “do there”” (Sanchez-Vives and Slater, 2005; p.333). In this paper we focus on the former interpretation as most relevant to the phenomenology of presence.
Within psychiatry, presence is often discussed with reference to its disturbance or absence in syndromes such as DPD and early (prodromal) stages of psychoses. A useful characterization of DPD is provided by Ackner (1954): “a subjective feeling of internal and/or external change, experienced as one of strangeness or unreality.” A common description given by DPD patients is that their conscious experiences of the self and the world have an “as if” character; the objects of perception seems unreal and distant, or unreachable “as if” behind a mirror or window. DPD patients do not normally suffer delusions or hallucinations, marking a clear distinction from full-blown psychoses such as schizophrenia; however, it is increasingly recognized that symptoms of DPD may characterize prodromal stages of psychosis (Moller and Husby, 2000) potentially providing diagnostic, prognostic, and explanatory value. There is a clear overlap between the usages of presence in DPD and VR in picking out the subjective feeling of “being there.” In the former case the sense of “being there” is lost, and in the latter, its generation is desired. More generally, presence can be considered as a constitutive property of conscious experience. Following Metzinger, a “temporal window of presence” can be understood as precipitating a subjective conscious “now” from the flow of objective time (Metzinger, 2003). Metzinger further connects the concept of presence to that of transparency, which refers to the fact that our perceptions of the world and of the self appear direct, unmediated by the neurocognitive mechanisms that in fact give rise to them. Here, we do not treat explicitly the temporal aspect of presence, and transparency and presence are treated synonymously. Considered this way, although presence can vary in its intensity, it is a characteristic of conscious experiences generally and not an instance of any specific conscious experience (e.g., an experience of a red mug); in other words, presence can be considered to be a “structural property” of consciousness (Seth, 2009).
Considering these perspectives together, there is a natural ambiguity about whether it is presence itself, or its absence in particular conditions, that is the core phenomenological explanatory target. However, in either case it remains necessary to formulate a model describing the relevant neurocognitive constraints. We now introduce such a model.
Figure 1 depicts the functional architecture of the proposed model. It consists of two primary components, an “agency component” and a “presence component,” mutually interacting according to hierarchical Bayesian principles and connected, respectively, with a sensorimotor system and an autonomic/motivational system. Each main component has a “state module” and an “error module.” The core concept of the model is that a sense of presence arises when informative interoceptive prediction signals are successfully matched to inputs so that prediction errors are suppressed. It is not sufficient simply for there to be zero interoceptive prediction error signals, as could happen for example in the absence of any interoceptive signals allowing a simple prediction of “no signal.” Rather, presence depends on a match between informative interoceptive signals and top-down predictions arising from a dynamically evolving brain–body–world interaction. The same considerations apply as well to the agency component.
Figure 1. An interoceptive predictive coding model of conscious presence. Both agency and presence components comprise state and error units; state units generate control signals (Aout, Pout) and make predictions [Apred, Ppred, Apred(p)] about the consequent incoming signals (Ain, Pin); error units compare predictions with afferents, generating error signals [Aerr, Perr, Aerr(p)]. In the current version of the model the agency component is hierarchically located above the presence component, so that it generates predictions about the interoceptive consequences of sensory input generated by motor control signals.
The agency component is based on Frith’s well-established “comparator model” of schizophrenia (Frith, 1987, 2011; Blakemore et al., 2000), recently extended to a Bayesian framework (Fletcher and Frith, 2009). In the state module of this component, motor signals are generated which influence the sensorimotor system (Aout); these motor signals are accompanied by prediction signals (Apred) which attempt to predict the sensory consequences of motor actions via a forward model informed by efference copy and/or corollary discharge signals (Sommer and Wurtz, 2008). Predicted and afferent sensory signals are compared in the error module, generating a prediction error signal Aerr. In this model, the subjective sense of agency depends on successful prediction of the sensory consequences of action, i.e., suppression or “explaining away” of the exteroceptive prediction error Apred. Following previous models (Fletcher and Frith, 2009; Synofzik et al., 2010), disturbances in sensed agency arise not simply from predictive mismatches, but from pathologically imprecise predictions about the sensory consequences of action. Predictive coding schemes by necessity involve estimates of precision (or inverse variance) since prediction errors per se are otherwise meaningless. Experimentally, it has been shown that imprecise predictions prompt patients to rely more strongly on (and therefore adapt more readily to) external cues, accounting for a key feature of schizophrenic phenomenology in which actions are interpreted as having external rather than internal causes (Synofzik et al., 2010). The precision of prediction error signals has been associated specifically with dopaminergic activity (Fiorillo et al., 2003), suggesting a proximate neuronal origin of schizophrenic symptomatology in terms of abnormal dopaminergic neurotransmission (Fletcher and Frith, 2009). Prediction error precision also features prominently in recent models of hierarchical Bayesian networks, discussed in Section “Prediction, Perception, and Bayesian Inference” (Friston et al., 2006; Friston, 2009).
In the presence component, the autonomic system is driven both by afferent sensory signals and by internally generated control signals from the state module (Pout), modulating the internal physiological milieu. The state module is responsible for the generation of subjective emotional (feeling) states in accordance with the principles of James and Lange, i.e., that subjective feelings arise from perceptions of bodily responses to emotive stimuli (Critchley et al., 2004; Craig, 2009) or equally, in accordance with the Schachter and Singer model of emotion in which emotional feelings arise through interpretation of interoceptive arousal signals within a cognitive context (e.g., Schachter and Singer, 1962; Critchley et al., 2002). Extending these principles, in our model emotional content is determined by the nature of the predictive signals Ppred, and not simply by the “sensing” of interoceptive signals per se (i.e., we apply the Helmholtzian perspective of perception as inference to subjective feeling states, see Interoception As Inference: A New View of Emotion?). As in the agency component, there is also an error module which compares predicted interoceptive signals with actual interoceptive signals Pin via a forward model giving rise to an interoceptive prediction error Perr (Paulus and Stein, 2006). In our model, the sense of presence is underpinned by a match between informative predicted and actual interoceptive signals; disturbances of presence, as in DPD, arise because of disturbances in this predictive mechanism. Again, by analogy with the agency component (Fletcher and Frith, 2009; Synofzik et al., 2010) we propose that these disturbances arise because of imprecise prediction signals Ppred.
In our model, the presence and agency components are interconnected. Importantly, this connection is not just analogical (i.e., justified with respect to shared predictive principles) but is based on several lines of evidence. First, disorders of agency and presence often (but not always) co-occur (Robertson, 2000; Sumner and Husain, 2008; Ruhrmann et al., 2010; Sierra and David, 2011; see Summary). Second, manipulations of perceived agency can influence reported presence, as shown in both healthy subjects and schizophrenic patients (Lallart et al., 2009; Gutierrez-Martinez et al., 2011). Third, as discussed below, abundant evidence points to interactions between interoceptive and exteroceptive processes, which in our model mediate interactions between the agency and presence components. In the present version of the model, agency is functionally localized at a higher hierarchical level than presence, such that the agency state module generates both sensorimotor predictions (Apred) and interoceptive predictions [Apred(p)]; correspondingly, interoceptive prediction error signals are conveyed to the agency state module [Aerr(p)] as well as to the presence state module. This arrangement is consistent with evidence showing that reported presence is modulated by perceived agency (Lallart et al., 2009; Gutierrez-Martinez et al., 2011). Interestingly, in this arrangement an additional generative component is needed to generate predictive interoceptive signals given the current state of both agency and presence components. We speculate that this integrative generative model may be a key component of a core sense of selfhood, in line with recent hierarchical models of the self (Northoff and Bermpohl, 2004; Feinberg, 2011) including those based on perceptual aspects of global body ownership (Blanke and Metzinger, 2009).
As just mentioned, a connection between presence and agency mechanisms, whether hierarchical or reciprocal, in our model requires interacting interoceptive and exteroceptive processes. Theory and evidence regarding such interactions have a long history, extending back at least as far as James (1890) and prominent in modern neural theories of consciousness (e.g., Edelman, 1989; Humphrey, 2006; Craig, 2009). Consistent with our model, interoceptive responses have recently been argued to shape predictive inference during visual object recognition via affective predictions generated in the orbitofrontal cortex (Barrett and Bar, 2009). Intriguingly, susceptibility to the rubber-hand illusion (Botvinick and Cohen, 1998) is anticorrelated with interoceptive sensitivity (as measured by a heartbeat detection task; Tsakiris et al., 2011), suggesting an interaction between predictive models of body ownership and interoception. People with lower interoceptive predictive ability may more readily assimilate exteroceptive (e.g., correlated visual and tactile) cues in localizing interoceptive and proprioceptive signals, while people with good interoceptive predictive ability may rely less on these exteroceptive cues. Strikingly, rubber-hand illusory experiences are associated with cooling of the real hand, indicating an interaction between predictive mechanisms and autonomic regulation (Moseley et al., 2008).
Despite the connection proposed between agency and presence, our model implies that perceived agency is neither necessary nor sufficient for presence, and vice versa. This position is consistent with evidence that (i) experimental manipulations of perceived agency need not evoke changes in autonomic responses such heart rate and skin conductance (David et al., 2011), (ii) these autonomic signals need not correlate with judgments of agency (David et al., 2011), and (iii) as already mentioned, disorders of agency and presence do not always co-occur (see Disorders of Agency and Presence and Summary).
The model implicates a broad network of brain regions for both the agency and the presence components. Neural correlates of the sense of agency have been studied extensively, primarily by manipulating spatial or temporal delays to induce exteroceptive predictive mismatches. Regions identified include motor areas (ventral premotor cortex, supplementary, and pre-supplementary motor areas and basal ganglia), the cerebellum, the posterior parietal cortex, the posterior temporal sulcus, subregions of the prefrontal cortex, and the anterior insula (Haggard, 2008; Tsakiris et al., 2010; Nahab et al., 2011). Among these areas the pre-supplementary motor area plays a key role in implementing complex, open decisions among alternative actions and has been suggested as a source of the so-called “readiness potential” identified in the classic experiments of Libet on volition (Haggard, 2008). The right angular gyrus of the inferior parietal cortex, and more generally the temporo-parietal-junction, are associated specifically with awareness of the discrepancy between intended and actual movements (Farrer et al., 2008; Miele et al., 2011) and have been implicated in multisensory integration underlying exteroceptive aspects of global body ownership relevant to selfhood (Blanke and Metzinger, 2009).
The presence component also implicates a broad neural substrate. We suggest that areas contributing to interoceptive predictive coding include specific brainstem (nucleus of the solitary tract, periaqueductal gray, locus coeruleus), subcortical (substantia innominata, nucleus accumbens, amygdala), and cortical (insular, orbitofrontal, and anterior cingulate) regions, potentially forming at least a loose hierarchy (Critchley et al., 2004; Tamietto and de Gelder, 2010). Among these areas, the insular cortex appears central to the integration of interoceptive and exteroceptive signals and to the generation of subjective feeling states. The posterior and mid insula support the primary cortical representation of interoceptive signals (Critchley et al., 2002, 2004; Harrison et al., 2010), with the anterior insula (AIC) operating as a comparator or error module (Paulus and Stein, 2006; Preuschoff et al., 2008; Palaniyappan and Liddle, 2011). Interestingly, the AIC is also differentially activated by changes in the sense of agency (Tsakiris et al., 2010; Nahab et al., 2011), supporting a link between mechanisms underlying agency and presence.
Autonomic control signals Pout are suggested to originate in regions of anterior cingulate cortex (ACC) which can be interpreted as “visceromotor cortex” for their function in the autonomic modulation of bodily arousal to meet behavioral demand (Pool and Ransohoff, 1949; Critchley et al., 2003; Critchley, 2009). Equally, during motor behavior, premotor, supplementary, and primary motor cortices are direct generators of autonomic vascular changes through central command (Delgado, 1960) and a parallel, partly reciprocal, system of antisympathetic and parasympathetic efferent drive operates through subgenual cingulate and ventromedial prefrontal cortex (Nagai et al., 2004; Critchley, 2009; Wager et al., 2009). The neural basis of interoceptive prediction signals Ppred is suggested to overlap with these control mechanisms, with emphasis on the ACC and the orbitofrontal cortex. The ACC has been associated with autonomic “efference copy” signals (Harrison et al., 2010) and medial sectors of the orbitofrontal cortex have robust connections with limbic, hypothalamic, midbrain, brainstem, and spinal cord areas involved in internal state regulation (Barbas, 2000; Barbas et al., 2003; Barrett and Bar, 2009). It is noteworthy that ventromedial prefrontal including medial orbital cortices also support primary and abstract representations of value and reward across modalities (Grabenhorst and Rolls, 2011).
The AIC and the ACC (see Figure 2) are often coactivated despite being spatially widely separated, forming a “salience network” in conjunction with the amygdala and the inferior frontal gyrus (Seeley et al., 2007; Medford and Critchley, 2010; Palaniyappan and Liddle, 2011). The AIC and ACC are known to be functionally (Taylor et al., 2009) and structurally (van den Heuvel et al., 2009) connected. Interestingly, Craig has suggested that AIC–ACC connections are mediated via their distinctive populations of von Economo neurons, which have rapid signal propagation properties and are rich in dopamine D1 receptors (Hurd et al., 2001; Craig, 2009). These areas have also been broadly implicated in representations of reward expectation and reward prediction errors in reinforcement learning contexts (see Disorders of Agency and Presence and Rushworth and Behrens, 2008). A recent model of medial prefrontal cortex, and especially ACC, proposes that competing accounts treating error likelihood, conflict, and volatility and reward can be unified by a simple scheme involving population-based predictions of action–outcome pairings, whether good or bad (Alexander and Brown, 2011). ACC responses are also modulated by the effort associated with an expected reward (Croxson et al., 2009), implicating agency. These observations provide further support for considering the salience network as a central neural substrate of our model.
Figure 2. The human cingulate (red) and insular (blue) cortices. Image generated using Mango (http://ric.uthscsa.edu/mango/).
The human insular cortex is a large and highly interconnected structure, deeply embedded in the brain (see Figure 2; Augustine, 1996; Medford and Critchley, 2010; Deen et al., 2011). The insula has been divided into several subregions based on connectivity and cytoarchitectonic features (Mesulam and Mufson, 1982a,b; Mufson and Mesulam, 1982; Deen et al., 2011), with all subregions implicated in visceral representation. Posterior and mid insula support a primary representation of interoceptive information, relayed from brainstem centers, notably the nucleus of the solitary tract, which receives convergent visceral afferent inputs from cranial nerves, predominantly vagus and glossopharyngeal nerves (Mesulam and Mufson, 1982b), and spinal cord, particularly the lamina-1 spinal tract (Craig, 2002). Blood-borne afferent signals may also reach posterior insula via the solitary nucleus due to its interaction with the area postrema (Shapiro and Miselis, 1985). A secondary (re-)representation of interoceptive information within AIC is proposed to arise from forward flow of information from posterior and mid insular cortices (Craig, 2002), augmented by direct input from ventroposteriomedial thalamus. Bidirectional connections with amygdala, nucleus accumbens, and orbitofrontal cortex further suggest that the AIC is well placed to receive input about (positive and negative) stimulus salience (Augustine, 1996). Generally, AIC is considered as the principal cortical site for the integration of interoceptive and exteroceptive signals.
The AIC is engaged across a wide range of processes that share as a common factor visceral representation, interoception, and emotional experience (Craig, 2002, 2009; Critchley et al., 2004; Singer et al., 2009). The AIC is proposed to instantiate interoceptive representations that are accessible to conscious awareness as subjective feeling states (Critchley et al., 2004; Singer et al., 2009). Evidence for this view comes in part from a study in which individual differences in interoceptive sensitivity, as measured by heartbeat detection, could be predicted by AIC activation and morphometry (better performance associated with higher activation and higher gray matter volume) which in turn accounted for individual differences in reported emotional symptoms. These observations suggest a role for AIC both in interoceptive awareness and in the generation of associated emotional feeling states (Critchley et al., 2004; though see Khalsa et al., 2009 who show that the AIC is not necessary for interoceptive sensitivity). Close topographical relationships between different qualities of subjective emotional experience and differences in visceral autonomic state have subsequently been reported within insula subregions (Harrison et al., 2010). Also, the AIC is activated by observation, experience, and imagination of a strong emotion (disgust), though with different functional connectivity patterns in each case (Jabbi et al., 2008). Most generally, Craig (2009) suggests the AIC as a “central neural correlate of consciousness,” drawing additional attention to its possible role in the perception of flow of time.
Taken together, the evidence summarized so far underscores AIC involvement in interoceptive processing, its contribution (in particular with the ACC) to a wider salience network and its role in the integration of exteroceptive signals with stimulus salience. These processes within AIC appear to underlie subjective feeling states. Consistent with this interpretation, we propose AIC to be a comparator underlying the sense of presence. Specific support for our model includes (i) evidence for predictive coding in the AIC; (ii) hypoactivation of AIC in patients with DPD, and (iii) modulation of AIC activity by reported subjective presence in VR experiments. Before turning to this evidence we next discuss the principles of predictive coding in more detail.
Following the early insights of von Helmholtz, there is now increasing recognition of the importance of prediction, and prediction error, in perception, cognition, and action (Hinton and Dayan, 1996; Rao and Ballard, 1999; Lee and Mumford, 2003; Egner et al., 2008; Friston, 2009; Summerfield and Egner, 2009; Bubic et al., 2010; Mathews et al., 2012). The concept of “predictive coding” overturns classical notions of perception as a largely bottom-up process of evidence-accumulation or feature-detection driven by impinging sensory signals, proposing instead that perceptual content is determined by top-down predictive signals arising from multi-level generative models of the external causes of sensory signals, which are continually modified by bottom-up prediction error signals communicating mismatches between predicted and actual signals across hierarchical levels (see Figure 3). In this view, even low-level perceptual content is determined via a cascade of predictions flowing from very general abstract expectations which constrain successively more detailed (fine-grained) predictions. We emphasize that in these frameworks bottom-up/feed-forward signals convey prediction errors, and top-down/feed-back signals convey predictions determining content. The great power of predictive coding frameworks is that they formalize the concept of inductive inference, just as classical logic formalizes deductive inference (Dorling, 1982; Barlow, 1990).
Figure 3. A schematic of hierarchical predictive coding across three cortical regions; the “lowest” (R1) on the left and the “highest” (R3) on the right. Light blue cells represent state units, orange cells represent error units. Note that predictions and prediction errors are sent and received from each level in the hierarchy. Feed-forward signals conveying prediction errors originate in superficial layers and terminate in deep (infragranular) layers of their targets, are associated with gamma-band oscillations, and are mediated by GABA and fast AMPA receptor kinetics. Conversely, feedback signals conveying predictions originate in deep layers and project to superficial layers, are associated with beta-band oscillations, and are mediated by slow NMDA receptor kinetics. Adapted from (Friston, 2009; see also Wang, 2010).
Predictive coding models are now well-established in accounting for various features of perception (Rao and Ballard, 1999; Yuille and Kersten, 2006), cognition (Grush, 2004), and motor control (Wolpert and Ghahramani, 2000) (see Bubic et al., 2010 for a review). Two examples from visual perception are worth highlighting. In an early study (Rao and Ballard, 1999) implemented a model of visual processing utilizing a predictive coding scheme. When exposed to natural images, simulated neurons developed receptive-field properties observed in simple visual cells (e.g., oriented receptive-fields) as well as non-classical receptive-field effects such as “end-stopping.” These authors pointed out that predictive coding is computationally and metabolically efficient since neural networks learn the statistical regularities embedded in their inputs, reducing redundancy by removing the predictable components of afferent signals and transmitting only residual errors. More recently, Egner and colleagues elegantly showed that repetition suppression (decreased cortical responses to familiar stimuli) is better explained by predictive coding than by alternative explanations based on adaptation or sharpening of representations. Their key finding is that repetition suppression can be abolished when the local likelihood of repetitions is manipulated so that repetitions become unexpected (Egner et al., 2008).
Theoretically, computational accounts of predictive coding have now reached high levels of sophistication (Dayan et al., 1995; Hinton and Dayan, 1996; Rao and Ballard, 1999; Lee and Mumford, 2003; Friston et al., 2006; Friston, 2009). These accounts leverage the hierarchical organization of cortex to show how generative models underlying top-down predictions can be induced empirically via hierarchical Bayesian inference. Bayesian methods provide a computational mechanism for estimating the probable causes of data (posterior distribution) given the observed conditional probabilities of the data and associated priors; in other words, Bayes’ theorem relates a conditional probability (which can be observed) to its inverse (which cannot be observed, but knowledge of which is desired).
As illustrated in Figure 3, in these models each layer attempts to suppress activity in the layer immediately below, as well as within the same layer, and each layer passes prediction errors related to its own activity both internally and to the layer immediately above. From a Bayesian perspective, top-down influences constitute empirically induced priors on the causes of their input. Advances in machine learning theory based on hierarchical Bayesian inference (Dayan et al., 1995; Neal and Hinton, 1998; Lee and Mumford, 2003; Friston et al., 2006; Friston, 2009) show how these schemes may operate in practice. Recent attention has focused on Friston’s “free energy” principle (Friston et al., 2006; Friston, 2009) which, following earlier work by Hinton and colleagues (e.g., Hinton and Dayan, 1996; Neal and Hinton, 1998), shows how generative models can be hierarchically induced from data by assuming that the brain minimizes a bound on the evidence for a model of the data. The machine learning algorithms able to perform this minimization are based on so-called “variational Bayes” worked out by (Neal and Hinton, 1998) among others; these algorithms have plausible neurobiological implementations, at least in cortical hierarchies (Hinton and Dayan, 1996; Lee and Mumford, 2003; Friston et al., 2006; Friston, 2009).
Interestingly, the precision of prediction error signals plays a key role in these models on the grounds that hierarchical models of perception require optimization of the relative precision of top-down predictions and bottom-up evidence (Friston, 2009). This process corresponds to modulating the gain of error units at each level, implemented by neuromodulatory systems. While for exteroception this may involve cholingeric neurotransmission via attention (Yu and Dayan, 2005); for interoception, proprioception, and value-learning, prediction error precision is suggested to be encoded by dopamine (Fiorillo et al., 2003; Friston, 2009). The role of dopamine in our model is discussed further in Section “The Role of Dopamine.”
It is important to emphasize that in predictive coding frameworks, predictions and prediction errors interact over rapid (synchronic) timescales providing a constitutive basis for the corresponding perceptions, cognitions, and actions. This timescale is distinct from the longer (diachronic) timescales across which the brain might learn temporal relations among stimuli (Schultz and Dickinson, 2000), or form expectations about the timing and nature of future events (Suddendorf and Corballis, 2007).
In summary, predictive coding may capture a general principle of cortical functional organization. It fluently explains a broad range of evidence (though a key prediction, that of distinct “state” and “error” neurons in different cortical laminae, remains to be established) and has attractive computational properties, at least in the context of visual perception. It has been applied to agency, where by extending Frith’s comparator model it suggests that disorders of agency arise from pathologically imprecise predictions about the sensory consequences of self-generated actions. However the framework has not yet been formally applied to interoception or to presence.
Predictive coding models of interoceptive processing have not yet been elaborated. Such a model forms a key component of our model of presence, offering a starting point for predictive models of interoception and emotion generally.
Interoceptive concepts of emotion were first crystallized by James and Lange who argued that emotions arise from perception of physiological changes in the body. This basic idea has been influential over the last century, underpinning more recent frameworks for understanding emotion such as the “somatic marker hypothesis” of Damasio (2000), the “sentient self” model (Craig, 2002, 2009), and “interoceptive awareness” (Critchley et al., 2004). Despite the advances embedded in these frameworks, interoception remains generally understood along “feed-forward” lines, similar to classical feature-detection or evidence-accumulation theories of visual perception. However, it has long been recognized that cognitively explicit beliefs about the causes of physiological changes can influence subjective feeling states (Cannon, 1915). Some 50 years ago, Schachter and Singer (1962) famously demonstrated that injections of adrenaline, proximally causing a variety of significant physiological changes, could give rise to either anger or elation depending on the concurrent context (an irritated or elated confederate), an observation formalized in their “two factor” theory in which subjective emotions are determined by a combination of cognitive factors and physiological conditions.
Though it involves expectations, Schachter and Singer’s theory falls considerably short of a full predictive coding model of emotion. Drawing a parallel with models of perception, predictive interoception would involve hierarchically cascading top-down interoceptive predictions counter flowing with bottom-up interoceptive prediction errors, with subjective feeling states being determined by the joint content of the top-down predictions across multiple hierarchical levels. In other words, according to the model emotional content is determined by a suite of hierarchically organized generative models predicting interoceptive responses to external stimuli and/or internal physiological control signals (Figure 4).
Figure 4. Predictive coding applied to interoception. Motor control and autonomic control signals evoke interoceptive responses [intero(actual)] either directly (autonomic control) or indirectly via the musculoskeletal system and the environment (motor control). These responses are compared to predicted responses [intero(pred)], which are generated by hierarchically organized forward/generative models informed by motor and autonomic efference copy signals. The comparison, which may take place in AIC, generates a prediction error which refines the generative models. Subjective feeling states are associated with predicted interoceptive signals intero(pred). The figure is adapted from a general schematic of predictive coding in (Bubic et al., 2010).
It is important to distinguish interoceptive predictive coding from more generic interactions between prediction and emotion. As already mentioned, predictive coding involves prediction at synchronic, fast time-scales, such that predictions (and prediction errors) are constitutive of (emotional) content. Approaching this idea, Barrett and Bar (2009) propose that affective (interoceptive) predictions shape visual object recognition at fast timescales, however they do not contend that such predictions are the constitutive basis of emotions in the full predictive coding sense. Many previous studies have examined how predictions can influence emotion over longer, diachronic, timescales (Ploghaus et al., 1999; Porro et al., 2003; Ueda et al., 2003; Gilbert and Wilson, 2009); the brain networks involved in emotional predictions across time reliably include prefrontal cortex and the ACC.
A key requirement of our model is that the AIC participates in interoceptive predictive coding. In a related influential model of anxiety, Paulus and Stein (2006) suggest that insular cortex compares predicted to actual interoceptive signals, with subjective anxiety associated with heightened interoceptive prediction error signals. In line with their model, highly anxious individuals show increased AIC activity during emotion processing (Paulus and Stein, 2006). AIC responses to stimuli are modulated by expectations: When participants are exposed to a highly aversive taste, while falsely expecting only a moderately aversive taste, they report less aversion than when having accurate information about the stimulus, with corresponding attenuation of evoked activity within AIC (and adjacent frontal operculum; Nitschke et al., 2006). Moreover, AIC responses to expected aversive stimuli are larger if expectations are uncertain (Sarinopoulos et al., 2010). The AIC is also activated by anticipation of painful (Ploghaus et al., 1999) and tactile stimuli (Lovero et al., 2009). Direct experimental evidence of insular predictive coding, though not specifically regarding interoceptive signals, comes from an fMRI study of a gambling task in which activity within spatially separate subregions of the AIC encoded both predicted risk and risk prediction error (Preuschoff et al., 2008). The risk prediction error signal exhibits a fast onset, whereas the risk prediction signal (localized to a slightly more superior and anterior AIC subregion) exhibits a slow onset; these dynamics are consistent with respective bottom-up and top-down origins in predictive coding frameworks (Preuschoff et al., 2008).
Consistent with the above findings, during performance of the Iowa gambling task, AIC responses reflect risk prediction error while striatal responses reflect reward prediction errors (d’Acremont et al., 2009). During more classical instrumental learning both AIC and striatal responses reflect reward prediction error signals where, in contrast to striatal activity, AIC responses correlate negatively with reward prediction error and during “loss” trials only, possibly reflecting aversive prediction error (Pessiglione et al., 2006). Risk, reward, and interoception are clearly closely linked, as underlined by theories of decision-making and associated empirical data that emphasize the importance of internal physiological responses in shaping apparently rational behavior (Bechara et al., 1997; Damasio, 2000). These links are also implied by the structural and functional interconnectivity of AIC with the ACC and with orbitofrontal cortex and other reward-related and decision-making structures (see The Insular Cortex, Interoception, and Emotion).
Anterior insular cortex responses are implicated in other prediction frameworks: AIC responses occur for conscious but not unconscious errors made in an antisaccade task (Klein et al., 2007). The AIC is proposed to be specifically involved in updating previously existing prediction models in reward learning contexts (Palaniyappan and Liddle, 2011), and AIC activity elicited during intentional action is suggested to provide interoceptive signals essential for evaluating the affective consequences of motor intentions (Brass and Haggard, 2010). This view aligns with our model in emphasizing a connection between agency and presence.
A different source of evidence for interoceptive predictive coding comes from exogenous manipulations of interoceptive feedback. The experimental induction of mismatch between predicted and actual interoceptive signals by false physiological feedback enhances activation of right AIC (Gray et al., 2007), showing the region to be a comparator. Moreover, this AIC activation, in conjunction with amygdala, is associated with an increased emotional salience attributed to previously unthreatening stimuli, consistent with revision of top-down interoceptive predictions in the face of unexplained error (Gray et al., 2007).
In summary, there is accumulating evidence for predictive signaling in AIC relevant to risk and reward, as well as limited evidence for interoceptive predictive coding arising from false feedback evidence. Direct evidence for interoceptive predictive coding in the AIC has not yet been obtained and stands as a key test of the present model.
A useful model should be able to account for features of relevant disorders. As discussed, schizophrenic delusions of control are well explained by the comparator model of agency in terms of problems with kinematic and sensory aspects of the forward modeling component (Frith, 2011). Specifically, reduced precision of exteroceptive predictions coincides with greater delusions of control, consistent with abnormal dopaminergic neurotransmission (Synofzik et al., 2010; see also The Role of Dopamine). Other first-rank symptoms, for example thought insertion, are however less well accounted for by current comparator models (Frith, 2011). Here, we focus on the less extensively discussed disorders of presence.
Depersonalization and derealization symptoms manifest as a disruption of conscious experience at very basic, preverbal level, most colloquially as a “feeling of unreality” which can be equally interpreted as the absence of normal feelings of presence (American Psychiatric Association, 2000). Depersonalization and derealization are common as brief transient phenomena in healthy individuals, but may occur as a chronic disabling condition, either as a primary disorder, DPD, or secondary to other neuropsychiatric illness such as panic disorder, post-traumatic stress disorder, and depression. Recent surveys of clinical populations suggest that depersonalization/derealization may be the third most common psychiatric symptom after anxiety and low mood (Stewart, 1964; Simeon et al., 1997), and are experienced by 1.2–2% of the general population in any given month (Bebbington et al., 1997; Hunter et al., 2004). The chronic expression of these symptoms in DPD is characterized by “alteration in the perception or experience of the self so that one feels detached from and as if one is an outside observer of one’s own mental processes”(American Psychiatric Association, 2000). Two recent studies of DPD phenomenology have shown that the condition is best considered a syndrome, as chronic depersonalization involves qualitative changes in subjective experience across a range of experiential domains (Sierra et al., 2005; Simeon et al., 2008), encompassing abnormalities of bodily sensation and emotional experience. Notably, DPD is often accompanied by alexithymia, which refers to a deficiency in understanding, processing, or describing emotions; more generally a deficiency of conscious access to subjective emotional states (Simeon et al., 2009). In short, DPD can be summarized as a psychiatric condition marked by the selective diminution of the subjective reality of the self and world; a presence deficit.
Neuroimaging studies of DPD, though rare, reveal significantly lower activation in AIC (and bilateral cingulate cortex) as compared to normal controls when viewing aversive images (Phillips et al., 2001). It has been suggested that DPD is associated with a suppressive mechanism grounded in fronto-limbic brain regions, notably the AIC, which “manifests subjectively as emotional numbing, and disables the process by which perception and cognition become emotionally colored, giving rise to a subjective feeling of unreality”(Sierra and David, 2011). This mechanism may therefore also underlie comorbid alexithymia.
In our model, DPD symptoms correspond to abnormal interoceptive predictive coding dynamics. Whereas anxiety has been associated with heightened prediction error signals (Paulus and Stein, 2006), we suggest that DPD is associated with imprecise interoceptive prediction signals Ppred in analogy with predictive models of disorders of agency (Fletcher and Frith, 2009; Synofzik et al., 2010). Our model therefore extends that of Paulus and Stein (2006): Chronically high anxiety may result from chronically elevated interoceptive prediction error signals, leading to overactivation in AIC as a result of inadequate suppression of these signals. In contrast, the imprecise interoceptive prediction signals associated with DPD may result in hypoactivation of AIC since there is an excessive but undifferentiated suppression of error signals.
Both psychotic illness and dissociative conditions encompass disorders of perception and disorders of belief (delusions). In psychoses such as schizophrenia, disordered perception is manifest as hallucinations while delusions are characterized by bizarre or irrational self-referential beliefs such as thought insertion by aliens or government agencies (Maher, 1974; Fletcher and Frith, 2009). In dissociative disorders, disordered perceptions are characterized by symptoms of self disturbance as in DPD which can evolve into frankly psychotic delusional conditions such as the Cotard delusion in which patients believe that they are dead (Cotard, 1880; Young and Leafhead, 1996). Fletcher and Frith (2009) propose that, for positive symptoms in psychoses, a Bayesian perspective can accommodate hallucinations and delusions within a common framework. In their compelling account, a shift from hallucination to delusion reflects readjustment of top-down predictions within successively higher levels of cortical hierarchies, in successive attempts to explain away residual prediction errors.
A similar explanation can apply to a transition from non-delusional interoceptive dissociative symptoms in DPD to full-blown (psychotic) delusions in Cotard and the like. To the extent that imprecise predictions at low levels of (interoceptive) hierarchies are unable to suppress interoceptive prediction error signals, imprecise predictions will percolate upward, eventually leading not only to generalized imprecision across cortical hierarchical levels but also to re-sculpting of abstract predictive models underlying delusional beliefs. This account augments the proposal of Corlett et al. (2010) who suggest that the lack of emotional engagement experienced by Cotard patients is surprising (in the Bayesian sense), engendering prediction errors and re-sculpting of predictive models; they do not however propose a role for interoceptive prediction error. The account is also consistent with Young and Leafhead (1996) who argued that the Cotard delusion develops as an attempt to explain (“explain away,” in our view) the experiential anomalies of severe depersonalization (Young and Leafhead, 1996). Interestingly, in one case study DPD symptoms ceased once full-blown Cotard and Fregoli (another rare misidentification delusion in which a familiar person is believe to be an imposter) delusions were co-expressed (Lykouras et al., 2002), suggesting that even a highly abstracted belief structure can be sufficient to suppress chronically aberrant perceptual signals.
The phenomenon of intentional binding is relevant in this context: actions and consequences accompanied by a sense of agency are perceived as closer together in time than they objectively are; conversely, if the consequence is not perceived as the result of the action, the events are perceived as more distant in time than they actually are (Haggard et al., 2002). Importantly, intentional binding has both a predictive and a retrospective component: Schizophrenic patients with disorders of agency show stronger intentional binding than controls (Voss et al., 2010), with abnormalities most evident in the predictive component, reflecting indiscriminate (i.e., imprecise) predictions (Synofzik et al., 2010). In contrast, prodromal individuals (before development of frankly psychotic symptoms) show an increased influence of both predictive and retrospective components, consistent with elevated prediction error signals (Hauser et al., 2011). These results suggest a process through which abnormal prediction errors lead, over time, to imprecise (and eventually reformulated) top-down predictions. A similar account may apply for dissociative symptoms: As with psychosis, anxiety (associated with enhanced interoceptive prediction error) is often prodromal to DPD and is a typical general context for DPD symptoms (Paulus and Stein, 2006).
Dopaminergic neurotransmission is implicated at several points in the discussion so far, most prominently as encoding precisions within predictive coding. Here we expand briefly on the potential importance of dopamine for the present model.
Seminal early work relevant to predictive coding showed that dopaminergic responses to reward, recorded in the monkey midbrain, diminish when reward become predictable over repeated phasic (diachronic) stimulus-reward presentations suggesting that dopamine encodes a reward prediction error signal useful for learning (Schultz and Dickinson, 2000; Chorley and Seth, 2011). More recently, Pessiglione et al. (2006) found that reward prediction errors in humans are modulated by dopamine levels. Modulation was most apparent in the striatum but was also evident in the AIC. In considering this evidence it is important to distinguish the phasic diachronic role of dopamine in signaling reward prediction error (Schultz and Dickinson, 2000) from its synchronic role in modulating (or optimizing) the precision of prediction errors by modulating signal-to-noise response properties in neuronal signaling (Fiorillo et al., 2003; Friston, 2009, 2010). Although our model emphasizes the latter role, the learning function of dopamine may nonetheless mediate the transition from disordered perception to delusion. In this view, dopamine-modulated learning underlies the re-sculpting of generative models to accommodate persistently elevated prediction error signals (Corlett et al., 2010). Dopaminergic neurotransmission may therefore govern the balance between (synchronic) optimization of precisions at multiple hierarchical levels (for both agency and presence) and the reformulation of predictive models themselves, with both mechanisms contributing to delusion formation. This account is also compatible with an alternative interpretation of short-latency dopaminergic signaling in identifying aspects of environmental context and behavior potentially responsible for causing unpredicted events (Redgrave and Gurney, 2006). In this view, short-latency prediction error signals arising in the midbrain ventral tegmental area are implicated in discerning whether afferent sensory signals are due to self-generated actions or to external causes.
Abnormal dopaminergic neurotransmission is observed in the ACC of individuals with schizophrenia (Dolan et al., 1995; Takahashi et al., 2006). Although nothing appears to be known specifically about dopaminergic processing in the insula in individuals with either DPD or schizophrenia, the AIC is rich in dopamine D1 receptors (Williams and Goldman-Rakic, 1998), and both insula and the ACC also express high levels of extrastriatal dopamine transporters, indicating widespread synaptic availability of dopamine in these regions. Dopamine is also a primary neurochemical underpinning a set of motivational functions that engage the AIC, including novelty-seeking, craving, and nociception (Palaniyappan and Liddle, 2011). A more general role for dopamine in modulating conscious contents is supported by a recent study showing that dopaminergic stimulation increases both accuracy and confidence in the reporting of rapidly presented words (Lou et al., 2011).
To recap, we propose that presence results from successful suppression by top-down predictions of informative interoceptive signals evoked (directly) by autonomic control signals and (indirectly) by bodily responses to afferent sensory signals. Testing this model requires (i) the ability to measure presence and (ii) the ability to experimentally manipulate predictions and prediction errors independently with respect to both agency and presence.
Measuring presence remains an important challenge. Subjective measures depend on self-report and can be formalized by questionnaires (Lessiter et al., 2001); however these measures can be unstable in that prior knowledge can influence the results (Freeman et al., 1999). Directly asking about presence may also induce or reduce experienced presence (Sanchez-Vives and Slater, 2005). Alternatively, specific behavioral measures can test for equivalence between real environments and VEs. However these measures are most appropriate for a behavioral interpretation of presence (Sanchez-Vives and Slater, 2005). Physiological measures can also be used to infer presence, for example by recording heart rate variability in stressful environments (Meehan et al., 2002). Presence can be measured indirectly by the extent to which participants are able to perform cognitive memory and performance tasks that depend on features of the VE (Bernardet et al., 2011), though again these measures may correspond to a behavioral rather than a phenomenal interpretation of presence. An alternative, subjective approach, involves asking subjects to modify aspects of a VE until they report a level of immersion equivalent to that of a “reference” VE (Slater et al., 2010a). Finally, presence could be inferred by the ability to induce so-called “breaks in presence” which would not be possible if presence was lacking in the first place (Slater and Steed, 2000). In practice, a combination of the above strategies is likely to be the most useful.
Several technologies are available for experimentally manipulating predictions and prediction errors. Consider first manipulations of prediction error. In the agency component, these errors can be systematically manipulated by, for example, interposing a mismatch between actions and sensory feedback using either VR (Nahab et al., 2011) or by standard psychophysical methods (Blakemore et al., 1999; Farrer et al., 2008). In the presence component, prediction errors could be manipulated by subliminal presentation of emotive stimuli prior to target stimuli (Tamietto and de Gelder, 2010) or by false physiological feedback (Gray et al., 2007). Manipulations of top-down expectations could be achieved by modifying the context in which subjects are tested. For example, expectations about self-generated versus externally caused action can be manipulated by introducing a confederate as a potential actor in a two-player game (Wegner, 2004; Farrer et al., 2008) or by explicitly presenting emotionally salient stimuli to induce explicit expectations of interoceptive responses.
Important constraints on neural models of presence come from experiments directly manipulating the degree of presence while measuring neural responses. VR technology, especially when used in combination with neuroimaging, offers a unique opportunity to perform these manipulations (Sanchez-Vives and Slater, 2005). In one study, a virtual rollercoaster ride was used to induce a sense of presence while brain activity was measured using fMRI. This study revealed a distributed network of brain regions elements of which were both correlated, and anticorrelated, with reported presence (Baumgartner et al., 2008). Areas showing higher activity during strong presence include extrastriate and dorsal visual areas, superior parietal cortex, inferior parietal cortex, parts of the ventral visual stream, premotor cortex, and thalamic, brainstem, and hippocampal regions, and notably the AIC. Other relevant studies have examined behavioral correlates of presence as modulated by VR. In a non-clinical population, immersion in a VE enhances self-reported dissociative symptoms on subsequent re-exposure to the real environment, indicating that VR does indeed modulate the neural mechanisms underpinning presence (Aardema et al., 2010). In another study, self-reported presence anticorrelated with memory recall in a structured VE (Bernardet et al., 2011). Two recent studies speak to a connection between presence and agency. In the first, the ability to exert control over events in a VE substantially enhances self-reported presence in healthy subjects (Gutierrez-Martinez et al., 2011). In the second, schizophrenic patients performing a sensorimotor task in a VE reported lower presence than controls, and for control subjects only, presence was modulated by perceived agency which was manipulated by modulating visual feedback in the VE (Lallart et al., 2009). These results are consistent with our model in which predictive signals emanating from the agency component influence presence.
Virtual reality has also been used to study the neural basis of experienced agency. For example, VR-based manipulation of the relationship between intended and (virtual) experienced hand movements, applied in combination with fMRI, revealed a network of brain regions that correlate with experienced agency, with the right supramarginal gyrus identified as the locus of mismatch detection (Nahab et al., 2011). Several recent studies have used VR to generalize the rubber-hand illusion to induce experiences of heautoscopy (Petkova and Ehrsson, 2008; Blanke and Metzinger, 2009) and body transfer into a VE (Slater et al., 2010b) [Heautoscopy is intermediate between autoscopy and full-blown out of body experience (Blanke and Metzinger, 2009)]. These studies have focused on body ownership and exteroceptive multisensory integration rather than on presence or agency directly (though see Kannape et al., 2010 with respect to agency). We speculate that one reason why these so-called “full-body illusions” are difficult to induce is that, despite converging exteroceptive cues, there remains an “interoceptive anchor” grounding bodily experience in the physical body.
Here we briefly describe related theoretical models of presence and of insula function. Models of agency have already been mentioned (see An Interoceptive Predictive-Coding Model of Conscious Presence) and are extensively discussed elsewhere (David et al., 2008; Fletcher and Frith, 2009; Corlett et al., 2010; Synofzik et al., 2010; Voss et al., 2010; Frith, 2011; Hauser et al., 2011). Riva et al. (2011) interpret presence as “the intuitive perception of successfully transforming intentions into actions (enaction).” Their model differs from the present proposal by focusing on action and behavior, by assuming a much greater phenomenological and conceptual overlap between presence and agency, and by not considering the role of interoception or the AIC. Verschure et al. (2003) adopt a phenomenological interpretation of presence, proposing an association with predictive models of sensory input based on learned sensorimotor contingencies (Bernardet et al., 2011). While this model incorporates predictions it does not involve interoception or propose any specific neuronal implementation. Baumgartner and colleagues propose a model based on activity within the dorsolateral prefrontal cortex (DLPFC). In their model, DLPFC activity downregulates activity in the visual dorsal stream, diminishing presence (Baumgartner et al., 2008). Conversely, decreased DLPFC activity leads to increased dorsal visual activity, which is argued to support attentive action preparation in the VE as if it were a real environment. Supporting their model, bilateral DLPFC activity was anticorrelated with self-reported presence in their virtual rollercoaster experiment (Baumgartner et al., 2008). However, application of transcranial direct current stimulation to right DLPFC, decreasing its activity, did not enhance reported presence (Jancke et al., 2009).
Models of insula function are numerous and cannot be covered exhaustively here. Among the most relevant is a model in which AIC integrates exteroceptive and interoceptive signals with computations about their uncertainty (Singer et al., 2009). In this model, the AIC is assumed to engage in predictive coding for both risk-related and interoceptive signals, however no particular mechanistic implementation is specified. The anxiety model of Paulus and Stein (2006) introduces the idea of interoceptive prediction errors in the AIC but does not specify a computational mechanism or elaborate the notion of interoceptive predictive coding as the constitutive basis of emotion. Palaniyappan and Liddle (2011) leverage the concept of a salience network (see The Insular Cortex, Interoception, and Emotion) to ascribe the insula with a range of functions including detecting salient stimuli and modulating autonomic and motor responses via coordinating switching between large-scale brain networks implicated in externally oriented attention and internally oriented cognition and control. In this model, psychotic hallucinations result from inappropriate proximal salience signals which in turn may arise from heightened uncertainty regarding the (diachronic) predicted outcome of events. To our knowledge, no extant model proposes that the AIC engages in interoceptive predictive coding underlying conscious presence.
We have described a theoretical model of the mechanisms underpinning the subjective sense of presence, a basic property of normal conscious experience. The model is based on parallel predictive coding schemes, one relating to agency reflecting existing “comparator” models of schizophrenia (Frith, 1987, 2011), and a second based on interoceptive predictive coding. The model operationalizes presence as the suppression of informative interoceptive prediction error, where predictions (and corresponding errors) arise (i) directly, via autonomic control signals, and (ii) indirectly, via motor control signals which generate sensory inputs. By analogy with models of agency (Synofzik et al., 2010), the sense of presence is specifically associated with the precision of interoceptive predictive signals, potentially mediated by dopaminergic signaling. Importantly, presence in the model is associated with informative interoceptive afferent and predictive signals, and not with the absence of interoceptive prediction errors per se. The role of the agency component with respect to presence is critical; it provides predictions about future interoceptive states on the basis of a parallel predictive model of sensorimotor interactions. The joint activity of these predictive coding models may instantiate key features of an integrated self-representation, especially when considered alongside models of body ownership based on proprioception and multisensory integration (Blanke and Metzinger, 2009; Tsakiris, 2010). Converging evidence points to key roles for the AIC and the ACC in instantiating predictive models, both for interoceptive and exteroceptive signals, in line with growing opinion that the AIC is a core neural substrate for conscious selfhood (Critchley et al., 2004). In addition, the model suggests a novel perspective on emotion, namely as interoceptive inference along Helmholtzian lines. In this view, emotional states are constituted by interoceptive predictions when matched to inputs, extending early two-factor theories of emotion (Schachter and Singer, 1962) as well as more recent proposals contending that rapid affective predictions can shape exteroceptive perceptions (Barrett and Bar, 2009) or that interoceptive predictions can be useful for homeostatic regulation (Paulus and Stein, 2006).
The model is consistent with known neurobiology and phenomenology of disorders of presence and agency. Presence deficits are particularly apparent in DPD, which is known to involve hypoactivity in the AIC. Associating disturbances of presence with imprecise interoceptive predictions is also consistent with the frequently comorbid alexithymia exhibited by DPD patients. Anxiety, often prodromal or comorbid with DPD is also accommodated by the model in terms of enhanced prediction error signals, which when sustained could lead to the imprecise predictions underlying dissociative symptoms. The hierarchical predictive coding scheme may also account for transitions from disordered perception to delusion as predictive mismatches percolate to successively more abstract representational levels, eventually leading to dopaminergically governed re-sculpting of predictive models underlying delusional beliefs.
The model is amenable to experimental testing, especially by leveraging powerful combinations of VR, neuroimaging, and psychophysiology. These technological developments need however to be accompanied by more sophisticated subjective scales reflecting more accurately the phenomenology of presence. A basic prediction of the model is that artificially induced imprecision in interoceptive predictions should lead to diminished conscious presence and abnormal AIC activity; by contrast, simple elevation of interoceptive prediction error signals should lead instead to increased anxiety. As described in Section “Testing the Model,” these manipulations could be engendered either by preexposure to emotionally ambiguous but salient stimuli or by direct pharmacological manipulation affecting dopaminergic neuromodulation in the AIC. A second basic prediction is that the AIC, as well as other areas involved in interoceptive processing, should show responses consistent with interoceptive predictive coding. For example, by analogy with studies of repetition suppression, AIC should show reduced responses for well predicted interoceptive signals and enhanced responses when expectations are violated. Third, the model predicts that distortions of presence may not necessarily lead to distortions of agency; they will only do so if agency-component predictions realign or change their precision or structure in order to suppress faulty interoceptive prediction errors. Further predictions can be based on the relative timing of activity. In the visual domain, expectations about upcoming sensory input reduce the latency of neuronal signatures differentiating seen and unseen stimuli (Melloni et al., 2011); in other words, expectations speed up conscious access. By analogy, an expected interoceptive signal may be perceived as occurring earlier than an unexpected interoceptive signal. This hypothesis could be tested by manipulations of physiological feedback (Gray et al., 2007). Potentially, VR experimental environments could be used not only for testing the model but also for therapeutic purposes with DPD patients.
Several challenges may be raised to the model as presently posed. First, in contrast to exteroceptive (particularly visual) processing (Felleman and Van Essen, 1991; Nassi and Callaway, 2009), evidence for hierarchical organization of interoceptive processing and autonomic control is less clear. Complicating any such interpretation are multiple levels of autonomic control including muscle reflex autonomic responses mediated at spinal levels, direct influences of motor cortex on sympathetic responses to muscle vasculature, varying degrees of voluntary effects on visceral state, and poorly understood effects of lateralization for both afferent and efferent signals (Delgado, 1960; Craig, 2005; Critchley et al., 2005; McCord and Kaufman, 2010). On the other hand, there is reasonable evidence for somatotopic coding in brainstem nuclei (e.g., nucleus of the solitary tract and area postrema), and subsequently in parabrachial nuclei, thalamic nuclei, and posterior insula (Craig, 2002), consistent with hierarchical organization. It nonetheless remains as a challenge to explore the extent to which interoception can be described, anatomically and functionally, as hierarchical, when considered for example in comparison to object representation in visual or auditory systems.
A second challenge is that predictive coding schemes for visual perception are often motivated by the need for efficient processing of high-bandwidth and highly redundant afferent visual sensory signals (Rao and Ballard, 1999). The functional architecture of interoception appears very different, undermining any direct analogy. However, interoceptive pathways involve dozens or probably hundreds of different dedicated receptors often distributed broadly throughout the body (Janig, 2008), posing potentially even greater computational challenges.
Third, clinical experience suggests that disorders of agency and presence do not always coincide; for example it is not possible to elicit reports of subjective disturbances of conscious presence in all patients with schizophrenia (Ruhrmann et al., 2010). Moreover, depersonalization and derealization are not generally associated with disorders of agency, as shown for example in Alien Hand syndrome (Sumner and Husain, 2008) and Tourette syndrome (Robertson, 2000). While our model specifically allows for independent effects and proposes that agency and presence are neither necessary nor sufficient for each other, additional research is needed to examine experimentally their interactions. It is possible that such studies could invert the hierarchical relationship between agency and presence (see Figure 1) or reframe it as a bidirectional, symmetric relationship. Further, lesions to insular cortex do not always give rise to dissociative symptoms (Jones et al., 2010), raising the possibility that the predictive processes underlying presence play out across multiple brain regions with key nodes potentially extending down into brainstem areas (Damasio, 2010). Alternatively, dissociative symptoms could in fact require an intact insula in order to generate the imprecise predictions underlying the subjective phenomenology.
Finally, our model remains agnostic as to whether it is presence itself, or the experience of its disturbance or absence, that is the core phenomenological explanatory target. Arguably, disturbances of presence are more phenomenologically salient than the background of presence characterizing normal conscious experience. Reportable experience of presence per se may require additional reflective attention of the form induced by subjective questionnaires. A full treatment of this issue would refer back at least as far as the phenomenological work of Heidegger and Husserl (Heidegger, 1962; Husserl, 1963), by way of Metzinger’s discussion of transparency (see The Phenomenology of Presence), lying well beyond the present scope. Nonetheless, by proposing specific neurocognitive constraints our model provides a framework for understanding presence as a structural property of consciousness that is susceptible to breakdown (inducing an experience of the “absence of presence”) in particular and predictable circumstances.
Addressing the above challenges will require multiple research agendas. However, three key tests underpinning many of them are (i) to search explicitly for signs of interoceptive predictive coding in the AIC, (ii) to establish the nature of the target representation of discrete channels of afferent viscerosensory information instantiating such predictive coding schemes, and (iii) to correlate subjective disturbances in presence with experimental manipulations of interoceptive predictions and prediction errors. More prospectively, the model requires extension to address explicitly issues of selfhood. We believe considerable promise lies in integrating interoceptive predictive coding with existing proprioceptive and multisensory models of selfhood (Blanke and Metzinger, 2009; Tsakiris, 2010), potentially explaining the force of “interoceptive anchors” in grounding bodily experience.
In conclusion, our model integrates previously disparate theory and evidence from predictive coding, interoceptive awareness and the role of the AIC and ACC, dopaminergic signaling, DPD and schizophrenia, and experiments combining VR and neuroimaging. It develops a novel view of emotion as interoceptive inference and provides a computationally explicit, neurobiologically grounded account of conscious presence, a fundamental but understudied phenomenological property of conscious experience. We hope the model will motivate new experimental work designed to test its predictions and address its objections. Such efforts are likely to generate important new findings in both basic and applied consciousness science.
The authors declare that the research was conducted in the absence of any commercial or financial relationships that could be construed as a potential conflict of interest.
The authors acknowledge the following funding sources: the Dr. Mortimer and Theresa Sackler Foundation (AKS, HDC); EU project CEEDS (FP7-ICT-2009-5, 258749; AKS, KS); and EPSRC Leadership Fellowship EP/G007543/1 (AKS). We are grateful to Neil Harrison, Nick Medford, Bjorn Merker, Thomas Metzinger, Natasha Sigala, Paul Verschure, and our reviewers for comments that helped improve the paper.
Aardema, F., O’Connor, K., Cote, S., and Taillon, A. (2010). Virtual reality induces dissociation and lowers sense of presence in objective reality. Cyberpsychol. Behav. Soc. Netw. 13, 429–435.
Alexander, W. H., and Brown, J. W. (2011). Medial prefrontal cortex as an action-outcome predictor. Nat. Neurosci. 14, 1338–1344.
American Psychiatric Association. (2000). DSM-IV: Diagnostic and Statistical Manual of Mental Health Disorders, 4th Edn. Washington, DC: APA.
Augustine, J. R. (1996). Circuitry and functional aspects of the insular lobe in primates including humans. Brain Res. Brain Res. Rev. 22, 229–244.
Barbas, H. (2000). Connections underlying the synthesis of cognition, memory, and emotion in primate prefrontal cortices. Brain Res. Bull. 52, 319–330.
Barbas, H., Saha, S., Rempel-Clower, N., and Ghashghaei, T. (2003). Serial pathways from primate prefrontal cortex to autonomic areas may influence emotional expression. BMC Neurosci. 4, 25. doi:10.1186/1471-2202-4-25
Barlow, H. (1990). Conditions for versatile learning, Helmholtz’s unconscious inference, and the task of perception. Vision Res. 30, 1561–1571.
Barrett, L. F., and Bar, M. (2009). See it with feeling: affective predictions during object perception. Philos. Trans. R. Soc. Lond. B Biol. Sci. 364, 1325–1334.
Baumgartner, T., Speck, D., Wettstein, D., Masnari, O., Beeli, G., and Jancke, L. (2008). Feeling present in arousing virtual reality worlds: prefrontal brain regions differentially orchestrate presence experience in adults and children. Front. Hum. Neurosci. 2:8. doi:10.3389/neuro.09.008.2008
Bebbington, P. E., Marsden, L., and Brewin, C. R. (1997). The need for psychiatric treatment in the general population: the Camberwell needs for care survey. Psychol. Med. 27, 821–834.
Bechara, A., Damasio, H., Tranel, D., and Damasio, A. R. (1997). Deciding advantageously before knowing the advantageous strategy. Science 275, 1293–1295.
Bernardet, U., Valjamae, A., Inderbitzin, M., Wierenga, S., Mura, A., and Verschure, P. F. (2011). Quantifying human subjective experience and social interaction using the experience induction machine. Brain Res. Bull. 85, 305–312.
Blakemore, S. J., Frith, C. D., and Wolpert, D. M. (1999). Spatio-temporal prediction modulates the perception of self-produced stimuli. J. Cogn. Neurosci. 11, 551–559.
Blakemore, S. J., Smith, J., Steel, R., Johnstone, C. E., and Frith, C. D. (2000). The perception of self-produced sensory stimuli in patients with auditory hallucinations and passivity experiences: evidence for a breakdown in self-monitoring. Psychol. Med. 30, 1131–1139.
Blanke, O., and Metzinger, T. (2009). Full-body illusions and minimal phenomenal selfhood. Trends Cogn. Sci. (Regul. Ed.) 13, 7–13.
Bossaerts, P. (2010). Risk and risk prediction error signals in anterior insula. Brain Struct. Funct. 214, 645–653.
Brass, M., and Haggard, P. (2010). The hidden side of intentional action: the role of the anterior insular cortex. Brain Struct. Funct. 214, 603–610.
Bubic, A., von Cramon, D. Y., and Schubotz, R. I. (2010). Prediction, cognition and the brain. Front. Hum. Neurosci. 4:25. doi:10.3389/fnhum.2010.00025
Cannon, W. B. (1915). Bodily Changes in Pain, Hunger, Fear, and Rage: An Account of Recent Researches into the Function of Emotional Excitement. Appleton.
Chorley, P., and Seth, A. K. (2011). Dopamine-signaled reward predictions generated by competitive excitation and inhibition in a spiking neural network model. Front Comput Neurosci 5:21. doi:10.3389/fncom.2011.00021
Corlett, P. R., Taylor, J. R., Wang, X. J., Fletcher, P. C., and Krystal, J. H. (2010). Toward a neurobiology of delusions. Prog. Neurobiol. 92, 345–369.
Cotard, J. (1880). Du delire hypocondriaque dans une forme grave de la melancolie anxieuse. Memoire lu a la Societe medicopsychophysiologique dans la Seance du 28 Juin 1880. Ann. Med. Psychol. (Paris) 168–174.
Craig, A. D. (2002). How do you feel? Interoception: the sense of the physiological condition of the body. Nat. Rev. Neurosci. 3, 655–666.
Craig, A. D. (2003). Interoception: the sense of the physiological condition of the body. Curr. Opin. Neurobiol. 13, 500–505.
Craig, A. D. (2005). Forebrain emotional asymmetry: a neuroanatomical basis? Trends Cogn. Sci. (Regul. Ed.) 9, 566–571.
Craig, A. D. (2009). How do you feel – now? The anterior insula and human awareness. Nat. Rev. Neurosci. 10, 59–70.
Critchley, H. D. (2009). Psychophysiology of neural, cognitive and affective integration: fMRI and autonomic indicants. Int. J. Psychophysiol. 73, 88–94.
Critchley, H. D., Mathias, C. J., and Dolan, R. J. (2002). Fear conditioning in humans: the influence of awareness and autonomic arousal on functional neuroanatomy. Neuron 33, 653–663.
Critchley, H. D., Mathias, C. J., Josephs, O., O’Doherty, J., Zanini, S., Dewar, B. K., Cipolotti, L., Shallice, T., and Dolan, R. J. (2003). Human cingulate cortex and autonomic control: converging neuroimaging and clinical evidence. Brain 126(Pt 10), 2139–2152.
Critchley, H. D., Taggart, P., Sutton, P. M., Holdright, D. R., Batchvarov, V., Hnatkova, K., Malik, M., and Dolan, R. J. (2005). Mental stress and sudden cardiac death: asymmetric midbrain activity as a linking mechanism. Brain 128(Pt 1), 75–85.
Critchley, H. D., Wiens, S., Rotshtein, P., Ohman, A., and Dolan, R. J. (2004). Neural systems supporting interoceptive awareness. Nat. Neurosci. 7, 189–195.
Croxson, P. L., Walton, M. E., O’Reilly, J. X., Behrens, T. E., and Rushworth, M. F. (2009). Effort-based cost-benefit valuation and the human brain. J. Neurosci. 29, 4531–4541.
d’Acremont, M., Lu, Z. L., Li, X., Van der Linden, M., and Bechara, A. (2009). Neural correlates of risk prediction error during reinforcement learning in humans. Neuroimage 47, 1929–1939.
Damasio, A. (2000). The Feeling of What Happens: Body and Emotion in the Making of Consciousness. New York: Harvest Books.
Damasio, A. (2010). Self Comes to Mind: Constructing the Conscious Brain. New York: William Heinemann.
David, N., Newen, A., and Vogeley, K. (2008). The “sense of agency” and its underlying cognitive and neural mechanisms. Conscious. Cogn. 17, 523–534.
David, N., Stenzel, A., Schneider, T. R., and Engel, A. K. (2011). The feeling of agency: empirical indicators for a pre-reflective level of action awareness. Front. Psychol. 2:149. doi:10.3389/fpsyg.2011.00149
Dayan, P., Hinton, G. E., Neal, R. M., and Zemel, R. S. (1995). The Helmholtz machine. Neural. Comput. 7, 889–904.
Deen, B., Pitskel, N. B., and Pelphrey, K. A. (2011). Three systems of insular functional connectivity identified with cluster analysis. Cereb. Cortex 21, 1498–1506.
Delgado, J. M. (1960). Circulatory effects of cortical stimulation. Physiol. Rev. Suppl. 4, 146–178.
Dolan, R. J., Fletcher, P., Frith, C. D., Friston, K. J., Frackowiak, R. S., and Grasby, P. M. (1995). Dopaminergic modulation of impaired cognitive activation in the anterior cingulate cortex in schizophrenia. Nature 378, 180–182.
Dorling, J. (1982). Further Illustrations of the Bayesian Solution of Duhem’s Problem. Available at: http://www.princeton.edu/(bayesway/Dorling/dorling.html
Egner, T., Summerfield, C., Trittschuh, E. H., Monti, J. M., and Mesulam, M. M. (2008). Neural repetition suppression reflects fulfilled perceptual expectations. Nat. Neurosci. 11, 1004–1006.
Farrer, C., Frey, S. H., Van Horn, J. D., Tunik, E., Turk, D., Inati, S., and Grafton, S. T. (2008). The angular gyrus computes action awareness representations. Cereb. Cortex 18, 254–261.
Felleman, D. J., and Van Essen, D. C. (1991). Distributed hierarchical processing in the primate cerebral cortex. Cereb. Cortex 1, 1–47.
Fiorillo, C. D., Tobler, P. N., and Schultz, W. (2003). Discrete coding of reward probability and uncertainty by dopamine neurons. Science 299, 1898–1902.
Fletcher, P. C., and Frith, C. D. (2009). Perceiving is believing: a Bayesian approach to explaining the positive symptoms of schizophrenia. Nat. Rev. Neurosci. 10, 48–58.
Freeman, J., Avons, S. E., Pearson, D. E., and Isselstein, W. A. (1999). Effects of sensory information and prior experience on direct subjective ratings of presence. Presence (Camb.) 8, 1–13.
Friston, K. (2009). The free-energy principle: a rough guide to the brain? Trends Cogn. Sci. (Regul. Ed.) 13, 293–301.
Friston, K. (2010). The free-energy principle: a unified brain theory? Nat. Rev. Neurosci. 11, 127–138.
Friston, K., Kilner, J., and Harrison, L. (2006). A free energy principle for the brain. J. Physiol. Paris 100, 70–87.
Frith, C. (1987). The positive and negative symptoms of schizophrenia reflect impairments in the perception and initiation of action. Psychol. Med. 17, 631–648.
Frith, C. (2011). Explaining delusions of control: the comparator model 20 years on. Conscious. Cogn. Available at: http://dx.doi.org/10.1016/j.concog.2011.06.010. [Epub ahead of print].
Gilbert, D. T., and Wilson, T. D. (2009). Why the brain talks to itself: sources of error in emotional prediction. Philos. Trans. R. Soc. Lond. B Biol. Sci. 364, 1335–1341.
Grabenhorst, F., and Rolls, E. T. (2011). Value, pleasure and choice in the ventral prefrontal cortex. Trends Cogn. Sci. (Regul. Ed.) 15, 56–67.
Gray, M. A., Harrison, N. A., Wiens, S., and Critchley, H. D. (2007). Modulation of emotional appraisal by false physiological feedback during fMRI. PLoS ONE 2, e546. doi:10.1371/journal.pone.0000546
Grush, R. (2004). The emulation theory of representation: motor control, imagery, and perception. Behav. Brain Sci. 27, 377–396; discussion 396–442.
Gutierrez-Martinez, O., Gutierrez-Maldonado, J., and Loreto-Quijada, D. (2011). Control over the virtual environment influences the presence and efficacy of a virtual reality intervention on pain. Stud. Health Technol. Inform. 167, 111–115.
Haggard, P., Clark, S., and Kalogeras, J. (2002). Voluntary action and conscious awareness. Nat. Neurosci. 5, 382–385.
Harrison, N. A., Gray, M. A., Gianaros, P. J., and Critchley, H. D. (2010). The embodiment of emotional feelings in the brain. J. Neurosci. 30, 12878–12884.
Hauser, M., Moore, J. W., de Millas, W., Gallinat, J., Heinz, A., Haggard, P., and Voss, M. (2011). Sense of agency is altered in patients with a putative psychotic prodrome. Schizophr. Res. 126(1–3), 20–27.
Hunter, E. C., Sierra, M., and David, A. S. (2004). The epidemiology of depersonalisation and derealisation. A systematic review. Soc. Psychiatry Psychiatr. Epidemiol. 39, 9–18.
Hurd, Y. L., Suzuki, M., and Sedvall, G. C. (2001). D1 and D2 dopamine receptor mRNA expression in whole hemisphere sections of the human brain. J. Chem. Neuroanat. 22, 127–137.
Husserl, E. (1963). Ideas: A General Introduction to Pure Phenomenology, trans. W. R. Boyce-Gibson. New York: Collier Books.
Jabbi, M., Bastiaansen, J., and Keysers, C. (2008). A common anterior insula representation of disgust observation, experience and imagination shows divergent functional connectivity pathways. PLoS ONE 3, e2939. doi:10.1371/journal.pone.0002939
Jancke, L., Cheetham, M., and Baumgartner, T. (2009). Virtual reality and the role of the prefrontal cortex in adults and children. Front. Neurosci. 3:1. doi:10.3389/neuro.01.006.20009
Janig, W. (2008). Integrative Action of the Autonomic Nervous System: Neurobiology of Homeostasis. Cambridge: Cambridge University Press.
Jones, C. L., Ward, J., and Critchley, H. D. (2010). The neuropsychological impact of insular cortex lesions. J. Neurol. Neurosurg. Psychiatr. 81, 611–618.
Kannape, O. A., Schwabe, L., Tadi, T., and Blanke, O. (2010). The limits of agency in walking humans. Neuropsychologia 48, 1628–1636.
Khalsa, S. S., Rudrauf, D., Feinstein, J. S., and Tranel, D. (2009). The pathways of interoceptive awareness. Nat. Neurosci. 12, 1494–1496.
Klein, T. A., Endrass, T., Kathmann, N., Neumann, J., von Cramon, D. Y., and Ullsperger, M. (2007). Neural correlates of error awareness. Neuroimage 34, 1774–1781.
Lallart, E., Lallart, X., and Jouvent, R. (2009). Agency, the sense of presence, and schizophrenia. Cyberpsychol. Behav. 12, 139–145.
Lee, T. S., and Mumford, D. (2003). Hierarchical Bayesian inference in the visual cortex. J. Opt. Soc. Am. A. Opt. Image Sci. Vis. 20, 1434–1448.
Lessiter, J., Freeman, J., Keogh, E., and Davidoff, J. (2001). A cross-media presence questionnaire: the ITC-sense-of-presence inventory. Presence (Camb.) 10, 282–297.
Lombard, M., and Ditton, T. (1997). At the heart of it all: the concept of presence. J. Comput. Mediat. Commun. 2. doi: 10.1111/j.1083-6101.1997.tb00072.x
Lou, H. C., Skewes, J. C., Thomsen, K. R., Overgaard, M., Lau, H. C., Mouridsen, K., and Roepstorff, A. (2011). Dopaminergic stimulation enhances confidence and accuracy in seeing rapidly presented words. J. Vis. 11, 15.
Lovero, K. L., Simmons, A. N., Aron, J. L., and Paulus, M. P. (2009). Anterior insular cortex anticipates impending stimulus significance. Neuroimage 45, 976–983.
Lykouras, L., Typaldou, M., Gournellis, R., Vaslamatzis, G., and Christodoulou, G. N. (2002). Coexistence of Capgras and Fregoli syndromes in a single patient. Clinical, neuroimaging and neuropsychological findings. Eur. Psychiatry 17, 234–235.
Mathews, Z., Bermudez i Badia, S., and Verschure, P. (2012). PASAR: an integrated model of prediction, anticipation, attention and response for artificial sensorimotor systems. Inf. Sci. (N. Y.) 186, 1–19.
McCord, J. L., and Kaufman, M. P. (2010). “Reflex autonomic responses evoked by group III and IV muscle afferents,” in Translational Pain Research: From Mouse to Man, Chap. 12, eds L. Kruger and A. R. Light (Boca Raton, FL: CRC Press).
Medford, N., and Critchley, H. D. (2010). Conjoint activity of anterior insular and anterior cingulate cortex: awareness and response. Brain Struct. Funct. 214, 535–549.
Meehan, M., Insko, B., Whitton, M., and Brooks, F. P. (2002). Physiological measures of presence in stressful environments. ACM Trans. Graph. 21, 645–652.
Melloni, L., Schwiedrzik, C. M., Muller, N., Rodriguez, E., and Singer, W. (2011). Expectations change the signatures and timing of electrophysiological correlates of perceptual awareness. J. Neurosci. 31, 1386–1396.
Mesulam, M. M., and Mufson, E. J. (1982a). Insula of the old world monkey. I. Architectonics in the insulo-orbito-temporal component of the paralimbic brain. J. Comp. Neurol. 212, 1–22.
Mesulam, M. M., and Mufson, E. J. (1982b). Insula of the old world monkey. III: Efferent cortical output and comments on function. J. Comp. Neurol. 212, 38–52.
Miele, D. B., Wager, T. D., Mitchell, J. P., and Metcalfe, J. (2011). Dissociating neural correlates of action monitoring and metacognition of agency. J. Cogn. Neurosci. 23, 3620–3636.
Moller, P., and Husby, R. (2000). The initial prodrome in schizophrenia: searching for naturalistic core dimensions of experience and behavior. Schizophr. Bull. 26, 217–232.
Moseley, G. L., Olthof, N., Venema, A., Don, S., Wijers, M., Gallace, A., and Spence, C. (2008). Psychologically induced cooling of a specific body part caused by the illusory ownership of an artificial counterpart. Proc. Natl. Acad. Sci. U.S.A. 105, 13169–13173.
Mufson, E. J., and Mesulam, M. M. (1982). Insula of the old world monkey. II: Afferent cortical input and comments on the claustrum. J. Comp. Neurol. 212, 23–37.
Nagai, Y., Critchley, H. D., Featherstone, E., Trimble, M. R., and Dolan, R. J. (2004). Activity in ventromedial prefrontal cortex covaries with sympathetic skin conductance level: a physiological account of a “default mode” of brain function. Neuroimage 22, 243–251.
Nahab, F. B., Kundu, P., Gallea, C., Kakareka, J., Pursley, R., Pohida, T., Miletta, N., Friedman, J., and Hallett, M. (2011). The neural processes underlying self-agency. Cereb. Cortex 21, 48–55.
Nassi, J. J., and Callaway, E. M. (2009). Parallel processing strategies of the primate visual system. Nat. Rev. Neurosci. 10, 360–372.
Neal, R. M., and Hinton, G. (1998). “A view of the EM algorithm that justifies incremental, sparse, and other variants,” in Learning in Graphical Models, ed. M. I. Jordan (New York: Kluwer Academic Publishers), 355–368.
Nitschke, J. B., Dixon, G. E., Sarinopoulos, I., Short, S. J., Cohen, J. D., Smith, E. E., Kosslyn, S. M., Rose, R. M., and Davidson, R. J. (2006). Altering expectancy dampens neural response to aversive taste in primary taste cortex. Nat. Neurosci. 9, 435–442.
Northoff, G., and Bermpohl, F. (2004). Cortical midline structures and the self. Trends Cogn. Sci. (Regul. Ed.) 8, 102–107.
Palaniyappan, L., and Liddle, P. F. (2011). Does the salience network play a cardinal role in psychosis? An emerging hypothesis of insular dysfunction. J. Psychiatry Neurosci. 36, 100176.
Pessiglione, M., Seymour, B., Flandin, G., Dolan, R. J., and Frith, C. D. (2006). Dopamine-dependent prediction errors underpin reward-seeking behaviour in humans. Nature 442, 1042–1045.
Petkova, V. I., and Ehrsson, H. H. (2008). If I were you: perceptual illusion of body swapping. PLoS ONE 3, e3832. doi:10.1371/journal.pone.0003832
Phillips, M. L., Medford, N., Senior, C., Bullmore, E. T., Suckling, J., Brammer, M. J., Andrew, C., Sierra, M., Williams, S. C., and David, A. S. (2001). Depersonalization disorder: thinking without feeling. Psychiatry Res. 108, 145–160.
Ploghaus, A., Tracey, I., Gati, J. S., Clare, S., Menon, R. S., Matthews, P. M., and Rawlins, J. N. (1999). Dissociating pain from its anticipation in the human brain. Science 284, 1979–1981.
Pool, J. L., and Ransohoff, J. (1949). Autonomic effects on stimulating rostral portion of cingulate gyri in man. J. Neurophysiol. 12, 385–392.
Porro, C. A., Cettolo, V., Francescato, M. P., and Baraldi, P. (2003). Functional activity mapping of the mesial hemispheric wall during anticipation of pain. Neuroimage 19, 1738–1747.
Preuschoff, K., Quartz, S. R., and Bossaerts, P. (2008). Human insula activation reflects risk prediction errors as well as risk. J. Neurosci. 28, 2745–2752.
Rao, R. P., and Ballard, D. H. (1999). Predictive coding in the visual cortex: a functional interpretation of some extra-classical receptive-field effects. Nat. Neurosci. 2, 79–87.
Redgrave, P., and Gurney, K. (2006). The short-latency dopamine signal: a role in discovering novel actions? Nat. Rev. Neurosci. 7, 967–975.
Riva, G., Waterworth, J. A., Waterworth, W. L., and Mantovani, F. (2011). From intention to action: the role of presence. New Ideas Psychol. 29, 24–37.
Robertson, M. M. (2000). Tourette syndrome, associated conditions and the complexities of treatment. Brain 123(Pt 3), 425–462.
Ruhrmann, S., Schultze-Lutter, F., and Klosterkotter, J. (2010). Probably at-risk, but certainly ill – advocating the introduction of a psychosis spectrum disorder in DSM-V. Schizophr. Res. 120, 23–37.
Rushworth, M. F., and Behrens, T. E. (2008). Choice, uncertainty and value in prefrontal and cingulate cortex. Nat. Neurosci. 11, 389–397.
Sanchez-Vives, M. V., and Slater, M. (2005). From presence to consciousness through virtual reality. Nat. Rev. Neurosci. 6, 332–339.
Sarinopoulos, I., Grupe, D. W., Mackiewicz, K. L., Herrington, J. D., Lor, M., Steege, E. E., and Nitschke, J. B. (2010). Uncertainty during anticipation modulates neural responses to aversion in human insula and amygdala. Cereb. Cortex 20, 929–940.
Schachter, S., and Singer, J. E. (1962). Cognitive, social, and physiological determinants of emotional state. Psychol. Rev. 69, 379–399.
Schultz, W., and Dickinson, A. (2000). Neuronal coding of prediction errors. Annu. Rev. Neurosci. 23, 473–500.
Seeley, W. W., Menon, V., Schatzberg, A. F., Keller, J., Glover, G. H., Kenna, H., Reiss, A. L., and Greicius, M. D. (2007). Dissociable intrinsic connectivity networks for salience processing and executive control. J. Neurosci. 27, 2349–2356.
Seth, A. K. (2009). Explanatory correlates of consciousness: theoretical and computational challenges. Cognit. Comput. 1, 50–63.
Shapiro, R. E., and Miselis, R. R. (1985). The central neural connections of the area postrema of the rat. J. Comp. Neurol. 234, 344–364.
Sherrington, C. S. (1906). The Integrative Action of the Nervous System. Yale: Yale University Press.
Sierra, M., Baker, D., Medford, N., and David, A. S. (2005). Unpacking the depersonalization syndrome: an exploratory factor analysis on the Cambridge Depersonalization Scale. Psychol. Med. 35, 1523–1532.
Sierra, M., and David, A. S. (2011). Depersonalization: a selective impairment of self-awareness. Conscious. Cogn. 20, 99–108.
Simeon, D., Giesbrecht, T., Knutelska, M., Smith, R. J., and Smith, L. M. (2009). Alexithymia, absorption, and cognitive failures in depersonalization disorder: a comparison to posttraumatic stress disorder and healthy volunteers. J. Nerv. Ment. Dis. 197, 492–498.
Simeon, D., Gross, S., Guralnik, O., Stein, D. J., Schmeidler, J., and Hollander, E. (1997). Feeling unreal: 30 cases of DSM-III-R depersonalization disorder. Am. J. Psychiatry 154, 1107–1113.
Simeon, D., Kozin, D. S., Segal, K., Lerch, B., Dujour, R., and Giesbrecht, T. (2008). De-constructing depersonalization: further evidence for symptom clusters. Psychiatry Res. 157, 303–306.
Singer, T., Critchley, H. D., and Preuschoff, K. (2009). A common role of insula in feelings, empathy and uncertainty. Trends Cogn. Sci. (Regul. Ed.) 13, 334–340.
Slater, M., Spanlang, B., and Corominas, D. (2010a). Simulating virtual environments within virtual environments as the basis for a psychophysics of presence. ACM Trans. Graph. 29, 92.
Slater, M., Spanlang, B., Sanchez-Vives, M. V., and Blanke, O. (2010b). First person experience of body transfer in virtual reality. PLoS ONE 5, e10564. doi:10.1371/journal.pone.0010564
Sommer, M. A., and Wurtz, R. H. (2008). Brain circuits for the internal monitoring of movements. Annu. Rev. Neurosci. 31, 317–338.
Suddendorf, T., and Corballis, M. C. (2007). The evolution of foresight: what is mental time travel, and is it unique to humans? Behav. Brain Sci. 30, 299–313; discussion 313–251.
Summerfield, C., and Egner, T. (2009). Expectation (and attention) in visual cognition. Trends Cogn. Sci. (Regul. Ed.) 13, 403–409.
Sumner, P., and Husain, M. (2008). At the edge of consciousness: automatic motor activation and voluntary control. Neuroscientist 14, 474–486.
Synofzik, M., Thier, P., Leube, D. T., Schlotterbeck, P., and Lindner, A. (2010). Misattributions of agency in schizophrenia are based on imprecise predictions about the sensory consequences of one’s actions. Brain 133(Pt 1), 262–271.
Takahashi, H., Higuchi, M., and Suhara, T. (2006). The role of extrastriatal dopamine D2 receptors in schizophrenia. Biol. Psychiatry 59, 919–928.
Tamietto, M., and de Gelder, B. (2010). Neural bases of the non-conscious perception of emotional signals. Nat. Rev. Neurosci. 11, 697–709.
Taylor, K. S., Seminowicz, D. A., and Davis, K. D. (2009). Two systems of resting state connectivity between the insula and cingulate cortex. Hum. Brain Mapp. 30, 2731–2745.
Tsakiris, M. (2010). My body in the brain: a neurocognitive model of body-ownership. Neuropsychologia 48, 703–712.
Tsakiris, M., Longo, M. R., and Haggard, P. (2010). Having a body versus moving your body: neural signatures of agency and body-ownership. Neuropsychologia 48, 2740–2749.
Tsakiris, M., Tajadura-Jimenez, A., and Costantini, M. (2011). Just a heartbeat away from one’s body: interoceptive sensitivity predicts malleability of body-representations. Proc. Biol. Sci. 278, 2470–2476.
Ueda, K., Okamoto, Y., Okada, G., Yamashita, H., Hori, T., and Yamawaki, S. (2003). Brain activity during expectancy of emotional stimuli: an fMRI study. Neuroreport 14, 51–55.
van den Heuvel, M. P., Mandl, R. C., Kahn, R. S., and Hulshoff Pol, H. E. (2009). Functionally linked resting-state networks reflect the underlying structural connectivity architecture of the human brain. Hum. Brain Mapp. 30, 3127–3141.
Verschure, P. F., Voegtlin, T., and Douglas, R. J. (2003). Environmentally mediated synergy between perception and behaviour in mobile robots. Nature 425, 620–624.
Voss, M., Moore, J., Hauser, M., Gallinat, J., Heinz, A., and Haggard, P. (2010). Altered awareness of action in schizophrenia: a specific deficit in predicting action consequences. Brain 133, 3104–3112.
Wager, T. D., Waugh, C. E., Lindquist, M., Noll, D. C., Fredrickson, B. L., and Taylor, S. F. (2009). Brain mediators of cardiovascular responses to social threat: part I: reciprocal dorsal and ventral sub-regions of the medial prefrontal cortex and heart-rate reactivity. Neuroimage 47, 821–835.
Wang, X. J. (2010). Neurophysiological and computational principles of cortical rhythms in cognition. Physiol. Rev. 90, 1195–1268.
Wegner, D. M. (2004). Precis of the illusion of conscious will. Behav. Brain Sci. 27, 649–659; discussion 659–692.
Williams, S. M., and Goldman-Rakic, P. S. (1998). Widespread origin of the primate mesofrontal dopamine system. Cereb. Cortex 8, 321–345.
Wolpert, D. M., and Ghahramani, Z. (2000). Computational principles of movement neuroscience. Nat. Neurosci. 1212–1217.
Young, A. W., and Leafhead, K. M. (1996). “Betwixt life and death: case studies of the Cotard delusion,” in Method in Madness, eds P. W. Halligan and J. C. Marshall (Hove, UK: Psychology Press), 155.
Keywords: presence, consciousness, depersonalization disorder, agency, interoception, insular cortex, virtual reality, predictive coding
Citation: Seth AK, Suzuki K and Critchley HD (2012) An interoceptive predictive coding model of conscious presence. Front. Psychology 2:395. doi: 10.3389/fpsyg.2011.00395
Received: 04 November 2011;
Paper pending published: 05 December 2011;
Accepted: 20 December 2011;
Published online: 10 January 2012.
Edited by:
Morten Overgaard, Aalborg University, DenmarkReviewed by:
Ryota Kanai, University College London, UKCopyright: © 2012 Seth, Suzuki and Critchley. This is an open-access article distributed under the terms of the Creative Commons Attribution Non Commercial License, which permits non-commercial use, distribution, and reproduction in other forums, provided the original authors and source are credited.
*Correspondence: Anil K. Seth, Department of Informatics, Sackler Centre for Consciousness Science, University of Sussex, Brighton BN1 9QJ, UK. e-mail:YS5rLnNldGhAc3Vzc2V4LmFjLnVr
Disclaimer: All claims expressed in this article are solely those of the authors and do not necessarily represent those of their affiliated organizations, or those of the publisher, the editors and the reviewers. Any product that may be evaluated in this article or claim that may be made by its manufacturer is not guaranteed or endorsed by the publisher.
Research integrity at Frontiers
Learn more about the work of our research integrity team to safeguard the quality of each article we publish.