- 1 Max Wertheimer Minerva Center for Cognitive Studies, Technion – Israel Institute of Technology, Haifa, Israel
- 2 Lauder School of Government, Diplomacy and Strategy, Interdisciplinary Center, Herzliya, Israel
Experience-based decisions can be defined as decisions emanating from direct or vicarious reinforcements that were received in the past. Typically, in experience-based decision tasks an agent repeatedly makes choices and receives outcomes from the available alternatives, so that choices are based on past experiences, with no explicit description of the payoff distributions from which the outcomes are drawn. The study of experience-based decisions has long roots in the works of mathematical psychologists during the 1950s and 1960s of the last century (e.g., Estes and Burke, 1953; Bush and Mosteller, 1955; Katz, 1964). This type of task has been viewed as a natural continuation of the behaviorist tradition involving animals as subjects, and multiple trials in which feedback is obtained on each trial. During the 1970s and 1980s seminal studies focusing on choices among descriptive gambles began to dominate the field of Judgment and Decision Making, paving the wave for the successful and influential works of Tversky and Kahneman (e.g., Kahneman and Tversky, 1979). Indeed, a review of the decision making literature from 1970 to 1998 conducted by Weber et al. (2004) shows prominent use of description-based tasks over experience-based tasks.
Yet the study of experience-based decisions has continued to evolve. Some of the workers in this subfield were neuropsychologists who used experience-based tasks as a natural way to evaluate individual differences owing to these tasks having many choice trials (e.g., Bechara et al., 1994). Others were interested in the complex relations between learning and decision making (Erev and Roth, 1998). An interesting finding that has finally defined the importance of contrasting the two types of tasks – experience-based decisions and description-based decisions, was obtained by Ido Erev and his colleagues. Kahneman and Tversky (1979) showed that individuals overweight small probability events in their decisions from description. For instance, in selecting between an alternative producing $3 for sure or a gamble producing 10% chance to receive $32 (and otherwise zero), most people pick the riskier alternative, behaving as if they give greater weight to the relatively rare event (see Hau et al., 2009). Erev and colleagues have demonstrated a reverse phenomenon in decisions from experience (Barron and Erev, 2003; Hertwig et al., 2004; Yechiam et al., 2005). People tend to experientially select alternatives as if what happens most of the time has more weight than the rare event. Thus, people overweight small probability events in decisions from description while underweighting them in decisions from experience. This has been referred to as the description–experience (D–E) gap (Hertwig et al., 2004). The studies exploring the D–E gap were followed by further investigations examining the divergent and convergent processes in these task types (e.g., Rakow et al., 2008; Barron and Yechiam, 2009; Wu et al., 2011).
In parallel to the recent advancements in experience-based decisions within the field of Judgment and Decision Making, there have been numerous studies of this type of decisions in Neuroscience. For example, the feedback-based error-related negativity (fERN; see below; e.g., Gehring and Willoughby, 2002) and the role of non-declarative knowledge in selecting advantageously (Bechara et al., 1997) were found in experience-based decisions. Several studies have explicitly showed that that experience-based tasks result in higher correlation between studied brain variables and over behavior. For example, in Aharon et al.’s (2001) fMRI study, participant evaluated the attractiveness of face images either descriptively or by making choices and receiving feedback. Brain activation levels in the reward circuitry (particularly, the nucleus accumbens) matched the evaluation patterns only in the experiential condition. Similarly, severe damage to the orbitofrontal cortex was found to lead to decision impairments in experience-based tasks, but not in description-based tasks (Leland and Grafman, 2005). Still, many of the investigations of these neuroscientific aspects have borrowed their theoretical underpinning from the study of decisions from description, and have not been guided by relevant theories of experience-based decisions. At the same time, many of the decision making studies of experience-based tasks have taken place without awareness of the relevant brain studies using this paradigm.
In an attempt to highlight the necessity of integrating the two bodies of research (JDM and neuroscience studies), we present three dissociations (or “gaps”) between brain activation patterns and behavioral choices in these tasks. The majority of this paper is devoted to describing the three gaps in order to encourage further research. Additionally, we also suggest some directions for exploring and explaining these inconsistencies.
Three Brain-Behavior Gaps in Experience-Based Decisions
Brain Activation and Behavioral Responses to Rare Events
The very famous “oddball” paradigm examines people’s brain responses following low-probability events compared to more frequent events. The typical result is an elevated fronto-central signal approximately 300 ms following the rare event, which is known as P300. The original oddball paradigm normally required a response following the rare event, and thus confounded the rarity of the event and its performance requirements (Squires et al., 1975). Yet the same findings were also replicated in task-irrelevant rare events (Debener et al., 2005) and in reward prediction tasks (Karis et al., 1983). The elevated neural activation following the rare event appears to be inconsistent with the tendency to underweight rare events in experience-based decisions. One might argue, though, that the dependent variable in decision tasks (i.e., the choice proportion) is also affected by the size of the rare payoff, and this component may be insufficiently integrated upon making choices. Still, this would be inconsistent with the standard way in which the underweighting phenomenon is explained (Hertwig et al., 2004). Moreover, as we shall see below, inconsistency between brain activation and behavior also emerges when the target event (e.g., a loss) is similar in size to the control event (e.g., a gain).
Brain Activation and Behavioral Responses to Losses
In a seminal EEG study, Gehring and Willoughby (2002) demonstrated that a large portion of the frontal cortex exhibits greater rapid activation following losses than following equivalent gains. This event-related brain potential (ERP) has been referred to as medial frontal negativity (MFN; Gehring and Willoughby, 2002) or fERN (Yeung and Sanfey, 2004). Gehring and Willoughby (2002) suggested that the existence of the increased cortical response following losses is consistent with the behavioral principle of “loss aversion,” which denotes an increased subjective weight of losses compared to gains (Kahneman and Tversky, 1979). Furthermore, they argued that the asymmetric fERN response to losses represents the brain mechanism directly contributing to the representation of subjective value, or “instant utility.” However, in Gehring and Willoughby’s (2002) research, as in other studies of experience-based decisions (e.g., Erev et al., 2008; Silberberg et al., 2008; Hochman and Yechiam, 2011; Yechiam and Telpaz, 2011, in press), participants actually made behavioral choices as if they were loss neutral and not loss-averse. Specifically, the fERN was observed in choices between an alternative producing +5 or −5 US cents and a second alternative producing +5 or −25 cents. Loss aversion implies that in choice between a pair of alternatives with symmetric gains and losses, individuals should prefer the alternative producing lower losses. However, the two choice alternatives were selected at about the same rate.
This gap was replicated in follow-up ERP studies, some using higher payoffs (e.g., Nieuwenhuis et al., 2004; Yeung and Sanfey, 2004; Masaki et al., 2006). It has also been demonstrated in studies of autonomic arousal: Losses were found to have greater effect on pupil diameter and heart rate, even in the absence of reliable loss aversion (Hochman and Yechiam, 2011; Yechiam and Telpaz, 2011). Interestingly, the ERP studies noted above did not make a big issue out of this discrepancy, and did not consider it as deserving special attention. Yet we feel that it is a critical issue because participants make experiential choices as if they are loss neutral while their brain acts as if it is loss sensitive.
The Effect of Experience on Risk Taking
The third brain–behavior gap we would like to draw attention to involves a discrepancy between what is known about the dynamic function of the dopaminergic system and experiential choice behavior. Under current models of the striatum, the dopaminergic system adapts to reinforcement using a “Delta” learning rule (Rumelhart et al., 1986), whereby a new outcome from a given alternative is compared to the expectancy of the alternative prior to the outcomes, and the expectancy is updated as a function of the difference between the new outcome and the old expectancy. Brain areas within the striatum were found to exhibit activation patterns consistent with the implied mechanism of the delta rule (Schultz et al., 1997). This rule axiomatically leads to a clear behavioral prediction involving the effect of experience on risk taking behavior (Niv et al., 2002; Denrell, 2007): Under the Delta rule, decision makers should be more risk averse with experience because they invariably sample the risky alternative in a biased fashion. For example, say that in an experience-based decision you have a choice between getting zero for sure and a risky alternative producing equal chances to win or lose $10. In the first 10 trials in which the risky alternative is chosen there is about 40% chance that in reality the likelihood of winning $10 will be less than 50%. This can lead participants to avoid the risky alternative in future choices. Moreover, as soon as the risky alternative is avoided, there is no mechanism that reduces this bias. The chance of biased sampling from the risky alternative continues in the next trials as well, and under the Delta learning rule, it is expected to slowly lead participants away from the risky alternative. But this is not how participants behave in experiential tasks! In several studies involving such symmetric risky alternative (with equal chance of winning or losing) participants were found to show remarkable flatness in their learning curves (see Figure 1). This gap between the mechanism considered to govern learning and actual experiential behavior in tasks involving mixed gains and losses bears some similarity to the issue of loss sensitivity because in both cases the brain activation pattern is in the direction of avoiding risk (and potential losses) while behavior leans toward risk neutrality1.
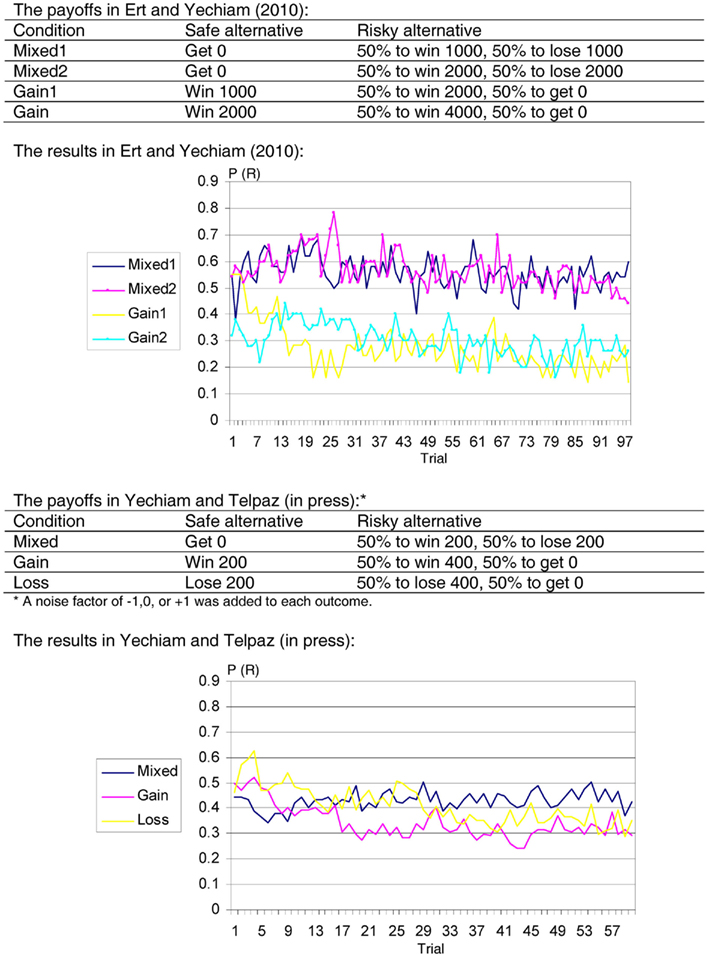
Figure 1. Risk taking in two studies of experience-based decisions. Top: Ert and Yechiam (2010). Bottom: Yechiam and Telpaz(in press). Each task involves the selection among a Safe option (S) and a Risky option (R). The results show the mean proportion of selections from the risky alternative in each trial [P(R)] in different conditions. The participants take less risk over time when payoffs are predominantly gains or losses but not in mixed gains and losses.
Partial Answers
A technical way out of theses gap involves the typically different temporal resolution of brain activation measures and behavioral choices. The fERN patterns, for instance, were revealed in the first 300 ms after the choice outcomes are presented. However, in most studies, decision makers are given much more time to make behavioral decisions. Thus, behavioral loss neutrality might be the product of delayed brain processes implicated in executive control. For instance, some executive functions of verbal working memory have been known to result in activation peaks approximately 600 ms after the relevant stimuli (Gunter et al., 2000) and even later (Tu et al., 2009). Still, while this is a possible interpretation for these gaps, it is quite tentative as the relevant delayed processes have not been uncovered.
An alternative suggestion is that the rapid fronto-central activation following monetary outcomes does not represent “instant utility” weighting of these outcomes (as proposed, for instance, by Gehring and Willoughby, 2002). Possibly, it could represent an attentional phenomenon. We (Yechiam and Hochman, 2011; Yechiam and Telpaz, 2011) suggested that an encompassing increase in frontal activation may represent the intensity of the attentional orienting response. Attention may be drawn by losses for instance, and this may increase the overall investment of cognitive resources in the task, in a symmetric fashion to both gains and losses. A related explanation involves the surprise value of incentives (Nevo and Erev, 2011). Under these explanations, the noted gaps are accounted for by the assertion that brain activation to incentives may represent cognitive processes that do not have a direct effect on the subjective valuation of the stimuli that have elicited them.
Footnote
- ^Risk neutrality over time is also inconsistent with Kahneman and Tversky’s (1979) “loss aversion” hypothesis. As noted in Section “Brain Activation and Behavioral Responses to Losses,” studies of experience-based decisions (e.g., Erev et al., 2008) typically do not find reliable behavioral manifestations of loss aversion. The studies reported in Figure 1 replicate this pattern.
References
Aharon, I., Etcoff, N., Ariely, D., Chabris, C. F., O’Donner, E., and Breiter, H. C. (2001). Beautiful faces have variable reward value: fMRI and behavioral evidence. Neuron 32, 537–551.
Barron, G., and Erev, I. (2003). Small feedback-based decisions and their limited correspondence to description based decisions. J. Behav. Desci. Mak. 16, 215–233.
Barron, G., and Yechiam, E. (2009). The coexistence of overestimation and underweighting of rare events and the contingent recency effect. Judgm. Decis. Mak. 4, 447–460.
Bechara, A., Damasio, A. R., Damasio, H., and Anderson, S. (1994). Insensitivity to future consequences following damage to human prefrontal cortex. Cognition 50, 7–15.
Bechara, A., Damasio, H., Tranel, D., and Damasio, A. R. (1997). Deciding advantageously before knowing the advantageous strategy. Science 275, 1293–1295.
Debener, S., Makeig, S., Delorme, A., and Engel, A. K. (2005). What is novel in the novelty oddball paradigm? Functional significance of the novelty P3 event-related potential as revealed by independent component analysis. Brain Res. Cogn. Brain Res. 22, 309–322.
Erev, I., Ert, E., and Yechiam, E. (2008). Loss aversion, diminishing sensitivity, and the effect of experience on repeated decisions. J. Behav. Decis. Mak. 21, 575–597.
Erev, I., and Roth, A. E. (1998). Predicting how people play games: Reinforcement learning in experimental games with unique, mixed strategy equilibria. Am. Econ. Rev. 88, 848–881.
Ert, E., and Yechiam, E. (2010). Consistent constructs in individuals’ risk taking in decisions from experience. Acta Psychol. (Amst.) 134, 225–232.
Estes, W. K., and Burke, C. J. (1953). A theory of stimulus variability in learning. Psychol. Rev. 6, 276–86
Gehring, W. J., and Willoughby, A. R. (2002). The medial frontal cortex and the rapid processing of monetary gains and losses. Science 295, 2279–2282.
Gunter, T. C., Friederici, A. D., and Schriefers, H. (2000). Syntactic gender and semantic expectancy: ERPs reveal early autonomy and late interaction. J. Cogn. Neurosci. 12, 556–568.
Hau, R., Pleskac, T. J., and Hertwig, R. (2009). Decisions from experience and statistical probabilities: why they trigger different choices than a priori probabilities. J. Behav. Decis. Mak. 23, 48–68.
Hertwig, R., Barron, G., Weber, E. U., and Erev, I. (2004). Decisions from experience and the effect of rare events in risky choices. Psychol. Sci. 15, 534–539.
Hochman, G., and Yechiam, E. (2011). Loss aversion in the eye and in the heart: the autonomic nervous system’s responses to losses. J. Behav. Decis. Mak. 24, 140–156.
Kahneman, D., and Tversky, A. (1979). Prospect theory: an analysis of decision under risk. Econometrica 47, 263–291.
Karis, D., Chesney, G. L., and Donchin, E. (1983). “Twas ten to one; And yet we ventured…”: P300 and decision making. Psychophysiology 20, 260–268.
Katz, L. (1964). Effects of differential monetary gain and loss on sequential two-choice behavior. J. Exp. Psychol. 68, 245–249.
Leland, J. W., and Grafman, J. (2005). Experimental tests of the somatic marker hypothesis. Games Econ. Behav. 52, 386–409.
Masaki, H., Takeuchi, S., Gehring, W., Takasawa, N., and Yamazaki, K. (2006). Affective-motivational influences on feedback-related ERPs in a gambling task. Brain Res. 1105, 110–121.
Nieuwenhuis, S., Yeung, N., Holroyd, C. B., Schurger, A., and Cohen, J. D. (2004). Sensitivity of electrophysiological activity from medial frontal cortex to utilitarian and performance feedback. Cereb. Cortex 14, 741–747.
Niv, Y., Joel, D., Meilijson, I., and Ruppin, E. (2002). Evolution of reinforcement learning in uncertain environments: a simple explanation for complex foraging behaviors. Adapt. Behav. 10, 5–24.
Rakow, T., Demes, K. A., and Newell, B. R. (2008). Biased samples not mode of presentation: re-examining the apparent underweighting of rare events in experience-based choice. Organ. Behav. Hum. Dec. Process. 106, 168–179.
Rumelhart, D. E., McClelland, J. E., and The PDP Research Group. (1986). Parallel Distributed Processing: Explorations in the Microstructure of Cognition, Vols. 1 and 2. Cambridge, MA: MIT Press.
Schultz, W., Dayan, P., and Montague, R. (1997). A neural substrate of prediction and reward. Science 14, 1593–1599.
Silberberg, A., Roma, P. G., Huntsberry, M. E., Warren-Boulton, F. R., Takayuki, S., Ruggiero, A. M., and Suomi, S. J. (2008). On loss aversion in capuchin monkeys. J. Exp. Anal. Behav. 89, 145–155.
Squires, N. K., Squires, K. C., and Hillyard, S. A. (1975). Two varieties of long-latency positive waves evoked by unpredictable auditory stimuli in man. Electroencephalogr. Clin. Neurophysiol. 38, 387–401.
Tu, S., Li, H., Jou, J., Zhang, Q., Wang, T., Yu, C., and Qiu, J. (2009). An event-related potential study of deception to self preferences. Brain Res. 1247, 142–148.
Weber, E. U., Shafir, S., and Blais, A.-R. (2004). Predicting risk sensitivity in humans and lower animals: risk as variance or coefficient of variation. Psychol. Rev. 111, 430–445.
Wu, S.-W., Delgado, M. R., and Maloney, L. T. (2011). The neural correlates of subjective utility of monetary outcome and probability weight in economic and in motor decision under risk. J. Neurosci. 31, 8822–8831.
Yechiam, E., Barron, G., and Erev, I. (2005). The role of personal experience in contributing to different patterns of response to rare terrorist attacks. J. Conflict Resolut. 49, 430–439.
Yechiam, E., and Hochman, G. (2011). Losses as modulators of attention: review and analysis of the unique effects of losses over gains. [Submitted].
Yechiam, E., and Telpaz, A. (2011). To take risk is to face loss: a tonic pupilometry study. Front. Psychol. 2:344. doi: 10.3389/fpsyg.2011.00344
Yechiam, E., and Telpaz, A. (in press). Losses induce consistency in risk taking even without loss aversion. J. Behav. Decis. Mak. doi: 10.1002/bdm.758
Citation: Yechiam E and Aharon I (2012) Experience-based decisions and brain activity: three new gaps and partial answers. Front. Psychology 2:390. doi: 10.3389/fpsyg.2011.00390
Received: 28 November 2011;
Accepted: 12 December 2011;
Published online: 05 January 2012.
Copyright: © 2012 Yechiam and Aharon. This is an open-access article distributed under the terms of the Creative Commons Attribution Non Commercial License, which permits non-commercial use, distribution, and reproduction in other forums, provided the original authors and source are credited.
*Correspondence: yeldad@tx.technion.ac.il