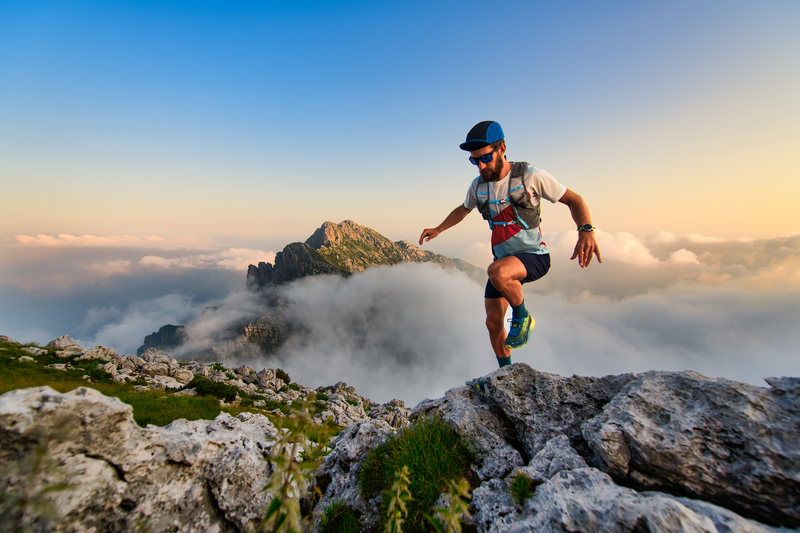
94% of researchers rate our articles as excellent or good
Learn more about the work of our research integrity team to safeguard the quality of each article we publish.
Find out more
ORIGINAL RESEARCH article
Front. Psychol. , 22 November 2011
Sec. Developmental Psychology
volume 2 - 2011 | https://doi.org/10.3389/fpsyg.2011.00345
This article is part of the Research Topic Grounding Word Learning in Attention, Perception, and Memory View all 12 articles
Children acquire attentional biases that help them generalize novel words to novel objects. Researchers have proposed that these biases arise from regularities in the early noun vocabulary children learn and suggest that the specifics of the biases should be tied to the specifics of individual children’s vocabularies. However, evidence supporting this proposal to date comes from studies of group means. The current study examines the relations between the statistics of the nouns young children learn and the similarities and differences in the biases they demonstrate. We show that individual differences in vocabulary structure predict individual differences in novel noun generalization. Thus, these data support the proposal that word learning biases emerge from the regularities present in individual children’s vocabularies and, importantly, that children’s on-line attention during an experiment is mediated by instances of past learning.
A central question in the study of development is how the structure of a child’s environment relates to the structure of his or her thinking (Gibson, 1969). Assessing the nature of experience in terms of available structure can make clear how seemingly unsolvable problems in development are solvable. Language development might be the paradigm case of this as much recent research has detailed ways in which children take advantage of rich structure to “solve” language problems previously thought to be impossible.
For example, research on statistical learning has shown that infants can exploit transitional probabilities at the boundaries of words to segment them from the continuous speech stream (Saffran et al., 1996b). Additional research has shown that infants can use known words (including their own name) to help in segmentation (Bortfeld et al., 2005). Likewise, adults are able to identify the referent of an utterance from muted videos of mother–child conversations (Gillette et al., 1999) suggesting that the structure of social interaction provides enough information to select the correct referent for a novel word from the logically infinite number of possibilities (Quine, 1960). Research also demonstrates that Quine’s problem is further alleviated by the physical structure of children’s bodies; their short arms mean that much of the time the number of possible referents in an adult’s view when a novel name is presented is reduced to a single item for a child (Smith et al., 2011). Together these studies suggest a very different picture of the “logically impossible” referent selection problem: the child’s prior experiences and knowledge, when combined with their physical characteristics and the structure of the interaction, narrow the possibilities such that a solution is likely.
In the current study, we aim to show how structure in the input similarly helps to make the problem of category extension solvable. Even after the word has been segmented from the speech stream and the initial link between a novel word form and referent has been established, a child must learn the proper adult extension for that word; that the word refers to other instances besides the one she has seen named and what properties those instances have in common. Given that the words children are expected to learn early name categories that vary from objects to substances to colors and actions, and the fact that many early learned words name hierarchically nested categories, this too is a very challenging problem. Nevertheless, young children are particularly adept at solving this problem and learning new words. The literature on early word learning suggests that biases or constraints may help children overcome some of the inherent difficulties in generalization by narrowing the scope of possibilities children consider for the organizational structure of newly learned nominal categories. For example, young children reliably demonstrate a tendency to map novel names to novel whole-object referents rather than familiar objects (mutual exclusivity constraint) or parts of objects (whole-object constraint; Markman, 1989, 1992). Likewise, 2- and 3-year-old children generalize novel names of solid objects by similarity in shape rather than material or color, a behavior known as the shape bias (Landau et al., 1988; Smith et al., 2002).
The current study focuses in particular on the shape bias because there is a growing body of research providing a mechanistic account of the tie between language structure and the specifics of the children’s biased attention to shape. To the extent that other word learning biases are the developmental product of children’s growing knowledge about objects, language, and vocabulary, however, our examination of how the structure of this input affects the shape bias, should shed light on our understanding of the solvability of other word learning biases. More generally, even, the data presented here will highlight how children’s on-line attention in a task is shaped by their prior experiences and knowledge; a mechanism that would be available to guide development across a range of domains.
Smith and colleagues originally proposed the term “shape bias” to refer to the finding that young children presented with a novel solid object exemplar and given a novel name, will generalize the novel name to test objects that match the exemplar in shape rather than test objects that match the exemplar in other ways (Landau et al., 1988). Subsequent research suggested that a bias to attend to shape would be beneficial in that the majority of the first 300 nouns children learn early in vocabulary development are names for concrete artifact categories organized by shape similarity (Samuelson and Smith, 1999). Further, the shape bias has been shown to be fundamentally developmental; experimental evidence demonstrates that children begin attending to shape in noun generalization tasks after they have learned some nouns and that attention to shape increases with development (Samuelson and Smith, 1999; Gershkoff-Stowe and Smith, 2004).
Smith and colleagues have proposed a four-step process to explain the emergence of the shape bias from prior learning of individual nominal categories (Smith et al., 2002). According to this proposal, as children learn names for individual instances of an object category (step 1) and individual object categories (step 2), the regular association of solid things and categories organized by similarity in shape in the early English noun vocabulary helps them to learn to attend to shape when learning new words for solid objects (step 3). Further, Smith et al. (2002) proposed that once children have learned to attend to shape in the context of a novel noun for a novel solid object, the fact that there are many such words to learn will set them up to learn new words more quickly (step 4).
Support for this proposal comes from longitudinal studies showing that teaching children names of multiple categories organized by similarity in shape helps them develop a precocious shape bias and acquire vocabulary more quickly than those not given such training (Samuelson, 2002; Smith et al., 2002). Furthermore, cross-linguistic studies show that the biases children demonstrate depend on the language (and therefore the specific regularities) being learned (Smith et al., 2003). Likewise, studies of atypical learners (such as children with autism and late-talkers) show that they do not develop the same attentional biases in word learning as typically developing children (Jones and Smith, 2005; Tek et al., 2008). All of these studies point to a connection between the specifics of the vocabulary being learned and the development and the direction of the bias that develops.
This body of evidence is augmented by recent training studies that have examined the influence of more specific aspects of vocabulary structure on subsequent word learning. These studies have examined how variability, both in terms of the exemplars children see when learning categories (Perry et al., 2010), and in the statistics within the noun vocabulary children learn (Samuelson and Schiffer, in preparation), influences the development of the shape bias. Specifically, Samuelson and Schiffer found that children taught a vocabulary dominated by count nouns that named solid objects in categories well organized by similarity in shape, learned biases differently from children taught a vocabulary containing equal numbers of count and mass nouns, names for solid and non-solid things, and names for categories organized by shape or material. Importantly, the robustness and stability of these biases depended on the size of the children’s vocabularies when they started the study, such that those with smaller starting vocabularies were more affected by the vocabulary acquired during the study (Samuelson and Schiffer, in preparation).
Perry et al. (2010) found that variability in the exemplars used to teach novel names leads to a more discriminating shape bias (generalizing names for novel solid things by similarity in shape but names for novel non-solid things by similarity in material) and an increase in vocabulary development. Furthermore, mixed logistic regression models that incorporated subject and item variables showed how individual children’s performance at each step in the four-step process influenced what they learn and the bias that developed (Perry et al., 2010). These models demonstrate the links between the four steps in Smith et al.’s (2002) proposed process, and thus come closer to making a direct connection between individual vocabulary and the shape bias.
Together, the Samuelson and Schiffer (in preparation) and Perry et al. (2010) training studies demonstrate that the word learning biases that children develop are clearly influenced by the statistics of prior vocabulary learning, in terms of both the (1) between-category organization and overlap of category organization, solidity, and syntax, and (2) the specifics of the within-category variability children are exposed to. These studies also support the idea that what a child knows determines how they will be influenced by the regularities available to learn next. However, no work to date has examined in detail the relation between individual children’s vocabulary structures and the biases they develop. This is the focus of the current paper. Specifically, we examine whether the statistics of individual children’s vocabularies predict their individual likelihood to demonstrate a shape bias.
Noun generalization data are not typically analyzed at the level of individual children because capturing detailed individual data can be difficult. One exception is a longitudinal study conducted by Gershkoff-Stowe and Smith (2004). They examined the vocabularies of individual children on a week-by-week basis in terms of both the total number of nouns and the number of count nouns produced to find the point in vocabulary development at which children first demonstrated a systematic bias to attend to shape in a novel noun generalization (NNG) task. Gershkoff-Stowe and Smith (2004) found that as a group, children who knew more than 50 nouns showed a shape bias. Importantly, however, there was neither a “critical mass” of count nouns, or names for categories organized by similarity in shape, nor a specific age or amount of time in the study that determined whether individual children demonstrated a shape bias in the NNG task. Rather, the specific session at which individual children showed an acceleration and attention to shape differed across children, and the number of nouns children knew at that point was variable. This finding suggests that the statistics of the early noun vocabulary may not be the same for all children at the point when children first demonstrate a shape bias in the NNG task. In part, this could be because only one NNG trial was collected from each child at each visit. Data from children this young are notoriously variable, thus it is possible that the patterns of individual children’s shape biased performance with respect to their vocabulary structures were not statistically reliable, even if the group mean was. Clearly, multiple NNG trials are necessary to examine individual performance.
The lack of an informative predictor in the Gershkoff-Stowe and Smith (2004) study could also be related to the way they classified the vocabulary. Gershkoff-Stowe and Smith (2004) looked at the number of object names in productive vocabulary in relation to generalization biases. However, it is possible that another part of the vocabulary, or an even more specific classification of the words in the vocabulary, would be more predictive of noun generalization behavior. For example, some object names that children learn early in vocabulary development refer to things that are solid (e.g., ball) and some refer to things that are non-solid (e.g., pudding). Samuelson and Smith (1999) used adult judgments of the nominal categories listed on the MacArthur–Bates Communicative Developmental Inventory (MCDI) to examine the structure of the early noun vocabulary in terms of these more specific classifications. Adults were asked to judge whether each of the 312 nouns referred to a category of solid objects or non-solid substances, a category organized by similarity in shape or material, and whether each noun was a count or mass noun. As can be seen in Figure 1, Samuelson and Smith found that there were more nouns referring to solid objects than non-solid substances, more categories organized by similarity in shape than similarity in material, and more count nouns than mass nouns. Furthermore, there was more overlap between solidity, category organization, and syntax for the set of words that would support a shape bias – the “shape side” (solid + shape + count) – than between the set of words that would support a bias to attend to material substance when generalizing a name for a non-solid – the “material side” (non-solid + material + mass).
Figure 1. Overlap between solidity, syntax, and category organization for the “shape side” (left) and “material side” (right) of the early noun vocabulary based on Samuelson and Smith’s (1999) analysis of adult judgments.
Using these judgments of the MCDI noun structure, Samuelson and Smith (1999) then examined both the number of nouns children knew of each type, and how these numbers were related to the mean proportion shape responding in a NNG task. Looking at a wide range of children in terms of both age (17–33 months) and noun vocabulary size (0–309 words), they showed that children at all vocabulary levels had more names for solid objects, categories organized by similarity in shape, and count nouns than names for non-solid things, categories organized by similarity in material, and mass nouns. However, only children with more than 151 nouns in their productive vocabularies demonstrated a shape bias. This suggests, then, that just because a child knows more words that fall into the classifications on the “shape side” does not mean that she will demonstrate a shape bias – otherwise children in all the vocabulary groups would have demonstrated this bias.
Clearly then, to understand the relation between vocabulary structure and bias development we need to look at more than just the number of nouns of different types children know. In particular, the fact that the vocabulary size at onset of shape bias acquisition differs across studies, and across children, suggests that there is no critical mass of nouns to be learned before demonstrating a shape bias. Additionally these studies show that shape bias development cannot just be due to the total number of nouns a child knows because the early vocabulary is always dominated by words on the “shape side,” Thus, Samuelson and Smith’s and Gershkoff-Stowe and Smith (2004) data together suggest that what may matter most for shape bias acquisition is the relative proportions of words that fall into classifications on the “shape side” to other word types. Furthermore, in addition to learning words from the “shape side” and “material side,” children also learn nouns that are exceptions to this ontological divide. These words, such as chalk (solid + material), might be said to “go against the system” in that they would not support a link between solid objects and attention to shape or non-solid substances and attention to material. In fact, given that all the 17- to 33-month-old children in Samuelson and Smith’s sample had many solid + shape + count words in their vocabularies, looking at differences in children’s knowledge of these nouns should not be very revealing. Rather, it might be more informative to look at differences across children in their knowledge of nouns that that “go against the system” and how these relate to NNG performance.
The nature of the MCDI also makes examination of these types of words critical. Samuelson and Smith chose to examine the MCDI in their study because it a reliable and valid measure with extensive normative testing (Fenson et al., 1994). However, Samuelson and Smith’s analysis tells us that this measure is itself biased toward count nouns that name solid things in categories well organized by similarity in shape. Accordingly, any child’s vocabulary measured with this tool will almost certainly have more of these kinds of nouns than others (as seen in Samuelson and Smith, 1999). Thus, this measure will only let us detect relationships between vocabulary structure and noun generalization performance if we look at the parts of the vocabulary that vary more between children. One might argue that a measure other than the MCDI might be preferable. However, continuing with this measure has the advantages that (1) the MCDI is still the standard measure of vocabulary development for children in the age-range we are interested in, (2) switching measures would necessitate obtaining new judgments of solidity, category structure, and syntax, and (3) it enables comparison to prior work. In addition, restricting the vocabulary of interest to the MCDI means we can examine our findings in the context of the known proportions of words on that measure; a comparison that would not be possible if we used another measure or allowed parents to record words not on the MCD. That is, we can look at the number of names for solid things in categories organized by similarity in material with respect to the known proportion of those kinds of words in the possible vocabulary we are examining. So while the MCDI might not be perfectly representative of all children’s knowledge of all categories, it is useful in that it is the “average” vocabulary of an “average” child at a given age.
The specific question for the current study is this: if the four-step process is about forming general word learning biases from the structure present in one’s vocabulary, what would happen if one’s structure differed from that of the average? To answer this question, we first we ask how the vocabulary could vary with respect to dominance. Clearly, as can be seen in Figure 1, given the fact that there are so few words total on the material side relative to the shape side, we are not going to find many children who have vocabularies dominated by non-solid + material + mass words. It would be nearly impossible, for example, for a child to learn all of the words on the material side and none of the words on the shape side. In fact, evidence from a longitudinal training study suggests that while teaching multiple names of categories organized by similarity in shape helps children acquire new nominal categories organized by shape similarity more quickly, but teaching multiple categories organized by similarity in material does not lead to an increase in learning new nominal categories organized by material (Samuelson, 2002). This might be because of the relative proportions of each that are available to learn. So while we will not be able to look at individual children in terms of “children who know only material side words” and “children who know only shape side words,” we can look at how the relative proportion of material words to the number of nouns a child knows compared to the average child affects the attentional biases they demonstrate.
Next we ask how the vocabulary could vary with respect to correlation between classifications. As can be seen in Figure 1, there is a lot of overlap between solidity, syntax, and category organization on the shape side of the vocabulary and considerably less overlap on the material side. The lack of correlation on the material side means that non-overlapping areas actually overlap with classifications on the shape side. Words that have classifications from both “sides” are ones that go against the system. The lack of overlap on the material side, then, suggests that there are actually more words that “go against the system” than those that support the material side of the ontology (see Figure 1). That there are more “against the system” words than material side words, could potentially allow for them to have more of an impact than words from the material side, further necessitating an examination of both types of noun classifications and their influence on attentional biases.
For this reason, then, we propose that knowing more words that “go against” the structure of the average child’s vocabulary (based on Samuelson and Smith, 1999 analysis of the MCDI) would lead to a less robust bias to attend to shape and potentially increase other attentional biases in NNG. While Samuelson and Smith did not detail this structure of overlapping classifications that went against the system, we propose that doing so might help us understand potential individual differences in shape bias development. For example, knowing many names for solid objects in categories organized by similarity in material (such as apple, chalk, and ice) could theoretically lead to a material bias for solids.
The goals of the present study, therefore, are to better understand the development of word learning biases such as the shape bias by (1) exploring the structure of individual children’s productive vocabularies with respect to individual and joint noun classifications, especially those words that go against the typical structure, and (2) using vocabulary structure to predict performance in NNG. We expect that the statistics of a child’s vocabulary should mediate their on-line attention in our task. Specifically, we expect to find differences in the words that individual children know, especially in those joint classifications that go against the typical structure (e.g., solids in material categories), that are linked to their likelihood of demonstrating a shape bias.
Seventy-five 15- to 24-month-old monolingual English speakers (M = 1 year, 7 months, 14 days) participated. There were 40 boys and 35 girls in the final sample. Informed consent was obtained from children’s parent or guardian prior to the first experimental session. Children were recruited via birth records and received a small toy for participation after each session.
Eighteen familiar objects and 30 novel objects were used. Familiar objects formed six sets of two identical objects and one completely different object (e.g., two identical blue cups and one yellow rabbit) used for a warm-up task. Novel objects were used in the NNG test. These formed six sets of five objects each. Each set consisted of an exemplar object, two objects of the same shape as the exemplar but different in color and material (shape matches), and two objects made from the same material as the exemplar but different in color and shape (material matches). Figure 2 shows each stimuli set. Six nonce words were used as names and were randomly assigned to each stimulus set and counterbalanced across participants.
Figure 2. Stimuli sets used in novel noun generalization. Each set is named after the material of its exemplar. In each display, the exemplar is to the far left, the two shape matches are on top, and the two material matches are on bottom.
Participants came to the laboratory for three experimental sessions spaced no more than 8 days apart (M: 3 days, range: 1–8 days). At each session the child completed four NNG test trials for two of the novel sets, so that after three sessions they had completed four trials for all six sets. Parents completed the MCDI: Words and Sentences (Fenson et al., 1994) at the first visit and reviewed it at each subsequent visit to add any new words the children began to produce over the course of the three visits to the laboratory.
On each trial the child explored the exemplar object, one shape match test object and one material match test object for about 10 s. The experimenter then placed the two test objects on the tray, held up the exemplar saying, for example, “This is my kiv. Can you get your kiv?” and pushed the tray forward. Each of the two shape match objects in a stimulus set were presented with each of the two material match objects once for a total of four trials per stimulus set. After a child completed all four trials for a given stimulus set the experimenter moved on to the next set. The order of experimental trials within each stimulus set, as well as the order of the stimulus set was counterbalanced across children and across visits.
Sessions were videotaped and coded offline. Twenty-five percent of sessions were recoded for reliability; inter-coder agreement was 100%. All results are reported as proportion shape choice. We analyze NNG performance in two ways: (1) using t-tests against chance to examine overall performance and (2) using mixed logistic regression to examine the effects of vocabulary on noun generalization performance. We use mixed logistic regression because recent arguments suggest that ANOVA’s on categorical outcome variables, such as those in a forced-choice NNG task, are inappropriate (see Jaeger, 2008). This approach has recently been used to demonstrate the links between the four steps of Smith et al.’s (2002) four-step process (Perry et al., 2010). Furthermore, these models are advantageous for our individual differences approach because we can include random subject and item effects and thereby examine the influence of variance contributed by individual differences in children’s vocabulary structure as well as differences caused by the particular stimuli. We removed colinearity from our models by sum-coding the data and scaling continuous variables. To determine appropriate random effects structure, we began with a completely specified random effects structure including random slopes for all variables in a given model. Then, using model comparison, we systematically removed uninformative random effects to find an appropriate model (c.f. Jaeger, 2009). All final models included random intercepts for subject, item, and session.
Our primary goal is to understand the relationship between an individual’s vocabulary structure and the development of the shape bias. To do so, we examine participants’ performance in three ways. First we examine the results of our NNG test. This will tell us overall how likely our participants, as a group, were to demonstrate a shape bias. Second we examine the structure of children’s vocabularies. This will tell us how the relative proportions of various noun classifications change with total noun vocabulary size. Finally, we examine how well the number of nouns a child knows within a given noun classification account for his or her performance in our NNG task.
We ask if participants show a significant tendency to generalize novel nouns for novel solid objects to other objects that match in shape. Because earlier studies examining shape bias development have typically stopped measuring NNG performance once a shape bias is demonstrated, we do not currently have a good idea of how stable children’s performance is in this task. Therefore, we also examine performance at each session and for each stimulus set.
We begin by examining participants’ overall performance in the NNG task. Figure 3A shows NNG performance across all participants, sessions, and stimuli as proportion shape response out of total responses1. As this task is a two item forced-choice task, chance is at 50%; above chance performance indicates a tendency to choose the shape match object, below chance indicates a tendency to choose the material match. All NNG results are graphed in this way. Overall, children demonstrated a bias to choose the shape match test object at test at greater than chance levels (0.50), M = 0.57, t(446) = 5.81, p < 0.0001. This suggests that overall children were biased to attend to shape when generalizing the names of these novel solid objects. In addition, as can be seen in Figure 3B, shape choices were significantly higher than chance for each of the three sessions, Session 1: M = 0.59, t(75) = 3.75, p < 0.001, Session 2: M = 0.58, t(75) = 3.76, p < 0.001; Session 3: M = 0.55, t(74), p < 0.05.
Figure 3. (A) Overall proportion shape responding in novel noun generalization. (B) Proportion shape responding for each session. (C) Proportion shape responding for each stimulus set.
However, when we next examine performance by stimulus set, we see that children were more likely to choose the shape match for some sets than others. Figure 3C shows performance across all participants and sessions for each of the six stimulus sets. As can be seen in the figure, children showed significant shape responding for the clay, M = 0.64, t(74) = 5.16, p < 0.0001, and wood sets, M = 0.68, t(73) = 5.90, p < 0.0001, and marginally significant shape responding for the sand, M = 0.56, t(73) = 1.89, p < 0.10, and Styrofoam sets, M = 0.55, t(74) = 1.76, p < 0.10. However, children’s choice of the shape match test object did not differ from chance for the plaster, M = 0.54, t(73) = 1.17, ns, and scrubber sponge sets, M = 0.48, t(73) = 0.65, ns. Overall, then the results of our t-tests against chance show that while children generally attended to shape most of the time (across all sessions and for four of the six stimulus sets) there is some variability in their attention to shape that is related to the stimuli used.
We next overview participants’ vocabulary structure in terms of single and joint classifications of solidity, syntax, and category organization based on the adult participants’ judgments reported in Samuelson and Smith (1999). To facilitate comparison to earlier work, we began by breaking children into vocabulary subgroups based on the number of nouns in their productive vocabulary at the third session. We used the same groupings used previously by Samuelson and Smith (1999): 0–50 nouns, 51–150 nouns, 151–250 nouns, 251+ nouns. Table 1 provides NNG performance, the means and ranges of the total noun vocabulary, as well as the number of known nouns within each individual classification of the vocabulary for each subgroup. The left-most column gives the proportion of words in each noun classification out of the total number of nouns on the MCDI. Overall, relative proportions of each of the single classifications were similar to that of the structure of the MCDI and that found by Samuelson and Smith (1999). For example, the proportion of count nouns/mass nouns for each subgroup (from lowest to highest) were 0.79/0.10, 0.75/0.11, 0.75/0.10, and 0.75/0.10 respectively, compared to 0.74/0.10 of MCDI nouns.
Table 1. Proportion shape response and mean, SD, range, and proportion of nouns in each vocabulary classification for each vocabulary subgroup.
Table 2 shows the means and ranges of joint classifications for each of these subgroups. For each of these classifications, we list the proportion of all the nouns children know that represent an overlap between classifications. These classifications are exclusive such that each noun is only counted in the most complete classification in which it fits. Table 3 shows how all 312 nouns on the MCDI are accounted for: both those that fall into these joint classifications and those that do not. We found that the proportions of joint classifications were also similar to the structure of the MCDI and that found by Samuelson and Smith. For example, as can be seen in Table 2, the proportion of mass nouns naming non-solid substances for each subgroup were 0.03, 0.02, 0.02, and 0.02, compared to 0.02 of MCDI nouns.
Table 2. Mean, range, and proportion of nouns in each joint vocabulary classification for each vocabulary subgroup.
Table 3. Number of MCDI nouns in each classification per area based on Samuelson and Smith’s (1999) adult judgments of solidity, syntax, and category organization.
Our overview of the vocabulary structure goes above and beyond the work of Samuelson and Smith (1999), however, in that we examined the number of words children know that fall into joint classifications that go against the system, examining nouns that do not support the link between solidity, shape, and count syntax or the link between non-solidity, material, and mass syntax. There are four such joint classifications of nouns on the MCDI. These include: mass nouns that name solid objects, such as “meat” (0.006 of nouns); count and mass nouns that name categories organized by similarity in both shape and material, such as “popcorn” (0.006 of nouns); count nouns that name categories organized by similarity in material, such as “towel” (0.006 of nouns); and names of solid objects in categories organized by similarity in material, such as “chalk” (0.08 of nouns). Importantly, as can be seen in Table 3, there were actually more nouns that name solid objects in categories organized by similarity in material than there are in all classifications that support the material side combined (25 vs. 22). This relatively large number of words that fall into this classification support further examination of the relationship between differences in individual children’s vocabulary structure and their NNG performance.
We next examine how knowing the names of words in each of these classifications influenced the likelihood of demonstrating a shape bias. To do so, we first looked at the NNG performance of each of the vocabulary subgroups. We scored responses such that a shape response received a 1 and material response received a 0. Thus the higher the score (out of the 24 possible trials), the more biased a participant was to attend to shape. We present these data graphically in Figure 4. All four groups were significantly likely to choose shape matches, 0–50: M = 0.55, t(40) = 3.68, p < 0.001; 51–150: M = 0.59, t(19) = 4.11, p < 0.001; 151–250; M = 0.57, t(8) = 2.80, p < 0.05; 251; M = 0.71, t(4) = 2.82, p < 0.05. However, it is also apparent from the ranges of individual performance within each of these subgroups that not all individual children chose the shape match on every trial (see Figure 4). In fact, only in the highest vocabulary group did all children have a score above 12 (corresponding to above chance performance), while children in the lower three vocabulary subgroups had a wider range of scores. Thus, for the lower vocabulary subgroups, despite overall high attention to shape, there were many children who either performed at chance or demonstrated a material bias. In fact, a mixed logistic regression model accounting for random subject, item, and session factors, showed that the three lowest vocabulary groups were significantly less likely than the highest to generalize by shape, z = 2.55, p < 0.05. We next ask whether differences in the amount of shape responding relate to specific differences in the relative proportions of various types of nouns within the vocabulary.
Figure 4. Proportion shape responding and productive noun vocabulary size for each participant. Vocabulary subgroup divisions marked by vertical gray lines.
Using mixed logistic regression, we examined the effects of the interaction between subgroup and each area of the vocabulary – shape side, material side, and against the system – on shape choice in NNG. We did this by regressing out the number of total nouns a child knew from the number of nouns he or she knew within a given classification, such that this predictor is the number of nouns a child knew in a classification above and beyond what would be predicted based on their group. Thus, as can be seen in Table 3, the shape side predictor includes the number of count nouns that name solid objects, count nouns that name shape categories, and the names of solid objects in shape categories. The material side predictor includes the number of mass nouns that name non-solid substances, mass nouns that name material categories, and the names of non-solid substances in material categories. The against the system predictor includes the number of count nouns that name material categories, mass nouns that name solid objects, mass nouns that name shape categories, and the names of solid objects in material categories.
In order to examine differences in vocabulary, we must consider the possibility that such differences might not present themselves equally in each of our vocabulary subgroups. Because the MCDI has a fixed structure, knowing the majority of words on it will mean having a vocabulary that most closely conforms to that structure. Thus, those children with the largest vocabularies will have much less room to vary from the MCDI or from each other. Children with smaller vocabularies, however have more room to vary. As can be seen in Figure 4, these differences in variability were also present in NNG performance. In particular, the variability in the smallest vocabulary group was such that children with the lowest amount of shape responding actually showed a material bias. By including subgroup in our regression models, then, we can account for such variability and are actually able to better examine individual differences.
The model shows that the number of words a child knew on the shape side was a significant predictor of NNG performance such that knowing more of these words leads to a bias to attend to shape, z = 2.19, p < 0.05. There was also a significant interaction such that children with smaller vocabularies who knew more of these words were more likely to attend to shape, z = 2.56 p < 0.05. The model also shows that the number of words a child knew that go against the system was a negatively significant predictor of NNG such that knowing more of these words lead to a bias to attend to material, z = −2.22, p < 0.05. There was also a significant interaction such that children with smaller vocabularies who knew more of these words were more likely to attend to material, z = −2.56, p < 0.05. Finally, the model shows that the number of words a child knew on the material side was not a significant predictor of NNG, z = −0.62, ns.
We next conducted model comparison to examine which of these predictors was necessary to account for children’s performance in NNG. A model without the shape side predictor, (containing only the against the system and material side predictors) was significantly worse than a model that contained all three predictors, X2(1) = 11.28, p < 0.05. A model without the against the system predictor (i.e., containing only the shape side and the material side predictors) was significantly worse than a model that contained all three predictors, X2(1) = 13.78, p < 0.01. However, a model without the material predictor (i.e., containing only the shape side and the against the system predictors) was not statistically different than a model that contained all three predictors, X2(1) = 0.64, ns. Further model comparison revealed that a model containing only the shape side predictor was significantly worse than a model with all three predictors, X2(1) = 16.78, p < 0.05. A model containing only the against the system predictor, however, was no different than the model with all three predictors, X2(1) = 11.85, ns. Thus, the number of words children know that go against the system can account for their NNG performance.
To understand this result, we next consider the composition of classifications of nouns that go against the system. There are 31 MCDI nouns in classifications that go against the system (see Table 3). However, 25 of these name solid objects in categories organized by material, while the other 6 are spread across 3 other classifications: 2 count nouns that name material categories, 2 mass nouns that name solid objects, 2 nouns that name categories organized by both shape and material. Furthermore, there was only ever one child in the smallest vocabulary group who knew a word from each of these other classifications. Clearly, most of the work of the against the system predictor is being done just by the number of names of solid objects in categories organized by similarity in material. In fact, a model of just the interaction between vocabulary group and the number of nouns a child knew that named solid objects in material categories was able to account for children’s NNG. The more of these words children knew, the more likely they were to demonstrate a material bias, z = −2.21, p < 0.05. Furthermore, there was an interaction such that children with smaller vocabularies who knew more of these words were more likely to demonstrate a material bias, z = −2.48, p < 0.05. We present the results of this model in Figure 5. This figure shows, for each vocabulary group, how the number of names of solid objects in material categories children know above and beyond what we would expect given their vocabulary group predicts their likelihood of demonstrating a shape bias. Thus, especially for those children with the smallest vocabularies, knowing a lot of names for solid objects in categories that are organized by similarity in material leads children to attend to material when learning the names of solid objects.
Figure 5. Relationship between number of names of solid objects in material categories a child knows above and beyond what we would expect given their vocabulary group and likelihood of choosing shape match.
We began our analyses by asking (1) how did children perform overall in our NNG task both in terms of stability across sessions and stimulus sets, (2) what was the structure of children’s vocabulary in terms of various noun classifications, and (3) how did this structure relate to individual children’s performance in our NNG task. We found that overall, children showed a significant bias to attend to shape when generalizing novel names for solid objects. This finding adds to earlier research (i.e., Gershkoff-Stowe and Smith, 2004) by demonstrating stability in performance across sessions. Next we found that there was some stability across vocabulary development in the relative proportion of most noun classifications children know. This too is consistent with earlier findings (i.e., Samuelson and Smith, 1999). Additionally, we described the structure of the part of the vocabulary that goes “against the system” and found that there children know a larger number of words that “go against the system” than that support the “material side.” Finally, our mixed logistic regression models show that the number of words children knew that went against the system, particularly those that named solid objects in categories organized by material, predict NNG performance. This is a novel finding that strongly supports the proposal that children’s word learning biases emerge from the regularities present within their individual vocabularies (e.g., Smith et al., 2002).
In the current study we asked whether the statistics of an individual child’s vocabulary could predict the attentional biases they acquire. Importantly, this is the first study to look at individual children’s vocabulary at this level of depth and examine how subsets of known words influenced on-line attention in NNG tasks. Overall, our results suggest that the structure of a child’s vocabulary does, in fact, predict the direction of attentional bias she will demonstrate in an NNG task with solid objects. To understand this link between vocabulary structure and noun generalization, we considered three areas of the early vocabulary: words on the “shape side,” words on the “material side,” and words that go against the system.
One might think that knowing many words on the “shape side” of the vocabulary would be the best predictor of attention to shape in NNG tasks because knowing a lot of shape categories should direct attention to shape. However, we found that it was not the case that differences in the number of names for solid objects in categories organized by shape that children knew could independently account for differences in their attentional biases. This is because all children have vocabularies dominated by words on the “shape side” of the vocabulary, and thus these words alone cannot distinguish between children’s patterns of noun generalizations. There are two possible reasons we saw the dominance of names for solid objects in categories well organized by similarity in shape across the entire range of vocabulary we examined. First, it could be that words on the “shape side” of the vocabulary are either easier to acquire or more prevalent in early linguistic input and thus all children know more of them than other types of words. It is also possible, however that the apparent dominance is a product of a biased measure of children’s vocabularies – the MCDI. While it is clear the MCDI does reflect a biased vocabulary structure dominated by words on the shape side, it has also been established as valid and reliable list of the possible words within an “average” child’s vocabulary (Fenson et al., 1994). Thus, we would argue that the dominance of the shape side is present both within our measure itself and in what we are attempting to measure.
Another possible cause of individual differences in attentional biases could be the number of names on the “material side” of the vocabulary different children know. Knowing many names of non-solid substances in categories organized by material helps children know when shape is not important, thus making it more clear when shape is important (i.e., in the case of solid objects; see also Perry et al., 2010). We found, however, that the “material side” predictor was not significantly related to NNG performance. This could be because children who know many words on the “material side” of the vocabulary are only more likely to attend to material when generalizing the names of novel non-solid stimuli, which we did not examine in this study. It is also possible, however, that there are so few words on the “material side” that even knowing many of them has little effect on NNG performance.
A review of Samuelson’s (2002) neural network simulations of vocabulary acquisition effects on noun generalization can help tease these possibilities apart. When a network was trained with a vocabulary that had an equal number of material side and shape side words, it became biased to generalize by shape for solid objects and material for non-solid substances. However, when the network was trained on the natural statistics found in the MCDI, corresponding to an average child’s vocabulary (i.e., more names on the “shape side”), it overgeneralized a shape bias to both solid and non-solid stimuli. This suggests, then, that the children in our study did not show a connection between the number of material words they know and their NNG performance because there are so few material words to learn (as in Samuelson’s natural statistics condition) rather than because they were tested with solid stimuli.
We did find, however, that knowing many nouns that “went against the system,” was a significant predictor of NNG performance. In particular, if a child’s vocabulary contained many nouns that name solid objects in categories organized by similarity in material, they were more likely to demonstrate a material bias when generalizing novel nouns for solid objects. This provides a concrete link between individual children’s vocabulary structure and their on-line performance in NNG tasks. This also demonstrates how words that are not part of the dominate segment of the vocabulary statistics – those that go “against the system” – affect the biases that emerge. We found that quite a few of the nouns children learn early actually fall into such classifications.
This may seem surprising given that we often conceptualize English as having a strict ontological divide between solids and non-solids, such that solid things are named by count syntax and belong to categories organized by shape while non-solid things are named by mass syntax and belong to categories organized by material substance (e.g., Soja et al., 1991). However, this ontological divide is not so clear in the structure of the early noun vocabulary (Samuelson and Smith, 1999, 2000). Based on adult judgments of MCDI nouns, there are more words that go against this system (31) then there are on the material side (22) of the early learned noun vocabulary. Furthermore, children have to learn many different types of words with many different types of category organizations, and attending to material when learning to name novel solid objects is actually appropriate in many cases. Adults are clearly able to have multiple construals for solid things – we can understand a table as either the object “table” (shape construal) or as something made of wood (material construal; Prasada et al., 2002). Thus, English speakers need to be able to flexibly attend to shape or material (or both) for solid objects. This fits with the fact that the four-step process of shape bias development does not predict that there is anything special about paying attention to shape per se, but rather it proposes that the dominance of the shape side of the vocabulary and the overlap between these classifications lead to a bias to attend to shape relatively early in development – other biases would be expected if the vocabulary being learned provided different statistics.
Our primary question in this line of work was: given that the attentional biases children demonstrate when generalizing nouns are the developmental product of the structure of the early noun vocabulary, what happens if one’s structure is measurably different from the typical structure. The data presented here suggest that the specifics of the early vocabulary do change the specifics of the biases children demonstrate, even in a sample of children all learning the same language. These data, then, show how children’s in-the-moment learning about novel nominal categories is grounded in the specifics of their prior vocabulary knowledge via attentional biases. Given this link between individual children’s vocabulary statistics and their current word learning, an important next question is where did the variability in the specifics of the learned vocabulary come from? That is, why did some of the children in our sample know so many of the “against the system” words?
One possible origin of the individual differences we saw is differences in the words and objects that these children were exposed to earlier in development. Cross-linguistic analyses show that there are systematic differences in the way Japanese mothers and American mothers discuss objects (Fernald and Morikawa, 1993) that relate to children’s vocabulary development (Yoshida and Smith, 2001, 2003). In a similar way, there could also be are also subtle differences that exist within cultures in the way parents talk to children about objects. Parents who talk more about objects’ materials or who name more objects in categories organized by material, or who allow their children to play with the non-solid food items presented at mealtimes (c.f. Perry et al., in preparation), could influence their children to attend more to the material of solid objects. These children would then more readily learn the names of categories of solid objects organized by similarity in material.
Alternatively, there could be differences in the attentional abilities of children that lead them to focus on material. Late-talkers, or children who fall below the 20th percentile in productive vocabulary development, show a bias to generalize the names of novel objects by similarity in texture (Jones, 2003) and have difficulty recognizing known objects’ abstract shapes (Jones and Smith, 2005). This work suggests that attentional differences between children relate to differences in their word learning biases and vocabulary development. These types of attentional differences could lead to differences in the types of words children learn. If a child develops a bias to attend to the texture or material of objects rather than their shape, she might be more likely to learn the names of categories organized by similarity in material compared to names of categories organized by similarity in shape. Both this idea, that differences in children’s perceptual and attentional abilities create vocabulary differences, and the idea that differences in early exposure create vocabulary differences, could account for the variability we found in our study.
While the primary focus of our study is explaining what the differences between children can tell us about the development of word learning, an important secondary contribution is demonstrating how similarity between children emerges. There has been a tendency in the literature to think that the great amount of similarity seen among children means word learning is innately constrained (e.g., Soja et al., 1991). However, our data suggest instead that more general process of attention and memory build these biases over time, and that while children end up with similar biases, they begin much more variably. In particular, the data presented here show that differences in vocabulary and noun generalization were more pronounced in the lowest vocabulary groups and less pronounced in the highest vocabulary groups. What happens over the course of noun acquisition that leads to increasing stability within and between children’s vocabulary and the subsequent attentional biases they demonstrate?
One possibility is that the decreased variability in vocabulary structure as children learn more nouns reflects the nature of development itself. Perhaps initial differences in the structure of children’s vocabulary reflect differences in potential developmental trajectories open to any child early in development. Research on the development of reaching serves as a useful analogical tool for considering the development of word learning in this way. Thelen et al. (1993) followed the developmental trajectories of four children as they learned to reach, and found that each child had a vastly different trajectory. For example, Gabriel initially made large flapping motions with his whole body toward an object, while Hannah only made small timid movements with her hands. Because they each had different starting points, their developmental paths were necessarily different. For example, Gabriel’s movements had to become more controlled and limited to the arms in order to stabilize a reach to a particular point in space to grasp an object. Hannah’s movements, in contrast, had to become stronger and further removed from her body in order for her to get to things that were presented at a distance. Nevertheless, by the time they were able to successfully reach for an object, the children’s movements were very similar to each other’s, despite the fact they had started in such different places and had taken such different developmental paths.
This example highlights how early individual differences may be reduced over the course of development as intrinsic differences become increasingly constrained. At first, as children learn new words they might do so relatively randomly – based on the specifics of what they are interested in, exposed to, and what they happen to learn. Just like Hannah and Gabriel starting to reach in two different ways two children might start out with two very different vocabularies. One child might first learn the words “ball” and “cup” and start attending to shape while another child might learn “ice” and “chalk” and start attending to material. Given, however, the fact that the words they are learning early all come from a relatively fixed set (words you say to children) with a fixed structure (the structure of the MCDI), as these children learn more and more words, their vocabularies will become more and more similar in structure. Thus, the more nouns children know, the less their vocabulary structure will vary from each other and from the MCDI, as our data confirm.
This increase in vocabulary constraints over development further explains why we found our strongest effects on NNG in the groups of children with the smallest vocabularies. As children learn more words, their vocabularies will more closely reflect the structure of the normative vocabulary and thus their NNG behaviors will be less variable (see Figure 4). Children might take many different pathways to get there, but eventually they mostly all get to the same place: a bias to attend to shape. Thus, constraints on behavior that lead children to achieve the same developmental outcomes, do not need to be built into the child because they themselves are the product of the developmental system that includes the child and the word learning environment.
Our examination of the detailed statistics of children’s vocabularies sheds light on this developmental cascade of word learning constraints. We acknowledge that our conclusions about these statistics are influenced by the fact that these children’s vocabularies were already relatively developed by the time they visited the laboratory, and by the fact that our measure of children’s vocabulary at this point (i.e., the MCDI) is itself limited. Additionally, there is still a question of possible pre-linguistic origins for the biased vocabulary structure. However, the children in the smallest vocabulary group tended to have more variable vocabulary structures (and NNG performance). This suggests that the first words children learn are more free to vary and are influenced by many factors, including but not limited to what objects children are surrounded by, what their parents talk about, what they like to play with, and what their language names. These factors all likely affect young children’s attention as well, and future research clearly needs to address the sources of potential pre-linguistic attentional differences and the role they play on early learned words and early word learning biases.
Nevertheless, we believe our study is one of the strongest tests of the four-step process. We have shown a connection between individual children’s vocabularies and their NNG, demonstrating how attention is mediated by past learning. Further, we have demonstrated not only how individual differences in the structure of vocabulary knowledge constrain attention in word learning, but also how these constraints increase over development and thereby create stability in generalization behavior both within and between children. The developmental trajectory seen here, wherein children come to have more and more similar vocabularies points to the common structure of English pushing what may start out as slightly or someone different attentional biases across individual children to have more similar vocabularies. This vocabulary then drives attentional word learning biases. That then influence the words children subsequently learn. That then further changes their attentional biases. Thus, the development of word learning biases is an interaction between attention and word learning across time.
In this way, then, category extension does not present children with an unsolvable problem because the rich structure present in children’s previously acquired vocabulary smartly directs category extensions and facilitates future word learning. And, importantly, this vocabulary structure and the emergent on-line constraints it creates to direct word learning are themselves the developmental product of cascading changes in attention. In this way, then, we have clearly demonstrated how the structure of vocabulary affects shape bias development. When we consider this demonstration in the context of other examples of how children exploit linguistic (e.g., Saffran et al., 1996a), social (e.g., Gillette et al., 1999), and physical structures (e.g., Smith et al., 2011) to support their word learning we can start to see how such an unsolvable problem becomes solvable.
The authors declare that the research was conducted in the absence of any commercial or financial relationships that could be construed as a potential conflict of interest.
Research was supported by grant R01 HD045713 awarded to Larissa Samuelson by the Eunice Kennedy Shriver National Institute of Child Health and Human Development. The content is solely the responsibility of the authors and does not necessarily represent the official views of the Eunice Kennedy Shriver National Institute of Child Health and Human Development or the National Institutes of Health. We thank Susan Cook for help with the analyses, Ian Messenger and Ryan Schiffer for their assistance with study design and data collection, and the children and parents for participating.
Bortfeld, H., Morgan, J. L., Golinkoff, R. M., and Rathbun, K. (2005). Mommy and me: familiar names help launch babies into speech-stream segmentation. Psychol. Sci. 16, 298–304.
Fenson, L., Dale, P. S., Reznick, J. S., Bates, E., Thal, D., and Pethick, S. J. (1994). Variability in Early Communicative Development Monographs of the Society for Research in Child Development, 59.
Fernald, A., and Morikawa, H. (1993). Common themes and cultural variations in japanese and american mothers’ speech to infants. Child Dev. 64, 637–656.
Gershkoff-Stowe, L., and Smith, L. B. (2004). Shape and the first hundred nouns. Child Dev. 74, 1098–1114.
Gibson, E. J. (1969). Principles of Perceptual Learning and Development. Englewood Cliffs, NJ: Prentice-Hall.
Gillette, J., Gleitman, H., Gleitman, L., and Lederer, A. (1999). Human simulations of vocabulary learning. Cognition 73, 135–176.
Jaeger, T. F. (2008). Categorical data analysis: away from ANOVAs (transformation or not) and towards logit mixed models. J. Mem. Lang. 59, 434–446.
Jaeger, T. F. (2009). Random effects: Should I stay or should I go? [web log message]. Available at: http://hlplab.wordpress.com/2009/05/14/random-effect-structure/
Jones, S. S., and Smith, L. B. (2005). Object name learning and object perception: a deficit in late talkers. J. Child Lang. 32, 223–240.
Landau, B., Smith, L. B., and Jones, S. S. (1988). The importance of shape in early lexical learning. Cogn. Dev. 3, 299–321.
Markman, E. M. (1992). “Constraints on word learning: speculations about their nature, origins, and domain specificity,” in Modularity and Constraints in Language and Cognition. The Minnesota Symposia on Child Psychology, eds M. R. Gunnar, and M. Maratsos (Hillsdale, NJ: Lawrence Erlbaum Associates), 59–101.
Perry, L. K., Samuelson, L. K., Malloy, L. M., and Schiffer, R. N. (2010). Learn locally, think globally: exemplar variability supports higher-order generalization and word learning. Psychol. Sci. 21, 1894–1902.
Prasada, S., Ferenz, K., and Haskell, T. (2002). Conceiving of entities as objects and stuff. Cognition 83, 141–165.
Saffran, J. R., Aslin, R. N., and Newort, E. L. (1996a). “Statistical cues in language acquisition: word segmentation by infants,” in Proceedings of the Eighteenth Annual Conference of the Cognitive Science Society, La Jolla, CA, 376–380.
Saffran, J. R., Aslin, R. N., and Newport, E. L. (1996b). Statistical learning by 8-month-old infants. Science 274, 1926–1928.
Samuelson, L. K. (2002). Statistical regularities in vocabulary guide language acquisition in connectionist models and 15-20-month-olds. Dev. Psychol. 38, 1016–1037.
Samuelson, L. K., and Smith, L. B. (1999). Early noun vocabularies: do ontology, category organization and syntax correspond? Cognition 73, 1–33.
Samuelson, L. K., and Smith, L. B. (2000). Children’s attention to rigid and deformable shape in naming and non-naming tasks. Child Dev. 71, 1555–1570.
Smith, L. B., Colunga, E., and Yoshida, H. (2003). Making an ontology: cross-linguistic evidence. Cognitie Creier Comportament 7, 61–90.
Smith, L. B., Jones, S. S., Landau, B., Gershkoff-Stowe, L., and Samuelson, L. K. (2002). Object name learning provides on-the-job training for attention. Psychol. Sci. 13, 13–19.
Smith, L. B., Yu, C., and Pereira, A. F. (2011). Not your mother’s view: the dynamics of toddler visual experience. Dev. Sci. 14, 9–17.
Soja, N. N., Carey, S., and Spelke, E. S. (1991). Ontological categories guide young children’s inductions of word meaning: object terms and substance terms. Cognition 38, 179–211.
Tek, S., Jaffery, G., Fein, D., and Naigles, L. R. (2008). Do children with autism spectrum disorders show a shape bias in word learning? Autism Res. 1, 208–222.
Thelen, E., Corbetta, D., Kamm, K., Spencer, J. P., Schneider, K., and Zernicke, R. F. (1993). The transition to reaching: mapping intention and intrinsic dynamics. Child Dev. 64, 1058–1098.
Yoshida, H., and Smith, L. B. (2001). Early noun lexicons in English and Japanese. Cognition 82, B63–B74.
Keywords: word learning, categorization, individual differences
Citation: Perry LK and Samuelson LK (2011) The shape of the vocabulary predicts the shape of the bias. Front. Psychology 2:345. doi: 10.3389/fpsyg.2011.00345
Received: 22 September 2011; Accepted: 03 November 2011;
Published online: 22 November 2011.
Edited by:
Haley Vlach, University of California, USAReviewed by:
Ji Yun Son, California State University, USACopyright: © 2011 Perry and Samuelson. This is an open-access article subject to a non-exclusive license between the authors and Frontiers Media SA, which permits use, distribution and reproduction in other forums, provided the original authors and source are credited and other Frontiers conditions are complied with.
*Correspondence: Lynn K. Perry, Department of Psychology and Delta Center, University of Iowa, E11 Seashore Hall, Iowa City, IA 52242, USA. e-mail:bHlubi1wZXJyeUB1aW93YS5lZHU=
Disclaimer: All claims expressed in this article are solely those of the authors and do not necessarily represent those of their affiliated organizations, or those of the publisher, the editors and the reviewers. Any product that may be evaluated in this article or claim that may be made by its manufacturer is not guaranteed or endorsed by the publisher.
Research integrity at Frontiers
Learn more about the work of our research integrity team to safeguard the quality of each article we publish.