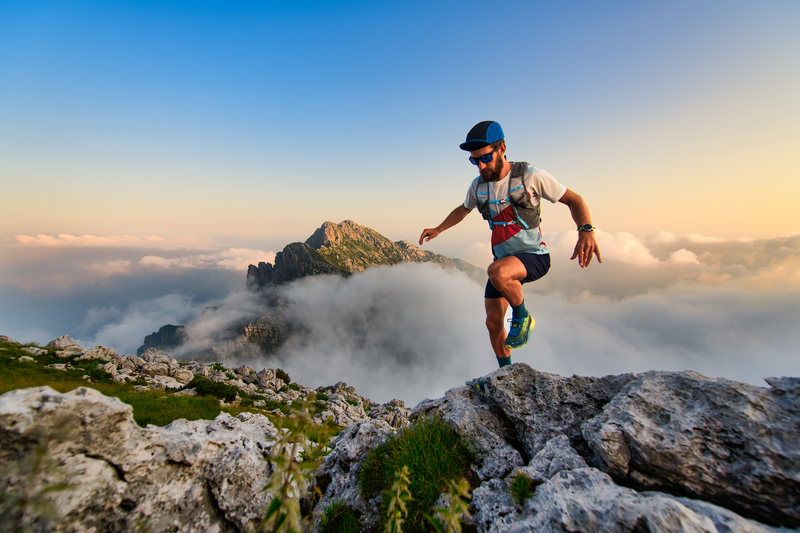
94% of researchers rate our articles as excellent or good
Learn more about the work of our research integrity team to safeguard the quality of each article we publish.
Find out more
ORIGINAL RESEARCH article
Front. Psychol. , 21 November 2011
Sec. Cognition
volume 2 - 2011 | https://doi.org/10.3389/fpsyg.2011.00340
This article is part of the Research Topic Interfaces Between Language And Cognition View all 18 articles
Human capacity to quickly learn new words, critical for our ability to communicate using language, is well-known from behavioral studies and observations, but its neural underpinnings remain unclear. In this study, we have used event-related potentials to record brain activity to novel spoken word forms as they are being learnt by the human nervous system through passive auditory exposure. We found that the brain response dynamics change dramatically within the short (20 min) exposure session: as the subjects become familiarized with the novel word forms, the early (∼100 ms) fronto-central activity they elicit increases in magnitude and becomes similar to that of known real words. At the same time, acoustically similar real words used as control stimuli show a relatively stable response throughout the recording session; these differences between the stimulus groups are confirmed using both factorial and linear regression analyses. Furthermore, acoustically matched novel non-speech stimuli do not demonstrate similar response increase, suggesting neural specificity of this rapid learning phenomenon to linguistic stimuli. Left-lateralized perisylvian cortical networks appear to be underlying such fast mapping of novel word forms unto the brain’s mental lexicon.
As a communication tool, human language is far more complex than any signaling system developed by other animal species. Amongst the many features making human language unique is the impressive size of our vocabularies, which reach into tens of thousands of words (Corballis, 2009). To acquire this knowledge, humans learn new words with high speed and efficiency – as children acquiring their native tongue and as adults mastering a new one. This capacity for rapid learning of language, also known as “fast mapping,” has been demonstrated in numerous behavioral studies and observations (Carey and Bartlett, 1978; Dollaghan, 1985) which have indicated immediate behavioral effects of fast word learning present even before the nervous system has had a chance of consolidating the new information. However, the neural underpinnings of this crucial human skill still remain obscure. On the systems level, much experimentation has been done on longer-term effects of learning revealing neural correlates of days and weeks of practice or at least an overnight consolidation (see Davis and Gaskell, 2009, for a review), whereas the rapid aspect of word learning has remained a difficult task for neurobiological studies.
Indeed, addressing immediate plastic changes in the healthy human brain, as it is learning new words, is not a trivial task. Unlike animal research, invasive measures that provide direct assessment of neural activity are generally not possible in humans. This implies the need to use other tools that either address neural activity indirectly (such as behavioral or hemodynamic methods) or, even if they deal with mass neuronal activation (such as electro and magnetoencephalography, EEG/MEG), their limited resolution normally requires presentation of multiple trials to acquire a stable image of brain activity. These methodological limitations prevent straightforward recording of dynamic neural changes in the learning process. This is why most neuroimaging attempts so far could only provide a derived and abstracted picture of fast learning processes in the brain, failing to capture the online progression of language elements from novel to learnt. To date, only a small number of experiments combining modern neuroimaging tools with carefully designed linguistic paradigms have been preformed to explore the human brain dynamics in language learning.
One such study trained adult functional magnetic resonance imaging (fMRI) subjects on a novel vocabulary of concrete nouns that were assigned meaning via a word–picture associative learning paradigm, which took place during the scanning (Breitenstein et al., 2005). Rather than comparing different conditions, this study monitored changes in the hemodynamic brain activation throughout the experiment by quantifying BOLD responses over five consecutive experimental sub-blocks. It showed changes in the hippocampus in the learning exposure accompanied by a complex pattern of activity involving a variety of neocortical structures: selective activation of right inferior-frontal gyrus, suppression in left fusiform gyrus, and activation increase in left inferior parietal lobe. Investigations using positron-emission tomography (PET) showed that changes in activity in bilateral posterior superior temporal gyri correlate with behavioral performance in non-word learning task (Majerus et al., 2005). Another PET study indicated a left-lateralized network of neocortical areas – temporal lobe, inferior-frontal gyrus, temporo-parietal junction – as taking part in rapid word learning, along with parahippocampal structures (Paulesu et al., 2009). Importantly, such studies not only confirm hippocampal involvement in encoding that had been known from previous animal neurophysiology research and neuropsychological studies in brain-damaged patients, but they also indicate a complex neocortical pattern of activation and de-activation that takes place in the learning process. On one hand, this does map onto a generally accepted two-stage or “complementary” learning systems approach, which maintains that initial encoding takes place in hippocampus with a later slow-rate (days/weeks) transfer of memory representations to neocortex (McClelland et al., 1995); on the other hand, this questions the slowness of neocortical memory trace formation and clearly suggests neocortical involvement in initial encoding stages.
Whilst hemodynamic brain imaging has exquisite spatial resolution, its temporal resolution – on the order of seconds – is poor; furthermore, it does not measure neural processes directly but addresses them by proxy, via cerebral blood flow and metabolism. For these principled reasons, metabolic neuroimaging cannot measure rapid neuronal activations that are known to take place on the millisecond range. Language-elicited brain dynamics is known to unfold extremely rapidly with a number of processing stages reflected in complex neuronal activation patterns in the first few hundred milliseconds of stimulus arrival (Friederici, 2002; Pulvermüller and Shtyrov, 2009; Shtyrov et al., 2010a). Clearly, to better understand neural processes of language learning, there is a need for a more direct measure of electric neuronal activity; this can be afforded by neurophysiological time-resolved imaging tools such as electroencephalography.
To explore electrophysiological correlates of rapid word learning, some EEG studies have used N400, a negative deflection in the brain’s event-related potentials that is known to be sensitive to lexical and semantic stimulus features. Mestres-Misse et al. (2007), whose subjects were required to discover the meaning of a visually presented novel word from its context, found that just after a few exposures to novel words, their N400 response amplitudes were virtually indistinguishable from those to previously known words. Very similar electrophysiological dynamics was obtained in a more recent N400 study using context-restricted novel word learning, also in the visual modality (Borovsky et al., 2010). Interestingly, in an EEG study that involved learning an artificial language, an increase of N400 in response to newly learnt words was found already after 1 min of exposure (De Diego Balaguer et al., 2007).
Whilst N400 is an established linguistic ERP component, in sentential context it likely reflects not only, and not so much the word learning processes per se, but rather the integration of the new items into a larger context (Friederici, 2002). It has also been argued that neural access to lexical word information commences much earlier than 400 ms and can already be reflected in evoked responses at 100–150 ms (Shtyrov et al., 2005; Shtyrov and Pulvermüller, 2007; Pulvermüller et al., 2009). Thus, the need to directly address learning of individual words as such is still open. Behavioral studies suggested that a mere repetitive exposure to a novel word form creates a lexical entry (Gaskell and Dumay, 2003). This was directly tested in a recent EEG study (Shtyrov et al., 2010b), where the subjects were passively exposed in a very short session to a repetitive presentation of the same novel word form, with an acoustically similar real word serving as a control. Importantly, whilst the N400 studies above used visual presentation, this experiment was performed in the auditory modality, the native modality for language in which most of natural language acquisition occurs in real life. To test the dynamics of the stimuli’s lexical status in the subjects’ mental lexicon, this study used passive oddball stimulus presentation that is known to generate diverging patterns for words and unfamiliar pseudo-words: the early (∼120 ms) passive oddball response to a spoken word is enhanced in comparison with similar pseudo-word, and this enhancement is believed to be a neural signature of a word-specific memory trace activation (Pulvermüller and Shtyrov, 2006; Shtyrov et al., 2010a). In the first minutes of the exposure session, an enhanced activity for known words was found, indexing the ignition of their underlying memory traces. However, just after ∼14 min of learning exposure, the novel word forms exhibited a significant increase in response magnitude matching in size with that to real words. This activation increase, as it was proposed, reflects rapid mapping of new word forms onto neural representations formed in left temporal/perisylvian neocortex.
This study was, however, limited in its findings as it only used a single token of novel word form. This was presented in an oddball paradigm, a rather unnatural stimulus presentation mode in which one frequent stimulus is presented hundreds of times and is occasionally replaced by a diverging auditory event. Although the single-item approach is similar to the earliest behavioral research which reported fast mapping of novel words using a single token (Carey and Bartlett, 1978) and such findings cannot be refuted per se, generalizability of such a result is rather limited. Furthermore, none of the previous studies controlled the specificity of fast mapping effects to language by employing comparable non-linguistic conditions. In this study, we have set out to overcome the shortcomings of earlier research. We investigated online neural correlates of novel word form learning using a small acoustically matched group of known words and novel spoken word forms which were presented, at a natural speech rate, to experimental participants in a passive auditory exposure together with acoustically matched novel non-speech stimuli, whilst online measures of the participants’ brain activity were taken using multi-channel electroencephalographic recordings.
Sixteen healthy right-handed (handedness assessed according to Oldfield, 1971) native Finnish-speaking subjects (Helsinki University students, age 18–29, seven males) with normal hearing and no record of neurological diseases were presented with spoken Finnish language stimuli in two experimental conditions. All subjects gave their written consent to take part in the study and were paid for their participation.
For stimulus presentation, we employed a small group of controlled bi-syllabic stimuli which were closely matched in their acoustic features and were produced by recombining the same set of two first and four second syllables to generate eight spoken items with different lexical properties: four previously unfamiliar novel word forms (so called “pseudo-words”) and four known words used as a control, as well as two additional non-speech controls. Two Finnish syllables [pa] and [ta] were combined with syllables [ko], [ku], [ke], [ki], which resulted in the following combinations: pakko, *pakku, pakki, *pakke, in one of the conditions, and *takko, takku, takki, *takke in the other condition (double consonant in Finnish stands for a geminate stop signifying the extended silent closure before the [k], 275 ms in this case; pseudo-words are preceded with an asterisk). Note that the stimulus combinations were minimally different in their acoustic features with the final consonant–vowel transition being sufficient to identify each item per se as well as differentiate between the known words and novel pseudo-words. This made sure that the time point when any possible lexical effects could commence was the same across all stimuli of interest – at the onset of the second syllable. This is essential for analyzing auditory ERP recordings that are highly sensitive to temporal and other physical-acoustic features of the stimuli; in this design, we could time-lock responses to the same time point for all stimuli. These minimal word-final differences also meant that the stimuli within each block belonged to the same cohort, i.e., had common lexical neighbors with similar onsets (as ta- and pa-starting stimuli were presented in two separate blocks). Effectively, the range of possible alternatives was restricted by the experimental settings to the stimulus set as no other completions were possible in each experimental block.
For stimulus production, we recorded multiple repetitions of these syllables uttered by a female native speaker of Finnish and selected a combination of the six items whose vowels matched in their fundamental frequency (F0) as well as sound energy and overall duration (Figure 1). The sounds were normalized to have the same loudness by matching their root-mean-square (RMS) power; this was separately normalized for the first ([pa]/[ka]) and for the second (“word-final”) syllables. Further, a signal-correlated noise (SCN) was produced by subjecting acoustic white noise to a fast Fourier-transform (FFT) filter, whose profile was modeled after the actual second syllables; the filtered noise was then given a temporal envelope of a CV-syllable and combined with the same two first syllables to produce two non-speech control stimuli. All individual syllables (including non-speech SCN) were 100 ms long and all complete stimuli were 475 ms in duration. The stress was always placed on the first syllable, as it is standard in the Finnish language. For the analysis and production of the stimuli we used the Cool Edit 2000 program (Syntrillium Software Corp., AZ, USA).
Figure 1. Waveforms of acoustic stimuli used in the experiments: all stimuli were composed of the same first syllables [pa] and [ta], which were recombined (after a 275 silent closure) with the second syllables [ku], [ko], [ke] [ki], and a matched non-speech sound. The stimuli were maximally matched for their acoustic properties, whilst their lexical status as familiar or novel items was systematically modulated.
Given previous behavioral linguistic research indicating that word learning reaches a plateau at ∼150 repetitions in a short behavioral exposure (Pittman, 2008), we presented our experimental subjects with the novel spoken pseudo-words, control words, and SCN stimuli 160 times per each stimulus in a passive listening task lasting approximately 20 min. Each of the two blocks ([pa]/[ta]) included 160 pseudo-random repetitions of five (four speech and one SCN) stimuli. All stimuli were presented via headphones at 50 dB above individual hearing threshold. Stimulus onset asynchrony was 750 ms, approximating natural speech rate in Finnish (Valo, 1994). The order of the two blocks was counterbalanced across the subject group. Previous research has suggested that initial lexical processing is automatic and that early neurophysiological effects may be masked by focused attention (Garagnani et al., 2009; Shtyrov et al., 2010a); participants’ attention was therefore diverted from the stimuli to a silent video film of their own choice whilst they listened passively to the auditory stimuli, as it was done in a previous study that successfully traced formation of novel memory traces for single words (Shtyrov et al., 2010b).
Subjects were seated in an electrically and acoustically shielded chamber. During the stimulation, electric activity of the subjects’ brain was continuously recorded (passband 0.01–100 Hz, sampling rate 500 Hz) with a 64-channel EEG set-up (Compumedics Neuroscan, El Paso, TX, USA), using gold-plated Ag/AgCl electrodes mounted in an extended 10–20-system custom-made electrode cap (Virtanen et al., 1996) and a separate nose reference electrode. To control for eye-movement artifacts, horizontal and vertical eye movements were recorded using two bipolar electrooculogram (EOG) electrodes.
The recordings were later filtered off-line (passband 1–20 Hz, 12 dB/oct). Event-related potentials were obtained by averaging epochs, which started 50 ms before the stimulus disambiguation point (second syllable onset) and ended 400 ms thereafter; −50 to 0 ms interval was used as a baseline. Epochs with voltage variation exceeding 100 μV at any EEG channel or at either of the two EOG electrodes were discarded; on average, this led to 117 accepted trials for each stimulus type. The remaining EEG data were recomputed against average reference. Following this, three types of analysis were used. We first compared data subsets covering the initial and final 10% of the learning session. Notably, these amounted to 16 or fewer trials for each individual stimulus, which is substantially below the standard auditory ERP studies that typically use in excess of 100 trials for averaging; as we hypothesized that rapid learning could occur within a short time interval, we had to limit the number of trials to see any potential learning effects. To overcome the low signal-to-noise ratio (SNR) resulting from the inherent small number of trials, we pulled together data from all novel pseudo-words and, separately, known words. Based on previous research (Shtyrov et al., 2010a,b), we extracted data from fronto-central midline electrodes where the auditory evoked response is typically maximal (Fz, FCz) in an a priori defined 20-ms window at 110–130 ms and submitted these to analyses of variance (ANOVA) with the factors Stimulus type (Word/Pseudo-word) and Exposure time (early/late in the session). As visual inspection of responses showed an additional presence of an earlier peak (∼80 ms), a second 20 ms time window centered on this earlier deflection was added to the analyses post hoc.
Our second analysis, aimed at finer-scale temporal changes in the responses over the course of the session, applied linear regression on individual subjects’ peak amplitude data obtained from consecutive 10% intervals for both word and pseudo-word responses. Having fitted the least-squares line to individual amplitude measurements for each subject, we submitted regression coefficients to ANOVAs in order to verify significance of any observed differences between stimulus types. Brain Vision Analyzer 1.05 (Brain Products, Gilching, Germany) was used for processing the EEG signal, Matlab 7.0 programming environment (Mathworks, Natick, MA, USA) was used for in the linear regression analyses; statistical analysis was implemented in Matlab 7.0 and in Statistica 7.1 (Statsoft, Tulsa, OK, USA).
In the final analysis, aimed at localizing cortical sources of the found learning effect (response increase for the novel pseudo-word), we performed L2 minimum-norm current estimation on ERP difference between the pseudo-word trials collected in the end and start (10%, i.e., last vs. first 2 min) of the exposure block. This distributed source analysis does not make a priori assumptions about underlying generators and attempts to minimize the overall activity that can account for the recorded electric potentials (Ilmoniemi, 1993). MNE solutions were calculated for grand-average responses rather than individual data; calculating solutions on grand-average data has a benefit of substantially reduced noise and therefore improved SNR which MNE solutions are highly sensitive to (hence individual source solutions were not possible here due to the low SNR inherent to the small number of trials under consideration), although prevents assessing results statistically. A three-layer boundary element model with triangularized gray matter surface of a standardized brain (Montreal Neurological Institute) was used for computing source reconstruction solutions. The solutions were restricted to smoothed gray matter surface. CURRY 6.1 software (Compumedics Neuroscan, Hamburg, Germany) was used for these procedures. Based on the previous studies, our expectation was that of left-lateralized perisylvian activation for the newly formed memory representations.
All items elicited evoked responses, and ERPs were successfully calculated for the word and pseudo-word stimuli both early and late in the exposure session (Figures 2 and 3). Within a short time after the divergence point (∼70–130 ms), the ERP temporal dynamics demonstrated differences for the novel and familiar items early and late in the exposure session. The first analysis, concentrated on the a priori defined window centered on 120 ms, indicated a fronto-central maximum of positive polarity that showed a significant interaction Stimulus type × Exposure time [F(1,15) = 13.45, p = 0.0023]. Investigating this interaction with planned comparisons, we found that it was due to the word response remaining unchanged between the start and the end of the exposure block (p > 0.5), while the pseudo-word response enhanced significantly with time [F(1,15) = 16.79, p = 0.0009]. Visual inspection of the data (Figure 2) indicated that exposure-related ERP effects were occurring also in an earlier time window, with a word-elicited maximum peaking at 80 ms. To account for this earlier activation, we added a second 20-ms window (70–90 ms) to the analysis. This combined analysis supported the Stimulus type × Exposure time interaction [F(1,15) = 5.83, p = 0.0289]; again, planned comparisons confirmed that it was due to the absence of changes in the word response (p > 0.9) and a significant increase in the pseudo-word activity [F(1,15) = 11.62, p = 0.0034]. A marginally significant interaction of the newly introduced factor Window (80 vs. 120 ms) with Stimulus type [F(1,15) = 4.03, p = 0.06] suggested an earlier peak for the word than pseudo-word stimuli (also visible in the ERP patterns). We therefore directly compared the slightly later activation for pseudo-words with the earlier word peak. This comparison, for the third time, confirmed the differential word/pseudo-word dynamics over the learning session as a significant interaction [F(1,15) = 11.73, p = 0.0038]. Furthermore, investigation of this interaction with planned comparisons showed that whilst the word response significantly exceeded that to pseudo-word in the beginning of the session [F(1,15) = 6.10, p = 0.025], the difference between the two was absent in the end of the exposure (p > 0.13).
Figure 2. Electric brain response (global activation computed as RMS across all EEG electrodes; grand-average data) for word and pseudo-word stimuli early and late in the learning session. Responses are time-locked to the stimulus uniqueness points (second syllable onsets) when each stimulus could first be identified. Note the larger word response early in the session and the pseudo-word response increase by the end of the exposure.
Figure 3. Electric brain response (global activation computed as RMS across all EEG electrodes; grand-average data) for word and pseudo-word stimuli early and late in the learning session and voltage topography maps for comparison between the early and late response (based on “late” minus “early” subtraction). Note the larger change in the pseudo-word response by the end of the exposure, topographically visible as an increased left-frontal positivity in the voltage maps.
To quantify the development of language-evoked brain activity throughout the entire recording session, linear regression analysis was applied to word- and pseudo-word-elicited activation calculated for successive sub-averages (10%) obtained from each individual, pulled across both analysis windows (Figure 4). Least-squares lines fitted to word ERPs demonstrated a stable pattern, whereas for the newly learnt pseudo-words the regression analysis showed a significant increase in event-related activity with exposure time. The specific increase of brain responses to pseudo-words was further confirmed by a statistical comparison of regression slopes (beta values) obtained from each subject individually and entered into group analysis [F(1,15) = 4.89; p < 0.045].
Figure 4. Assessment of ERP magnitude change through the exposure session using linear regression over consecutive 10% sub-blocks. Note the relative stability of the word response in contrast with the marked increase in the pseudo-word response amplitude. Data from both time windows (70–90 and 110–130 ms) from midline electrodes (Fz, FCz) were used for computing linear regression for each participant’s responses to known words and novel pseudo-words.
ERP topography (Figure 2) suggested that the word responses had a consistent bias toward left-hemispheric lateralization early and late in the training session, whilst the pseudo-word response appeared to shift from a central to a left-biased distribution with exposure progress (see also maps in Figure 3); this interaction, however, did not reach significance. To further localize the cortical sources potentially underlying the rapid emergence of memory traces for novel word forms, L2 minimum-norm current estimation was applied to ERP difference between the pseudo-word trials collected in the end and start of session. Sources of this neurophysiological effect were localized to bilateral temporal and inferior-frontal cortices with a noticeable lateralization of activity to left-perisylvian neocortex (Figure 5), in line with the ERP signal topography (Figure 3) and our original predictions. As grand-average data were used in this analysis in order to improve the SNR for computing the solutions, these results could not be verified statistically and should therefore be treated with caution.
Figure 5. Cortical source distributions (L2 minimum-norm) in the left and right cerebral hemisphere accounting for the increase in novel word form activation over the exposure session.
Finally, the non-speech SCN stimulus did not exhibit any significant changes over the duration of repetitive perceptual exposure. Its time course (Figure 6) was markedly different from that elicited by the spoken stimuli and in the early interval near 100 ms was suggestive of a response decline with the reverse taking place after 200 ms. However, no significant exposure-related differences could be located (p > 0.6).
Figure 6. Electric brain response (global activation computed as RMS across all EEG electrodes; grand-average data) for the non-speech signal-correlated noise control stimuli early and late in the learning session. Note the marked difference in the SCN time course from that elicited by the spoken stimuli (cf. Figure 3). No significant exposure-related differences could be located for this non-speech elicited activation.
We recorded brain’s responses to previously unfamiliar novel spoken word forms, acoustically matched real familiar words and non-linguistic sounds. These were randomly and repetitively presented in a passive auditory exposure that lasted approximately 20 min. Electric brain responses were generated by all types of stimuli; changes in their dynamics over the course of the perceptual learning session were scrutinized using a factorial analysis which compared ERPs in the beginning and end of the recording, and a linear regression approach that looked for stable patterns over successive sub-averages throughout the session.
The earliest activity that was registered here and exhibited differential dynamics was that around 70–130 ms from the point in time when the information in the auditory input allowed for stimulus identification. This deflection had a fronto-central distribution of positive polarity (using average reference) and showed a markedly different dynamics between the stimulus types. The familiar known words produced a stable pattern with minimal changes between the beginning and the end of the session. This stability is in line with previously postulated robustness of neural circuits acting as word-specific memory traces (Garagnani et al., 2009; Shtyrov, 2010). In contrast, novel word forms, which initially produced a smaller response than that to words, demonstrated a dramatic change with the exposure progress and finally matched in size (and visually even overtook) the response to words.
This pseudo-word-specific activation modulation with exposure time, as we would like to propose, reflects rapid mapping of new word forms onto neural representations. Importantly, this activation is remarkably early (∼100 ms) and occurs in a passive perceptual exposure, when the subjects are not paying attention to the stimuli. These two factors largely exclude the possibility that it may be linked to secondary post-comprehension processes, an argument that could in principle be made in relation to metabolic or even N400 studies. Such a neural correlate of rapid word form learning emerging within minutes of passive perceptual exposure confirms that our brain may effectively form new linguistic memory circuits online, as it gets exposed to novel speech patterns in the sensory input.
A similar result of a rapidly increased activity for a novel pseudo-word has been demonstrated earlier (Shtyrov et al., 2010b). However, the important advance in the current study is that it used multiple tokens of word and pseudo-word stimuli presented within the natural range of speech rate, thus offering a much stronger experimental base for this phenomenon. Furthermore, here we have also employed a non-speech control stimulus set. Although the stimuli it included were highly similar acoustically to the speech syllables, they generated a different ERP dynamics in general and, most importantly, did not exhibit any learning-related changes. The latter suggests that although the human capacity to rapidly learn new words may have common roots with animal learning mechanisms (Kaminski et al., 2004), it appears to have developed into a sophisticated neural machinery specific to language learning. Even if rapid learning is not specific to human language function (as it has been argued by, e.g., Markson and Bloom, 1997) and may be an expression of a more general neurobiological learning mechanism, the extremely efficient application of this mechanism to the learning of vocabularies of thousands of words is, of course, a human feature. This feature is potentially facilitated by human-specific neuroanatomical advantages in the form of efficient connections within left temporo-frontal perisylvian networks (Catani et al., 2005; Saur et al., 2008).
Indeed, left-hemispheric temporo-frontal structures were indicated as playing the dominant part in the rapid learning of novel words in the current study. Although our source analysis here was based on grand-average data and thus not verifiable statistically, these structures were also indicated by previous metabolic imaging studies of fast mapping (Majerus et al., 2005; Rauschecker et al., 2008; Paulesu et al., 2009). The brain structures engaged by such rapid passive word form learning are part of those also effective in the processing of meaningful words, such as superior temporal cortex included in the “what” stream of auditory processing (Rauschecker and Scott, 2009). Partial involvement of the right hemisphere that is suggested by the source analysis here has also been shown before, specifically a strong involvement of right inferior-frontal gyrus in fast mapping of novel words as seen in fMRI (Breitenstein et al., 2005) is confirmed by the current source analysis results. Importantly, the present study along with the earlier studies we have reviewed above makes a strong case for a network of neocortical areas that take part in online word acquisition and that may include most notably perisylvian structures of the left hemisphere (temporal lobe, inferior-frontal gyrus), as well as temporo-parietal, premotor, and prefrontal regions. This network may be underpinning a neocortical “fast track” for word acquisition which subserves the vital function of rapid language learning not directly dependant on long-term consolidation processes traditionally linked to hippocampus (McClelland et al., 1995; Born et al., 2006). This suggestion is well supported by a recent neuropsychological investigation showing a near-normal fast mapping ability in patients with severely damaged hippocampus that critically depends on intact left temporal cortex (Sharon et al., 2011).
In addition to supporting the previously made notion of rapid (∼100 ms) lexical effects in auditory ERPs that can also be used for tracking word memory trace formation, this study has shown three noticeable differences from the earlier investigations. First, in at least one previous similar study that demonstrated such an effect, it had a negative surface polarity (Shtyrov et al., 2010b), whereas here the entire action is occurring on the positive end of the voltage scale, although the fronto-central distribution largely remains the same. This is likely explained by differences in the paradigm we employed: whilst the previous investigation used an oddball single token approach and monosyllabic stimuli, here were presented a selection of different bi-syllabic items mixed equiprobably. The higher (and more natural) rate of stimulus presentation here, along with the analysis focus on the second syllables may mean that the negativity usually seen at this latency is greatly suppressed due to habituation resultant from continuous auditory stimulation (Rosburg et al., 2006). In time, the effects seem to generally correspond to the traditional N100 latency range as well as the time when lexical MMN effects have been demonstrated, and could thus be related to these auditory ERPs; however, the unusual polarity dynamics call for future exploration of these effects’ neural origins. Interestingly, in at least one earlier EEG experiment on rapid language learning, an increase in frontal positivity with peak latency shortly before 200 ms (i.e., P2 range) has also been observed, but it was linked to rule acquisition rather than word learning processes (De Diego Balaguer et al., 2007).
Second, the results suggested a later peak for the pseudo-word response (particularly noticeable in the end of the learning exposure, Figures 2 and 3) than for the word-elicited ERP. Although this difference was only marginally supported by statistics (p = 0.06), it indicates a potentially interesting phenomenon. Recent studies into automatic activation of memory traces for spoken words of different lexical frequencies suggest that less frequently used items possess less integrated memory traces and therefore take longer to activate; this activation lag manifests itself as a delayed peak latency of corresponding ERP responses (Aleksandrov et al., 2011; Shtyrov et al., 2011). The current findings are in line with this: as the novel word forms are certainly not a frequently used item in the subjects’ lexicon, intrinsic neural connections in their newly formed memory circuits cannot be as strong as those for the previously known words, which may be a reason for the lag in activation.
Finally, it appears that the pseudo-word activation in size overtakes that elicited by words in the end of the recording session. Although this effect does not reach significance, it may be an additional sign of the ongoing learning process: novel auditory stimuli early in the process of learning have been shown to produce a larger-scale activation, whilst at later stages tuning of neural representations takes place which optimizes the use of neural resources and prunes unnecessarily activation (Kujala et al., 2003).
Here, we used a passive non-attend paradigm approach which has been repeatedly shown to be a sensitive tool for recording lexical memory trace activations (Shtyrov and Pulvermüller, 2007), which also seems to be the case in the current study. Although the lack of attention to stimuli may be suggestive of certain automaticity in the learning process, this issue was not specifically under investigation here and remains to be explored in future studies which could achieve this by systematically modulating attention on stimuli and manipulating stimulus-related tasks.
We have recorded event-related potentials elicited in the brain by novel spoken word forms as they are being learnt through passive auditory exposure. We observed a dramatic change in the brain response dynamics within the short exposure session: as the subjects become familarized with the novel word forms, the early (∼100 ms) fronto-central activity they elicit increases in magnitude and becomes similar to that of previously known real words. Acoustically similar real words used as control stimuli show a stable response throughout the recording session, a sign of robustness of existing linguistic representations. Acoustically matched novel non-speech stimuli do not demonstrate a learning-related response increase, suggesting neural specificity of the rapid learning phenomenon to language. These results suggest that the human brain may efficiently form new cortical circuits online, as it gets exposed to novel linguistic patterns in the sensory input. Left-lateralized perisylvian neocortical networks appear to be underlying such fast mapping of novel word forms unto the brain’s mental lexicon.
The author declares that the research was conducted in the absence of any commercial or financial relationships that could be construed as a potential conflict of interest.
Yury Shtyrov is supported by the Medical Research Council (MRC), UK (U.1055.04.014.00001.01, MC_US_A060_0043). The study was supported by the MRC, University of Helsinki, and Academy of Finland. The author wishes to thank Teija Kujala, Pasi Piiparinen, and Friedemann Pulvermüller for their help at different stages of this study.
Aleksandrov, A. A., Boricheva, D., Pulvermüller, F., and Shtyrov, Y. (2011). Strength of word-specific neural memory traces assessed electrophysiologically. PLoS ONE 6, e22999. doi: 10.1371/journal.pone.0022999
Borovsky, A., Kutas, M., and Elman, J. (2010). Learning to use words: event-related potentials index single-shot contextual word learning. Cognition 116, 289–296.
Breitenstein, C., Jansen, A., Deppe, M., Foerster, A. F., Sommer, J., Wolbers, T., and Knecht, S. (2005). Hippocampus activity differentiates good from poor learners of a novel lexicon. Neuroimage 25, 958–968.
Carey, S., and Bartlett, E. (1978). Acquiring a single new word. Papers Rep. Child Lang. Dev. 15, 17–29.
Catani, M., Jones, D. K., and ffytche, D. H. (2005). Perisylvian language networks of the human brain. Ann. Neurol. 57, 8–16.
Davis, M. H., and Gaskell, M. G. (2009). A complementary systems account of word learning: neural and behavioural evidence. Philos. Trans. R. Soc. Lond. B Biol. Sci. 364, 3773–3800.
De Diego Balaguer, R., Toro, J. M., Rodriguez-Fornells, A., and Bachoud-Lévi, A.-C. (2007). Different neurophysiological mechanisms underlying word and rule extraction from speech. PLoS ONE 2, e1175. doi: 10.1371/journal.pone.0001175
Dollaghan, C. (1985). Child meets word: “fast mapping” in preschool children. J. Speech Hear. Res. 28, 449–454.
Friederici, A. (2002). Towards a neural basis of auditory sentence processing. Trends Cogn. Sci. (Regul. Ed.) 6, 78–84.
Garagnani, M., Shtyrov, Y., and Pulvermüller, F. (2009). Effects of attention on what is known and what is not: MEG evidence for functionally discrete memory circuits. Front. Hum. Neurosci. 3:10. doi: 10.3389/neuro.09.010.2009
Gaskell, M. G., and Dumay, N. (2003). Lexical competition and the acquisition of novel words. Cognition 89, 105–132.
Kaminski, J., Call, J., and Fischer, J. (2004). Word learning in a domestic dog: evidence for “fast mapping.” Science 304, 1682–1683.
Kujala, A., Huotilainen, M., Uther, M., Shtyrov, Y., Monto, S., Ilmoniemi, R. J., and Näätänen, R. (2003). Plastic cortical changes induced by learning to communicate with non-speech sounds. Neuroreport 14, 1683–1687.
Majerus, S., Van der Linden, M., Collette, F., Laureys, S., Poncelet, M., Degueldre, C., Delfiore, G., Luxen, A., and Salmon, E. (2005). Modulation of brain activity during phonological familiarization. Brain Lang. 92, 320–331.
Markson, L., and Bloom, P. (1997). Evidence against a dedicated system for word learning in children. Nature 385, 813–815.
McClelland, J. L., McNaughton, B. L., and O’Reilly, R. C. (1995). Why there are complementary learning systems in the hippocampus and neocortex: insights from the successes and failures of connectionist models of learning and memory. Psychol. Rev. 102, 419–457.
Mestres-Misse, A., Rodriguez-Fornells, A., and Munte, T. F. (2007). Watching the brain during meaning acquisition. Cereb. Cortex 17, 1858–1866.
Oldfield, R. C. (1971). The assessment and analysis of handedness: the Edinburgh inventory. Neuropsychologia 9, 97–113.
Paulesu, E., Vallar, G., Berlingeri, M., Signorini, M., Vitali, P., Burani, C., Perani, D., and Fazio, F. (2009). Supercalifragilisticexpialidocious: how the brain learns words never heard before. Neuroimage 45, 1368–1377.
Pittman, A. L. (2008). Short-term word-learning rate in children with normal hearing and children with hearing loss in limited and extended high-frequency bandwidths. J. Speech Lang. Hear. Res. 51, 785–797.
Pulvermüller, F., and Shtyrov, Y. (2006). Language outside the focus of attention: the mismatch negativity as a tool for studying higher cognitive processes. Prog. Neurobiol. 79, 49–71.
Pulvermüller, F., and Shtyrov, Y. (2009). Spatiotemporal signatures of large-scale synfire chains for speech processing as revealed by MEG. Cereb. Cortex 19, 79–88.
Pulvermüller, F., Shtyrov, Y., and Hauk, O. (2009). Understanding in an instant: neurophysiological evidence for mechanistic language circuits in the brain. Brain Lang. 110, 81–94.
Rauschecker, A. M., Pringle, A., and Watkins, K. E. (2008). Changes in neural activity associated with learning to articulate novel auditory pseudowords by covert repetition. Hum. Brain Mapp. 29, 1231–1242.
Rauschecker, J. P., and Scott, S. K. (2009). Maps and streams in the auditory cortex: nonhuman primates illuminate human speech processing. Nat. Neurosci. 12, 718–724.
Rosburg, T., Trautner, P., Boutros, N. N., Korzyukov, O. A., Schaller, C., Elger, C. E., and Kurthen, M. (2006). Habituation of auditory evoked potentials in intracranial and extracranial recordings. Psychophysiology 43, 137–144.
Saur, D., Kreher, B. W., Schnell, S., Kummerer, D., Kellmeyer, P., Vry, M. S., Umarova, R., Musso, M., Glauche, V., Abel, S., Huber, W., Rijntjes, M., Hennig, J., and Weiller, C. (2008). Ventral and dorsal pathways for language. Proc. Natl. Acad. Sci. U.S.A. 105, 18035–18040.
Sharon, T., Moscovitch, M., and Gilboa, A. (2011). Rapid neocortical acquisition of long-term arbitrary associations independent of the hippocampus. Proc. Natl. Acad. Sci. U.S.A. 108, 1146–1151.
Shtyrov, Y. (2010). Automaticity and attentional control in spoken language processing: neurophysiological evidence. Ment. Lex. 5, 255–276.
Shtyrov, Y., Kimppa, L., Pulvermuller, F., and Kujala, T. (2011). Event-related potentials reflecting the frequency of unattended spoken words: a neuronal index of connection strength in lexical memory circuits? Neuroimage 55, 658–668.
Shtyrov, Y., Kujala, T., and Pulvermüller, F. (2010a). Interactions between language and attention systems: early automatic lexical processing? J. Cogn. Neurosci. 22, 1465–1478.
Shtyrov, Y., Nikulin, V. V., and Pulvermüller, F. (2010b). Rapid cortical plasticity underlying novel word learning. J. Neurosci. 30, 16864–16867.
Shtyrov, Y., Pihko, E., and Pulvermüller, F. (2005). Determinants of dominance: is language laterality explained by physical or linguistic features of speech? Neuroimage 27, 37–47.
Shtyrov, Y., and Pulvermüller, F. (2007). Language in the mismatch negativity design: motivations, benefits and prospects. J. Psychophysiol. 21, 176–187.
Valo, M. (1994). Käsitykset ja vaikutelmat äänestä. Kuuntelijoiden arviointia radiopuheen äänellisistä ominaisuuksista. Jyväskylä: Jyväskylän yliopisto.
Keywords: brain, cortex, language, word, event-related potential, electroencephalography, lexical memory trace, fast mapping
Citation: Shtyrov Y (2011) Fast mapping of novel word forms traced neurophysiologically. Front. Psychology 2:340. doi: 10.3389/fpsyg.2011.00340
Received: 09 August 2011; Paper pending published: 26 August 2011;
Accepted: 01 November 2011; Published online: 21 November 2011.
Edited by:
Andriy Myachykov, University of Glasgow, UKReviewed by:
Andriy Myachykov, University of Glasgow, UKCopyright: © 2011 Shtyrov. This is an open-access article subject to a non-exclusive license between the authors and Frontiers Media SA, which permits use, distribution and reproduction in other forums, provided the original authors and source are credited and other Frontiers conditions are complied with.
*Correspondence: Yury Shtyrov, Cognition and Brain Sciences Unit, Medical Research Council, 15 Chaucer Road, CB2 7EF Cambridge, UK. e-mail:eXVyeS5zaHR5cm92QG1yYy1jYnUuY2FtLmFjLnVr
Disclaimer: All claims expressed in this article are solely those of the authors and do not necessarily represent those of their affiliated organizations, or those of the publisher, the editors and the reviewers. Any product that may be evaluated in this article or claim that may be made by its manufacturer is not guaranteed or endorsed by the publisher.
Research integrity at Frontiers
Learn more about the work of our research integrity team to safeguard the quality of each article we publish.