- Neuroscience Center, University of Helsinki, Helsinki, Finland
Alpha-frequency band (8–14 Hz) oscillations are among the most salient phenomena in human electroencephalography (EEG) recordings and yet their functional roles have remained unclear. Much of research on alpha oscillations in human EEG has focused on peri-stimulus amplitude dynamics, which phenomenologically support an idea of alpha oscillations being negatively correlated with local cortical excitability and having a role in the suppression of task-irrelevant neuronal processing. This kind of an inhibitory role for alpha oscillations is also supported by several functional magnetic resonance imaging and trans-cranial magnetic stimulation studies. Nevertheless, investigations of local and inter-areal alpha phase dynamics suggest that the alpha-frequency band rhythmicity may play a role also in active task-relevant neuronal processing. These data imply that inter-areal alpha phase synchronization could support attentional, executive, and contextual functions. In this review, we outline evidence supporting different views on the roles of alpha oscillations in cortical networks and unresolved issues that should be addressed to resolve or reconcile these apparently contrasting hypotheses.
Introduction
Human perceptual and mental experiences are serial, introspectively coherent, and have a limited capacity of three to four objects (Luck and Vogel, 1997; Marois et al., 2004; Vogel and Machizawa, 2004). Yet, the underlying neuronal processing is parallel, distributed, and has a very large capacity. The systems-level neuronal mechanisms that bind scattered neuronal activity into serial, capacity-limited cognitive operations have remained poorly understood.
A large body of data suggests that synchronized neuronal oscillations could serve such a binding role through coordination and regulation of both local processing and inter-areal interactions (Womelsdorf et al., 2006; Womelsdorf and Fries, 2007; Lakatos et al., 2008; Cardin et al., 2009; Singer, 2009). Two physiological mechanisms underlie the putative functional significance of neuronal synchronization and oscillations. First, synchronized synaptic excitation is much more likely to evoke an action potential in a downstream neuron than temporally uncorrelated inputs. Synchronization thus endows a neuronal population an advantage in the competition of engaging the target neurons (Singer, 1999; Uhlhaas et al., 2009). Second, rhythmic membrane potential fluctuations enhance neuronal excitability (Pastor et al., 2002) in one phase of a cycle and suppress it in the other. Therefore, oscillations impose excitability windows that are in a position to be powerful temporal regulators of neuronal activity and to thereby facilitate inter-areal interactions with appropriate phase differences while suppressing inputs from uncorrelated or out-of-phase assemblies. Neuronal oscillations, in general, may thus play the key mechanistic role in coordinating inter-areal communication (Fries, 2005) and neuronal collectivity, and thereby mechanistically underlie the integration of anatomically distributed neuronal activity into coherent cognitive states.
Much of the work on neuronal synchronization has focused on beta (14–30 Hz) and gamma (30–200 Hz) frequency band activities. In the lower frequency regime, delta (1–4 Hz), theta (4–8 Hz), and alpha (8–14 Hz) frequency band oscillations appear to be able to support long-distance cortical interactions and would thus be particularly suitable for large-scale cognitive integration (Kopell et al., 2000; von Stein et al., 2000; Palva et al., 2005a). Interestingly, their period is also in the time-scale of perceptual, cognitive, and motor operations as reflected in a body of psychophysical data (VanRullen and Koch, 2003; Palva and Palva, 2007). While several lines of evidence indeed link at least local delta- (Lakatos et al., 2008) and theta-band (Romei et al., 2011) rhythmicity with active neuronal processing for cognitive functions, the data on local amplitude dynamics of alpha-band oscillations suggest an opposite role.
In this review, we explore some recent studies addressing the functional correlates of alpha-band phase and amplitude dynamics both in local and large-scale cortical networks from the viewpoints of two alternative, albeit not mutually incompatible, hypotheses: the inhibition hypothesis and the active-processing hypothesis. We advance here a framework for reconciling these views and suggest that the characterization of alpha-band inter-areal and cross-frequency (CF) phase interactions is critical for understanding the functional significance of alpha oscillations in human brain.
Inhibition vs. Active-Processing Hypotheses – Possibilities for a Unified Framework?
The functional role of local alpha-band oscillations is widely considered to be the inhibition of corresponding local cortical processing. Phenomenologically, large-amplitude alpha oscillations appear to suppress task-irrelevant processing and small-amplitude alpha to reflect an overall disinhibited state where active-processing is facilitated (Ray and Cole, 1985; Pfurtscheller, 2003; Klimesch et al., 2007). Mechanistically, this is thought to be achieved in a phasic or “pulsing” manner, where one phase of the alpha cycle is associated with gross inhibition of neuronal activity in the underlying local cortical circuitry so that the effectivity of this inhibition is correlated with the magnitude of the macroscopic alpha oscillation (Klimesch et al., 2007; Mathewson et al., 2009; Jensen and Mazaheri, 2010). Functionally, this kind of inhibition could play a role in gating information flows by imposing an attentionally controlled “closed gate” to task-irrelevant information (Gould et al., 2011; Haegens et al., 2011). Along the lines of this “inhibition hypothesis,” several studies indeed show both anticipatory and post-stimulus alpha-band amplitude decreases from baseline levels in task-relevant regions and increases in the presumably task-irrelevant regions according to perceptual, attentional, and working memory task demands (for reviews, see Klimesch et al., 2007; Jensen and Mazaheri, 2010). The task-relevant regions are typically considered to be sensory regions in the hemisphere contralateral to the task-relevant stimuli (Thut et al., 2006; Klimesch et al., 2007; Jensen and Mazaheri, 2010). The enhancement of alpha oscillations is also correlated with successful suppression of task-irrelevant information at the behavioral level (Sauseng et al., 2009). Trans-cranial magnetic stimulation (TMS) studies corroborate this notion by showing that exogenously evoked alpha oscillations in task-relevant sensory regions suppress perceptual performance (Romei et al., 2010) and that a disruption of anticipatory alpha amplitude suppression by TMS degrades behavioral performance (Capotosto et al., 2009). Moreover, alpha-band TMS of regions ipsilateral to task-relevant sensory information and contralateral to distractors supports distractor suppression and enhances the behavioral outcome (Sauseng et al., 2009; Romei et al., 2010).
Converging evidence thus indicates that the amplitude of alpha-band oscillations is negatively correlated with cortical excitability and task performance in sensory cortices (see also Romei et al., 2008) and, as reflected in the blood-oxygenation level dependent (BOLD) signal in functional magnetic resonance imaging (fMRI) recordings, also in the dorsal attention network (Laufs et al., 2003; Sadaghiani et al., 2010). It may, however, be premature to conclude that alpha oscillations serve exclusively an inhibitory purpose. As discussed below, alpha-band oscillations in several cognitive tasks are enhanced in frontal and parietal regions as well as in sensory regions high in the processing hierarchy (Jensen et al., 2002; Palva et al., 2005a, 2011; Haegens et al., 2011; Mo et al., 2011). In many cases, categorization of these high-level regions as task-relevant or irrelevant is not straightforward and therefore the amplitude increase can be interpreted to reflect either inhibition of task-irrelevant activity or integration of task-relevant processing per se. Moreover, a majority of research on alpha oscillations has used the oscillation’s amplitude as an index of synchronization in the underlying neuronal population. Many factors other than synchronization can influence the macroscopic oscillation amplitudes and the amplitudes cannot index long-range synchronization. Hence it is not easy to draw physiological conclusions from amplitude data. In fact, an accumulating body of data shows that alpha amplitude suppression may be associated with a concurrent increase in inter-areal alpha-band phase synchrony (Freunberger et al., 2008, 2009; Doesburg et al., 2009; Palva et al., 2010) of which the behavioral relevance implies an active role for alpha rhythmicity in cognitive operations. This possibility can be formulated into an “active-processing hypothesis” where the phase dynamics of alpha oscillations play a role in coordinating neuronal processing also in task-relevant cortical structures (von Stein et al., 2000; Palva and Palva, 2007). In this framework, the inter-areal alpha phase interactions directly support the neuronal processing underlying higher-level attentional, executive, and task-set maintenance functions.
In this review, we suggest that the inhibition and active-processing hypotheses could be reconciled by considering that inhibition at lower sensory and motor levels is, in fact, a key property of the higher-level frontal functions (Aron et al., 2004; Congdon et al., 2010), and could be mechanistically achieved by top-down alpha phase interactions.
Alpha Phase Dynamics Reflect Periodic Excitability Fluctuations and Predict Behavioral Performance
A growing body of electroencephalography (EEG) data shows that the phase of local theta- and alpha-frequency oscillations prior to the stimulus onset influences both the subsequent neuronal responses and stimulus perception (Busch et al., 2009; Mathewson et al., 2009, 2010; Busch and VanRullen, 2010). This observation is salient in theta/low-alpha-bands in threshold-stimulus detection tasks (TSDTs; Palva and Palva, 2011) in the visual modality (Busch et al., 2009; Mathewson et al., 2009; Busch and VanRullen, 2010), where stimuli arriving at one phase of the ongoing oscillation are more likely to reach sensory awareness than stimuli presented at the opposite phase. A similar effect in the alpha-band has also been reported in a visual entrainment task (Mathewson et al., 2010) although in the latter, it may be biased by concurrent amplitude and event-related dynamics. These systems-level data are thus in line with the cellular-level concept of neuronal oscillations reflecting cyclic membrane potential fluctuations and alternating phases of low and high excitability. Importantly, in the theta/low-alpha-band, the phase bias phenomenon is stronger for the attended than for the ignored visual hemifield (Busch and VanRullen, 2010), which is in line with the active-processing hypothesis and suggests that cyclic excitability fluctuations occur in task-relevant cortical regions and underlie periodic perceptual processing. Alternatively, if this periodicity was attributable to oscillations in task-irrelevant brain regions (Klimesch et al., 2007; Mathewson et al., 2009; Jensen and Mazaheri, 2010), it could be interpreted to reflect a periodic leakage of irrelevant information at un-inhibited phases that hinders task-relevant neuronal processing.
These studies thus support the idea that the alpha-, along with delta-, theta-, and beta-band phase dynamics impose time-windows that segment the neuronal processing underlying perceptual, attentional, cognitive, and motor actions into discrete periods. A similar concept was, in fact, proposed already in 1947 by Pitts and McCulloch and in 1948 by Wiener who suggested that alpha oscillations could reflect a clock-like scanning rhythm that serves a gating function (Wiener, 1948). Later Nunn and Osselton (1974) considered alpha-phase-related perceptual modulations to imply a shutter-like mechanism.
In addition to biasing the detection probability of weak sensory stimuli, alpha-band phase dynamics modulate also the speed of processing. Reaction times (RTs) to flashes were in the 1960s found to depend of the phase of the posterior alpha cycle (Callaway and Yeager, 1960). More recently, also saccadic RTs were found to be modulated by the alpha phase prior to target detection (Drewes and Vanrullen, 2011). Alpha phase affected the saccadic RTs also in several cognitive tasks with varying task difficulties so that in a difficult task, the effect was most pronounced in subjects with fast RTs. On the other hand, another study found that fast saccades are associated with weaker inter-trial alpha coherence than the slower saccades (Hamm et al., 2010), although in this study, the task was easy and not directly comparable to the previous study.
Finally, as independent observations of the cortical excitability fluctuations in the alpha-band, the pre-stimulus alpha phase is correlated with the magnitude of a visually evoked BOLD response in early visual regions (Scheeringa et al., 2011) as well as with the strength of the evoked responses in EEG (Brandt et al., 1991; Barry et al., 2004). Taken together, these data demonstrate that alpha, like oscillations in other frequency bands, underlies cyclic modulations of neuronal excitability. However, depending on the view taken, the data support both the inhibition and the active-processing hypothesis because the cortical regions underlying the observed phase effects have not been identified so far. MEG/EEG source modeling will be important in further studies on this issue.
Local Field Potential and Multi-Unit Activity Recordings Reveal Functionally Distinct Alpha-Band Oscillations
Unlike non-invasive MEG and EEG recordings, local field potential (LFP) and multi-unit activity (MUA) recordings provide valuable information of cellular-level characteristics of alpha-band oscillations. Concurrent LFP and MUA recordings reveal how the phase and amplitude of ongoing LFP oscillations are correlated with MUA in different cortical regions and layers. While LFP rhythmicity reflects local synchronized neuronal population activity that could be related to oscillations recorded at the scalp level, MUA can be considered to be an indicator of gross cortical excitability.
Lateral geniculate nucleus (LGN) is a key thalamic region in the transmission of visual information to the cortex and LGN and the thalamo-cortical (TC) neurons therein exhibit intrinsic burst firing at alpha frequencies, which was thought originally to act as a pacemaker rhythm for cortical alpha oscillations (Andersen, 1968). Later studies showed that thalamic alpha-band burst firing may occur both in synchrony with and independently of cortical EEG alpha-band oscillations (Lopes da Silva et al., 1973, 1974, 1980). Because thalamic burst firing was thought to lead to unreliable and imprecise information transmission (Silva et al., 1991), alpha-band activity was suggested to reflect a “closed” thalamic gate (Pfurtscheller, 2003). Several recent studies have shown that thalamus is indeed a key region in coordinating the cortical alpha-band activity (Hughes et al., 2004; Hughes and Crunelli, 2007) but also that alpha is not necessarily associated with all-or-none gating of information transmission. First, thalamic neurons relay information also in the bursting mode (Sherman, 2001; Nicolelis and Fanselow, 2002). Second, thalamic high threshold bursting is associated with EEG alpha oscillations (Hughes et al., 2004; Hughes and Crunelli, 2007). Third, in the LGN relay mode, TC neurons may divide into two anticorrelated neuronal coalitions, one of which shows cyclic suppression in the negative and the other in the positive alpha peak (Lorincz et al., 2009). Alpha-band oscillations may thus support qualitatively different modes of information transmission and may be concurrently associated with both suppression of communication and active information processing.
At the cortical level, recordings from visual regions V2, V4, and inferotemporal (IT) of behaving monkeys show that the MUA and gamma-band activity are correlated with alpha phase, which further corroborates that alpha oscillations reflect periodic excitability fluctuations (Bollimunta et al., 2008; Mo et al., 2011). This kind of a correlation is evident also in human electro-corticogram recordings where the alpha phase is correlated with gamma-frequency band amplitude fluctuations (Voytek et al., 2010; Maris et al., 2011). Several lines of data show that alpha oscillations in the early visual regions, V1, V2, and V4 are mechanistically different from those in a higher-order visual regions of the IT cortex (Bollimunta et al., 2008, 2011). In early visual regions, the infra-granular layer acts as a pacemaker driving the granular and supra-granular layers whereas in IT, the supra-granular layer has the driver function. Moreover in V1, the alpha rhythm is driven by a cortico-thalamo-cortical loop whereas in V2 and V4, cortico-cortical interactions dominate. (Bollimunta et al., 2011). In line with the inhibition hypothesis, alpha amplitude in V1, V2, and V4 was positively correlated with behavioral performance in an auditory task. However, in IT, the alpha amplitude was negatively correlated with the behavioral outcome, which indirectly supports an active attentional rather than a distractor-suppressing role for alpha in IT (Bollimunta et al., 2008). Direct evidence for this notion was obtained more recently in a study where the alpha oscillations were observed in LFP recordings in IT during an intermodal selective attention task (Mo et al., 2011). Alpha amplitude in IT was stronger during visual than during auditory attention and was positively correlated with both MUA and local gamma-band responses. These data indicate that in this high-level visual region, the alpha oscillations play an active and task-relevant role.
These data thus show that both the mechanisms and functional roles of alpha oscillations may vary along the cortical processing hierarchy. Specifically, there appears to be a mechanistic transition so that active-processing in early sensory regions is associated with decreased alpha amplitudes and attenuated synchronization (Bollimunta et al., 2008, 2011) whereas in attentional, executive, and higher-level sensory regions, alpha amplitude (Mo et al., 2011), and synchronization (von Stein et al., 2000) are positively correlated with task-related neuronal processing and local cortical excitability.
Post-Stimulus Alpha-Band Amplitude Dynamics and Their Correlation with Task Performance
Alpha amplitude is typically decreased after the sensory stimuli in cortical regions where the stimuli are processed. This amplitude decrease, also called event-related desynchronization, is often taken to reflect a state of increased neuronal excitability or disinhibition (Pfurtscheller, 2003). It has also been long known that internally oriented processing such as working memory maintenance may enhance rather than suppress alpha-band amplitudes (Ray and Cole, 1985; Busch and Herrmann, 2003; Leiberg et al., 2006; Grimault et al., 2009; Haenschel et al., 2009), which has been suggested reflect response inhibition and/or inhibition of distracting sensory inputs or the maintenance of task-irrelevant distractor information (Ray and Cole, 1985; Jokisch and Jensen, 2007; Klimesch et al., 2007; Tuladhar et al., 2007).
Recent MEG studies show that alpha amplitudes are positively correlated with memory-load in working memory tasks and suggest that these oscillations originate from occipito-parietal brain regions (Jensen et al., 2002; Osipova et al., 2006; Medendorp et al., 2007). We have recently investigated oscillation amplitude modulations in a delayed-match-to-sample task in which subjects memorized a Sample stimulus containing one to six colored squares for a 1.1-s memory retention period (Figure 1A). At the end of the retention period, a Test stimulus was presented and the subjects indicated with a forced-choice response whether the squares in the Test were colored as in the Sample. We used combined MEG and EEG (M/EEG) to record ongoing neuronal activity and cortically constrained minimum-norm-estimates for single-trial source reconstruction. We found that during visual working memory (VWM) maintenance, alpha-band amplitudes were load dependently strengthened in fronto-parietal regions together with beta- and gamma-band amplitudes, despite being load dependently suppressed in the visual regions (Palva et al., 2011; Figure 1B). The co-localization of alpha with beta and gamma oscillations that often are associated with active information processing (Fries, 2009; Singer, 2009) is also in line with the above mentioned recordings from monkey IT where alpha-band amplitudes were associated with active task-relevant neuronal processing (Mo et al., 2011). In addition, since frontal and parietal regions are thought to underlie attentional and central executive functions in VWM as indexed by fMRI (Prabhakaran et al., 2000; Rowe et al., 2000; Sakai et al., 2002; Curtis and D’Esposito, 2003; Petrides, 2005) as well as lesion (Voytek and Knight, 2010) studies, the fronto-parietal localization of the positive memory-load dependence of alpha-band oscillations is suggestive of an attentional functionality in VWM retention. In contrast to the alpha-band, beta-, and gamma-band amplitudes were load dependently strengthened also in several visual regions suggesting that they support the maintenance of visual information per se in the VWM. This finding further corroborates that in the lower levels of cortical hierarchy decreased alpha-band amplitudes may reflect cortical excitation associated with stimulus processing.
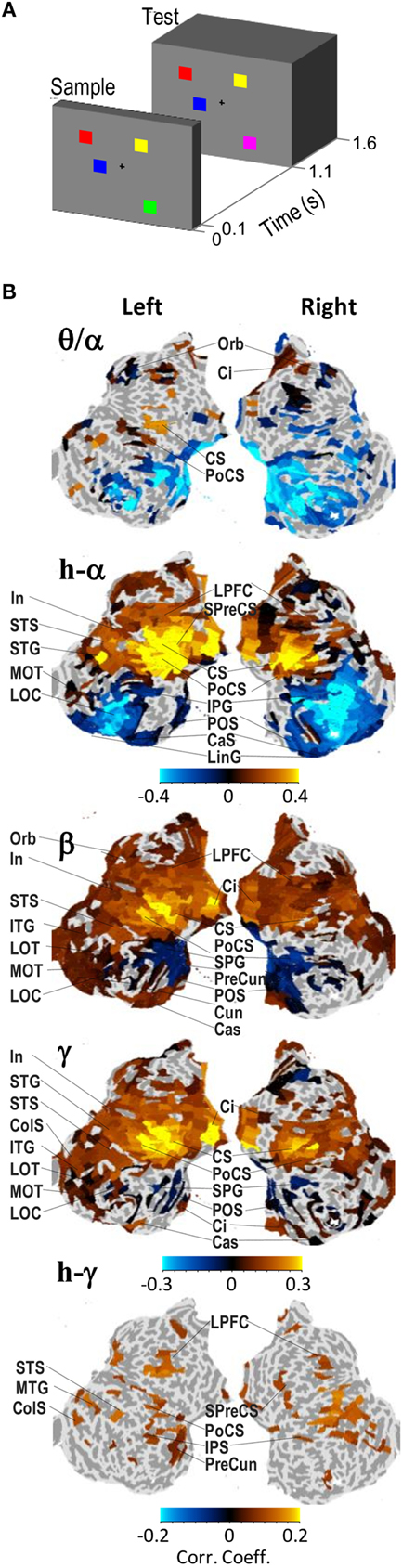
Figure 1. Alpha-, beta-, and gamma-band amplitudes are positively correlated with visual working memory (VWM) load in fronto-parietal cortical regions during the retention period. (A) A schematic representation of the VWM paradigm in this study. The subjects sustained a memory of the Sample stimulus and indicated with a force-choice response whether the Test stimulus was identical to the Sample or not. The stimuli contained one to six colored squares in random locations and in the 50% of Test stimuli, the color of one square was different from the color in the Sample stimulus. (B) Cortical localization of the memory-load dependence of oscillation amplitudes during the memory retention period (0.4–1 s). The complete cortical surfaces are flattened and oriented so that the anterior–posterior axis corresponds to up–down direction, respectively. The color values indicate the correlation coefficients obtained across subjects of the oscillation amplitude with the number of objects in the stimuli. The correlation values are shown for cortical regions that were significantly correlated with memory-load. Adapted from Palva et al. (2011).
Alpha-band amplitude may also be increased in other attention demanding tasks. In recent years, several studies have shown that the capacity of and the ability to coordinate visual attention (Green and Bavelier, 2003; Rueda et al., 2005) and working memory (Buschkuehl et al., 2008; Jaeggi et al., 2008) may be enhanced by computerized cognitive training for example by video games in healthy adults. Intriguingly, alpha-band power has recently been shown to be increased after video game training period in dual task performance that required the subjects to enhanced attentional switching (Maclin et al., 2011). This result is in line with observation of a positive correlation of alpha oscillations with BOLD signal (Sadaghiani et al., 2010) in the inferior frontal, insular, and cingulate cortices that play a key role in this cognitive function (Dosenbach et al., 2008) as well as with our observations that these regions can become transiently coupled by alpha-band phase synchronization with specific task-relevant networks (Palva et al., 2010a).
Local Alpha Phase Dynamics Reflect Task-Relevant Neuronal Processing
Several studies demonstrate that phase-locking of local ongoing alpha oscillations to stimulus onset, as measured with a phase-locking value or inter-trial coherence, is prominent in early sensory regions as well as in higher levels of the cortical hierarchy. Analysis-wise, such phase reorganization is not easy to differentiate from a phase bias caused by evoked responses. Nevertheless, the specificity of these phase effects to the alpha-band is an indication of the involvement of oscillatory mechanisms. For instance, in a somatosensory TSDT, alpha-band phase-locking to somatosensory stimuli presented at the threshold of detection is much stronger for the consciously perceived than for the unperceived stimuli in somatosensory regions (Palva et al., 2005b; Figure 2A). Moreover, alpha-band stimulus locking was observed exclusively for the detected stimuli in frontal and parietal sites. Another study shows that attention enhances both the phase-locked response and non-phase-locked alpha-band amplitude in the secondary somatosensory cortex (Dockstader et al., 2010). In VWM tasks, the alpha-band phase-locking is associated with the encoding of stimulus representations into VWM (Freunberger et al., 2009; Haenschel et al., 2010; Palva et al., 2011). The phase-locking of ongoing activity to VWM sample stimuli is anatomically more widespread than early amplitude increases or evoked responses at the same latency and involves also the frontal brain regions (Figure 2B; Palva et al., 2011). A phase reset of ongoing alpha oscillations could thus play a role in coordinating the large-scale fronto-parietal networks during VWM encoding. In this vein, the alpha-band phase-locking to memorized stimuli is lower in schizophrenic patients than in normal subjects so that the degradation of alpha-band phase-locking is correlated with the magnitude behavioral deficits in the patients (Haenschel et al., 2010). Post-stimulus alpha phase dynamics in local cortical networks are thus linked to both neuronal processing and behavioral performance in several cognitive tasks.
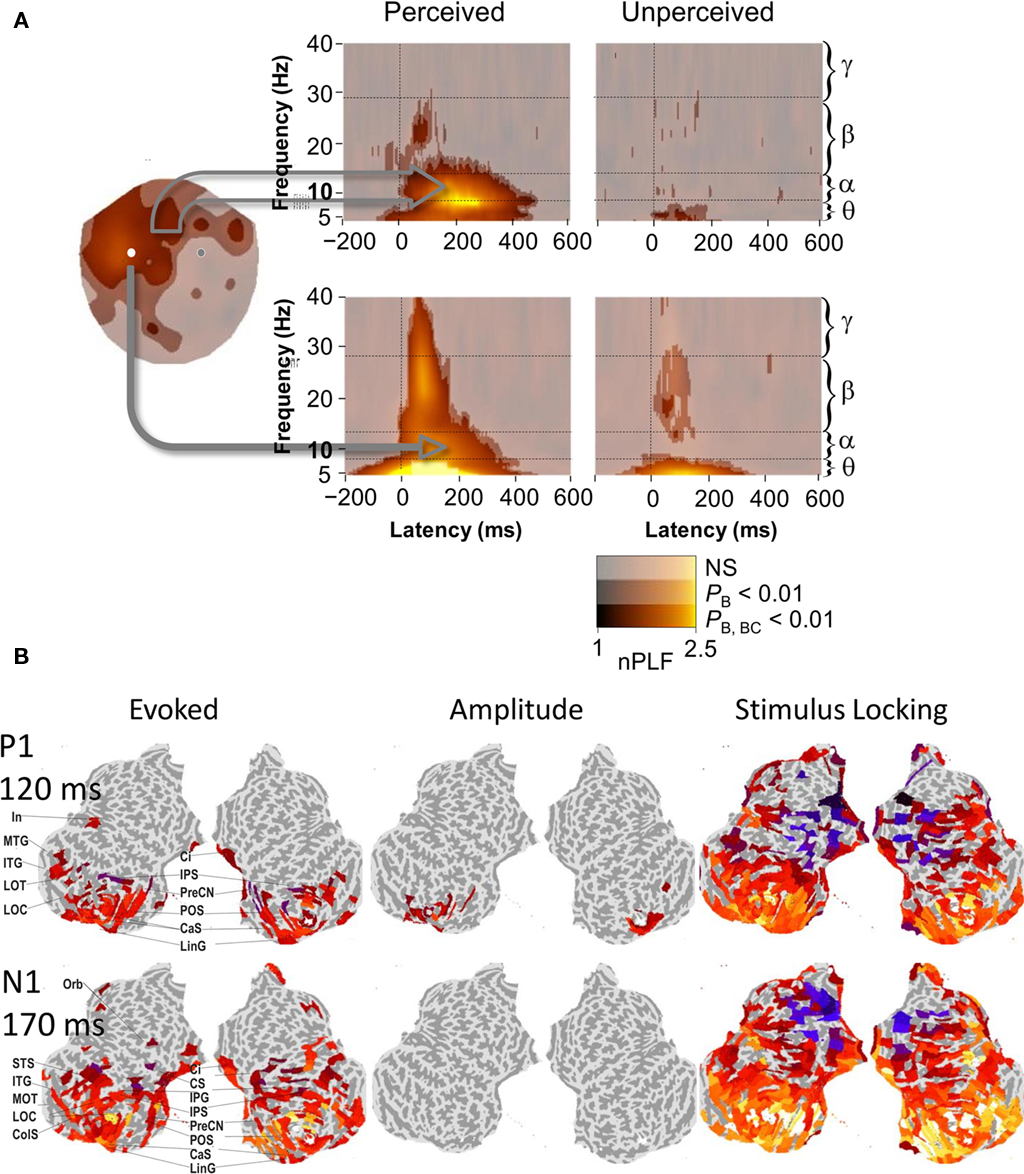
Figure 2. Post-stimulus phase dynamics of ongoing brain activity in frontal brain regions play a role in perception and working memory encoding. (A) Phase-locking of ongoing alpha, but not of theta or beta oscillations to threshold-level somatosensory stimuli dissociates conscious detection from unconscious stimulus processing. Cortically widespread phase-locking in the alpha-band was observed for perceived stimuli but not for the unperceived stimuli both in signals from a large set of MEG gradiometers over frontal regions (upper panel) as well as in signals from a single sensor over the putative contralateral primary somatosensory region (lower panel). Adapted from Palva et al. (2005b). (B) Cortical localization of evoked responses, positive oscillation amplitude modulations, and phase-locking to stimulus onset to Sample VWM stimuli (see Figure 1) of broadband (1–45 Hz) ongoing brain activity at the latencies of the P1 and N1 components of the visual evoked response. Unlike evoked responses (left panel), phase-locking (right panel) is observed in widespread and task-relevant frontal cortical regions. Conversely, enhanced oscillation amplitudes (middle panel), which are indicative of true stimulus-evoked additive components, are observed only in early visual regions. Color values indicate the response strength in significantly activated cortical regions. Adapted from Palva et al. (2011).
Alpha-Band Phase Synchrony in MEG and EEG is Correlated with Cognitive Task Performance
Converging evidence thus indicates that peri-stimulus cortical processing and behavioral outcome are correlated with alpha phase dynamics. However, neuronal processing is distributed anatomically and local phase dynamics conceivably must be coordinated across brain regions to support task-related processing. This coordination in alpha as well as in other frequency bands could be reflected in phase synchronization that is associated with a specific inter-areal phase difference, which subsequently facilitates or suppresses neuronal communication between these areas (Fries, 2005; Palva and Palva, 2007). However, only a few studies have explored inter-areal interactions in the alpha-band and their role in regulating inter-areal communication.
In a visuo-tactile integration task, the strength of occipito-temporal alpha-band coherence in EEG was positively correlated with psychophysical task performance at the single-trial level (Hummel and Gerloff, 2005). In an attention task where the subjects were cued to attend stimuli in one hemifield and ignore those in the other, the alpha-band phase synchrony was pronounced between task-relevant regions (inferior occipital gyrus and superior parietal lobule) in the hemisphere contralateral to the attended hemifield despite the concurrent amplitude suppression (Doesburg et al., 2009). Similar emergence of task-relevant long-range phase synchronization in the alpha-band with simultaneous local amplitude suppression was also observed to characterize successful memory maintenance (Freunberger et al., 2009) and during recognition of meaningful pictures (Freunberger et al., 2008). In an MEG study using a continuous mental calculation task involving parametric working memory-load and a resting-state condition, we observed that the working memory processing was associated with strengthening of phase synchrony in large-scale networks in alpha-, beta-, and gamma-bands (Figure 3). While these networks were qualitatively similar, the load dependent synchronization was most robust and involved the longest inter-areal distances in the alpha-band (Palva et al., 2005a). Taken together, several lines of MEG and EEG data point to the fact that long-range alpha-band phase synchrony is positively correlated with attentional and working memory functions. These studies are, however, based on MEG- and/or EEG-sensor-level analyses and thus yield only a limited insight into anatomical localization of the underlying cortical networks. In addition, inter-areal synchronization in MEG and EEG is confounded by volume conduction and signal mixing, which strongly bias the synchronization estimates. Finally, EEG and the different MEG sensor types have distinct sensitivities to cortical sources. MEG planar gradiometers are most sensitive to signals from superficial sulci whereas the MEG magnetometers and EEG have additional sensitivity to signals from gyral and deep sources. These issues can be tackled by M/EEG source modeling that can be used to merge the information from different sensor types, to acquire anatomical information about the neuronal sources, and to alleviate the signal-mixing-related problems.
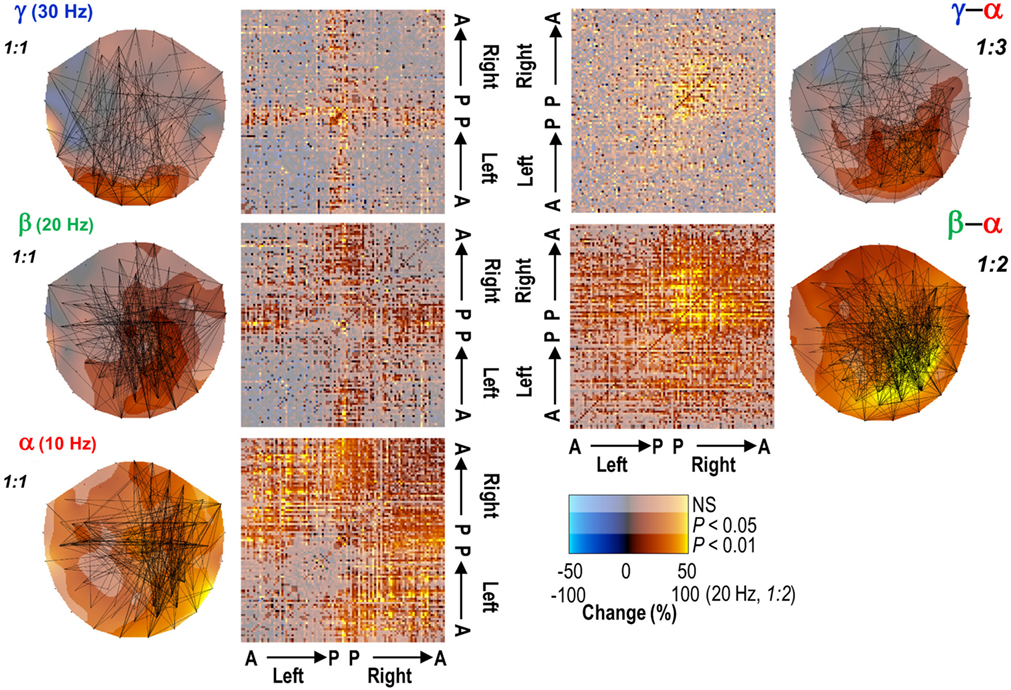
Figure 3. Long-range phase synchronization in the alpha- and to a lesser extent in the beta- and gamma-frequency bands is enhanced during the performance of a continuous working memory task (left panels) in planar MEG gradiometer data. Concurrent cross-frequency phase synchrony between the alpha- and beta-/gamma-frequency bands was also robustly enhanced (right panels). The connectivity matrices indicate the strength of synchronization relative to a rest condition in each MEG gradiometer pair (A, anterior; P, posterior). Adapted from Palva et al. (2005a).
In an MEG–fMRI visual recognition study, alpha-band phase synchronization between sources localized to the orbitofrontal cortex and fusiform gyrus was stronger in trials where a masked object was subsequently recognized than in those where the subject failed in the recognition task (Bar et al., 2006). The authors proposed that visual objects with low-spatial frequencies activated the dorsal visual stream leading to initial coarse object representations and that the subsequent alpha-band orbitofrontal–fusiform synchrony reflected top-down facilitation of object recognition in the ventral visual stream. Also the operation of the action–perception–cognition loop is associated with large-scale alpha-band synchronization in cortical networks. A series of MEG studies show that alpha-band interactions in a cortico-cortical, -cerebellar, and -thalamic network are a predominant characteristic of brain dynamics underlying the planning and execution of movements (Gross et al., 2002; Pollok et al., 2005a,b) as well as the reading and language comprehension (Salmelin and Kujala, 2006; Kujala et al., 2007). These data thus strongly suggest that the phase dynamics of alpha-band oscillations play a central role in coordinating human brain activity into coherent action, cognition, and perception (Palva and Palva, 2007).
M/EEG Source Localization Reveals Large-Scale Networks of Inter-Areal Interactions in the Alpha-Band
Recent advances in combining cortical source reconstruction and data-driven interaction analysis approaches have paved the way for mapping the anatomical and topological structures of synchronized cortical networks from M/EEG data (Palva et al., 2010a,b). To explore the network dynamics underlying VWM encoding and retention, we mapped cortical inter-areal synchronization with single-trial M/EEG source localization by using a cortical parcellation covering the entire cortical surfaces with small patches and obtaining all pair-wise synchrony estimates between the patches. The advantage of these kinds of mapping approaches over those where the parcellation is incomplete is that the results reveal the most robust phenomena and are not biased by parcellation-selection criteria or by a priori hypotheses. We observed that inter-areal phase synchrony in alpha-, beta-, and gamma-bands was memory-load dependently enhanced during VWM maintenance. Load dependent alpha synchronization was observed in fronto-parietal, cingulate, and insular cortices concurrently with synchronization in the beta- and gamma-band networks (Palva et al., 2010a; Figure 4). These cortical regions have earlier been identified in VWM studies using fMRI (Prabhakaran et al., 2000; Rowe et al., 2000; Munk et al., 2002; Pessoa et al., 2002; Mohr et al., 2006) suggesting that the hubs of oscillatory communication networks could coincide with the hot spots revealed in task-related fMRI studies. These regions also overlapped with those showing oscillation amplitude modulations (cf. with Figure 1B). As the fronto-parietal regions are thought to underlie the attentional and central executive functions of VWM (Prabhakaran et al., 2000; Rowe et al., 2000; Sakai et al., 2002; Curtis and D’Esposito, 2003; Petrides, 2005), the alpha-, beta-, and gamma-band phase interactions appear to concurrently support these functions. Importantly, the hubs of the VWM retention period alpha-band network were more frontal than those of the beta- and gamma-band networks, suggesting that the alpha-band interactions underlie higher-level attentional functions than those in the beta- or gamma-bands. Network hubs were also observed in the insula, cingulate, and orbitofrontal structures that have been suggested to play a role in task-set maintenance (Dosenbach et al., 2008) or tonic alertness (Sadaghiani et al., 2010). These regions were synchronized both with visual and with fronto-parietal regions suggesting that VWM retention is associated with concurrent activity in and phase interactions between distinct attentional systems (Gilbert and Sigman, 2007). It is notable, however, that the synchrony in the cingulo-opercular system was not restricted to any of the frequency bands but seemed to equally involve alpha-, beta-, and gamma-band networks. Finally, we also observed that the memory-load dependent plateau in the strength of phase synchrony in alpha and beta bands predicted the individual VWM capacity (Figure 4), which indicates a direct representational or attentional role to these frequency bands.
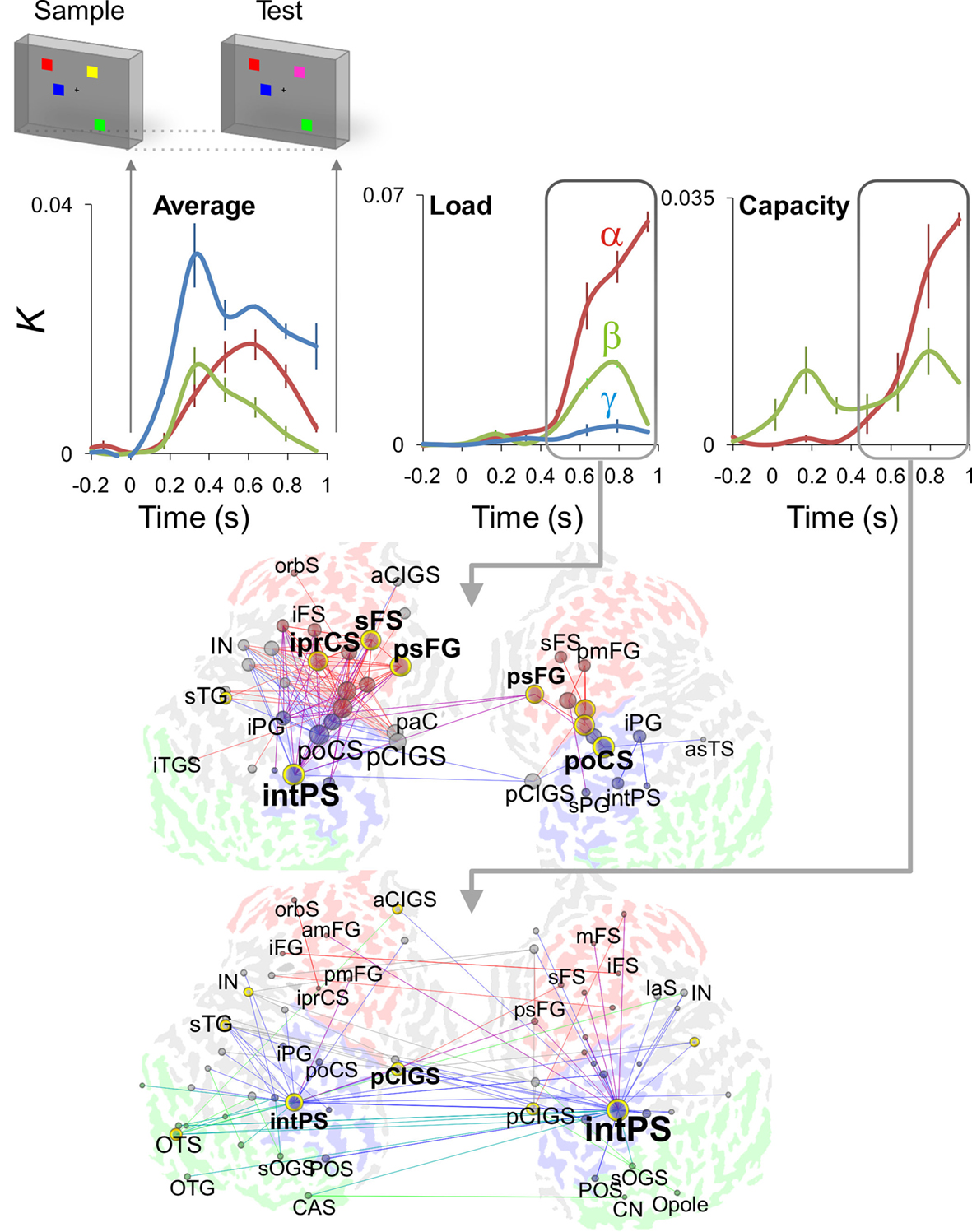
Figure 4. Single-trial source modeling of M/EEG data reveals dynamic phase synchronization during the VWM retention period of a parametric VWM task. Cortical inter-areal phase synchrony, as indexed by connection density K, was robust in alpha (red), beta (green), and gamma (blue) frequency bands throughout the working memory retention period (0.4–1 s) in data averaged across memory-loads from one to six objects (Average, upper panels). During the retention period, these inter-areal interactions were positively correlated with the memory-load (Load, upper panels) in fronto-parietal networks (middle panel, red, frontal; blue, parietal; green, occipital and occipito-temporal; the graph’s vertices are brain areas and the edges indicate memory-load dependent inter-areal synchronization in the alpha-band). A plateau in the memory-load dependence of a subset of interactions was positively correlated with the individual subjects’ behavioral working memory capacity (Capacity, upper panels) and networks where the intra-parietal sulcus (intPS) was the most prominent hub (lower panel, alpha-band synchronization correlated with memory capacity). Adapted from Palva et al. (2010).
These findings thus further support an attentional and/or executive role for alpha oscillations in high-level cortical regions. Taken together, these data suggest that concurrent and partially overlapping networks in alpha-, beta-, and gamma-bands among fronto-parietal, visual, and cingulo-opercular system support task performance. Moreover, co-localization of alpha-, beta-, and gamma-band networks also suggest that these networks play similar functional roles either in supporting active-processing or in inhibiting task-irrelevant neuronal activity. Importantly, a recent TMS–EEG study showed that neuronal activity in fronto-parietal regions may directly control the alpha amplitude in sensory cortex and demonstrated that the alpha-band amplitude is causally related to perception (Capotosto et al., 2009). We suggest that inter-areal alpha-band phase interactions in the fronto-parietal regions may underlie the top-down modulation of local oscillation amplitudes in sensory regions.
Possible loci for the interaction between frontal attentional/executive and sensory representational activities could be the regions that are high in the sensory processing hierarchy. One such interesting area is the posterior parietal cortex that is strongly and memory-load dependently phase synchronized with frontal regions and yet shows local alpha-band amplitude suppression below baseline levels during memory retention and a further negatively correlation of amplitude with memory-load (Figure 5). Also infero- and occipito-temporal regions show a similar behavior and may thus act as interfaces between frontal and sensory networks. Future studies exploiting directional interaction metrics are required to further understand these network phenomena.
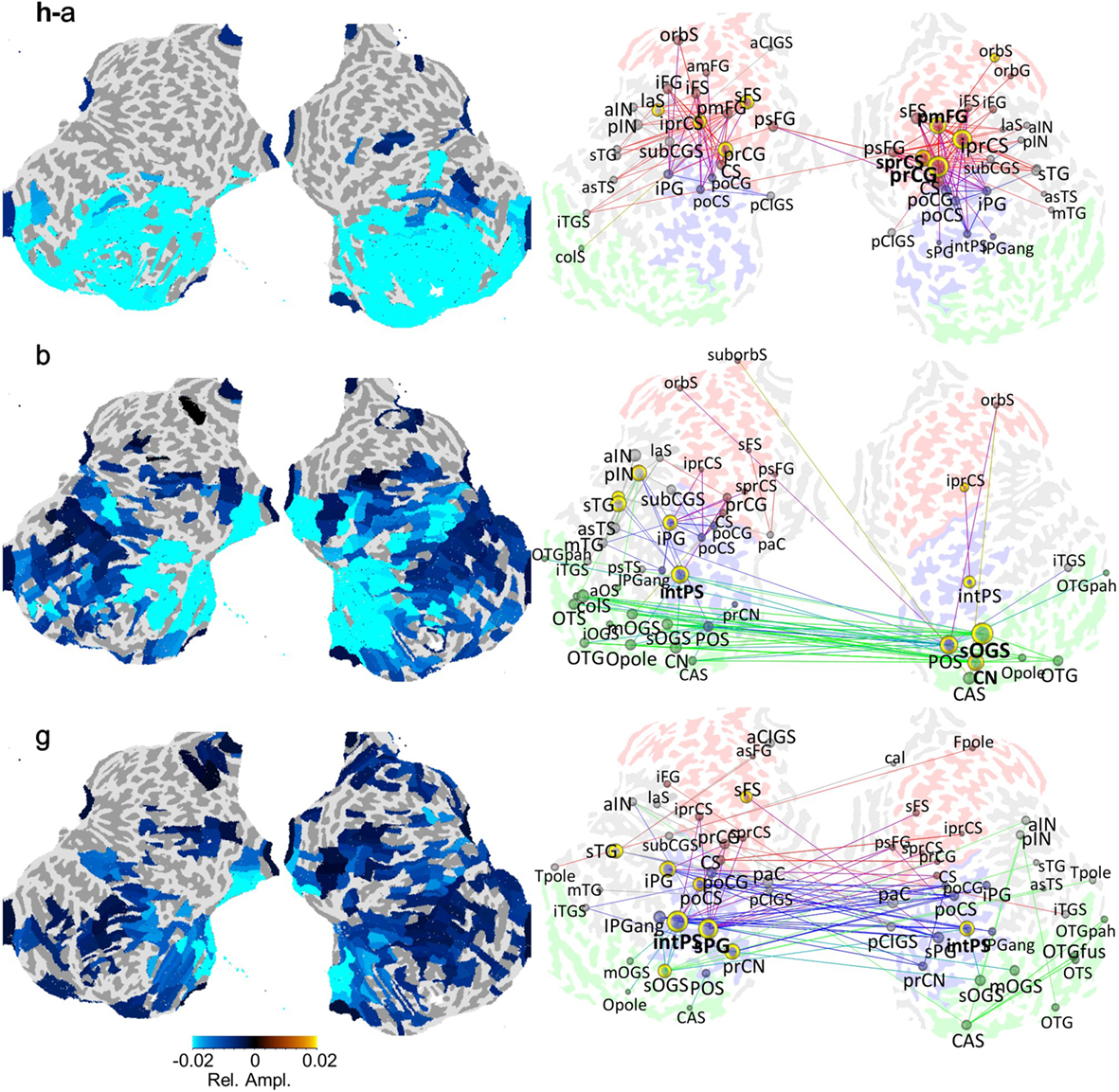
Figure 5. Local oscillation amplitudes may be suppressed concurrently with strengthened inter-areal synchronization in VWM task retention period (see Figures 1 and 4). The amplitudes (left panel) and networks of inter-areal phase synchronization (right panel) in the alpha-, beta-, and gamma-bands are displayed on flattened cortical surfaces. The amplitude color values (left panel) indicate the mean relative oscillation amplitude change from the baseline level and are shown for significantly modulated regions. The colored edges (right panel) indicate the temporally and spectrally most stable pairs of cortical areas between which the synchronization was strengthened above the baseline level within the alpha-, beta-, and gamma-bands during the VWM retention period. The edge colors indicate the cortical source/target region so that green denotes occipital and occipito-temporal regions, blue the parietal regions, and red the frontal regions. Adapted from Palva et al. (2010, 2011).
Cross-Frequency Interactions between Alpha and Other Frequency Bands
Alpha-band network oscillations often appear together with oscillations in theta-, beta-, and/or gamma-frequency bands. Although inter-areal synchronization in any given frequency band may coordinate the communication among brain regions, such within-frequency synchronization cannot support the integration of spectrally distributed processing or integration across temporal scales (Palva et al., 2005a). CF phase interactions may provide a mechanism for binding the spectrally distributed processing into coherent cognitive operations (Varela et al., 2001; Lakatos et al., 2005; Palva et al., 2005a; Palva and Palva, 2007; Schroeder and Lakatos, 2009). Specifically, phase–phase and phase–amplitude CF interactions are of interest in this context (see Box 1).
Box 1. Basics of probing phase and amplitude dynamics of cortical oscillations.
Electrophysiological recording techniques yield real-valued time series that can be used to estimate both in signal and in source space the local cortical phase and amplitude dynamics. In time domain, a wavelet transform or real-valued filtering together with the Hilbert transform yield complex-valued time series, x(t,f) = a(t,f)exp[iθ(t,f)] in a frequency-band f. The real part of x, Re(x), is the filtered waveform whereas the absolute value |x| = a gives the amplitude envelope and the argument of x, Arg(x) = θ yields the phase time series. Conversely, the amplitude and phase of each frequency band in a given time window can be estimated with the Fourier transform.
Local event-related dynamics. Vast majority of research on alpha oscillations has concentrated on event-related perturbations that are local in the sense that they are obtained for each EEG/MEG channel or source location separately. Event-related amplitude dynamics are obtained simply by averaging the amplitude time series across trials, <a(t,f)>, similarly to how conventional evoked responses are obtained by averaging real-valued time series across trials; <Re(x)>. Amplitude dynamics obtained in this manner are comparable with the event-related spectral perturbation (ERSP) method as well as with the event-related synchronization/desynchronization (ERS/ERD) approach where ERS denotes an increase and ERS a decrease in amplitude from a baseline level (Klimesch et al., 2007).
The phase-locking of ongoing oscillation to stimuli can be evaluated by averaging complex phase values across trials, |<exp[iθ(t,f)]>|, which yields a phase-locking value that ranges from 1 (perfect phase consistency across trials) to >0 (uniform phase distribution). This phase-locking value is comparable with inter-trial coherence (ITC) and captures both phase resetting of ongoing oscillations and additive evoked responses.
Inter-areal interactions. Local phase and amplitude time series can be used to yield estimates of inter-areal interactions as well. Perhaps the most studied type of these is within-frequency phase synchrony (A) which indicates a non-uniform distribution of phase differences between the two time series and quantifies the presence and strength of a phase correlation between signals x and y in a frequency-band f. The complex phase differences between x and y are given by φxy = xy*/|x||y| = exp(iθx − θy), where * denotes the complex conjugate. The uniformity of the phase difference distribution can subsequently be quantified with a number of metrics such as the phase-locking value that would be given by |<φxy>|. The frequency-domain equivalent to phase synchrony is termed phase coherence. Phase synchrony and phase coherence differ from the classical frequency-domain coherence in that coherence is biased by signal amplitudes.
Within-frequency phase synchrony is a special case (1:1) of general n:m-phase synchrony (B), where integers n and m define the ratio of frequencies mf1 = nf2 and the phase difference φxy,n:m = x(f1)my(f2) n*/|x||y| = exp[i(mθx − nθy)] (Palva et al., 2005a). Panel B illustrates a case of 1:4 synchronization between signals x and y in frequency-bands 4f1 = f2, respectively. Note that after the multiplication of slow oscillation’s phase, Arg(xf1), by m = 4, the presence of cross-frequency phase synchrony is salient as 1:1 coupling in the phase time series.
In addition to phase–phase couplings, such as n:m-phase synchrony, also phase–amplitude coupling (C) is prominent among cortical oscillations. Also known as “nested oscillations”, phase–amplitude coupling indicates that there is a correlation between the amplitude of the fast oscillation, |yf2|, and the phase, Arg(xf1), of the slower oscillation. Among other methods, phase–amplitude coupling can be estimated so that the amplitude envelope of the fast oscillation in band f2 is filtered, Ff1(.), with the filter used to obtain the lower band f1 after which the problem of detecting nested oscillations is reduced to a problem of detecting 1:1 phase synchronization (Vanhatalo et al., 2004).
Phase–phase interactions are typically quantified as n:m-phase synchrony (Tass et al., 1998), where n and m are small integers defining the frequency ratio of the coupled low and high frequency oscillations. In the coupling of neuronal oscillations, n:m coupling reflects an interaction where the phase of the slow oscillation is coupled with the phase of the faster oscillation, which has two consequences: first, the slow oscillation must operate roughly at a temporal precision of the faster oscillation. Second, because the firing of the slow oscillation takes place in a specific phase of the fast cycle, n:m coupling is compatible with phase-based coding and communication schemes (Palva et al., 2005a) similarly to the classical 1:1 within-frequency phase synchrony (Fries, 2005).
We addressed the presence of CF interactions in ongoing brain activity in an MEG study using a continuous mental calculation task with parametric working memory-load and no sensory stimuli (Palva et al., 2005a). 1:1 phase synchronization was observed in alpha-, beta-, and gamma-frequency bands in this task. The alpha-band networks were memory-load dependently 1:2-phase synchronized with the beta-band networks and 1:3–1:4 synchronized with gamma-band activity suggesting that dynamic phase interactions among alpha and faster oscillations are indeed used in cognitive operations (see Figure 3). CF phase synchrony has also been observed in EEG recordings among alpha and beta oscillations during rest (Nikulin and Brismar, 2006) and between theta and alpha oscillations during working memory task performance (Schack et al., 2005). Importantly, TMS-induced facilitation of working memory performance has been associated with enhanced 1:6 alpha–gamma phase synchronization that was concurrent with a decrease in alpha-band amplitude (Hamidi et al., 2009) indicating a direct functional relevance for CF phase–phase interactions in cognitive operations.
Phase–amplitude interactions, also known as nested oscillations, are another important type of CF interactions. In nested oscillations, the phase of the slow oscillation is correlated with the amplitude of the faster oscillation. Notably, in nested oscillations, the phase of the fast oscillation is uncorrelated with the phase of the slow oscillation and the phase–amplitude correlation thus reflects, for instance, a slow excitability fluctuation in the neuronal circuitry underlying the fast oscillation (Schroeder and Lakatos, 2009). The amplitude of alpha oscillations has been observed to be nested in infra-slow oscillations in the 0.01–0.1-Hz band both during sleep (Vanhatalo et al., 2004), and during the execution of a somatosensory threshold detection task (Figure 6; Monto et al., 2008). These correlations are behaviorally significant and likely to reflect slow resting-state network dynamics (Palva and Palva, 2011). In line with concept of hierarchically nested CF phase interactions (Lakatos et al., 2005; Palva et al., 2005a; Monto et al., 2008; He et al., 2010), the phase of alpha oscillations is also correlated with the amplitudes of faster oscillations. By using a phase-locking value based method for estimating phase–amplitude interactions (Vanhatalo et al., 2004), Voytek et al. (2010) show that during active performance of visual tasks, the phase of visual cortex alpha oscillations robustly modulates the amplitude gamma-band activity in those locations. In another study, the phase of alpha oscillations was correlated with gamma-band amplitude in human nucleus accumbens during reward processing (Cohen et al., 2009). These data thus both corroborate the overall role of alpha phase in modulating local neuronal excitability and, as predicted by the active-processing hypothesis, indicate that the phase dynamics of alpha oscillations are functionally significant specifically in the task-relevant cortical networks.
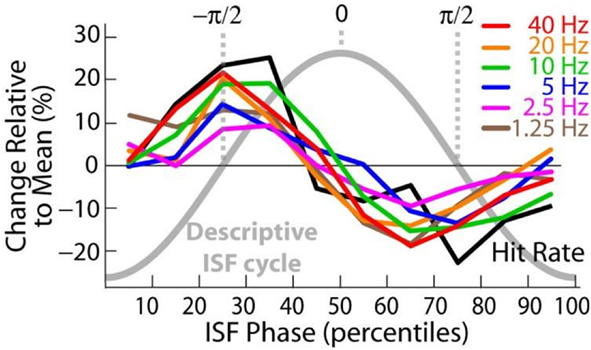
Figure 6. The phase of infra-slow (0.01–0.1 Hz) fluctuations is correlated similarly with both the amplitudes of fast (1–40 Hz) oscillations and the detection of threshold-level somatosensory stimuli. This suggests that the phase of infra-slow oscillations reflect global excitability modulations. Adapted from Monto et al. (2008).
Graph Theoretical View into the Alpha Networks
In addition to characterizing inter-areal synchronization according to its anatomical localization and of their hypothesized functional roles, anatomical and functional neuronal networks can be characterized with graph theoretical tools as they share properties with network representations of many other complex systems (Bullmore and Sporns, 2009). In graph representation of brain networks, brain areas are the graph’s vertices and the inter-areal interactions are the edges. Several graph metrics can be used to describe the topological properties of the network. Perhaps most importantly, anatomical networks share properties of small-world networks that can be seen as lattice structures (networks with only local connections) with rare long-range connections and that can be described by the clustering and average path length of the network. Anatomical networks have high clustering and short average path lengths (Sporns et al., 2005; Iturria-Medina et al., 2008; Gong et al., 2009; He et al., 2009) that are indicative of small-world structure in which dense local connectivity lead to greater local clustering than in random networks. Yet, because of the long-range “short-cut” connections, their characteristic path length, i.e., the mean of shortest paths from each node to another node, are close to those in random networks (Watts and Strogatz, 1998). Small-world networks are associated with high local and global efficiency in information transmission as well as facilitated parallel processing within hierarchically organized modules (Bassett and Bullmore, 2006; Bullmore and Sporns, 2009).
Several studies have addressed the graph properties of the synchronized networks at the EEG-sensor-level. The graph properties in the alpha-band are modulated by age (Smit et al., 2008, 2010; Micheloyannis et al., 2009; Boersma et al., 2010) and by sex (Smit et al., 2008; Boersma et al., 2010). These changes might reflect changes in the brain anatomical connections (Uhlhaas et al., 2009) or changes in the processing strategies. These studies hence show that topological properties of synchronized networks change as a function of development differentially across distinct frequency bands. However, although the topological properties change during development in the alpha-band they have been shown to remain constant across measurement sessions (Deuker et al., 2009) and to be heritable (Smit et al., 2008, 2010). In the time scales of cognitive task performance, recent M/EEG and MEG working memory data show that alpha- and beta-band networks are distinct from those in delta-, theta-, and gamma-bands both in terms of topological properties and between healthy and schizophrenic subject groups (Bassett et al., 2009; Palva et al., 2010b). During the VWM retention period, networks defined by alpha-band phase synchrony were compact around densely connected core structures being more clustered and small-world like (Figure 7) and hence locally more efficient and globally less efficient than the networks in other frequency bands (Palva et al., 2010b). On the other hand, gamma-band networks were locally less clustered and globally more efficient. These graph theoretical “fingerprints” suggest that oscillations in distinct frequency bands have different functional roles in supporting local and global communication in neuronal networks during VWM maintenance. Overall, graph theoretical analyses of human brain networks defined by synchronized oscillations converge on suggesting that dynamic alpha-band networks exhibit a small-world structure. However, further studies with direct cortical recordings and/or M/EEG source modeling are required to corroborate this observation and to address the physiological and behavioral significance of the network topologies.
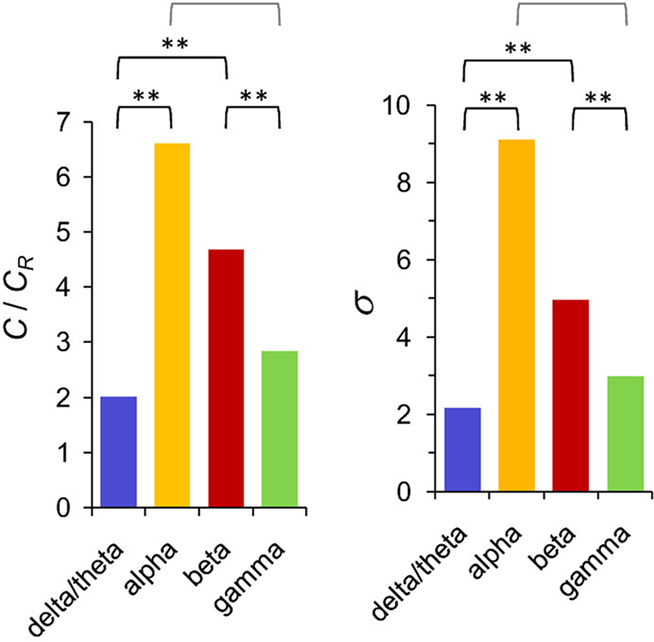
Figure 7. The networks of inter-areal synchronization in the alpha and beta bands are more clustered and small-world like than those in the delta- and gamma-frequency bands. C/CR indicates the relative clustering coefficient of the networks in these four frequency bands, whereas σ denotes their small-worldness. Modified from Palva et al. (2010b).
Conclusion and Challenges for Future Research
Alpha phase is correlated with cyclic modulation of neuronal excitability that biases neuronal and behavioral responses to sensory stimuli. Converging evidence points to an attentional role for alpha rhythmicity where the alpha phase plays a critical mechanistic role in influencing local neuronal timing and inter-areal phase relations (Klimesch et al., 2007; Palva and Palva, 2007). Nevertheless, the evidence is inconclusive on whether alpha oscillations support the inhibition of task-irrelevant processing (Klimesch et al., 2007; Jensen and Mazaheri, 2010), the executive control of behavioral responses (Klimesch et al., 2007), or active task-relevant processing (von Stein et al., 2000; Palva and Palva, 2007; Mo et al., 2011). We suggest that alpha may contribute to each of these three concurrently and reiterate our prior argument (Palva and Palva, 2007) that inter-areal phase interactions are the key characteristic that may dissociate or reconcile the different views on the functional significance of alpha oscillations.
New data and methodological approaches are thus needed to identify the possibly multiple functional roles of alpha-band synchronization in cortical networks. We outline here some essential questions for future research.
• Concurrent memory-load dependent amplitude increase and network phase synchrony in alpha-, beta-, and gamma-bands is observed in fronto-parietal structures during VWM maintenance. These structures include dorso- and ventro-lateral prefrontal and posterior parietal cortices, but also the sensorimotor regions and the cingulo-opercular system. Prior studies indicate a critical role for prefrontal and posterior parietal regions in WWM, which supports an active role in VWM for alpha, beta, and gamma oscillations in these regions. However, the role of sensorimotor and cingulo-opercular activity in VWM maintenance in unclear. Does the load dependent strengthening of alpha, together with beta and gamma, oscillations therein reflect a progressive disengagement of these cortical regions, which would be the interpretation predicted by the inhibition hypothesis? Alternatively, these regions could play a hitherto unrecognized role in VWM maintenance.
• The concern raised above generalizes to a question of how to define which cortical regions are task-relevant and which are task-irrelevant. The notion of task relevance is a critical component in the inhibition hypothesis that cannot be tested without having prior “gold standard” knowledge about the functional roles of specific cortical regions in the task context under study. fMRI might not yield such gold standard data because, for instance, synchronization phenomena can be critical for information processing and yet be associated with only minor metabolic demands.
• If fronto-parietal networks synchronized in alpha-, beta-, and gamma-bands directly support attentional and executive functions, is their top-down effect on sensory processing suppressive or facilitating? Does direct inter-areal phase synchrony mediate these top-down interactions? Do different levels of sensory hierarchy operate with distinct mechanisms?
• If beta and gamma oscillations are found to be co-localized with alpha and to be phenomenologically similar in terms of phase and amplitude dynamics, does the inhibition hypothesis predict an inhibitory role for these bands as well?
• From the perspective of the active-processing hypothesis, long-range phase synchronization in the alpha-band carries the functional role attributable to synchronization in other frequency bands, which is regulation of local and inter-areal communication. What is the significance of inter-areal synchronization in the context of the inhibition hypothesis that is largely formulated in terms of amplitude?
• Does alpha synchronization arise in attentional/executive regions as an excitatory process that imposes suppressive top-down inputs to the task-irrelevant regions? What are the mechanisms that allow for a top-down control of oscillation amplitude?
• What could be the neuronal mechanisms of cortical disengagement at the time of large-amplitude alpha oscillations in sensory cortices? Oscillations in all frequency bands involve inhibitory GABAergic currents and reflect periodic excitability fluctuations; why is alpha special?
Conflict of Interest Statement
The authors declare that the research was conducted in the absence of any commercial or financial relationships that could be construed as a potential conflict of interest.
References
Andersen, E. A. S. (1968). Physiological Basis of the Alpha Rhythm New York, NY: Appleton-Century-Crofts.
Aron, A. R., Robbins, T. W., and Poldrack, R. A. (2004). Inhibition and the right inferior frontal cortex. Trends Cogn. Sci. (Regul. Ed.) 8, 170–177.
Bar, M., Kassam, K. S., Ghuman, A. S., Boshyan, J., Schmid, A. M., Dale, A. M., Hamalainen, M. S., Marinkovic, K., Schacter, D. L., Rosen, B. R., and Halgren, E. (2006). Top-down facilitation of visual recognition. Proc. Natl. Acad. Sci. U.S.A. 103, 449–454.
Barry, R. J., Rushby, J. A., Johnstone, S. J., Clarke, A. R., Croft, R. J., and Lawrence, C. A. (2004). Event-related potentials in the auditory oddball as a function of EEG alpha phase at stimulus onset. Clin. Neurophysiol. 115, 2593–2601.
Bassett, D. S., Bullmore, E. T., Meyer-Lindenberg, A., Apud, J. A., Weinberger, D. R., and Coppola, R. (2009). Cognitive fitness of cost-efficient brain functional networks. Proc. Natl. Acad. Sci. U.S.A. 106, 11742–11747.
Boersma, M., Smit, D. J., de Bie, H. M., Van Baal, G. C., Boomsma, D. I., de Geus, E. J., Delemarre-van de Waal, H. A., and Stam, C. J. (2010). Network analysis of resting state EEG in the developing young brain: structure comes with maturation. Hum. Brain Mapp. 32, 413–425.
Bollimunta, A., Chen, Y., Schroeder, C. E., and Ding, M. (2008). Neuronal mechanisms of cortical alpha oscillations in awake-behaving macaques. J. Neurosci. 28, 9976–9988.
Bollimunta, A., Mo, J., Schroeder, C. E., and Ding, M. (2011). Neuronal mechanisms and attentional modulation of corticothalamic alpha oscillations. J. Neurosci. 31, 4935–4943.
Brandt, M. E., Jansen, B. H., and Carbonari, J. P. (1991). Pre-stimulus spectral EEG patterns and the visual evoked response. Electroencephalogr. Clin. Neurophysiol. 80, 16–20.
Bullmore, E., and Sporns, O. (2009). Complex brain networks: graph theoretical analysis of structural and functional systems. Nat. Rev. Neurosci. 10, 186–198.
Busch, N. A., Dubois, J., and VanRullen, R. (2009). The phase of ongoing EEG oscillations predicts visual perception. J. Neurosci. 29, 7869–7876.
Busch, N. A., and Herrmann, C. S. (2003). Object-load and feature-load modulate EEG in a short-term memory task. Neuroreport 14, 1721–1724.
Busch, N. A., and VanRullen, R. (2010). Spontaneous EEG oscillations reveal periodic sampling of visual attention. Proc. Natl. Acad. Sci. U.S.A. 107, 16048–16053.
Buschkuehl, M., Jaeggi, S. M., Hutchison, S., Perrig-Chiello, P., Dapp, C., Muller, M., Breil, F., Hoppeler, H., and Perrig, W. J. (2008). Impact of working memory training on memory performance in old-old adults. Psychol. Aging 23, 743–753.
Callaway, E. III, and Yeager, C. L. (1960). Relationship between reaction time and electroencephalographic alpha phase. Science 132, 1765–1766.
Capotosto, P., Babiloni, C., Romani, G. L., and Corbetta, M. (2009). Frontoparietal cortex controls spatial attention through modulation of anticipatory alpha rhythms. J. Neurosci. 29, 5863–5872.
Cardin, J. A., Carlen, M., Meletis, K., Knoblich, U., Zhang, F., Deisseroth, K., Tsai, L. H., and Moore, C. I. (2009). Driving fast-spiking cells induces gamma rhythm and controls sensory responses. Nature 459, 663–667.
Cohen, M. X., Axmacher, N., Lenartz, D., Elger, C. E., Sturm, V., and Schlaepfer, T. E. (2009). Good vibrations: cross-frequency coupling in the human nucleus accumbens during reward processing. J. Cogn. Neurosci. 21, 875–889.
Congdon, E., Mumford, J. A., Cohen, J. R., Galvan, A., Aron, A. R., Xue, G., Miller, E., and Poldrack, R. A. (2010). Engagement of large-scale networks is related to individual differences in inhibitory control. Neuroimage 53, 653–663.
Curtis, C. E., and D’Esposito, M. (2003). Persistent activity in the prefrontal cortex during working memory. Trends Cogn. Sci. (Regul. Ed.) 7, 415–423.
Deuker, L., Bullmore, E. T., Smith, M., Christensen, S., Nathan, P. J., Rockstroh, B., and Bassett, D. S. (2009). Reproducibility of graph metrics of human brain functional networks. Neuroimage 47, 1460–1468.
Dockstader, C., Cheyne, D., and Tannock, R. (2010). Cortical dynamics of selective attention to somatosensory events. Neuroimage 49, 1777–1785.
Doesburg, S. M., Green, J. J., McDonald, J. J., and Ward, L. M. (2009). From local inhibition to long-range integration: a functional dissociation of alpha-band synchronization across cortical scales in visuospatial attention. Brain Res. 1303, 97–110.
Dosenbach, N. U., Fair, D. A., Cohen, A. L., Schlaggar, B. L., and Petersen, S. E. (2008). A dual-networks architecture of top-down control. Trends Cogn. Sci. (Regul. Ed.) 12, 99–105.
Drewes, J., and Vanrullen, R. (2011). This is the rhythm of your eyes: the phase of ongoing electroencephalogram oscillations modulates saccadic reaction time. J. Neurosci. 31, 4698–4708.
Freunberger, R., Fellinger, R., Sauseng, P., Gruber, W., and Klimesch, W. (2009). Dissociation between phase-locked and nonphase-locked alpha oscillations in a working memory task. Hum. Brain Mapp. 30, 3417–3425.
Freunberger, R., Klimesch, W., Griesmayr, B., Sauseng, P., and Gruber, W. (2008). Alpha phase coupling reflects object recognition. Neuroimage 42, 928–935.
Fries, P. (2005). A mechanism for cognitive dynamics: neuronal communication through neuronal coherence. Trends Cogn. Sci. (Regul. Ed.) 9, 474–480.
Fries, P. (2009). Neuronal gamma-band synchronization as a fundamental process in cortical computation. Annu. Rev. Neurosci. 32, 209–240.
Gilbert, C. D., and Sigman, M. (2007). Brain states: top-down influences in sensory processing. Neuron 54, 677–696.
Gong, G., He, Y., Concha, L., Lebel, C., Gross, D. W., Evans, A. C., and Beaulieu, C. (2009). Mapping anatomical connectivity patterns of human cerebral cortex using in vivo diffusion tensor imaging tractography. Cereb. Cortex 19, 524–536.
Gould, I. C., Rushworth, M. F., and Nobre, A. C. (2011). Indexing the graded allocation of visuospatial attention using anticipatory alpha oscillations. J. Neurophysiol. 105, 1318–1326.
Green, C. S., and Bavelier, D. (2003). Action video game modifies visual selective attention. Nature 423, 534–537.
Grimault, S., Robitaille, N., Grova, C., Lina, J. M., Dubarry, A. S., and Jolicoeur, P. (2009). Oscillatory activity in parietal and dorsolateral prefrontal cortex during retention in visual short-term memory: additive effects of spatial attention and memory load. Hum. Brain Mapp. 30, 3378–3392.
Gross, J., Timmermann, L., Kujala, J., Dirks, M., Schmitz, F., Salmelin, R., and Schnitzler, A. (2002). The neural basis of intermittent motor control in humans. Proc. Natl. Acad. Sci. U.S.A. 99, 2299–2302.
Haegens, S., Handel, B. F., and Jensen, O. (2011). Top-down controlled alpha band activity in somatosensory areas determines behavioral performance in a discrimination task. J. Neurosci. 31, 5197–5204.
Haenschel, C., Bittner, R. A., Waltz, J., Haertling, F., Wibral, M., Singer, W., Linden, D. E., and Rodriguez, E. (2009). Cortical oscillatory activity is critical for working memory as revealed by deficits in early-onset schizophrenia. J. Neurosci. 29, 9481–9489.
Haenschel, C., Linden, D. E., Bittner, R. A., Singer, W., and Hanslmayr, S. (2010). Alpha phase locking predicts residual working memory performance in schizophrenia. Biol. Psychiatry 68, 595–598.
Hamidi, M., Slagter, H. A., Tononi, G., and Postle, B. R. (2009). Repetitive transcranial magnetic stimulation affects behavior by biasing endogenous cortical oscillations. Front. Integr. Neurosci. 3:14.
Hamm, J. P., Dyckman, K. A., Ethridge, L. E., McDowell, J. E., and Clementz, B. A. (2010). Preparatory activations across a distributed cortical network determine production of express saccades in humans. J. Neurosci. 30, 7350–7357.
He, B. J., Zempel, J. M., Snyder, A. Z., and Raichle, M. E. (2010). The temporal structures and functional significance of scale-free brain activity. Neuron 66, 353–369.
He, Y., Wang, J., Wang, L., Chen, Z. J., Yan, C., Yang, H., Tang, H., Zhu, C., Gong, Q., Zang, Y., and Evans, A. C. (2009). Uncovering intrinsic modular organization of spontaneous brain activity in humans PLoS ONE 4, e5226.
Hughes, S. W., and Crunelli, V. (2007). Just a phase they’re going through: the complex interaction of intrinsic high-threshold bursting and gap junctions in the generation of thalamic alpha and theta rhythms. Int. J. Psychophysiol. 64, 3–17.
Hughes, S. W., Lorincz, M., Cope, D. W., Blethyn, K. L., Kekesi, K. A., Parri, H. R., Juhasz, G., and Crunelli, V. (2004). Synchronized oscillations at alpha and theta frequencies in the lateral geniculate nucleus. Neuron 42, 253–268.
Hummel, F., and Gerloff, C. (2005). Larger interregional synchrony is associated with greater behavioral success in a complex sensory integration task in humans. Cereb. Cortex 15, 670–678.
Iturria-Medina, Y., Sotero, R. C., Canales-Rodriguez, E. J., Aleman-Gomez, Y., and Melie-Garcia, L. (2008). Studying the human brain anatomical network via diffusion-weighted MRI and graph theory. Neuroimage 40, 1064–1076.
Jaeggi, S. M., Buschkuehl, M., Jonides, J., and Perrig, W. J. (2008). Improving fluid intelligence with training on working memory. Proc. Natl. Acad. Sci. U.S.A. 105, 6829–6833.
Jensen, O., Gelfand, J., Kounios, J., and Lisman, J. E. (2002). Oscillations in the alpha band (9-12 hz) increase with memory load during retention in a short-term memory task. Cereb. Cortex 12, 877–882.
Jensen, O., and Mazaheri, A. (2010). Shaping functional architecture by oscillatory alpha activity: gating by inhibition. Front. Hum. Neurosci. 4:186.
Jokisch, D., and Jensen, O. (2007). Modulation of gamma and alpha activity during a working memory task engaging the dorsal or ventral stream. J. Neurosci. 27, 3244–3251.
Klimesch, W., Sauseng, P., and Hanslmayr, S. (2007). EEG alpha oscillations: the inhibition-timing hypothesis. Brain Res. Rev. 53, 63–88.
Kopell, N., Ermentrout, G. B., Whittington, M. A., and Traub, R. D. (2000). Gamma rhythms and beta rhythms have different synchronization properties. Proc. Natl. Acad. Sci. U.S.A. 97, 1867–1872.
Kujala, J., Pammer, K., Cornelissen, P., Roebroeck, A., Formisano, E., and Salmelin, R. (2007). Phase coupling in a cerebro-cerebellar network at 8-13 hz during reading. Cereb. Cortex 17, 1476–1485.
Lakatos, P., Karmos, G., Mehta, A. D., Ulbert, I., and Schroeder, C. E. (2008). Entrainment of neuronal oscillations as a mechanism of attentional selection. Science 320, 110–113.
Lakatos, P., Shah, A. S., Knuth, K. H., Ulbert, I., Karmos, G., and Schroeder, C. E. (2005). An oscillatory hierarchy controlling neuronal excitability and stimulus processing in the auditory cortex. J. Neurophysiol. 94, 1904–1911.
Laufs, H., Krakow, K., Sterzer, P., Eger, E., Beyerle, A., Salek-Haddadi, A., and Kleinschmidt, A. (2003). Electroencephalographic signatures of attentional and cognitive default modes in spontaneous brain activity fluctuations at rest. Proc. Natl. Acad. Sci. U.S.A. 100, 11053–11058.
Leiberg, S., Lutzenberger, W., and Kaiser, J. (2006). Effects of memory load on cortical oscillatory activity during auditory pattern working memory. Brain Res. 1120, 131–140.
Lopes da Silva, F. H., Hoeks, A., Smits, H., and Zetterberg, L. H. (1974). Model of brain rhythmic activity. The alpha-rhythm of the thalamus. Kybernetik 15, 27–37.
Lopes da Silva, F. H., van Lierop, T. H., Schrijer, C. F., and van Leeuwen, W. S. (1973). Organization of cortical and thalamic alpha rhythms. 35, 627–639.
Lopes da Silva, F. H., Vos, J. E., Mooibroek, J., and Van Rotterdam, A. (1980). Relative contributions of intracortical and thalamo-cortical processes in the generation of alpha rhythms, revealed by partial coherence analysis. Electroencephalogr. Clin. Neurophysiol. 50, 449–456.
Lorincz, M. L., Kekesi, K. A., Juhasz, G., Crunelli, V., and Hughes, S. W. (2009). Temporal framing of thalamic relay-mode firing by phasic inhibition during the alpha rhythm. Neuron 63, 683–696.
Luck, S. J., and Vogel, E. K. (1997). The capacity of visual working memory for features and conjunctions. Nature 390, 279–281.
Maclin, E. L., Mathewson, K. E., Low, K. A., Boot, W. R., Kramer, A. F., Fabiani, M., and Gratton, G. (2011). Learning to multitask: effects of video game practice on electrophysiological indices of attention and resource allocation. Psychophysiology 48, 1173–1183.
Maris, E., van Vugt, M., and Kahana, M. (2011). Spatially distributed patterns of oscillatory coupling between high-frequency amplitudes and low-frequency phases in human iEEG. Neuroimage 54, 836–850.
Marois, R., Yi, D. J., and Chun, M. M. (2004). The neural fate of consciously perceived and missed events in the attentional blink. Neuron 41, 465–472.
Mathewson, K. E., Fabiani, M., Gratton, G., Beck, D. M., and Lleras, A. (2010). Rescuing stimuli from invisibility: inducing a momentary release from visual masking with pre-target entrainment. Cognition 115, 186–191.
Mathewson, K. E., Gratton, G., Fabiani, M., Beck, D. M., and Ro, T. (2009). To see or not to see: prestimulus alpha phase predicts visual awareness. J. Neurosci. 29, 2725–2732.
Medendorp, W. P., Kramer, G. F., Jensen, O., Oostenveld, R., Schoffelen, J. M., and Fries, P. (2007). Oscillatory activity in human parietal and occipital cortex shows hemispheric lateralization and memory effects in a delayed double-step saccade task. Cereb. Cortex 17, 2364–2374.
Micheloyannis, S., Vourkas, M., Tsirka, V., Karakonstantaki, E., Kanatsouli, K., and Stam, C. J. (2009). The influence of ageing on complex brain networks: a graph theoretical analysis. Hum. Brain Mapp. 30, 200–208.
Mo, J., Schroeder, C. E., and Ding, M. (2011). Attentional modulation of alpha oscillations in macaque inferotemporal cortex. J. Neurosci. 31, 878–882.
Mohr, H. M., Goebel, R., and Linden, D. E. (2006). Content- and task-specific dissociations of frontal activity during maintenance and manipulation in visual working memory. J. Neurosci. 26, 4465–4471.
Monto, S., Palva, S., Voipio, J., and Palva, J. M. (2008). Very slow EEG fluctuations predict the dynamics of stimulus detection and oscillation amplitudes in humans. J. Neurosci. 28, 8268–8272.
Munk, M. H., Linden, D. E., Muckli, L., Lanfermann, H., Zanella, F. E., Singer, W., and Goebel, R. (2002). Distributed cortical systems in visual short-term memory revealed by event-related functional magnetic resonance imaging. Cereb. Cortex 12, 866–876.
Nicolelis, M. A., and Fanselow, E. E. (2002). Thalamocortical [correction of thalamcortical] optimization of tactile processing according to behavioral state. Nat. Neurosci. 5, 517–523.
Nikulin, V. V., and Brismar, T. (2006). Phase synchronization between alpha and beta oscillations in the human electroencephalogram. Neuroscience 137, 647–657.
Nunn, C. M., and Osselton, J. W. (1974). The influence of the EEG alpha rhythm on the perception of visual stimuli. Psychophysiology 11, 294–303.
Osipova, D., Takashima, A., Oostenveld, R., Fernandez, G., Maris, E., and Jensen, O. (2006). Theta and gamma oscillations predict encoding and retrieval of declarative memory. J. Neurosci. 26, 7523–7531.
Palva, J. M., Monto, S., Kulashekhar, S., and Palva, S. (2010a). Neuronal synchrony reveals working memory networks and predicts individual memory capacity. Proc. Natl. Acad. Sci. U.S.A. 107, 7580–7585.
Palva, S., Monto, S., and Palva, J. M. (2010b). Graph properties of synchronized cortical networks during visual working memory maintenance. Neuroimage 49, 3257–3268.
Palva, J. M., and Palva, S. (2011). Roles of multi-scale brain activity fluctuations in shaping the variability and dynamics of psychophysical performance. Prog. Brain Res. 193, 335–350.
Palva, J. M., Palva, S., and Kaila, K. (2005a). Phase synchrony among neuronal oscillations in the human cortex. J. Neurosci. 25, 3962–3972.
Palva, S., Linkenkaer-Hansen, K., Naatanen, R., and Palva, J. M. (2005b). Early neural correlates of conscious somatosensory perception. J. Neurosci. 25, 5248–5258.
Palva, S., Kulashekhar, S., Hamalainen, M., and Palva, J. M. (2011). Localization of cortical phase and amplitude dynamics during visual working memory encoding and retention. J. Neurosci. 31, 5013–5025.
Palva, S., and Palva, J. M. (2007). New vistas for alpha-frequency band oscillations. Trends Neurosci. 30, 150–158.
Pastor, M. A., Artieda, J., Arbizu, J., Marti-Climent, J. M., Penuelas, I., and Masdeu, J. C. (2002). Activation of human cerebral and cerebellar cortex by auditory stimulation at 40 hz. J. Neurosci. 22, 10501–10506.
Pessoa, L., Gutierrez, E., Bandettini, P., and Ungerleider, L. (2002). Neural correlates of visual working memory: fMRI amplitude predicts task performance. Neuron 35, 975–987.
Petrides, M. (2005). Lateral prefrontal cortex: architectonic and functional organization. Philos. Trans. R. Soc. Lond. B Biol. Sci. 360, 781–795.
Pfurtscheller, G. (2003). Induced oscillations in the alpha band: functional meaning. Epilepsia 44(Suppl. 12), 2–8.
Pollok, B., Sudmeyer, M., Gross, J., and Schnitzler, A. (2005a). The oscillatory network of simple repetitive bimanual movements. Brain Res. Cogn. Brain Res. 25, 300–311.
Pollok, B., Gross, J., Muller, K., Aschersleben, G., and Schnitzler, A. (2005b). The cerebral oscillatory network associated with auditorily paced finger movements. Neuroimage 24, 646–655.
Prabhakaran, V., Narayanan, K., Zhao, Z., and Gabrieli, J. D. (2000). Integration of diverse information in working memory within the frontal lobe. Nat. Neurosci. 3, 85–90.
Ray, W. J., and Cole, H. W. (1985). EEG alpha activity reflects attentional demands, and beta activity reflects emotional and cognitive processes. Science 228, 750–752.
Romei, V., Brodbeck, V., Michel, C., Amedi, A., Pascual-Leone, A., and Thut, G. (2008). Spontaneous fluctuations in posterior alpha-band EEG activity reflect variability in excitability of human visual areas. Cereb. Cortex 18, 2010–2018.
Romei, V., Driver, J., Schyns, P. G., and Thut, G. (2011). Rhythmic TMS over parietal cortex links distinct brain frequencies to global versus local visual processing. Curr. Biol. 21, 334–337.
Romei, V., Gross, J., and Thut, G. (2010). On the role of prestimulus alpha rhythms over occipito-parietal areas in visual input regulation: correlation or causation? J. Neurosci. 30, 8692–8697.
Rowe, J. B., Toni, I., Josephs, O., Frackowiak, R. S., and Passingham, R. E. (2000). The prefrontal cortex: response selection or maintenance within working memory? Science 288, 1656–1660.
Rueda, M. R., Rothbart, M. K., McCandliss, B. D., Saccomanno, L., and Posner, M. I. (2005). Training, maturation, and genetic influences on the development of executive attention. Proc. Natl. Acad. Sci. U.S.A. 102, 14931–14936.
Sadaghiani, S., Scheeringa, R., Lehongre, K., Morillon, B., Giraud, A. L., and Kleinschmidt, A. (2010). Intrinsic connectivity networks, alpha oscillations, and tonic alertness: a simultaneous electroencephalography/functional magnetic resonance imaging study. J. Neurosci. 30, 10243–10250.
Sakai, K., Rowe, J. B., and Passingham, R. E. (2002). Active maintenance in prefrontal area 46 creates distractor-resistant memory. Nat. Neurosci. 5, 479–484.
Salmelin, R., and Kujala, J. (2006). Neural representation of language: activation versus long-range connectivity. Trends Cogn. Sci. (Regul. Ed.) 10, 519–525.
Sauseng, P., Klimesch, W., Heise, K. F., Gruber, W. R., Holz, E., Karim, A. A., Glennon, M., Gerloff, C., Birbaumer, N., and Hummel, F. C. (2009). Brain oscillatory substrates of visual short-term memory capacity. Curr. Biol. 19, 1846–1852.
Schack, B., Klimesch, W., and Sauseng, P. (2005). Phase synchronization between theta and upper alpha oscillations in a working memory task. Int. J. Psychophysiol. 57, 105–114.
Scheeringa, R., Mazaheri, A., Bojak, I., Norris, D. G., and Kleinschmidt, A. (2011). Modulation of visually evoked cortical fMRI responses by phase of ongoing occipital alpha oscillations. J. Neurosci. 31, 3813–3820.
Schroeder, C. E., and Lakatos, P. (2009). Low-frequency neuronal oscillations as instruments of sensory selection. Trends Neurosci. 32, 9–18.
Sherman, S. M. (2001). Tonic and burst firing: dual modes of thalamocortical relay. Trends Neurosci. 24, 122–126.
Silva, L. R., Amitai, Y., and Connors, B. W. (1991). Intrinsic oscillations of neocortex generated by layer 5 pyramidal neurons. Science 251, 432–435.
Singer, W. (1999). Neuronal synchrony: a versatile code for the definition of relations? Neuron 24, 49–65; 111–125.
Singer, W. (2009). Distributed processing and temporal codes in neuronal networks. Cogn. Neurodyn. 3, 189–196.
Smit, D. J., Boersma, M., van Beijsterveldt, C. E., Posthuma, D., Boomsma, D. I., Stam, C. J., and de Geus, E. J. (2010). Endophenotypes in a dynamically connected brain. Behav. Genet. 40, 167–177.
Smit, D. J., Stam, C. J., Posthuma, D., Boomsma, D. I., and de Geus, E. J. (2008). Heritability of “small-world” networks in the brain: a graph theoretical analysis of resting-state EEG functional connectivity. Hum. Brain Mapp. 29, 1368–1378.
Sporns, O., Tononi, G., and Kotter, R. (2005). The human connectome: a structural description of the human brain PLoS Comput. Biol. 1, e42.
Tass, P., Rosenblum, M. G., Weule, J., Kurths, J., Pikovsky, A., Volkmann, J., Schnitzler, A., and Freund, H. J. (1998). Detection of n: M phase locking from noisy data: application to magnetoencephalography. Phys. Rev. Lett. 81, 3291–3294.
Thut, G., Nietzel, A., Brandt, S. A., and Pascual-Leone, A. (2006). Alpha-band electroencephalographic activity over occipital cortex indexes visuospatial attention bias and predicts visual target detection. J. Neurosci. 26, 9494–9502.
Tuladhar, A. M., ter Huurne, N., Schoffelen, J. M., Maris, E., Oostenveld, R., and Jensen, O. (2007). Parieto-occipital sources account for the increase in alpha activity with working memory load. Hum. Brain Mapp. 28, 785–792.
Uhlhaas, P. J., Pipa, G., Lima, B., Melloni, L., Neuenschwander, S., Nikolic, D., and Singer, W. (2009). Neural synchrony in cortical networks: history, concept and current status. Front. Integr. Neurosci. 3:17.
Vanhatalo, S., Palva, J. M., Holmes, M. D., Miller, J. W., Voipio, J., and Kaila, K. (2004). Infraslow oscillations modulate excitability and interictal epileptic activity in the human cortex during sleep. Proc. Natl. Acad. Sci. U.S.A. 101, 5053–5057.
VanRullen, R., and Koch, C. (2003). Is perception discrete or continuous? Trends Cogn. Sci. (Regul. Ed.) 7, 207–213.
Varela, F., Lachaux, J. P., Rodriguez, E., and Martinerie, J. (2001). The brainweb: phase synchronization and large-scale integration. Nat. Rev. Neurosci. 2, 229–239.
Vogel, E. K., and Machizawa, M. G. (2004). Neural activity predicts individual differences in visual working memory capacity. Nature 428, 748–751.
von Stein, A., Chiang, C., and Konig, P. (2000). Top-down processing mediated by interareal synchronization. Proc. Natl. Acad. Sci. U.S.A. 97, 14748–14753.
Voytek, B., Canolty, R. T., Shestyuk, A., Crone, N. E., Parvizi, J., and Knight, R. T. (2010). Shifts in gamma phase-amplitude coupling frequency from theta to alpha over posterior cortex during visual tasks. Front. Hum. Neurosci. 4:191.
Voytek, B., and Knight, R. T. (2010). Prefrontal cortex and basal ganglia contributions to visual working memory. Proc. Natl. Acad. Sci. U.S.A. 107, 42.
Watts, D. J., and Strogatz, S. H. (1998). Collective dynamics of “small-world” networks. Nature 393, 440–442.
Wiener, N. (1948). Cybernetics: Or Control and Communication in the Animal and the Machine Paris: MIT Press.
Womelsdorf, T., and Fries, P. (2007). The role of neuronal synchronization in selective attention. Curr. Opin. Neurobiol. 17, 154–160.
Keywords: synchrony, source modeling, alpha, magnetoencephalography, electroencephalography, phase, amplitude
Citation: Palva S and Palva JM (2011) Functional roles of alpha-band phase synchronization in local and large-scale cortical networks. Front. Psychology 2:204. doi: 10.3389/fpsyg.2011.00204
Received: 16 March 2011; Paper pending published: 16 April 2011;
Accepted: 11 August 2011; Published online: 05 September 2011.
Edited by:
Ole Jensen, Radboud University, NetherlandsReviewed by:
Rufin VanRullen, Centre de Recherche Cerveau et Cognition, Toulouse, FranceKyle Elliott Mathewson, Beckman Institute, University of Illinois, USA
Copyright: © 2011 Palva and Palva. This is an open-access article subject to a non-exclusive license between the authors and Frontiers Media SA, which permits use, distribution and reproduction in other forums, provided the original authors and source are credited and other Frontiers conditions are complied with.
*Correspondence: Satu Palva, Neuroscience Center, University of Helsinki, 00014 Helsinki, Finland. e-mail: satu.palva@helsinki.fi