- 1 The Cognitive Neurophysiology Laboratory, Children’s Evaluation and Rehabilitation Center, Department of Pediatrics and Dominick P. Purpura Department of Neuroscience, Albert Einstein College of Medicine, Bronx, NY, USA
- 2 The Cognitive Neurophysiology Laboratory, Program in Cognitive Neuroscience, Departments of Psychology and Biology, City College of the City University of New York, New York, NY, USA
Evidence has amassed from both animal intracranial recordings and human electrophysiology that neural oscillatory mechanisms play a critical role in a number of cognitive functions such as learning, memory, feature binding and sensory gating. The wide availability of high-density electrical and magnetic recordings (64–256 channels) over the past two decades has allowed for renewed efforts in the characterization and localization of these rhythms. A variety of cognitive effects that are associated with specific brain oscillations have been reported, which range in spectral, temporal, and spatial characteristics depending on the context. Our laboratory has focused on investigating the role of alpha-band oscillatory activity (8–14 Hz) as a potential attentional suppression mechanism, and this particular oscillatory attention mechanism will be the focus of the current review. We discuss findings in the context of intersensory selective attention as well as intrasensory spatial and feature-based attention in the visual, auditory, and tactile domains. The weight of evidence suggests that alpha-band oscillations can be actively invoked within cortical regions across multiple sensory systems, particularly when these regions are involved in processing irrelevant or distracting information. That is, a central role for alpha seems to be as an attentional suppression mechanism when objects or features need to be specifically ignored or selected against.
“Sit in reverie, and watch the changing color of the waves that break
upon the idle seashore of the mind.”
-Henry Wadsworth Longfellow
The ability to selectively attend to a specific subset of one’s sensory realm is at the root of human cognition and negotiation of one’s environment. The alternative, whereby all information in one’s sensory domain is processed to the level of consciousness, does not allow for normal function and may be partially involved in certain neural disorders such as Autism spectrum disorders (cf. Markram and Markram, 2010) and attention deficit disorder (Armstrong et al., 2001). By necessity, successful task completion in daily life employs selective attention to one extent or another. By inference, the ability to attend is assumed to be a “capacity-limited” system and to some degree, humans have executive or conscious control over what aspects of their environment are tapping this limited system from moment-to-moment (e.g., Broadbent, 1958; Kahneman, 1973; Neely, 1977; Schneider and Shiffrin, 1977; Shiffrin and Schneider, 1977; Schneider and Fisk, 1982). This endogenous form of attention is distinct from attention that is captured by salient stimuli in the environment in an involuntary fashion (exogenous attention). How the brain achieves endogenous attentional selectivity has been a matter of vigorous research for some decades now. Advances in neuroimaging technologies such as the hemodynamic functional imaging methodologies (fMRI and PET) and the ability to conduct high-density multi-channel electro- and magneto- encephalographic recordings (EEG and MEG) have allowed for unprecedented advances in our understanding of the physiology of human selective attention. This report specifically addresses evidence for the role of oscillatory brain mechanisms in selective attention (e.g., Vanni et al., 1997; Foxe et al., 1998; Gruber et al., 1999; Shibata et al., 1999; Sokolov et al., 1999; Worden et al., 2000; Sauseng et al., 2005; Yamagishi et al., 2005; Kelly et al., 2006; Thut et al., 2006; Rihs et al., 2007; Snyder and Foxe, 2010).
Two basic varieties of attentionally mediated, selective modulation of the neural circuitry involved in processing a particular stimulus array can be envisioned. The first, and by far the most often reported, involves the selective enhancement of neural responsiveness or efficacy in those cells responsible for processing the particular stimulus upon which a subject’s attention is focused, whether that stimulus is already present or merely anticipated (e.g., Moran and Desimone, 1985; Haenny and Schiller, 1988; Corbetta et al., 1993; Luck et al., 1997). The second variety of attentional modulation involves selective inhibition or disengagement of those neurons responsible for processing stimuli outside of the focus of attention, so that competing or distracting information is not or will not be processed fully (e.g., Moran and Desimone, 1985; Motter, 1993; Foxe et al., 1998; Reynolds et al., 1999; Vanduffel et al., 2000; Worden et al., 2000). This would involve placing the neural circuitry normally responsible for processing such distracters in some form of inhibited or decreased receptivity state. Evidence from behavioral studies supports such a mechanism, as reaction times to stimuli presented at non-attended locations are slower relative to those at attended locations (e.g., Posner et al., 1980), and pre-cuing visual targets when they are presented with accompanying distracters improves performance to levels seen when no distracters were presented (Morgan et al., 1998).
Some evidence suggests that one correlate of the first variety of attention, enhancement of neural processing for stimuli at the focus of attention, is the selective modulation of evoked gamma-band oscillatory activity (e.g., Gruber et al., 1999; Shibata et al., 1999; Sokolov et al., 1999; Fries et al., 2001). The evoked gamma-band response has been implicated as a neural mechanism for feature binding (e.g., Singer, 1993, 1999), and these studies have suggested that this binding function can be selectively invoked during attention tasks. Attention effects in the gamma-band have typically been observed subsequent to stimulus onset. A key aspect of endogenous attention, however, is the ability to prepare attentional resources in preparation for an expected stimulus prior to its onset.
One mechanism that has been suggested for the complementary, suppressive form of attention in the visual domain is the selective invocation of cortico-cortical and cortico-thalamic oscillations (Lopes da Silva, 1991). Since alpha oscillations (8–14 Hz) are known to be related to general alerting and ongoing visual processing (Adrian and Matthews, 1934; Klimesch et al., 1998) and are seen in the EEG, even when subjects are involved in highly demanding tasks, we proposed that such oscillations may also be involved in selective attention mechanisms (Foxe et al., 1998). In support, the amplitudes of both the auditory and visual N1-P2 ERP components are modulated based on stimulus-preceding alpha amplitude (e.g., Brandt and Jansen, 1991; Brandt et al., 1991; Rahn and Başar, 1993a,b; Brandt, 1997) with the caveat that in these studies, the reported effects could be mainly due to arousal issues. More compellingly, Vanni et al. (1997) demonstrated a parieto-occipital alpha effect such that alpha level became higher in the period following presentation of a “non-object” stimulus as opposed to meaningful objects. They suggested that this represented a disengagement of attention by parietal attention gating structures in the inferior parietal region. We predicted that such attentional disengagement might also be a facet of anticipatory attentional biasing mechanisms. Our results regarding this prediction from a series of studies are discussed below.
Alpha and Anticipatory Gating of Attention
Anticipatory Biasing
In our laboratory, we have been particularly interested in detailing the top-down mechanisms by which the brain establishes biased attentional states – that is, anticipatory sets. Until the past decade or so the vast majority of attention research had concentrated on the effects of attention on the processing of attended versus unattended stimuli that occurs subsequently, as a result of attentional biasing (see Desimone and Duncan, 1995; Hillyard and Anllo-Vento, 1998). When the brain is involved in attentionally demanding tasks, it is advantageous to direct attention to the relevant stimulus, location, or action that is to be engaged in, prior to the arrival of the stimulus or execution of the action. We have reported a neural correlate of the second form of biasing mechanisms, anticipatory suppression, in a number of contexts: anticipatory suppression of one modality in favor of another (Foxe et al., 1998; Fu et al., 2001), suppression of portions of space that are likely to contain distractor information (Worden et al., 2000; Kelly et al., 2006), as well as suppression of visual features such as color or motion (Snyder and Foxe, 2010).
The Cuing Paradigm
In order to study the preparatory mechanisms of attentional biasing, we have employed the “cuing paradigm” to invoke endogenous biasing of the attentional system. This is an effective experimental means of ensuring invocation of a high level of endogenous control by the use of symbolic cues that actively instruct the subject in an unpredictable manner to switch attention between tasks. That is, a centrally presented cue such as an arrow, word, or sound is employed (stimulus 1 – S1), which informs of an upcoming task or indicates a specific location, modality, or feature for subsequent analysis (stimulus 2 – S2). This manipulation is a nice experimental version of what is a fairly natural way of apportioning attention in our environment. That is, humans spend a large part of their existence assigning attentive resources to their environment based on both external and internal instructional sets.
Such endogenous cuing paradigms can be classified into two basic categories: “probabilistic” and “instructional.” In a probabilistic cuing paradigm, participants are instructed to respond to all target S2’s, including those that may occur at an uncued location. Critically, the cue indicates the correct location of the target on a high proportion of trials, which encourages the participants to utilize the information provided by the cue. The uncued location in this case cannot be considered a source of distracters, however, since participants still need to respond to targets there. In contrast, an instructional cuing paradigm requires the participants to respond to targets occurring at the cued location only, and to ignore the uncued location completely. In this way, the uncued location becomes simply a source of potentially distracting information, since stimuli occurring there will never warrant a response. On the other hand, since no response is ever made to such distracters, a physiological measure is needed to evaluate the processing thereof.
Alpha-Band Effects in Intersensory Attention
Using an instructional endogenous cueing paradigm, we originally found differential modulation of parieto-occipital alpha-band (8–14 Hz) oscillatory activity in the period preceding an imperative stimulus (S2) when visually presented word-cues (S1) instructed subjects in an intersensory attention paradigm (Foxe et al., 1998; Figure 1). In that experiment, subjects were cued to attend either the visual or auditory portion of a subsequent compound audio–visual stimulus, which occurred predictably 1.1 s after the cue. We showed that the cue instruction to attend the auditory modality (the word “BEEP”) resulted in significantly larger 8–14 Hz activity over parieto-occipital cortex in the anticipatory period preceding the S2 than when subjects were cued to attend the visual modality (the word “FLASH”). This effect was not due to eye closing or gaze issues as eye movements were carefully monitored and subjects were instructed to maintain fixation during both attend-auditory and attend-visual anticipatory periods. We hypothesized that this oscillatory enhancement in the case of the attend-auditory instruction might reflect anticipatory gating of visual processing by parieto-occipital structures, which are known to be involved in attentional switching and disengagement within the visual modality (e.g., Posner et al., 1984; Farah et al., 1989; Posner and Petersen, 1990).
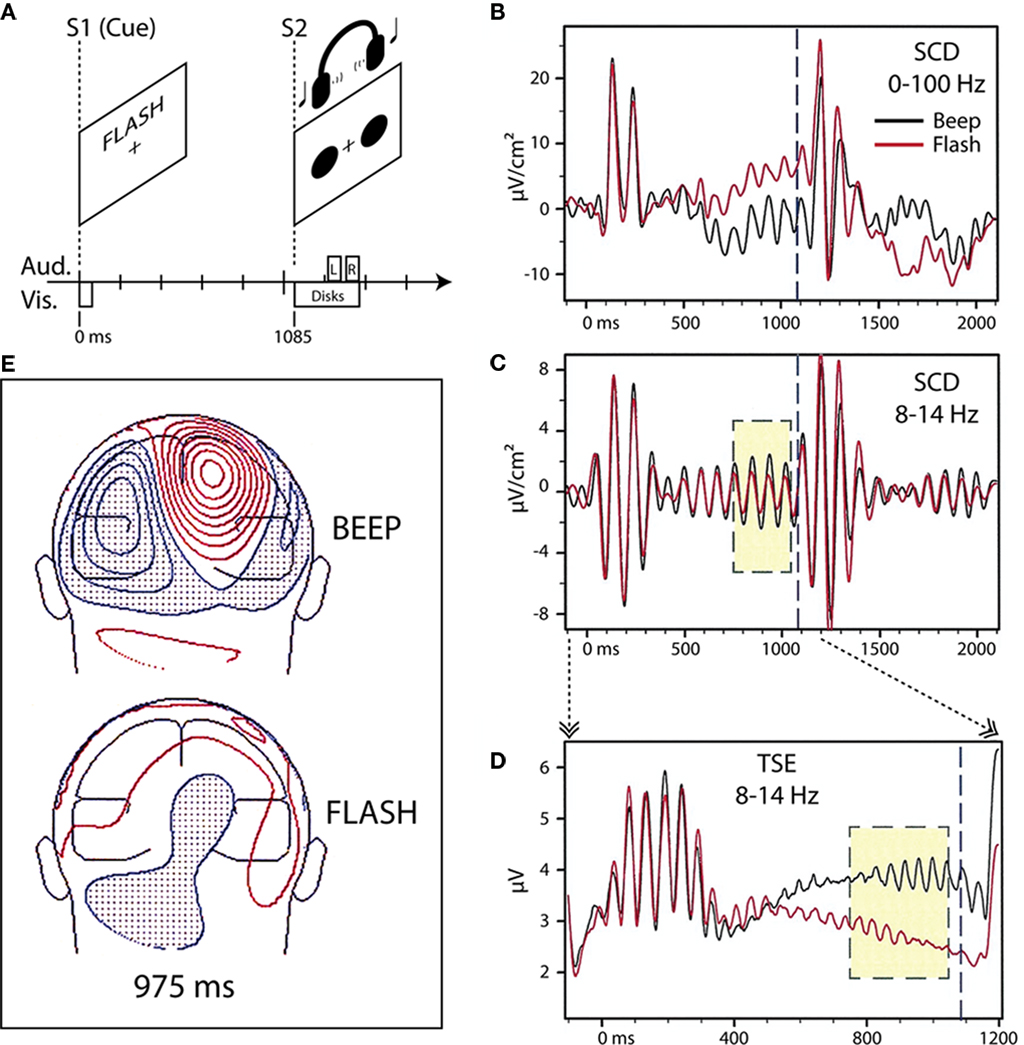
Figure 1. Adapted from Foxe et al. (1998). (A) Task schematic. Participants viewed a cue word (BEEP or FLASH) on a computer screen to start each trial. The cue indicated whether audition (BEEP) or vision (FLASH) was relevant for the trial. After a 1085-ms cue-target interval of no stimulation, the participants were presented with a pair of red disks on the screen and a concurrent pair of tones via headphones. For the visual target, one of the red disks was displaced slightly with respect to the reflection of the other disk across the vertical meridian (on standard trials, the two disks were symmetric about the vertical meridian). For the auditory target, the two tones had slightly different pitches (the two tones had the same pitch on standard trials). Participants were instructed to press a button upon detection of a target in the cued sensory modality, and to withhold response otherwise. Participants maintained central fixation throughout the trial. (B) Scalp current-density (SCD) waveforms from one subject (BH) at a right parieto-occipital scalp site to the beep (black trace) and flash (red trace) cues. (C) The same waveforms, band-pass filtered (8–14 Hz) reveal greater stimulus-locked alpha activity in the 300-ms (yellow box) before onset of the compound S2 (1085 ms, dashed blue line) when attention has been cued to the auditory modality. (D) Temporal–spectral evolution (TSE) waveforms for the alpha band, derived for the cue-target interval by full-wave rectifying the individual band-pass filtered trials prior to averaging, show a difference in the total alpha amplitude (i.e., both stimulus-locked and non-stimulus-locked). (E) Posterior view of the topographical distribution of current-density 975 ms following presentation of beep (top) and flash (bottom) cues for one subject (KH). Greater parietal field power is evident for the auditory cue compared to the visual cue. Red iso-contour lines (0.1 μV/cm2 increments) indicate positive values and blue, negative.
Alpha-Band Effects in Visual Spatial Attention
We interpreted the results of Foxe et al. (1998) in terms of visual spatial attention theories that posit parietal control of visual selective attention such as those of Van der Heijden (1991), LaBerge (1997). In such models, the posterior parietal areas of the fast dorsal visual stream mediate the extent of processing of a particular stimulus through parietal inputs to the thalamic relay nuclei, nucleus reticularis, and the putamen, and also through modulatory inputs to the ventral visual stream, which is specialized for the high level extraction of featural information from stimuli. That is, the spatially specialized dorsal stream areas control the gateways to ventral visual processing. How exactly this is achieved is currently a matter of debate. We suggested that the enhanced parieto-occipital alpha-band activity seen in our intersensory selective attention paradigm represented a selective inhibition of “all” of visual space and was one mechanism by which visual space could be selectively inhibited. The prediction from these results would be that during visual spatial selective attention paradigms, such alpha-gating mechanisms should be selectively deployed to regions of space that need to be ignored. That is, alpha-gating should occur in cortices that are likely to receive distracter information. We then examined this prediction in a visuo-spatial cueing study (Worden et al., 2000; Figure 2).
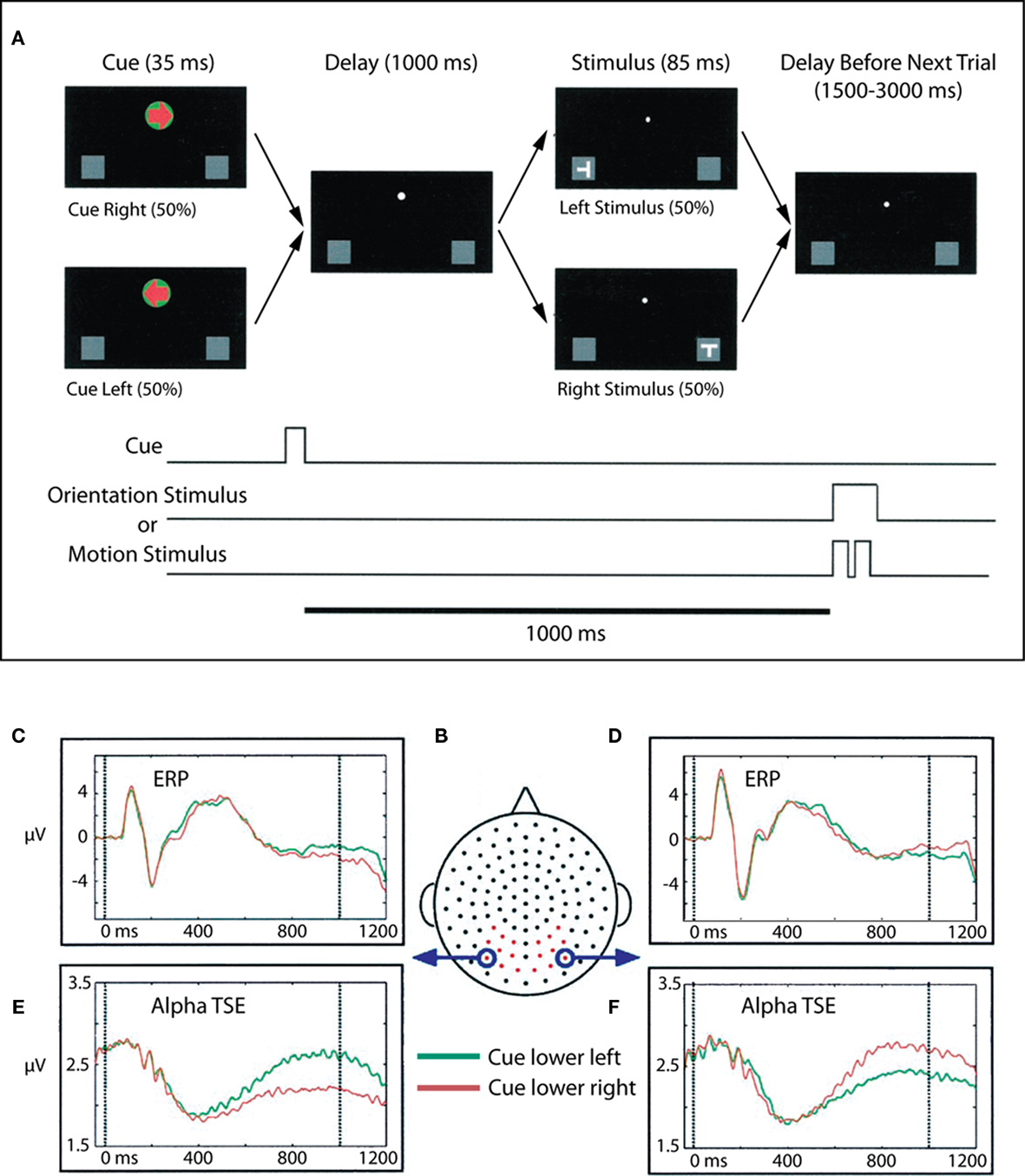
Figure 2. Adapted from Worden et al. (2000). (A) Task schematic. At the start of each trial, participants were presented with a central arrow cue that instructed them to attend to the left or right hemifield. Following a 1-s cue-target interval, a stimulus was presented at either the left or right location. Participants were charged with making a difficult orientation or motion discrimination of stimuli occurring at the cued location, while ignoring all events at the uncued location. Participants maintained central fixation throughout the trial. (B) Dorsal view (nose at top) of the electrode layout. Electrodes used for statistical analysis are plotted in red, circled electrodes were used to generate the accompanying waveforms. (C,D) ERPs to the lower left and right cues (collapsed across motion and orientation trials) for two occipital electrode sites, averaged over 10 subjects. Data for attend lower left are plotted in green, and data for attend lower right are plotted in red. (E,F) Corresponding alpha-band (8–14 Hz) TSE waveforms for the same electrodes. A sustained divergence in TSE amplitude is seen starting at ∼500 ms, which depends on both the cued direction of attention and the side of recording. TSE amplitudes are larger over occipital cortex ipsilateral to the direction of attention.
In this visuo-spatial experiment, centrally presented arrow cues were used to direct attention to the left or right visual fields and alternately to the upper and lower quadrants within the visual hemifields. Subjects were required to make orientation and motion-direction judgments upon the subsequent S2 stimuli. It should be emphasized that only stimuli occurring at the cued location were relevant to the task, such that stimuli occurring opposite to the cued location could be deemed distracters. Compellingly, we found that deployment of visual attention to one hemifield resulted in anticipatory sustained focal increases in alpha-band activity over occipital cortex ipsilateral to the cued direction of attention. Further, these foci also moved retinotopically depending on whether attention was deployed to the upper or lower quadrants. Thus, in a spatial selective attention condition, enhanced alpha-band activity was seen over the retinotopic area that was likely to contain distracter stimuli, consistent with a role for these oscillatory processes in inhibition of non-cued spatial locations and consistent with the predictions of Foxe et al. (1998). This basic finding of retinotopically specific alpha-band mediated suppression of potentially distracting locations has subsequently been replicated and expanded several times (Sauseng et al., 2005; Yamagishi et al., 2005; Kelly et al., 2006; Thut et al., 2006; Rihs et al., 2009; Cosmelli et al., 2011).
Cross-Sensory Cuing of Attention
In Foxe et al. (1998), we used visually presented word-cues to direct the deployment of attention to either the visual (FLASH) or auditory (BEEP) modalities. A central question in intersensory attention and more particularly in multisensory integration is the extent to which there are cross-sensory links in attention mechanisms. A number of papers have addressed this issue (Perrott et al., 1990, 1996; Spence and Driver, 1996, 1997a,b; Eimer and Schröger, 1998; Dufour, 1999; Eimer, 1999). One question is whether cues presented in a modality other than vision could invoke the alpha-gating mechanism reported by Foxe et al. (1998) and Worden et al. (2000). We investigated cross-sensory cuing effects upon the alpha-gating effect (Fu et al., 2001) by using auditory cues to inform subjects in an intersensory attention experiment similar to the one used in Foxe et al. (1998). We found that the use of auditory cues can indeed invoke the alpha-gating mechanism. In this experiment, subjects received an auditory cue (1 click = attend-auditory; 2 clicks = attend-visual) which was followed 1.2 s later by a compound auditory/visual imperative stimulus. As with our previous study, subjects were required to perform a difficult sensory discrimination task within the cued modality and to ignore the uncued/distracter modality, while maintaining fixation nonetheless. We inspected the alpha-band activity in the period following the cue-stimulus but preceding arrival of the to-be-attended imperative stimulus. The fundamental finding of Foxe et al. (1998) – increases in parieto-occipital alpha power in preparation for the auditory task – was replicated. This result indicates that alpha-mediated visual gating can be cross-sensorily cued. Similarly, Jones et al. (2010) used a visual cue to direct spatial attention in anticipation of a tactile S2 and observed spatially specific shifts in alpha band power over somatosensory cortices. Successful cross-sensory cuing of alpha-mediated gating mechanisms is significant because it underscores the endogenous origin of the effects. That is, mere low-level processing of the sensory aspects of the cue cannot account for the attentional effects.
Visual Feature-Based Attention
We also asked if this gating function of alpha-band activity was specific to spatial and intersensory attention, or rather if alpha could play a role in the biasing of attention between other stimulus parameters within a given modality, such as visual features (Snyder and Foxe, 2010; Figure 3). It was well established from prior research that attention can be deployed to non-spatial visual features, such as color or motion parameters, facilitating the processing of subsequent stimuli incorporating the attended feature, independently of spatial location (e.g., Corbetta et al., 1991; Martinez-Trujillo and Treue, 2004; Wylie et al., 2004, 2006; Most and Astur, 2007; Egner et al., 2008). We again utilized an instructional cuing paradigm, although in this case the cues directed participants to attend to either the color or motion of an upcoming stimulus consisting of many small moving and colored dots. Participants were tasked with making a difficult discrimination with respect to the cued feature. We chose the features of color and motion because they have well-described, spatially separated cortical processing centers in the dorsal and ventral visual streams, respectively (Ungerleider and Mishkin, 1982). Using independent components analysis (ICA), we were able to isolate cortical sources that were highly dynamic in the alpha band during the preparatory cue-S2 interval, and some of these sources differentiated between attention to color and attention to motion. Such sources located in putative dorsal stream regions showed increased power in the alpha band when motion was irrelevant, whereas sources located in ventral regions showed increased alpha power when color was irrelevant. This pattern of results is analogous to the suppression effect observed with spatial attention, in which cortical areas responsible for processing irrelevant or distracting information show anticipatory increases in alpha band power.
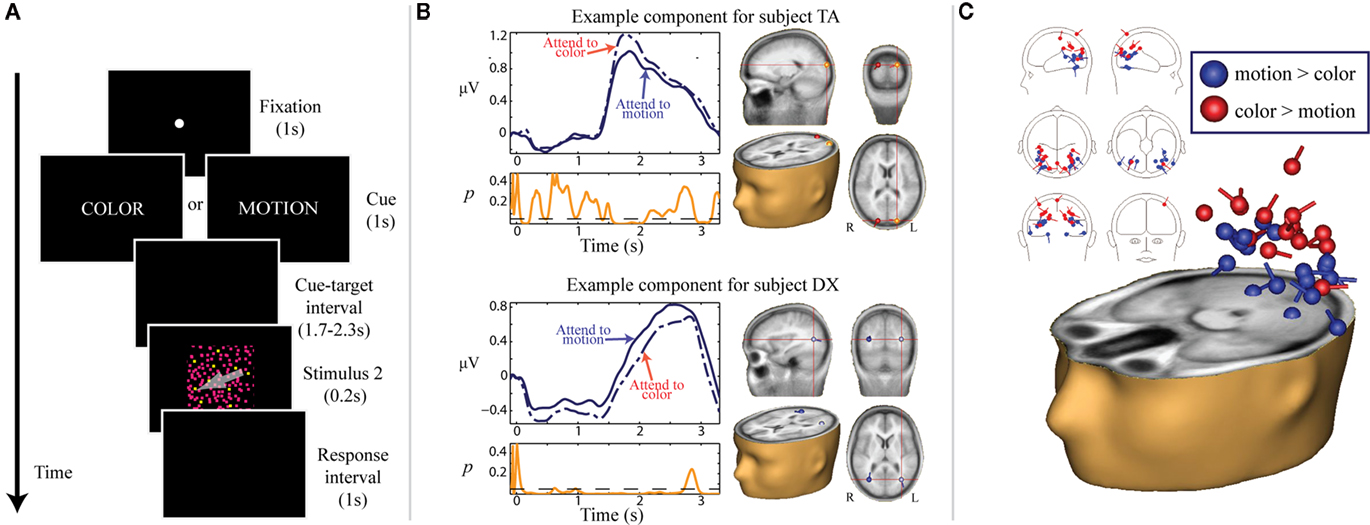
Figure 3. Adapted from Snyder and Foxe (2010). (A) Task schematic. Participants were presented with a word cue (e.g., COLOR or MOTION) at the start of each trial that indicated the relevant feature of the upcoming S2. Following a 1.7 to 2.3-s cue-target interval, a random dot stimulus (S2) was presented for 200 ms. Dots comprising the S2 were all colored and moving. For color targets, two different colors of dots were present in the stimulus (on standard trials, all dots were uniformly colored). For motion trials, some dots moved in a different direction from the rest (on standard trials, all dots moved in the same direction). Participants were instructed to respond with a button press if they detected a target in the cued feature, and to withhold response otherwise. (B) Examples of “feature-sensitive, alpha-reactive” components for two representative subjects. Scalp-recorded data were first separated into independent components using ICA. TSE waveforms were derived for each component by Hilbert-transforming band-pass filtered (8–15 Hz) single-trials and averaging the instantaneous amplitudes across trials. For each participant, a few components were highly dynamic in the alpha range during the preparatory period (“alpha-reactive” components), and some of these alpha-reactive components moreover showed power differences based on which feature was cued (“feature-sensitive”). TSE waveforms illustrating this effect are depicted in violet for two representative subjects. The solid lines represent attention to motion and the dashed/dotted lines represent attention to color. Below the TSE waveforms, running p-values for the alpha power difference between the two attention conditions is plotted in orange. To the right of the TSE waveforms, point-equivalent dipole source estimates for the corresponding components are depicted in a standard MNI brain volume. (C) Localization of all feature-sensitive alpha-reactive components across participants. Components are color-coded according to the direction of feature-sensitivity: components with greater alpha power for attention to color than for attention to motion are plotted in red, and components with greater alpha power for attention to motion than for attention to color are plotted in blue. The distributions of the estimated sources for two types of components are significantly different. Components with higher alpha power for attention to color are generally localized to putative dorsal visual stream regions, whereas components with higher alpha power for attention to motion are generally localized to putative ventral visual stream regions. This pattern of results is consistent with a suppressive role of anticipatory alpha-band power increases.
Auditory and Somatosensory Spatial Attention
Alpha-mediated suppression has thus been shown to be robustly involved in anticipatory attention in the visual domain. It is natural to ask if alpha-band activity also plays a role in the anticipatory suppression of information in other sensory domains. Several studies have investigated this possibility.
A role for alpha-mediated biasing of spatial attention has been reported when attention is directed to S2’s presented in the auditory domain (Kerlin et al., 2010; Banerjee et al., in press). Kerlin et al. (2010) directed participants to selectively attend to one of two competing speech streams in an auditory spatial attention task while the researchers recorded EEG. They found alpha-band increases over parietal cortex ipsilateral to the cued direction, consistent with the findings of Worden et al. (2000). It should be mentioned, however, that the researchers examined only the interval during which the stimulus was actually presented, rather than the preparatory interval. Additionally, a visual arrow cue was used, which has been shown to have strong exogenous effects on the allocation of visual attention (e.g., Ivanoff and Saoud, 2009), admitting a potentially confounding factor. In Banerjee et al. (in press), we used centrally presented symbolic auditory cues to direct attention to auditory S2’s appearing to the left and right of the participant. Participants’ only visual concern was to maintain fixation on a small dot on a computer screen. No visual information was available concerning the potential location of the S2’s, which were obscured behind curtains in the dimly lit recording chamber. It was observed that parieto-occipital alpha power increased ipsilateral to the cued direction during the cue-target interval. This suggests that alpha-mediated biasing operates within sensory modalities other than vision, since the participants in this case never had a need to bias visual processing to perform the task. Similar biasing effects in the alpha band have been observed for spatial attention in anticipation of a tactile event (e.g., Jones et al., 2010; Van Ede et al., 2011). In these tactile attention experiments, alpha band increases are typically observed over somatosensory cortex ipsilateral to the cued direction, suggesting some degree of sensory specificity to the mechanism. Such diverse observations of alpha-mediated suppression suggest that it might be a general mechanism for the suppression of parts of sensory space.
Alpha-mediated attentional gating has thus been robustly observed across a variety of attention tasks, in multiple sensory modalities and with respect to several different stimulus parameters. This prevalence of alpha-mediated gating suggests that it may be a general mechanism for attentional gating of cortical processing.
Can Alpha BE Considered an Active Mechanism for Attentional Suppression?
One alternative interpretation of alpha-band modulations in selective attention paradigms could be that alpha-band power is not increased in areas processing distracter information, but rather that such “increases” in fact reflect a return to baseline levels for areas processing distracters, whereas maintenance of relative desynchronization in the alpha band in areas processing potential target information reflects preparatory enhancement and leads to the observed topographical asymmetries. This argument has its historical roots in the notion that alpha activity represents a cortical “idling” state, whereas the relative absence of alpha reflects cortical activity (e.g., Pfurtscheller, 1992), and is of particular relevance when visual cues are used, as visual stimulus onset itself leads to substantial decreases in the amplitude of ongoing alpha oscillations (e.g., Mulholland, 1965; Pfurtscheller and Aranibar, 1977), complicating the picture of spectro-temporal dynamics. We investigated this possibility in an experiment that used rapid serial visual presentation to maintain a consistent level of stimulus-related desynchronization in the alpha band (Kelly et al., 2006; Figure 4). In this experiment, participants were presented with a stream of letter stimuli to both visual hemifields. Presentation rates varied from 9.45 to 17.01 Hz, and each stimulus had an 11-ms duration. A centrally presented arrow cue instructed participants to attend to one of the two streams, and the participants were instructed to count the occurrences of a target letter in the attended stream. The key result was that, following the cue, alpha power ipsilateral to the cued direction increased above pre-cue baseline levels, and no decreases in alpha power contralateral to the cued direction were observed. Because bilateral stimulation continued throughout each trial, we concluded that such increases in alpha power reflect active suppression of the to-be-ignored stimulus stream, rather than a mere return to baseline “idling.” It should also be noted that in our earlier study (Fu et al., 2001) where auditory cues were used and so stimulus-related alpha desynchronization was not observed, we also found that anticipatory alpha was statistically greater than pre-cue baseline levels.
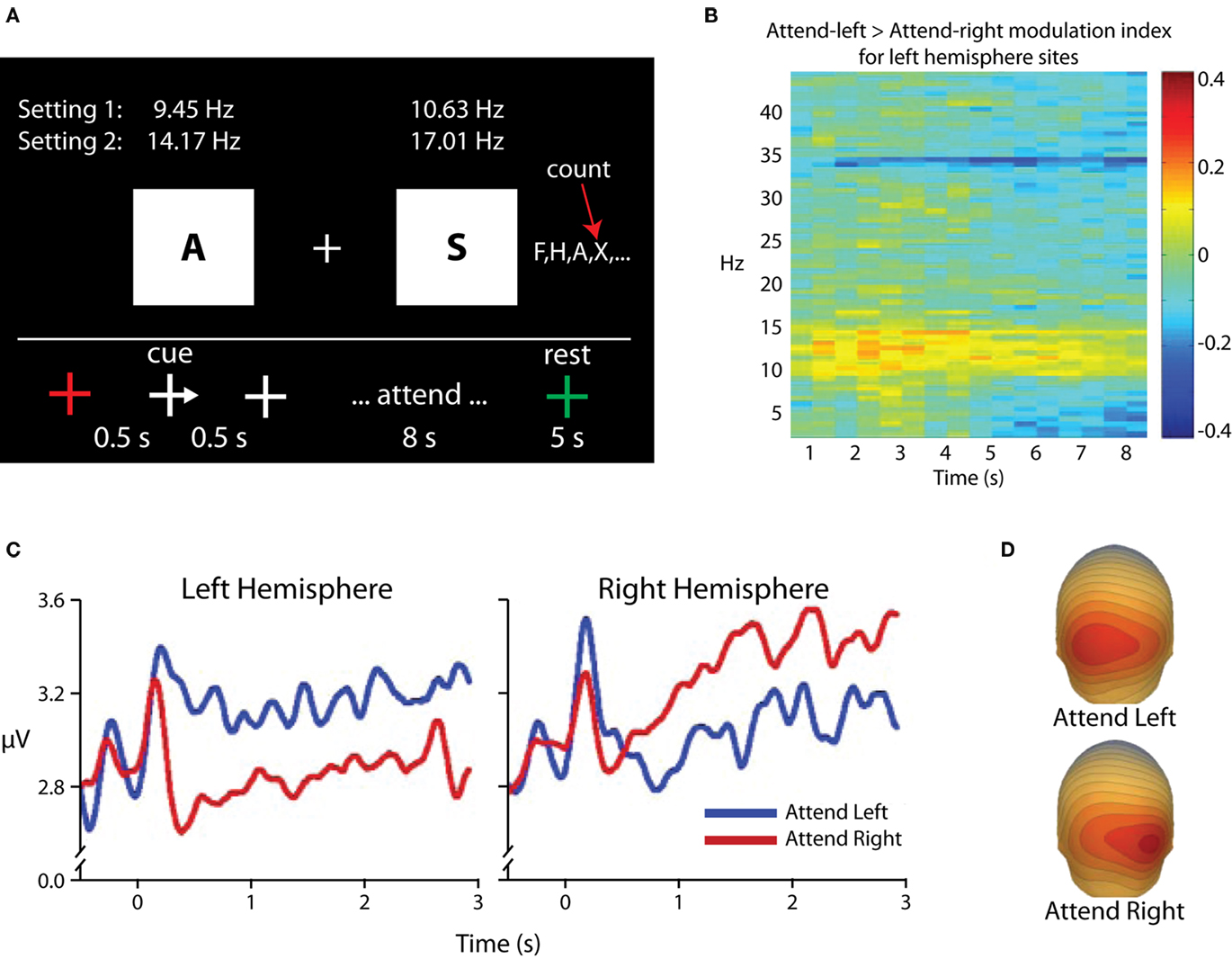
Figure 4. Adapted from Kelly et al. (2006). (A) Task schematic. Participants maintained fixation on the central cross while a rapid sequence of letters was presented to each hemifield. For each trial, a transiently presented arrowhead on the fixation cross directed attention to one of the two letter streams, and participants were instructed to count the occurrences of the letter “X” in the attended stream for 8 s. (B) Time–frequency surface plot for all time points within the attended period and for frequencies between 2 and 45 Hz, showing attentional modulation {measured as log [Power(attend-Left)/Power(attend-Right)]} for the left hemisphere parieto-occipital electrodes. Modulation is localized to the alpha band with additional modulations seen at stimulation frequencies and harmonics. (C) TSE waveforms time-locked to cue-onset (0 ms), averaged over three parieto-occipital electrodes from each hemiscalp. A marked increase relative to pre-cue baseline can be seen over each hemisphere when ignoring the contralateral hemifield. (D) Scalp topography of alpha-band power for attention deployments toward the left (top) and right (bottom) stimuli. The focus of increased alpha can be seen over parieto-occipital scalp contralateral to the ignored hemifield.
Alpha-band measures of selective attention have also now been shown to predict task performance. For example, detection (Mathewson et al., 2009) and discrimination (Hanslmayr, et al., 2007; Van Dijk et al., 2008) of centrally presented near-threshold visual stimuli have been shown to be inversely related to the amplitude of alpha preceding stimulus onset. In other words, processing is less effective when alpha amplitude is greater. Mathewson et al. (2009) moreover reported that phase of ongoing alpha predicted detection ability, a very interesting finding in need of further investigation in our view.
One study from our group examined the relationship between inter-individual differences in resting alpha band power and performance on a sustained attention to response task (SART; Dockree et al., 2007). In this paradigm, participants viewed the digits “1” through “9” presented sequentially on a computer screen. The participants were instructed to press a button to the onset of each digit, except for digit “3,” to which response was to be withheld. Prior work had established a component of the ERP in response to the preceding digit (i.e., “2”) that predicted whether participants would commit an error of commission when the digit “3” was presented. This so-called “late positive 1” (LP1) component was lower-amplitude before errors of commission than before correctly withheld responses. For this study, we compared the tonic levels of alpha in each participant to the amplitude of that participant’s LP1 component and task performance. It was found that participants with greater tonic alpha levels had larger LP1 components, and that this relationship predicted performance on the sustained attention task.
With respect to spatial selective attention, the strength of attentional biasing as reflected by the degree of asymmetry of alpha power over visual areas has been shown to be an effective predictor of task performance (Thut et al., 2006; Kelly, et al., 2009). Furthermore, the degree of such alpha asymmetry has been shown to scale with the probability of a valid cue in a probabilistically cued visual spatial attention paradigm (Gould et al., 2011), suggesting implicit optimization of the mechanism.
Does Alpha Shape Perception? Toward Studying Its Causal Role
A series of powerful experiments by Romei et al. (2008a,b, 2010) used transcranial magnetic stimulation (TMS) to demonstrate strong associations between ongoing alpha-band power over visual cortex and perceptual experience. In the first such study (Romei et al., 2008a), the researchers applied magnetic pulses to visual cortex of human participants, which resulted in some of the participants experiencing visual percepts (phosphenes). For each participant, the researchers determined the minimum strength of the magnetic pulse required to elicit phosphenes (the “phosphene threshold”). Additionally, the researchers recorded resting EEG from each participant. They found that phosphene threshold was positively correlated with resting power in the alpha band across individuals. That is, more intense stimulation was required to evoke visual percepts in individuals with greater alpha levels, consistent with a role for alpha activity as an active gating mechanism. Those individuals with the very greatest levels of resting alpha did not perceive phosphenes with even the highest-intensity TMS tested. It is worth noting that no relationship was found between phosphene threshold and activity in other canonical frequency bands tested (i.e., delta, theta, beta, or gamma). A follow-up study using concurrent TMS and EEG revealed that spontaneous fluctuations of alpha-band power reflect the excitability of cortex on a single-trial basis within single subjects and in a spatially specific manner (Romei et al., 2008b), providing another compelling demonstration of the intimate links between moment-to-moment alpha-band fluctuations within visual cortex and the ongoing susceptibility of those regions to be activated.
In the most direct test of the potentially causal role of alpha band activity in attentional suppression, Romei et al. (2010) again used TMS, although in this case they sought to externally drive rhythmic neural activity and observe the effects that such externally driven oscillations would have on perception. Rhythmic TMS pulses were applied either within the alpha range (10 Hz), or at a frequency above (20 Hz), or below (5 Hz) this range. The participants were asked to report when they perceived a near-threshold visual stimulus that was presented occasionally following the TMS stimulation. TMS was applied to occipital and parietal regions, and to left and right hemispheres. Additionally, so-called “sham” TMS conditions were included, which mimic the sound of true TMS without the stimulatory effects. The researchers found that TMS at 10 Hz impaired visual detection contralateral to the site of stimulation for both occipital and parietal stimulation sites, consistent with a spatially specific suppressive role of alpha-band activity with a cortical origin. Furthermore, visual detection was improved ipsilateral to the site of 10 Hz stimulation, suggesting a freeing of cognitive resources à la biased competition models. These effects had a short duration; subsiding within 3 s of TMS. Critically, no effects on visual detection were seen for stimulation at either 5 or 20 Hz.
The situation may be more complex than simple gating of low-level stimulus processing, however. Another study by our group (O’Connell et al., 2009) used a visual sustained attention task to examine the EEG correlates of attentional lapses, as indexed by performance errors. The imperative stimulus in this experiment constantly flickered at 25 Hz, leading to a steady-state visual-evoked potential (SSVEP) that served as the measure of low-level visual processing. We found that power in the alpha band was much higher preceding errors, and that such alpha increases could predict errors up to 20 s before they occurred. However, no differences in SSVEP amplitude were observed between periods preceding erroneous performance and those preceding correct performance. Thus, while we concluded that alpha-band power was a reliable index of attentional lapses, the results also suggested that its effect was not to diminish the efficacy of basic visual processing.
Neural Basis of the Alpha Rhythm
Given the compelling accumulation of evidence across multiple laboratories pointing to an active role for alpha-band oscillations in selective attentional suppression, it is of extreme interest to examine the putative underlying neural mechanisms that give rise to this brain rhythm. The earliest description of the alpha brain rhythm is generally credited to Hans Berger from his groundbreaking 1929 paper, “On the electroencephalogram in man”:
The electroencephalogram represents a continuous curve with continuous oscillations in which… one can distinguish larger first order waves with an average duration of 90 milliseconds [Alpha waves] and smaller second order waves of an average duration of 35 milliseconds [Beta waves]. (Berger, 1929)
Despite the fact that alpha was one of the first signatures of the EEG noted and is perhaps the best known brain rhythm outside of the neurosciences, and given that no specialized training whatsoever would be necessary to pick out this most prominent of brain rhythms from an ongoing EEG signal, it is perhaps rather surprising how incomplete our knowledge of the neural basis of the alpha remains. In what follows, we will attempt to synthesize some of the basic knowledge that does exist across species and methodologies, with the caveat that this will be a necessarily incomplete survey.
Thalamo-Cortical Contributions
A key combined PET and EEG study in humans showed an inverse correlation between thalamic metabolism and alpha power in the EEG during a relaxed state (Lindgren et al., 1999). That is, increased thalamic activity was correlated with less alpha EEG power while less thalamic metabolic activity was correlated with higher alpha power, supporting the notion that at least the non-specific alpha seen in the ongoing EEG of humans has a thalamic component. These findings are consistent with attention models such as those of LaBerge (1997) in that activity in the relay nuclei of thalamus might be suppressed by increased alpha oscillatory power in parietal cortex. Nevertheless, there may also be an important distinction between the alpha that is seen in the generally unaroused or eyes-closed state, as in the Lindgren study, and the alpha seen when subjects are involved in selective attention tasks such as those reviewed above, for which arousal is specifically controlled (see below for further discussion of variants of alpha waves).
Additional evidence for a thalamic contribution to alpha-band oscillations comes from investigations of ∼10 Hz sleep-spindles. As Steriade et al. (1990) point out; there has been an “unwritten consensus that alpha waves are congeners or embryos of spindle waves,” although this assumption has been becoming increasingly doubtful, as will be discussed below. Contreras et al. (1996) have shown that spindle oscillations are largely under the control of cortico-thalamic feedback. That is, by removing one cortical hemisphere in anesthetized cats, they showed that spindle sequences in thalamus did not retain their normal synchrony but became desynchronous. In addition, disrupting intracortical connectivity by making deep coronal cuts through the suprasylvian gyrus did not affect the synchronization of unaffected cortex. Destexhe et al. (1998) proposed a model based on these results and the extensive experimental findings at the Steriade laboratory whereby cortico-thalamic projections operate on the thalamus through excitatory inputs to GABAergic neurons in the nucleus reticularis, recruiting relay cells through a process of inhibition and rebound (Steriade et al., 1985; Destexhe et al., 1998). Importantly, reticular neurons of the visual perigeniculate nucleus (PGN) are known to inhibit thalamic relay neurons (Ahlsén et al., 1985). Their model predicts the occurrence of the experimentally observed oscillatory activity and also predicts a relative refractoriness for the network involved in such oscillations. This would appear to be consistent with the prediction of an inhibitory role for similar oscillations in our attention paradigms. However, if the alpha mechanisms that we reported in Foxe et al. (1998) were having their effect at the level of the thalamus, one would expect that such effects would be evident in the initial sensory response to the S2 stimulus. This was not the case though, suggesting that at least in that paradigm, putative oscillatory-inhibitory mechanisms may have been operating at the cortico-cortical level. The level of the hierarchy at which putative alpha inhibitory mechanisms will have their effect is likely to be task specific. This contention is supported by our findings of retinotopically specific alpha modulations in a visuo-spatial paradigm (Worden et al., 2000).
It should be mentioned, however, that a failure to observe effects of attention in the initial sensory response to the S2 might reflect type II error. The ability to detect effects at the scalp using EEG is intimately tied to the position and orientation of the cortical areas involved. When such areas are small and discrete, as in early stages of sensory processing, the likelihood of stimulating an appropriately oriented patch of cortex is relatively small when compared to the larger areas of coordinated activity characteristic of higher-order processes. In one experiment (Kelly et al., 2008), we presented visual stimuli systematically across different locations in the visual field for each participant and determined which stimulus position resulted in the largest C1 component (the first cortically evoked component of the visual-evoked potential, which reflects early V1 activation) separately for each individual. This “ideal” stimulus location was then used for a spatial selective attention task, highly similar to that used by Worden et al. (2000). Thus, each participant received a “customized” stimulus set designed to maximize the ability to detect effects in the initial afferent activity in V1. Using this design, which takes into account individual anatomical differences, we were able to detect modulation of the initial C1 component by spatial attention, consistent with modulation of the initial afferent volley in V1. Thus, absence of an observed effect during the initial sensory response cannot rule out a gating effect at the level of the thalamus.
It has been shown, but again in anesthetized animals, that the receptive field properties of V1 vary significantly in conjunction with changes in the level of synchronous activity in the cortico-thalamic visual system (Wörgötter et al., 1998; see also Li et al., 1999). These authors proposed that the high level of inhibition of lateral geniculate nucleus (LGN) cells by cells of the PGN that occurs during synchronous EEG states leads to bursting activity in LGN which results in larger and less specific V1 receptive fields. Alternately, when inhibition is relieved during asynchronous EEG activity, the LGN neurons are placed in a “tonic transmission mode” which sharpens the receptive fields. The authors go on to suggest behavioral significance for this process in that the animal’s behavioral state could result in an adaptation of the spatial resolution of primary sensory transmission by means of such oscillatory mechanisms. This speculation is in keeping with the models proposed by Foxe et al. (1998) and Worden et al. (2000) with the renewed caveat that the alpha modulations that we have reported and the oscillations that Wörgötter records in a sleeping animal may not rely on the same inhibitory mechanisms. Another possibility raised by this finding is that the alpha effects reported in the Foxe and Worden studies did indeed have an effect at the level of the thalamus, causing a change in the receptive field characteristics in a similar fashion to those reported by Wörgötter. This might explain why it is notoriously difficult to establish effects upon the initial processing of the S2’s in such designs using ERPs, since it would be unlikely that surface ERPs would be sensitive to changes in the size of receptive fields.
Cortico-Cortical Contributions
Capotosto et al. (2009) used concurrent EEG and TMS to demonstrate the involvement of frontal eye field (FEF) and intraparietal sulcus (IPS) structures in the attentional effects of alpha-band activity. Human participants performed a demanding visuo-spatial cued attention task similar to that of Worden et al. (2000), with the notable difference that participants were required to respond to all targets, including the relatively infrequent cases when targets were presented opposite to the cued location (i.e., invalidly cued). That is, all S2’s were potentially relevant, and there were no distracters in the paradigm. At the onset of the cue for each trial the researchers applied TMS in one of four ways: (1) to the right IPS, (2) to the right FEF, (3) to the right Pre-central gyrus, and (4) sham TMS near the scalp vertex. It should be emphasized that the researchers did not stimulate visual cortex directly, but rather targeted association areas implicated in theoretical attention networks (e.g., LaBerge 1997; Foxe et al., 2003). Following sham TMS, a bilateral decrease in alpha power over posterior scalp was typically observed. This decrease was greater contralateral to the cued direction. Anticipatory increases ipsilateral to the cue direction were not observed as in Worden et al. (2000), which is likely due to the potential relevance of both hemifields and lack of potential distracters as described above. That is, no region of visual space would be deserving of attentional suppression in this context. When TMS was applied to IPS or FEF (but not pre-central gyrus) such anticipatory decreases in alpha activity were reduced. In the case of IPS stimulation, in fact, anticipatory increases were seen. Critically, the researchers found that the degree of disruption by TMS of the typical anticipatory alpha decreases was related to the participants’ speed of responding to targets. These results strongly implicate the involvement of a fronto-parietal attention control network in the deployment of alpha-mediated gating mechanisms in visual cortex.
Bollimunta et al. (2008) examined the laminar profiles of alpha activity in cortex of alert monkeys performing an auditory discrimination task. By using a linear array of 14 electrodes, the researchers were able to record differential voltages across the approximately 2 mm laminar structure of the cortical mantle. These voltages could then be used to infer the locations of current sources and sinks within a cortical column. Such recordings were made from areas V2, V4, and IT. Supported by Granger causality analysis, the researchers concluded that in areas V2 and V4, infragranular layers serve as the local pacemakers for the alpha rhythm, with a small mediating influence from the granular layer. This laminar pattern suggests a primary influence of cortico-cortical feedback projections in the initiation of the rhythm with a modulatory input from thalamus, as descending cortico-cortical pathways typically terminate in extragranular layers (cf. Felleman and Van Essen, 1991), whereas thalamic projections to posterior cortex typically terminate in the granular layer (e.g., Jacobson and Trojanowski, 1975; Spatz, 1979). In contrast, alpha generation in area IT seemed confined to extragranular layers, with supergranular layers serving as the local pacemaker –a pattern that again implicates a primarily cortico-cortical influence.
Bollimunta et al. (2008) also examined the relationship between the amplitude of the alpha-band activity under investigation in each of the observed areas and the animals’ performance on the auditory task. Areas V2 and V4 showed strong negative correlations between alpha-band power and task performance, consistent with the hypothesis that alpha reflects suppression of competing visual information in these areas and consistent with the findings of the intersensory attention studies of Foxe et al. (1998) and Fu et al. (2001). Interestingly, area IT revealed a strong positive correlation between alpha power and task performance. The authors discussed that this could suggest a differential role of alpha in high- versus low-order processing areas. One thing that seems clear is that alpha activity is far from a unitary phenomenon, and discussions of alpha must take into account the anatomical and behavioral context.
Variants of Alpha Waves
As alluded to above, much of what we suppose to know about the cellular physiology and indeed the visual areas that might underlie the alpha rhythm comes from investigations conducted in sleeping and often anesthetized animals on the alpha-like ∼10 Hz sleep-spindle (e.g., Jahnsen and Llinás, 1984; Steriade and Deschênes, 1984). The extent to which sleep-spindles in an animal are generated by the same processes that generate the alpha rhythm in an awake human is really a matter of speculation but many authors have been willing to accept correspondence (e.g., Williamson et al., 1997). Steriade et al. (1990) go on to point out that although the frequencies are essentially the same, the timecourse of the wave groupings are quite distinct with alpha manifesting in long trains, in contrast to the short bursts that constitute the sleep-spindle. Further, the topographies of each differ extensively. Nonetheless, since some correspondence has been assumed, we will describe below some of the currently available knowledge regarding the neural mechanisms of the sleep-spindle. However, in the absence of any direct evidence for a correspondence between the mechanisms of sleep-spindles ∼10 Hz oscillations and waking human alpha, we believe that we must treat of these putative correspondences with the appropriate level of caution until such time as direct evidence is available.
The sleep-spindles themselves have been extensively studied and well characterized. For example, it has been shown that spindle oscillations are strongly controlled by intracortical inhibitory mechanisms and that shifts in this cortical inhibition can shift the oscillations to spike-wave desynchronous patterns (Contreras et al., 1997). These results were derived from computational modeling procedures, as the alternative of applying anti-inhibitory agents (GABAA-antagonists) to cortex results in epileptiform discharges. The authors stress the large-scale recruitment of inhibitory neurons in cortex by the ascending thalamo-cortical fibers. Thus the activity of cortical pyramidal neurons is dominated by inhibitory inputs and leads to a low-level of pyramidal cell discharge during the oscillatory mode, a putative gating mechanism. Spindle oscillations have also been shown to be governed by inhibitory inputs from nucleus reticularis (Steriade et al., 1985; Destexhe et al., 1998). Separating thalamic relay nuclei from their reticular inputs caused a loss of the spindle oscillations in these nuclei (Steriade et al., 1985). In contrast, the separated reticular nucleus maintains its rhythmic activity and is implicated as a pacemaker for oscillatory activity in the thalamic relay nuclei.
Some evidence specific to alpha rhythms in awake animals does exist, the earliest of which was seen in the dog. Evidence that cortico-cortical alpha oscillatory activity is at least partially independent of the thalamo-cortical alpha process was shown (Lopes da Silva et al., 1980; Lopes da Silva, 1991). The statistical technique of partialization was used to estimate the portion of measured intracortical coherence that could be predicted by concurrent thalamo-cortical coherence. Measuring alpha from one thalamic and two cortical sites in a dog, these authors first computed a cross power spectra between the thalamic recording and each of the two cortical recordings. Linear least-square predictions allowed them to derive a partial coherence measure by eliminating the part of the two cortical signals that could be predicted by the thalamic signal. Unfortunately, the use of a linear model does not in itself determine that cortico-cortical alpha can exist in isolation or that there is, as these authors claim, an independent component as it assumes that there is a linear relationship between the thalamo-cortical and cortico-cortical components of alpha. This may not be a reasonable assumption. Nonetheless, it does at least show that the cortico-cortical part of the alpha process has added non-linear dynamics. Of importance was an added finding that pulvinar was a much larger contributor to the cortical alpha coherence than was LGN, since pulvinar has been theorized to play an important role in selective attention (e.g., Petersen et al., 1987; Robinson, 1993).
It seems plausible that while “arousal” alpha might have a large thalamic component, the alpha that is specifically modulated during selective attention tasks might rely more heavily on the cortico-cortical component of this system. Closing the eyes disengages the visual system in the obvious way, and when straining to hear a faint sound we often close our eyes in order to concentrate effort on the task. However, the alpha modulations reported by Foxe et al. (1998) and Worden et al. (2000), and by many others since, were recorded during very difficult attentional tasks in which subjects were in a highly aroused state with their eyes open. How the general alpha rhythm, known to all electrophysiologists and normally associated with arousal state of the subject and these very specific modulations of 8–14 Hz oscillatory activity recorded during selective attention paradigms are related will take more studies to elucidate. As to the neural mechanisms of the attention sensitive alpha, it will fall largely to intracranial investigations in the awake behaving monkey to further resolve this puzzle.
Conclusion
We have described evidence for alpha-band oscillations as a mechanism for attentionally mediated selective suppression of distracting information. Alpha-band power has been shown to increase over cortical areas responsible for processing potentially distracting information. These attention-related sustained focal increases in alpha power occur prior to the arrival of an anticipated stimulus. Such alpha-band effects have been observed across a variety of selective attention tasks, including intersensory attention, spatial attention in the visual, auditory, and somatosensory domains, and visual feature-based attention. Beyond their robust occurrence during attention tasks, the state of alpha-band brain activity has been shown to predict visual cortical excitability and perceptual outcome. Specifically, higher alpha power indicates lower cortical excitability and higher perceptual thresholds. This is true for both naturally occurring and experimentally induced alpha-band activity in visual cortex.
We have also discussed the anatomical basis for alpha oscillations. The strongest evidence from human TMS studies and monkey intracranial recordings indicates that attention-related alpha modulations are predominately under the control of a cortico-cortical network involving frontal and parietal regions, with a possible secondary influence from thalamo-cortical connections. This is in contrast to arousal-related alpha, which is more likely thalamus-dominated, and is potentially a functionally distinct mechanism from the alpha-band modulations observed in selective attention.
Conflict of Interest Statement
The authors declare that the research was conducted in the absence of any commercial or financial relationships that could be construed as a potential conflict of interest.
The authors declare that the research was conducted in the absence of any commercial or financial relationships that could be construed as a potential conflict of interest.
References
Adrian, E. D., and Matthews, B. H. C. (1934). The Berger rhythm: potential changes from the occipital lobes in man. Brain 57, 355–385.
Ahlsén, G., Lindström, S., and Lo, F. S. (1985). Interaction between inhibitory pathways to principal cells in the lateral geniculate nucleus of the cat. Exp. Brain Res. 58, 134–143.
Armstrong, C. L., Hayes, K. M., and Martin, R. (2001). Neurocognitive problems in attention deficit disorder. Ann. N. Y. Acad. Sci. 931, 196–215.
Banerjee, S., Molholm, S., Snyder, A. C., and Foxe, J. J. (in press). Oscillatory alpha-band mechanisms and the deployment of spatial attention to anticipated auditory and visual target locations: common or separable control mechanisms? J. Neurosci.
Berger, H. (1929). Über das Elektroenkephalogramm des Menschen. Arch. Psychiatr. Nervenkr. 87, 527–570.
Bollimunta, A., Chen, Y., Schroeder, C. E., and Ding, M. (2008). Neuronal mechanisms of cortical alpha oscillations in awake-behaving macaques. J. Neurosci. 28, 9976–9988.
Brandt, M. E. (1997). Visual and auditory evoked phase resetting of the alpha EEG. Int. J. Psychophysiol. 26, 285–298.
Brandt, M. E., and Jansen, B. H. (1991). The relationship between prestimulus-alpha amplitude and visual evoked potential amplitude. Int. J. Neurosci. 61, 261–268.
Brandt, M. E., Jansen, B. H., and Carbonari, J. P. (1991). Pre-stimulus spectral EEG patterns and the visual evoked response. Electroencephalogr. Clin. Neurophysiol. 80, 16–20.
Capotosto, P., Babiloni, C., Romani, G. L., and Corbetta, M. (2009). Frontoparietal cortex controls spatial attention through modulation of anticipatory alpha rhythms. J. Neurosci. 29, 5863–5872.
Contreras, D., Destexhe, A., Sejnowski, T. J., and Steriade, M. (1996). Control of spatiotemporal coherence of a thalamic oscillation by corticothalamic feedback. Science 274, 771–774.
Contreras, D., Destexhe, A., and Steriade, M. (1997). Intracellular and computational characterization of the intracortical inhibitory control of synchronized thalamic inputs in vivo. J. Neurophysiol. 78, 335–350.
Corbetta, M., Miezin, F. M., Dobmeyer, S., Shulman, G. L., and Petersen, S. E. (1991). Selective and divided attention during visual discriminations of shape, color, and speed: functional anatomy by positron emission tomography. J. Neurosci. 11, 2383–2402.
Corbetta, M., Miezin, F. M., Shulman, G. L., and Petersen, S. E. (1993). A PET study of visuospatial attention. J. Neurosci. 13, 1202–1226.
Cosmelli, D., López, V., Lachaux, J.-P., López-Calderón, J., Renault, B., Martinerie, J., and Aboitiz, F. (2011). Shifting visual attention away from fixation is specifically associated with alpha band activity over ipsilateral parietal regions. Psychophysiology 48, 312–322.
Desimone, R., and Duncan, J. (1995). Neural mechanisms of selective visual attention. Annu. Rev. Neurosci. 18, 193–222.
Destexhe, A., Contreras, D., and Steriade, M. (1998). Mechanisms underlying the synchronizing action of corticothalamic feedback through inhibition of thalamic relay cells. J. Neurophysiol. 79, 999–1016.
Dockree, P. M., Kelly, S. P., Foxe, J. J., Reilly, R. B., and Robertson, I. H. (2007). Optimal sustained attention is linked to the spectral content of background EEG activity: greater ongoing tonic alpha (∼10 Hz) power supports successful phasic goal activation. Eur. J. Neurosci. 25, 900–907.
Dufour, A. (1999). Importance of attentional mechanisms in audiovisual links. Exp. Brain Res. 126, 215–222.
Egner, T., Monti, J. M. P., Trittschuh, E. H., Wieneke, C. A., Hirsch, J., and Mesulam, M.-M. (2008). Neural integration of top-down spatial and feature-based information in visual search. J. Neurosci. 28, 6141–6151.
Eimer, M. (1999). Can attention be directed to opposite locations in different modalities? An ERP study. Clin. Neurophysiol. 110, 1252–1259.
Eimer, M., and Schröger, E. (1998). ERP effects of intermodal attention and cross-modal links in spatial attention. Psychophysiology 35, 313–327.
Farah, M. J., Wong, A. B., Monheit, M. A., and Morrow, L. A. (1989). Parietal lobe mechanisms of spatial attention: modality-specific or supramodal? Neuropsychologia 27, 461–470.
Felleman, D. J., and Van Essen, D. C. (1991). Distributed hierarchical processing in the primate cerebral cortex. Cereb. Cortex 1, 1–47.
Foxe, J. J., McCourt, M. E., and Javitt, D. C. (2003). Right hemisphere control of visuospatial attention: line-bisection judgments evaluated with high-density electrical mapping and source analysis. Neuroimage 19, 710–726.
Foxe, J. J., Simpson, G. V., and Ahlfors, S. P. (1998). Parieto-occipital approximately 10 Hz activity reflects anticipatory state of visual attention mechanisms. Neuroreport 9, 3929–3933.
Fries, P., Reynolds, J. H., Rorie, A. E., and Desimone, R. (2001). Modulation of oscillatory neuronal synchronization by selective visual attention. Science 291, 1560–1563.
Fu, K. G., Foxe, J. J., Murray, M. M., Higgins, B. A., Javitt, D. C., and Schroeder, C. E. (2001). Attention-dependent suppression of distracter visual input can be cross-modally cued as indexed by anticipatory parieto-occipital alpha-band oscillations. Brain Res. Cogn. Brain Res. 12, 145–152.
Gould, I. C., Rushworth, M. F., and Nobre, A. C. (2011). Indexing the graded allocation of visuospatial attention using anticipatory alpha oscillations. J. Neurophysiol. 105, 1318–1326.
Gruber, T., MÜller, M. M., Keil, A., and Elbert, T. (1999). Selective visual-spatial attention alters induced gamma band responses in the human EEG. Clin. Neurophysiol. 110, 2074–2085.
Haenny, P. E., and Schiller, P. H. (1988). State dependent activity in monkey visual cortex. Exp. Brain Res. 69, 225–244.
Hanslmayr, S., Aslan, A., Staudigl, T., Klimesch, W., Herrmann, C. S., and Bäuml, K.-H. (2007). Prestimulus oscillations predict visual perception performance between and within subjects. Neuroimage 37, 1465–1473.
Hillyard, S. A., and Anllo-Vento, L. (1998). Event-related brain potentials in the study of visual selective attention. Proc. Natl. Acad. Sci. U.S.A. 95, 781–787.
Ivanoff, J., and Saoud, W. (2009). Nonattentional effects of nonpredictive central cues. Atten. Percept. Psychophys. 71, 872–880.
Jacobson, S., and Trojanowski, J. Q. (1975). Corticothalamic neurons and thalamocortical terminal fields: an investigation in rat using horseradish peroxidase and autoradiography. Brain Res. 85, 385–401.
Jahnsen, H., and Llinás, R. (1984). Ionic basis for the electro-responsiveness and oscillatory properties of guinea-pig thalamic neurons in vitro. J. Physiol. 349, 227–247.
Jones, S. R., Kerr, C. E., Wan, Q., Pritchett, D. L., Hämäläinen, M., and Moore, C. I. (2010). Cued spatial attention drives functionally relevant modulation of the mu rhythm in primary somatosensory cortex. J. Neurosci. 30, 13760–13765.
Kelly, S. P., Gomez-Ramirez, M., and Foxe, J. J. (2008). Spatial attention modulates initial afferent activity in human primary visual cortex. Cereb. Cortex 18, 2629–2636.
Kelly, S. P., Gomez-Ramirez, M., and Foxe, J. J. (2009). The strength of anticipatory biasing predicts target discrimination at attended locations: a high-density EEG study. Eur. J. Neurosci. 31, 2224–2234.
Kelly, S. P., Lalor, E. C., Reilly, R. B., and Foxe, J. J. (2006). Increases in alpha oscillatory power reflect an active retinotopic mechanism for distracter suppression during sustained visuospatial attention. J. Neurophysiol. 95, 3844–3851.
Kerlin, J. R., Shahin, A. J., and Miller, L. M. (2010). Attentional gain control of ongoing cortical speech representations in a “cocktail party.” J. Neurosci. 30, 620–628.
Klimesch, W., Doppelmayr, M., Russegger, H., Pachinger, T., and Schwaiger, J. (1998). Induced alpha band power changes in the human EEG and attention. Neurosci. Lett. 244, 73–76.
Li, B., Funke, K., Wörgötter, F., and Eysel, U. T. (1999). Correlated variations in EEG pattern and visual responsiveness of cat lateral geniculate relay cells. J. Physiol. 514, 857–874.
Lindgren, K. A., Larson, C. L., Schaefer, S. M., Abercrombie, H. C., Ward, R. T., Oakes, T. R., Holden, J. E., Perlman, S. B., Benca, R. M., and Davidson, R. J. (1999). Thalamic metabolic rate predicts EEG alpha power in healthy control subjects but not in depressed patients. Biol. Psychiatry 45, 943–952.
Lopes da Silva, F. H. (1991). Neural mechanisms underlying brain waves: from neural membranes to networks. Electroencephalogr. Clin. Neurophysiol. 79, 81–93.
Lopes da Silva, F. H., Vos, J. E., Mooibroek, J., and Van Rotterdam, A. (1980). Relative contributions of intracortical and thalamo-cortical processes in the generation of alpha rhythms, revealed by partial coherence analysis. Electroencephalogr. Clin. Neurophysiol. 50, 449–456.
Luck, S. J., Chelazzi, L., Hillyard, S. A., and Desimone, R. (1997). Neural mechanisms of spatial selective attention in areas V1, V2, and V4 of macaque visual cortex. J. Neurophysiol. 77, 24–42.
Markram, K., and Markram, H. (2010). The intense world theory – a unifying theory of the neurobiology of autism. Front. Hum. Neurosci. 4:224. doi: 10.3389/fnhum.2010.00224
Martinez-Trujillo, J. C., and Treue, S. (2004). Feature-based attention increases the selectivity of population responses in primate visual cortex. Curr. Biol. 14, 744–751.
Mathewson, K. E., Gratton, G., Fabiani, M., Beck, D. M., and Ro, T. (2009). To see or not to see: prestimulus alpha phase predicts visual awareness. J. Neurosci. 29, 2725–2732.
Moran, J., and Desimone, R. (1985). Selective attention gates visual processing in the extrastriate cortex. Science 229, 782–784.
Morgan, M. J., Ward, R. M., and Castet, E. (1998). Visual search for a tilted target: tests of spatial uncertainty models. J. Exp. Psychol. A 51, 347–370.
Most, S. B., and Astur, R. S. (2007). Feature-based attentional set as a cause of traffic accidents. Vis. Cogn. 75, 125–132.
Motter, B. C. (1993). Focal attention produces spatially selective processing in visual cortical areas V1, V2, and V4 in the presence of competing stimuli. J. Neurophysiol. 70, 909–919.
Mulholland, T. B. (1965). Occurrence of the electroencephalographic alpha rhythm with eyes open. Nature 206, 746.
Neely, J. H. (1977). Semantic priming and retrieval from lexical memory: roles of inhibitionless spreading activation and limited-capacity attention. J. Exp. Psychol. 106, 226–254.
O’Connell, R. G., Dockree, P. M., Robertson, I. H., Bellgrove, M. A., Foxe, J. J., and Kelly, S. P. (2009). Uncovering the neural signature of lapsing attention: electrophysiological signals predict errors up to 20 s before they occur. J. Neurosci. 29, 8604–8611.
Perrott, D. R., Cisneros, J., McKinley, R. L., and D’Angelo, W. R. (1996). Aurally aided visual search under virtual and free-field listening conditions. Hum. Factors 38, 702–715.
Perrott, D. R., Saberi, K., Brown, K., and Strybel, T. Z. (1990). Auditory psychomotor coordination and visual search performance. Percept. Psychophys. 48, 214–226.
Petersen, S. E., Robinson, D. L., and Morris, J. D. (1987). Contributions of the pulvinar to visual spatial attention. Neuropsychologia 25, 97–105.
Pfurtscheller, G. (1992). Event-related synchronization (ERS): an electrophysiological correlate of cortical areas at rest. Electroencephalogr. Clin. Neurophysiol. 83, 62–69.
Pfurtscheller, G., and Aranibar, A. (1977). Event-related cortical desynchronization detected by power measurements of scalp EEG. Electroencephalogr. Clin. Neurophysiol. 42, 817–826.
Posner, M. I., and Petersen, S. E. (1990). The attention system of the human brain. Annu. Rev. Neurosci. 13, 25–42.
Posner, M. I., Snyder, C. R., and Davidson, B. J. (1980). Attention and the detection of signals. J. Exp. Psychol. 109, 160–174.
Posner, M. I., Walker, J. A., Friedrich, F. J., and Rafal, R. D. (1984). Effects of parietal injury on covert orienting of attention. J. Neurosci. 4, 1863–1874.
Rahn, E., and Başar, E. (1993a). Prestimulus EEG-activity strongly influences the auditory evoked vertex response: a new method for selective averaging. Int. J. Neurosci. 69, 207–220.
Rahn, R., and Başar, E. (1993b). Enhancement of visual evoked potentials by stimulation during low prestimulus EEG stages. Int. J. Neurosci. 72, 123–136.
Reynolds, J. H., Chelazzi, L., and Desimone, R. (1999). Competitive mechanisms subserve attention in macaque areas V2 and V4. J. Neurosci. 19, 1736–1753.
Rihs, T. A., Michel, C. M., and Thut, G. (2007). Mechanisms of selective inhibition in visual spatial attention are indexed by α-band EEG synchronization. Eur. J. Neurosci. 25, 603–610.
Rihs, T. A., Michel, C. M., and Thut, G. (2009). A bias for posterior alpha-band power suppression versus enhancement during shifting versus maintenance of attention. Neuroimage 44, 190–199.
Robinson, D. L. (1993). Functional contributions of the primate pulvinar. Prog. Brain Res. 95, 371–380.
Romei, V., Gross, J., and Thut, G. (2010). On the role of prestimulus alpha rhythms over occipito-parietal areas in visual input regulation: correlation or causation? J. Neurosci. 30, 8692–8697.
Romei, V., Rihs, T., Brodbeck, V., and Thut, G. (2008a). Resting electroencephalogram alpha-power over posterior sites indexes baseline visual cortex excitability. Neuroreport 19, 203–208.
Romei, V., Brodbeck, V., Michel, C., Amedi, A., Pascual-Leone, A., and Thut, G. (2008b). Spontaneous fluctuations in posterior alpha-band EEG activity reflect variability in excitability of human visual areas. Cereb. Cortex 18, 2010–2018.
Sauseng, P., Klimesch, W., Stadler, W., Schabus, M., Doppelmayr, M., Hanslmayr, S., Gruber, W. R., and Birbaumer, N. (2005). A shift of visual spatial attention is selectively associated with human EEG alpha activity. Eur. J. Neurosci. 22, 2917–2926.
Schneider, W., and Fisk, A. D. (1982). Concurrent automatic and controlled visual search: can processing occur without resource cost? J. Exp. Psychol. Learn. Mem. Cogn. 8, 261–278.
Schneider, W., and Shiffrin, R. M. (1977). Controlled and automatic human information processing: I. Detection, search, and attention. Psychol. Rev. 84, 1–66.
Shibata, T., Shimoyama, I., Ito, T., Abla, D., Iwasa, H., Koseki, K., Yamanouchi, N., Sato, T., and Nakajima, Y. (1999). Attention changes the peak latency of the visual gamma-band oscillation of the EEG. Neuroreport 10, 1167–1170.
Shiffrin, R. M., and Schneider, W. (1977). Controlled and automatic human information processing: II. Perceptual learning, automatic attending and a general theory. Psychol. Rev. 84, 127–190.
Singer, W. (1993). Synchronization of cortical activity and its putative role in information processing and learning. Annu. Rev. Physiol. 55, 349–374.
Singer, W. (1999). Neuronal synchrony: a versatile code for the definition of relations? Neuron 24, 49–65, 111–125.
Snyder, A. C., and Foxe, J. J. (2010). Anticipatory attentional suppression of visual features indexed by oscillatory alpha-band power increases: a high-density electrical mapping study. J. Neurosci. 30, 4024–4032.
Sokolov, A., Lutzenberger, W., Marina, P., Hubert, P., Braun, C., and Birbaumer, N. (1999). Gamma-band MEG activity to coherent motion depends on task-driven attention. Neuroreport 10, 1997–2000.
Spatz, W. B. (1979). The retino-geniculo-cortical pathway in Callithrix. II. The geniculo-cortical projection. Exp. Brain Res. 36, 401–410.
Spence, C., and Driver, J. (1996). Audiovisual links in endogenous covert spatial attention. J. Exp. Psychol. Hum. Percept. Perform. 22, 1005–1030.
Spence, C., and Driver, J. (1997a). Audiovisual links in exogenous covert spatial orienting. Percept. Psychophys. 59, 1–22.
Spence, C., and Driver, J. (1997b). On measuring selective attention to an expected sensory modality. Percept. Psychophys. 59, 389–403.
Steriade, M., and Deschênes, M. (1984). The thalamus as a neuronal oscillator. Brain Res. 320, 1–63.
Steriade, M., Deschênes, M., Domich, L., and Mulle, C. (1985). Abolition of spindle oscillations in thalamic neurons disconnected from nucleus reticularis thalami. J. Neurophysiol. 54, 1473–1497.
Steriade, M., Gloor, P., Llinás, R. R., Lopes de Silva, F. H., and Mesulam, M. M. (1990). Report of IFCN committee on basic mechanisms. Basic mechanisms of cerebral rhythmic activities. Electroencephalogr. Clin. Neurophysiol. 76, 481–508.
Thut, G., Nietzel, A., and Pascual-Leone, A. (2006). Differential distribution of alpha-band electroencephalographic (EEG) activity over occipital cortex indexes visuo-spatial attention bias and predicts visual target detection. J. Neurosci. 26, 9494–9502.
Ungerleider, L. G., and Mishkin, M. (1982). “Two cortical visual systems,” in Analysis of Visual Behavior, eds D. J. Ingle, M. A. Goodale, and R. J. W. Mansfield (Cambridge, MA: MIT Press), 549–586.
Van Dijk, H., Schoffelen, J. M., Oostenveld, R., and Jensen, O. (2008). Prestimulus oscillatory activity in the alpha band predicts visual discrimination ability. J. Neurosci. 28, 1816–1823.
Van Ede, F., de Lange, F., Jensen, O., and Maris, E. (2011). Orienting attention to an upcoming tactile event involves a spatially and temporally specific modulation of sensorimotor alpha- and beta-band oscillations. J. Neurosci. 31, 2016–2024.
Vanduffel, W., Tootell, R. B., and Orban, G. A. (2000). Attention-dependent suppression of metabolic activity in the early stages of the macaque visual system. Cereb. Cortex 10, 109–126.
Vanni, S., Revonsuo, A., and Hari, R. (1997). Modulation of the parieto-occipital alpha rhythm during object detection. J. Neruosci. 17, 7141–7147.
Williamson, S. J., Kaufman, L., Lu, Z. L., Wang, J. Z., and Karron, D. (1997). Study of human occipital alpha rhythm: the alphon hypothesis and alpha suppression. Int. J. Psychophysiol. 26, 63–76.
Worden, M. S., Foxe, J. J., Wang, N., and Simpson, G. V. (2000). Anticipatory biasing of visuospatial attention indexed by retinotopically specific α-band electroencephalography increases over occipital cortex. J. Neurosci. 20, 1–6.
Wörgötter, F., Suder, K., Zhao, Y., Kerscher, N., Eysel, U. T., and Funke, K. (1998). State-dependent receptive-field restructuring in the visual cortex. Nature 396, 165–168.
Wylie, G. R., Javitt, D. C., and Foxe, J. J. (2004). Don’t think of a white bear: an fMRI investigation of the effects of sequential instructional sets on cortical activity in a task-switching paradigm. Hum. Brain Mapp. 21, 279–297.
Wylie, G. R., Javitt, D. C., and Foxe, J. J. (2006). Jumping the gun: is effective preparation contingent upon anticipatory activation in task-relevant neural circuitry? Cereb. Cortex 16, 394–404.
Keywords: alpha, brain, oscillations, selection, attention, biasing, suppression, EEG
Citation: Foxe JJ and Snyder AC (2011) The role of alpha-band brain oscillations as a sensory suppression mechanism during selective attention. Front. Psychology 2:154. doi: 10.3389/fpsyg.2011.00154
Received: 15 March 2011;
Accepted: 21 June 2011;
Published online: 05 July 2011.
Edited by:
Gregor Thut, University of Glasgow, UKReviewed by:
Gregor Thut, University of Glasgow, UKCopyright: © 2011 Foxe and Snyder. This is an open-access article subject to a non-exclusive license between the authors and Frontiers Media SA, which permits use, distribution and reproduction in other forums, provided the original authors and source are credited and other Frontiers conditions are complied with.
*Correspondence: John J. Foxe, Cognitive Neurophysiology Laboratory, Children’s Evaluation and Rehabilitation Center, Departments of Pediatrics and Neuroscience, Albert Einstein College of Medicine, 1225 Morris Park Avenue, Suite 1-C Bronx, NY 10461 USA. e-mail:am9obi5mb3hlQGVpbnN0ZWluLnl1LmVkdQ==