- 1 Department of Psychiatry, University of Wisconsin–Madison, Madison, WI, USA
- 2 Department of Psychology, University of Wisconsin–Madison, Madison, WI, USA
- 3 Max Plank Institute for Psycholinguistics, Radboud University Nijmegen, Nijmegen, Netherlands
- 4 Department of Psychology, Princeton University, Princeton, NJ, USA
Studies exploring the role of neural oscillations in cognition have revealed sustained increases in alpha-band (~8–14 Hz) power during the delay period of delayed-recognition short-term memory tasks. These increases have been proposed to reflect the inhibition, for example, of cortical areas representing task-irrelevant information, or of potentially interfering representations from previous trials. Another possibility, however, is that elevated delay-period alpha-band power (DPABP) reflects the selection and maintenance of information, rather than, or in addition to, the inhibition of task-irrelevant information. In the present study, we explored these possibilities using a delayed-recognition paradigm in which the presence and task relevance of shape information was systematically manipulated across trial blocks and electroencephalographic was used to measure alpha-band power. In the first trial block, participants remembered locations marked by identical black circles. The second block featured the same instructions, but locations were marked by unique shapes. The third block featured the same stimulus presentation as the second, but with pretrial instructions indicating, on a trial-by-trial basis, whether memory for shape or location was required, the other dimension being irrelevant. In the final block, participants remembered the unique pairing of shape and location for each stimulus. Results revealed minimal DPABP in each of the location-memory conditions, whether locations were marked with identical circles or with unique task-irrelevant shapes. In contrast, alpha-band power increases were observed in both the shape-memory condition, in which location was task irrelevant, and in the critical final condition, in which both shape and location were task relevant. These results provide support for the proposal that alpha-band oscillations reflect the retention of shape information and/or shape–location associations in short-term memory.
Introduction
The first published report of human electroencephalographic (EEG) data described elevated oscillations centered at the frequency of 10 Hz over posterior electrode sites, which increased in magnitude when the eyes were closed, and decreased when the eyes were opened (Berger, 1929). Replications and extensions of this finding have led to the widely held view that posterior alpha-band oscillations correspond to an “idling” state of the brain (Pfurtscheller et al., 1996), with higher frequency oscillations (e.g., in the beta- and gamma-band) predominating when the same networks are engaged in, for example, active visual processing. Subsequent studies have revealed alpha-band power modulations in both attention and working memory tasks, suggesting a larger role for alpha-band oscillations in cognition than the idling account would suggest. For instance, several studies have shown that shifts of covert spatial attention are accompanied by increased alpha-band oscillations over posterior sensors ipsilateral to the direction of attention, and decreased alpha contralateral to the focus of attention (see, e.g., Worden et al., 2000; Kelly et al., 2006; Thut et al., 2006). That is, alpha-band power decreases at attended and increases at unattended locations, suggesting a functional inhibitory role for shifts in posterior alpha-band power. Consistent with this possibility, spontaneous fluctuations in occipital alpha-band power have been found to predict cortical excitability, as indexed by the perception of TMS-induced visual phosphenes (Romei et al., 2008). Additionally, the detection of at-threshold visual stimuli is predicted by the pre-stimulus power (Hanslmayr et al., 2007; Van Dijk et al., 2008; Mathewson et al., 2009) and phase angle (Mathewson et al., 2009; Busch and VanRullen, 2010) of spontaneous posterior alpha-band oscillations.
Increased alpha-band power has also been observed throughout the delay period of tasks requiring the short-term retention of visual information (see, e.g., Klimesch et al., 1999; Jensen et al., 2002; Jokisch and Jensen, 2007; Tuladhar et al., 2007; Hamidi et al., 2009). For instance, Klimesch et al. (1999) observed increased posterior delay-period alpha-band power (DPABP) during the retention of unique alpha-numeric strings in working memory. Additionally, Jensen et al. (2002) found that DPABP parametrically increases as a function of memory load during performance of a modified Sternberg (1966) task.
These memory-related alpha-band power increases have been proposed to reflect either functional inhibition (Klimesch et al., 2007), or active processing related to the short-term retention of information in working memory (Palva and Palva, 2007). For example, Klimesch et al. (1999, 2007) has argued that increased DPABP reflects a mechanism of inhibitory top-down control that prevents the retrieval of stimuli that were remembered on previous trials, which could interfere with the encoding and maintenance of new information. Another proposal, emphasized in the work of Jensen and colleagues (Jokisch and Jensen, 2007; Jensen and Mazaheri, 2010), holds that task-specific increases in alpha-band power reflect the functional inhibition of cortical areas representing potentially disruptive task-irrelevant information. For instance, when remembering an object’s shape or color, optimal performance may depend on our ability to ignore task-irrelevant information, such as the object’s location, orientation, or direction of motion. If this were the case, elevated alpha-band power should be present over task-irrelevant cortical areas during the delay period. It is this version of the “alpha inhibition” hypothesis that will be the focus of this report.
In keeping with the idea of filtering out stimulus dimensions that are trial irrelevant, elevated DPABP has been observed over dorsal stream visual areas during the retention of face identities, which engages the ventral stream (Jokisch and Jensen, 2007). Similarly, Hamidi et al. (2009) observed a pronounced sustained posterior increase in DPABP during the retention of abstract shapes in working memory. Additionally, smaller but reliable elevations in DPABP were also observed on trials in which participants were instructed to remember the location, rather than the identity, of each shape. In another study (Grimault et al., 2009), participants were cued to remember stimuli appearing in either the left or right visual field, ignoring stimuli in the opposite visual field (as in Vogel and Machizawa, 2005). In keeping with findings from studies of spatial attention, DPABP increased at parietal electrodes ipsilateral to the remembered hemifield. That is, alpha-band power increased over visual areas representing locations that were to be ignored on that trial.
However, findings from the visual cognition literature raise questions about the functional inhibition interpretation of alpha-band power. First, although it is true that, when given enough time (~1000–1500 ms for location, and 500–1000 ms for features), task-irrelevant changes in object properties can be ignored when they occur at test (Logie et al., 2011), numerous studies exploring memory for object properties, such as shape, color, and orientation, have suggested that when objects are attended, spatial, and non-spatial features are spontaneously integrated in working memory (see, e.g., Jiang et al., 2000; Treisman and Zhang, 2006; Hollingworth and Rasmussen, 2010). Moreover, although changes in task-irrelevant information can have an impact on performance (see, e.g., Jiang et al., 2000), there is no evidence that we are aware of suggesting that the mere presence of task-irrelevant though non-distracting information (e.g., the task-irrelevant shape and location information in the studies described above) adversely affects performance.
Additionally, if alpha power increases reflect a general inhibitory mechanism that suppresses task-irrelevant cortical areas, increased alpha would be expected to be present over task-irrelevant areas whether the primary task is spatial or non-spatial in nature. However, in the experiments of Jokisch and Jensen (2007), alpha increases were observed over the dorsal stream in a face identity task that engaged the ventral stream, but were not seen over the ventral stream during retention of face orientations, which engaged the dorsal stream. Increased alpha was observed in the location-memory condition of Hamidi et al. (2009), but the magnitude of the increase was much lower than observed during shape retention, and our own unpublished observations suggest that these increases are only present when individual locations are marked by unique shapes, being largely absent when locations are marked by uniform circles.
An alternative account of DPABP, therefore, is that instead of, or in addition to, reflecting the inhibition of task-irrelevant information, sustained increases in alpha power observed in working memory tasks may be a critical component of the distributed network activity underlying the selection and maintenance of objects in working memory, as proposed by Palva and Palva (2007). By this view, load dependent increases in alpha-band power reflect increasing demands on attention- and maintenance-related neural systems, rather than inhibition.
In keeping with this possibility memory-load dependent increases in high alpha-, beta-, and gamma-band oscillations have been observed in frontal and parietal regions implicated in attentional and executive aspects of working memory, although the amplitudes of these oscillations were suppressed below baseline levels (Palva et al., 2011). Additionally, a role for alpha-band oscillations in object processing has received support from a recent study examining local field potentials and multi-unit activity in the inferior temporal (IT) cortex of macaques performing an intermodal (visual versus auditory) selective attention task (Mo et al., 2011). On each trial, monkeys received bimodal stimulation, but attention was directed to either visual or auditory information in alternating trial blocks. Results revealed higher pre-stimulus LFP power and multi-unit activity when monkeys attended to visual versus auditory stimuli. Moreover, increased pre-stimulus LFP alpha was predictive of stronger stimulus-evoked responses, suggesting that, in contrast to alpha activity observed in the occipital cortex, alpha oscillations in IT may play a direct role in amplifying task-relevant information.
In the present study, we test the predictions of the inhibitory view of alpha-band oscillations proposed by Jensen and Mazaheri (2010) and those of the object selection and maintenance hypothesis using a delayed-recognition paradigm in which the presence and task relevance of shape information was systematically manipulated across trial blocks and EEG was recorded to measure DPABP. In the first trial block, participants remembered locations marked by identical black circles. The second block featured the same instructions, but locations were marked by unique shapes. The third block consisted of two memory conditions featuring identical stimulus presentation, but with pretrial instructions indicating, on a trial-by-trial basis, whether memory for shape or location was required, the other dimension being irrelevant. In the final block, stimuli once again consisted of unique shapes appearing at distinct locations, as in Blocks 2–3. However, in this block participants were required to remember the unique pairing of shape and location for each stimulus, rather than shape or location alone, as in Block 3. Thus, Blocks 2–4 consisted of identical stimulus presentation, with the only difference being whether memory for location, memory for shape, or memory for shapes in specific locations was required.
If increased DPABP reflects the functional inhibition of task-irrelevant cortical areas, it should be observed in each condition that includes the presence of task-irrelevant information. Thus, DPAPB should be absent in Block 1, in which no unique task-irrelevant information was present to be inhibited. Conversely, alpha-band power should be elevated in Block 2, and in both conditions of Block 3, in which irrelevant shape information was present in the location task, and irrelevant location information was present in the shape task. Finally, DPABP should be reduced in Block 4, in which both shape and location were task relevant, and thus neither should be inhibited.
By contrast, the object selection and maintenance hypothesis makes the following predictions. Elevated DPABP should not be observed in Block 1, because no unique shape information was present. Conversely, DPABP should be elevated in Block 2, and in both conditions of Block 3. However, on the basis of our previous observations (Hamidi et al., 2009), we expect DPAPB to be greater in the shape-memory condition of Block 3, in which shapes were task relevant, than in any of the location-memory conditions, in which shape was present but did not need to be actively maintained. Finally, in contrast to the predictions of the functional inhibition account, DPABP should remain elevated in Block 4, which required the retention of shape–location associations.
Materials and Methods
Participants
Twelve adults [eight female, mean age = 21.58 (SD = 1.38)] were recruited from the University of Wisconsin–Madison community to participate in this study. All participants reported normal or corrected to normal visual acuity. Participants provided informed consent prior to the experiment, and received monetary compensation for their participation.
Stimuli and Procedures
Stimulus presentation and response recording was controlled with a Dell Inspiron laptop computer running E-Prime software (Psychology Software Tools, Inc.,). Stimuli were presented against a gray background on the laptop’s 15″ LCD screen at a viewing distance of approximately 70 cm. Memory targets and probe stimuli consisted of either black dots or black abstract shapes (Attneave and Arnoult, 1956) chosen from a set of 12 difficult-to-name shapes (Vanderplas and Garvin, 1959), depending on the task condition. Both memory targets and probe stimuli subtended ~2.3° of visual angle, although shape stimuli varied somewhat in overall size.
General task layout and timing across each block is depicted in Figure 1A. With the exception of Block 3, which began with task instructions, each trial began with the appearance of a fixation cross at the center of the screen for 300 ms, followed by the sequential presentation of four memory targets, one per quadrant, for 300 ms each, separated by a 300-ms fixation screen. In each screen quadrant, memory targets were presented in 1 of 12 possible positions randomly selected from a 4 × 3 grid positioned within a 9° × 5° screen region centered 9.75° diagonally from fixation (i.e., from the center of the screen). The final memory target was followed by a 3-s delay interval, during which the fixation cross remained at the center of the screen, after which a probe stimulus appeared. The probe stimulus remained visible for 3 s or until the subject made a response by pressing the “v” or “n” key on the laptop keyboard, indicating whether the probe stimulus matched (“v”) or did not match (“n”) the task-relevant attribute(s) of the memory targets on that trial. At the end of the trial, participants received feedback, with the word “Incorrect” appearing for 500 ms at fixation when an incorrect response was made. The feedback screen was followed by a 2-s ITI, and instructions to “press any key to continue” with the next trial.
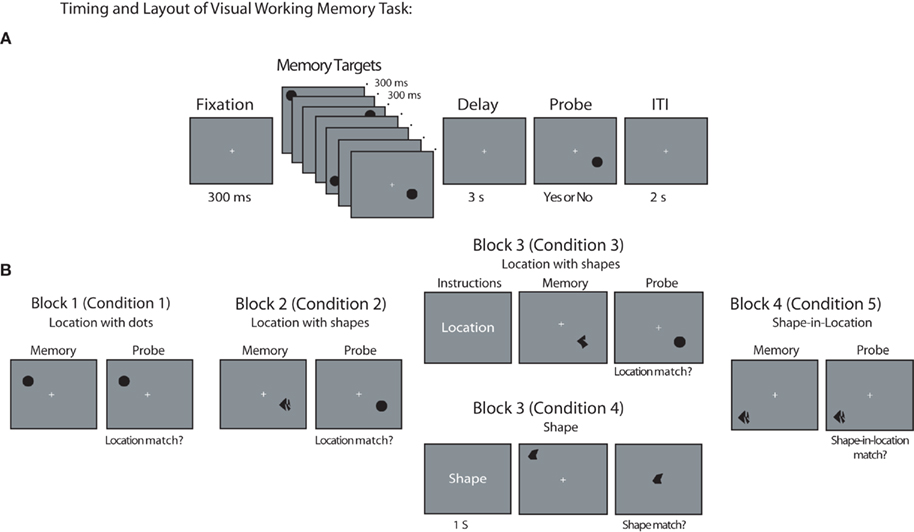
Figure 1. Behavioral task. (A) General timing of fixation, stimuli, delay period, probe, and ITI used during all task blocks of VSTM task. Note that Block 3 also included an additional 1-s presentation of task instructions at the beginning of each trial. (B) Example memory target and probe item for each task block and condition (in each case, a match trial is depicted). Stimuli are not drawn to scale.
Block and condition-specific differences in stimuli and task layout are illustrated in Figure 1B (in each case, a “match” trial is depicted). In Blocks 1–2, participants were instructed to remember the location of each memory target, with locations marked by uniform dots in Block 1 and abstract shapes in Block 2 (participants were informed that the shape of each object was task-irrelevant and could be ignored). For these blocks, the memory probe consisted of a single black dot presented at 1 of the 12 possible target positions within a given quadrant. For non-match trials, the probe was presented at 1 of the 11 screen positions that had not contained a target on that trial. In Block 3, two conditions, requiring either memory for shapes or memory for locations, were randomly intermixed, with equal probability. The location-memory condition was identical to the location-memory condition of Block 2, with the exception that each trial began with an instruction screen indicating that location was to be remembered on that trial. Similarly, each trial in the shape-memory condition of Block 3 began with an instruction screen indicating that the shape of each stimulus should be remembered on that trial. For these trials, the probe stimulus was an abstract shape presented at fixation. On non-match trials, the identity of the shape was chosen randomly from the set of eight shapes that were not presented as memory targets on that trial. Finally, in Block 4, the identity of the probe stimulus always matched the identity of one of the four memory targets presented on that trial. However, on non-match trials, the probe stimulus appeared at a screen location that was occupied by a different stimulus in the original memory display. That is, the probe stimulus changed positions with one of the other memory targets on that trial. Memory targets appearing in each sequential position (i.e., target 1, 2, 3, or 4) were probed equally often.
Task blocks were presented in the same order across participants to explore whether DPABP was sensitive to the task relevance of shape information, or was simply present whenever unique shape information was available in the memory display. Using a fixed block order ensured that each participant’s performance on the first two blocks would not be influenced by having previously performed trials in which stimulus shape was relevant. In this way, any changes observed in Block 2 relative to Block 1 could not be attributed to strategic factors, or to the influence of a previous behavioral set on performance. Participants completed 48 trials in Blocks 1–2 and 4, and 96 trials (48 location and 48 shape trials) in Block 3. There were an equal number of match and non-match trials in each block, and they occurred in an unpredictable order. Prior to each block, participants received written instructions and completed a block of 12 practice trials. The experimental session took ~2.5 h to complete, with ~1 h devoted to task performance.
EEG Recording and Data Preprocessing
The EEG was recorded from 60 Ag/AgCl electrodes mounted in an elastic cap, with electrode positions conforming to the international 10–20 system and a subset of intermediate 10% positions (Sharbrough et al., 1991). Electrode sites were recorded using a reference electrode placed on the forehead, and later algebraically re-referenced to the average of all 60 electrodes offline. The EEG was amplified by an Eximia 60-channel amplifier (Nextim, Helsinki, Finland). Data were acquired at a sampling rate of 1450 Hz, and were band pass filtered (0.1–100 Hz) and down sampled to 500 Hz offline using the EEGlab toolbox (Delorme and Makeig, 2004) running in a MATLAB environment (Mathworks, Natick, MA, USA). Large movement-related artifacts were first removed by visual inspection. Then, in a small number of cases, individual channels with excessive noise were reinterpolated using spherical spline interpolation (Perrin et al., 1989). Additional electrical artifacts due to eye movements, blinks, and 60-Hz channel noise were identified and removed using independent components analysis.
Time–Frequency Analysis
The Matlab toolbox Fieldtrip was used for time–frequency analysis (http://www.ru.nl/neuroimaging/fieldtrip; see Oostenveld et al., 2011). Delay-period oscillatory power across trial blocks/conditions was estimated using a multi-taper estimation method in which a frequency-dependent sliding time window was applied. The length of the window was three cycles/frequency of interest (5–30 Hz, in 1 Hz steps), applied in time-steps of 50 ms throughout the entirety of the 3-s delay interval (±500 ms). The data from each time window were then multiplied by three orthogonal Hanning tapers, Fourier transformed, and the power spectral densities were averaged over trials. Next, spectral estimates for each frequency from 5 to 30 Hz were normalized for each subject on a block-by-block basis by subtracting the mean spectral power estimated from the central 200-ms of the 300-ms fixation window preceding each trial in a given trial block/condition, from each time point in the delay-period time–frequency representation. Because behavioral condition was confounded with block order, normalization was conducted on a block-by-block basis to ensure that our results were not contaminated by task-independent changes in alpha-band power that developed across blocks (see Figure 4).
Statistical Analysis
Behavioral differences in accuracy (% correct) and reaction time (RT) were assessed using one-way repeated-measures ANOVAs, and were followed up with paired-comparison t-tests where necessary. Differences in DPABP across conditions were statistically assessed using a cluster-based permutation test that identifies clusters of sensors with significant changes, while correcting for multiple comparisons over sensors in within-subject comparisons (Maris and Oostenveld, 2007). Clusters were defined as two or more spatially contiguous sensors in which the t-statistics of power values averaged over the 8- to 15-Hz range across the central 500–2500 ms of the delay period exceeded a chosen threshold of p < 0.05. The comparison based on t-statistics identifies sensors with effects exceeding threshold, which are then used for the subsequent cluster-based permutation analysis. Thus, the power values to be tested do not need to be normally distributed. The cluster-level test statistic is defined as the sum of the t-statistics of the sensors in a cluster (i.e., in a group of sensors with effects exceeding a threshold of p = 0.05). The Type-I error rate for the complete set of 60 sensors was controlled using a non-parametric statistical test, which evaluates the cluster-level test statistic under the randomization null distribution of the maximum cluster-level test statistic. The null distribution was obtained by randomly permuting the data between the two experimental conditions for each participant, creating a reference distribution from 500 random sets of permutations. The p-value was estimated as the proportion of the elements in the randomization null distribution exceeding the observed maximum cluster-level test statistic.
Note that, although this non-parametric statistical procedure makes it possible to evaluate conditional data in any combination of time, frequency, and space while controlling for multiple comparisons, in the present case we were specifically interested in changes in mean alpha-band power during the delay interval. Thus, prior to the cluster analysis we reduced our three-dimensional (electrode × time × frequency) data to a single dimension (electrode) by averaging over the 8- to 15-Hz frequency band and a 500- to 2500-ms portion of the delay interval.
Results
Behavior
As shown in Figure 2A, accuracy (% correct) was uniformly high across conditions. Confirming this, a repeated-measures ANOVA revealed no significant main effect of memory condition on accuracy (F < 1). Although subjects were told to emphasize accuracy over speed in each condition, we also analyzed RT (see Figure 2B). In contrast to accuracy, RT steadily decreased (i.e., responses were faster) across Conditions 1–3, returning to the level observed in Condition 1 in Conditions 4–5. The apparent difference in RT across conditions was confirmed by a repeated-measures ANOVA, F(4,40) = 5.11, p = 0.002. Tests of simple effects revealed faster RTs in Conditions 2 and 3 (memory for locations marked with shapes, both when blocked separately and when intermixed) versus Conditions 4 and 5 (shape, and shape-in-location, respectively), all p’s < 0.01. No other comparisons were found to be significant (all p’s > 0.15), with the exception of a trend toward faster performance in Condition 3 versus Condition 1, p = 0.07. The steady decrease in RT in the location conditions over the first three blocks likely reflects learning on the spatial delayed-recognition task. Importantly, however, the finding of no differences in accuracy among conditions, and comparable RTs in conditions one, four, and five suggests that the shape and shape-in-location conditions were not any more demanding than the location-memory task.
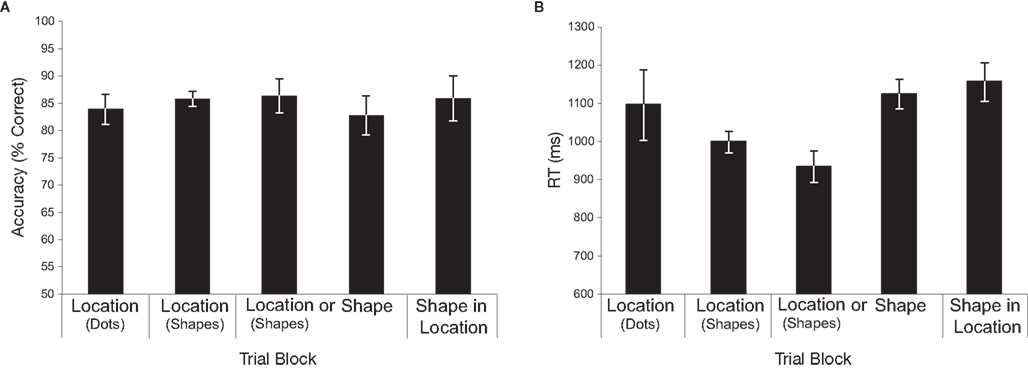
Figure 2. Behavioral data. (A) Repeated-measures ANOVA revealed no significant main effect of memory condition on accuracy (F < 1). (B) A repeated-measures ANOVA revealed a significant difference in RT across conditions [F(4,40) = 5.11, p = 0.002]. Follow up paired comparisons revealed faster RTs in each of the location-with-shapes conditions versus the shape and shape-in-location conditions (all p’s < 0.01). All other comparisons were non-significant. Error bars reflect within-subject confidence intervals (Cousineau, 2007).
Time–Frequency
Grand-averaged time–frequency representations for 11 participants across each trial block/condition are shown in Figure 3. One participant, who exhibited alpha-band power increases >3 SDs above the mean across subjects, was not included in any of the analyses reported here. Figure 3A shows topographic plots of mean power in the 8- to 15-Hz frequency band averaged over a 500- to 2500-ms portion of the delay across each block/condition. As can be seen, posterior DPABP was minimal in Condition 1 (location with dots), and was slightly elevated in Condition 2 (location with shapes, blocked separately) and Condition 3 (location with shapes, intermixed), and was markedly elevated in Condition 4 (shapes, intermixed) and Condition 5 (shape-in-location). Figure 3B shows the time–frequency representation for each condition across the full delay period (±500 ms) in the 5- to 30-Hz frequency range for the cluster of posterior electrodes highlighted in the inset topographical map. This confirms the presence of a sustained power increase throughout the delay interval in a frequency range extending from ~8 to 20 Hz, with a prominent peak in the high-alpha range (~10–12 Hz).
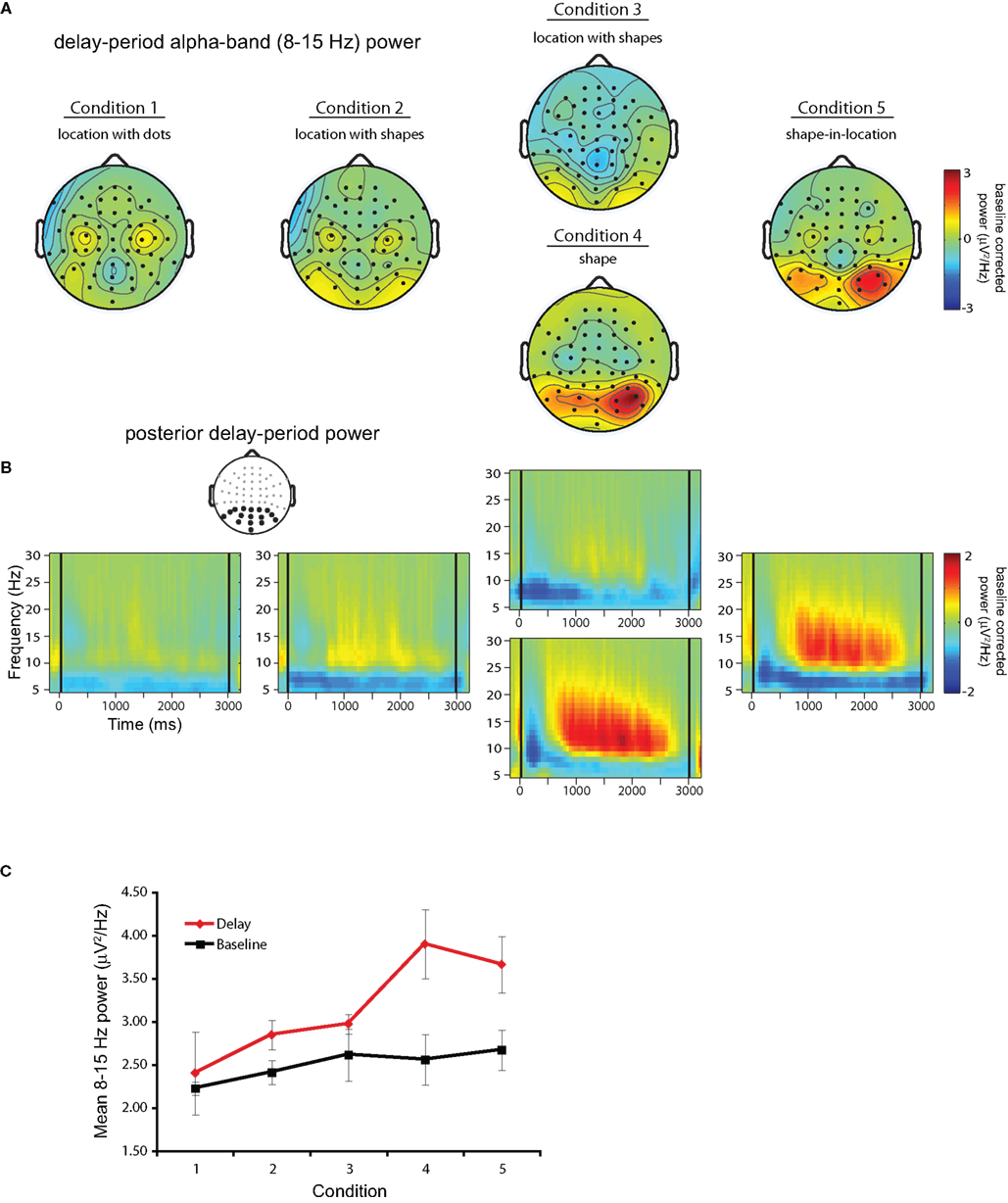
Figure 3. Delay-period alpha-band power. (A) Topographical plots of mean power in the 8- to 15-Hz frequency band averaged over the 500- to 2500-ms portion of the delay across each block/condition. (B) Time–frequency plots for each trial block across the full delay period (delay marked by black lines at 0 and 3000 ms) in the 5- to 30-Hz frequency range for the cluster of posterior electrodes highlighted in black in the inset topographical plot. (C) Comparison of uncorrected mean alpha-band power during the baseline interval (black solid line) and the delay period (red solid line).
Finally, to confirm that the increases in DPABP observed in Conditions 3–4 were indeed related to task performance, as opposed to an increase in resting alpha-band power across trial blocks, Figure 3C shows mean DPABP plotted separately for the baseline interval and the uncorrected delay period (500–2500 ms post delay onset) across conditions. As can be seen, although baseline alpha-band power did increase across blocks, this did not match the increase seen during the delay period. Thus, the elevated alpha-band power observed in Conditions 4–5 cannot be explained as an artifact of the fixed block order used here.
Results of the cluster-based permutation analysis are shown in Figure 4. In each panel, the results of the cluster analysis are plotted over the difference in DPABP between specific conditions of interest. Recall that both the inhibition and the active processing hypotheses predicted that DPABP would be minimal in Condition 1, and would increase with the addition of shape information in Conditions 2–3, in which locations were marked with unique shapes rather than uniform black dots. To assess this, our first analysis (Figure 4, leftmost panel) compared DPABP in Condition 1 to Condition 3. Using a dependent-t statistic, cluster-based analysis revealed no significant posterior clusters (all p’s > 0.10), although a significant negative cluster was observed over bilateral frontal electrodes, indicating higher alpha-band power over these areas in Condition 1 versus Condition 3. No significant clusters were observed between Conditions 1 and 2 or between Conditions 2 and 3 (all p’s > 0.10).
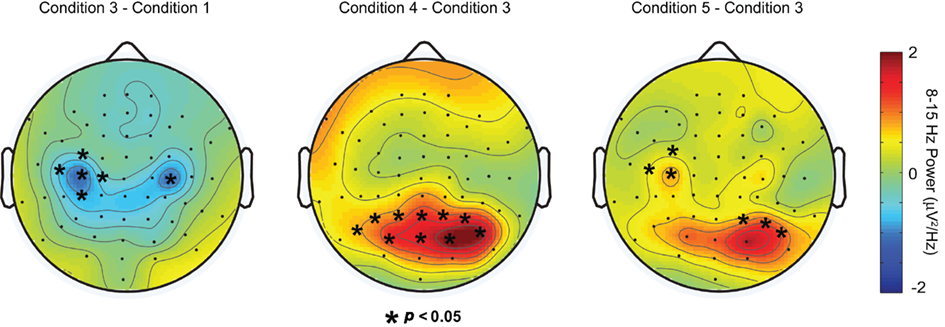
Figure 4. Results of cluster analysis plotted over the difference in delay-period alpha-band power between, from left to right, Conditions 3 and 1, 4 and 3, and 5 and 3, respectively. Asterisks overlayed on each topoplot indicate sensors showing significant differences in delay-period alpha power between conditions.
To determine if DPABP was greater when shapes were task relevant, versus when remembered locations were marked with unique task-irrelevant shapes, we next compared Condition 3 to Condition 4. Cluster analysis revealed a positive cluster of 11 electrodes exhibiting significantly elevated DPABP in Condition 4 (Figure 4, center panel).
Next, we compared DPABP in Condition 5 (shape-in-location) to Condition 3 (location with shapes, intermixed). Recall that the inhibition and object selection and maintenance hypotheses make opposite predictions regarding alpha-band power in this case. According to the inhibition hypothesis, increased DPABP reflects the functional inhibition of task-irrelevant dimensions (e.g., of location information in the shape task). Thus, in Condition 5, in which both the shape and the location of each stimulus is task relevant, DPABP should be minimal. Conversely, if increased DPABP is associated with the maintenance of shape information, or the binding of specific shapes to specific locations, DPABP should be elevated relative to Condition 3, in which only memory for location was required. As shown in Figure 4 (rightmost panel), cluster analysis revealed a positive cluster of three right posterior electrodes and three right frontal electrodes exhibiting increased DPABP in Condition 5 versus Condition 3. These findings support the proposal that elevated DPABP plays a role in the maintenance of shape information, rather than reflecting inhibition. Comparisons between Condition 5 (shape-in-location) and Condition 4 (shapes) revealed no significant clusters or trends, suggesting that the magnitude and topography of DPABP was comparable across these two conditions. Source localization of DPABP in Conditions 4–5 suggested distinct neural generators across conditions. However, these differences did not survive significance testing, and thus, are not discussed further here.
Discussion
The present study contrasted two views of the function of elevated DPABP. The first view holds that such increases reflect the functional inhibition of cortical areas representing potentially disruptive task-irrelevant information (see, e.g., Klimesch et al., 2007; Jensen and Mazaheri, 2010). The second view holds that increased alpha-band power represents an integral part of the distributed network activity related to the active processing of information in perceptual and cognitive tasks (see Palva and Palva, 2007). To explore these two possibilities, participants completed a series of delayed-recognition tasks in which the presence and task relevance of shape information was increased across trial blocks and the EEG was recorded to measure DPABP. In the critical task condition, participants were required to remember the unique pairing of shape and location for a small set of objects. We reasoned that, if DPABP primarily reflects the inhibition of task-irrelevant visual information, it should be minimal in this condition, because both shape and location information were task relevant. Conversely, if DPABP is related to the selection and maintenance of shape information, or shape–location associations, it should be elevated in this condition. Results were consistent with the latter possibility.
Specifically, time–frequency analysis revealed elevated DPABP when both the shape and the location of each object were retained in working memory. Increased DPABP was also observed during the retention of shapes alone, and, to a much lesser extent, during the retention of locations. However, in contrast to our previous unpublished observations and the predictions of the inhibition hypothesis, posterior DPABP did not increase significantly with the addition of unique task-irrelevant shapes in the location-memory condition. Thus, the pattern of results across conditions is consistent with the proposal that alpha-band oscillations play a role in the processing of shape information or shape–location associations in working memory.
Before accepting this conclusion, however, we need to consider whether the present results could be explained by the more general view of alpha-band power increases proposed by Klimesch et al. (2007). According to this view, elevated alpha-band oscillations observed during the delay period of working memory tasks reflects the operation of a top-down mechanism that suppresses the retrieval of potentially disruptive information, such as the representations of items that were remembered on previous trials. Thus, in addition to being present over task-irrelevant areas, elevated alpha could also be present over task-relevant areas, and in each case it would be inhibitory in nature. If this were the case, alpha power increases would be expected to occur during any task in which either (a) task-irrelevant information is present, or (b) stimuli are free to vary across conditions, and therefore the risk of proactive interference is high. Moreover, if these effects are assumed to be additive, alpha power increases should be maximal in conditions where both protection from proactive interference and from task-irrelevant information is necessary. Applied to the present study, therefore, alpha-band power should have been the highest in the location with shapes and the shape conditions, in which both the location and the shape of each of the stimuli varied unpredictably from trial to trial and task-irrelevant information was present (e.g., irrelevant shapes in the location with shapes condition). Conversely, the lowest alpha-band power should have been observed in the location with dots and the shape-in-location conditions, for which stimuli varied from trial to trial but no task-irrelevant information was present. In contrast to these predictions, however, what we observed was minimal alpha power during the delay period across each of the location-memory conditions, regardless of whether each location was marked by a uniform dot or a unique task-irrelevant shape, and elevated power in both the shape and shape-in-location conditions. Thus, this pattern of results does not appear to be consistent with the predictions of either variant of the inhibition hypothesis considered here.
Yet another possible explanation of our findings that would be consistent with the inhibition view, is that retaining both the shape and the location of a stimulus might be more demanding than remembering its shape or location alone. As a result, more “protection” may have been required to maintain a high level of performance in the shape-in-location condition, which could explain the increased alpha-band power in this condition. Indeed, several studies have demonstrated that alpha-band power increases as a function of task difficulty (see, e.g., Klimesch et al., 1999; Jensen et al., 2002; Händel et al., 2007). However, in the absence of behavioral evidence, either in terms of accuracy or RT, suggesting that the shape-in-location task was in fact harder than any of the other tasks, this explanation does not seem likely in the present case. Nonetheless, because performance was near ceiling across all conditions of the present study, it is not possible to completely rule it out. Therefore, in future studies it will be necessary to explicitly manipulate task difficulty, perhaps through the use of a wider range of set sizes than were used here.
The most plausible interpretation of our findings is that the alpha increases observed here reflect some aspect of the distributed activity related to the selection or maintenance of shape information in working memory. Precisely what role alpha-band oscillations are playing, however, will require further studies. One possibility is that the increased DPABP observed in the shape versus shape-in-location conditions are generated in different cortical regions for which alpha-band increases may have distinct functional consequences. For example, alpha-band oscillations generated in the occipital cortex have been associated with inhibitory effects (Bollimunta et al., 2008; Romei et al., 2008; Van Dijk et al., 2008), whereas alpha-band oscillations observed in frontoparietal (Palva et al., 2011) and IT cortex (Mo et al., 2011) have been associated with non-inhibitory perceptual and cognitive processing. Thus, although the topographies of the alpha-band effects from different conditions were strikingly similar, it is nonetheless possible that they reflect the activity of distinct cortical networks.
Conclusion
In the present study, we used EEG to explore the role of alpha-band oscillations in working memory. Our results were consistent with the view that sustained delay-period alpha-band oscillations reflect the functioning of neural processes involved in the selection and maintenance of shape information in working memory, rather than, or in addition to, reflecting the functional inhibition of task-irrelevant cortical areas. This adds to the growing body of evidence demonstrating that alpha-band oscillations can support multiple functions in the service of perception and goal-directed behavior.
Conflict of Interest Statement
The authors declare that the research was conducted in the absence of any commercial or financial relationships that could be construed as a potential conflict of interest.
Acknowledgments
We would like to thank Alex Shackman, Josh LaRoque, and Bornali Kundu for helpful discussions regarding various aspects of this project. This study was supported by grants MH88115-02 (Jeffrey S. Johnson), and MH064498-05 (Bradley R. Postle) from the National Institute of Mental Health.
References
Attneave, F., and Arnoult, M. D. (1956). Methodological considerations in the quantitative study of shape and pattern perception. Psychol. Bull. 53, 221–227.
Berger, H. (1929). Über das Elektroenkephalogramm des Menschen. Arch. Psychiatr. Nervenkr. 87, 527–570.
Bollimunta, A., Chen, Y., Schroeder, C. E., and Ding, M. (2008). Neuronal mechanisms of cortical alpha oscillations in awake-behaving macaques. J. Neurosci. 28, 9976–9988.
Busch, N. A., and VanRullen, R. (2010). Spontaneous EEG oscillations reveal periodic sampling of visual attention. Proc. Natl. Acad. Sci. U.S.A. 107, 16048–16053.
Cousineau, D. (2007). Confidence intervals in within-subjects designs: a simpler solution to Loftus and Masson’s method. Tutorials Quant. Methods Psychol. 1, 42–45.
Delorme, A., and Makeig, S. (2004). EEGLAB: an open source toolbox for analysis of single-trial EEG dynamics including independent component analysis. J. Neurosci. Methods 134, 9–21.
Grimault, S., Robitaille, N., Grova, C., Lina, J.-M., Dubarry, A.-S., and Jolicoeur, P. (2009). Oscillatory activity in parietal and dorsolateral prefrontal cortex during retention in visual short-term memory: additive effects of spatial attention and memory load. Hum. Brain Mapp. 30, 3378–3392.
Hamidi, M., Slagter, H. A., Tononi, G., and Postle, B. R. (2009). Repetitive transcranial magnetic stimulation affects behavior by biasing endogenous cortical oscillations. Front. Integr. Neurosci. 3:14. doi: 10.3389/neuro.07.014.2009
Händel, B., Lutzenberger, W., Thier, P., and Haarmeier, T. (2007). Opposite dependencies on visual motion coherence in human area MT+ and early visual cortex. Cereb. Cortex 17, 1542–1549.
Hanslmayr, S., Aslan, A., Staudigl, T., Klimesch, W., Hermann, C. S., and Bauml, K. H. (2007). Prestimulus oscillations predict visual perception performance between and within subjects. Neuroimage 37, 1465–1473.
Hollingworth, A., and Rasmussen, I. P. (2010). Binding objects to locations: the relationship between object files and visual working memory. J. Exp. Psychol. Hum. Percept. Perform. 36, 543–564.
Jensen, O., Gelfand, J., Kounios, J., and Lisman, J. E. (2002). Oscillations in the alpha band (9-12Hz) increase with memory load during retention in a short-term memory task. Cereb. Cortex 12, 877–882.
Jensen, O., and Mazaheri, A. (2010). Shaping functional architecture by oscillatory alpha activity: gating by inhibition. Front. Hum. Neurosci. 4:186. doi: 10.3389/fnhum.2010.00186
Jiang, Y., Olson, I. R., and Chun, M. M. (2000). Organization of visual short-term memory. J. Exp. Psychol. Learn. Mem. Cogn. 26, 683–702.
Jokisch, D., and Jensen, O. (2007). Modulation of gamma and alpha activity during a working memory task engaging the dorsal or ventral stream. J. Neurosci. 27, 3244–3251.
Kelly, S. P., Lalor, E. C., Reilly, R. B., and Foxe, J. J. (2006). Increases in alpha oscillatory power reflect an active retinotopic mechanism for distracter suppression during sustained visuospatial attention. J. Neurophysiol. 95, 3844–3851.
Klimesch, W., Doppelmayr, M., Schwaiger, J., Auinger, P., and Winker, T. (1999). “Paradoxical” alpha synchronization in a memory task. Brain Res. Cogn. Brain Res. 7, 493–501.
Klimesch, W., Sauseng, P., and Hanslmayr, S. (2007). EEG alpha oscillations: the inhibition-timing hypothesis. Brain Res. Rev. 53, 63–88.
Logie, R. H., Brockmole, J., and Jaswal, S. (2011). Feature binding in visual working memory is unaffected by task-irrelevant changes of location, shape, and color. Mem. Cognit. 39, 24–36.
Maris, E., and Oostenveld, R. (2007). Non-parametric statistical testing of EEG- and MEG-data. J. Neurosci. Methods 164, 177–190.
Mathewson, K. E., Gratton, G., Fabiani, M., Beck, D. M., and Ro, T. (2009). To see or not to see: prestimulus alpha phase predicts visual awareness. J. Neurosci. 29, 2725–2732.
Mo, J., Schroeder, C. E., and Ding, M. (2011). Attentional modulation of alpha oscillations in macaque inferotemporal cortex. J. Neurosci. 31, 878–882.
Oostenveld, R., Fries, P., Maris, E., and Schoffelen, J. M. (2011). FieldTrip: open source software for advanced analysis of MEG, EEG, and invasive electrophysiological data. Comput. Intell. Neurosci. doi: 10.1155/2011/156869
Palva, S., Kulashekhar, S., Hämäläinen, M., and Palva, J. M. (2011). Localization of cortical phase and amplitude dynamics during visual working memory encoding and retention. J. Neurosci. 31, 5013–5025.
Palva, S., and Palva, J. M. (2007). New vistas for α-frequency band oscillations. Trends Neurosci. 30, 150–158.
Perrin, F., Pernier, J., Bertrand, O., and Echallier, J. F. (1989). Spherical splines for scalp potential and current density mapping. Electroencephalogr. Clin. Neurophysiol. 72, 184–187.
Pfurtscheller, G., Stancak, A. Jr., and Neuper, C. (1996). Event-related synchronization (ERS) in the alpha band-an electrophysiological correlate of cortical idling: a review. Int. J. Psychophysiol. 24, 39–46.
Romei, V., Brodbeck, V., Michel, C., Amedi, A., Pascual-Leone, A., and Thut, G. (2008). Spontaneous fluctuations in posterior alpha-band EEG activity reflect variability in excitability of human visual areas. Cereb. Cortex 18, 2010–2018.
Sharbrough, F., Chatrian, G.-E., Lesser, R. P., LÜders, H., Nuwer, M., and Picton, T. W. (1991). American Electroencephalographic Society guidelines for standard electrode position nomenclature. J. Clin. Neurophysiol. 8, 200–202.
Thut, G., Nietzel, A., Brandt, S. A., and Pascual-Leone, A. (2006). Alpha-band electroencephalographic activity over occipital cortex indexes visuospatial attention bias and predicts visual target detection. J. Neurosci. 26, 9494–9502.
Treisman, A., and Zhang, W. (2006). Location and binding in visual working memory. Mem. Cognit. 34, 1704–1719.
Tuladhar, A. M., Ter Huurne, N., Schoffelen, J. M., Maris, E., Oostenveld, R., and Jensen, O. (2007). Parieto-occipital sources account for the increase in alpha activity with working memory load. Hum. Brain Mapp. 28, 785–792.
Van Dijk, H., Schoffelen, J. M., Oostenveld, R., and Jensen, O. (2008). Prestimulus oscillatory activity in the alpha band predicts visual discrimination ability. J. Neurosci. 28, 1816–1823.
Vanderplas, J. M., and Garvin, E. A. (1959). The association value of random shapes. J. Exp. Psychol. 57, 147–163.
Vogel, E. K., and Machizawa, M. G. (2005). Neural activity predicts individual differences in visual working memory capacity. Nature 428, 748–751.
Keywords: short-term memory, alpha band, oscillations, electroencephalography, inhibition
Citation: Johnson JS, Sutterer DW, Acheson DJ, Lewis-Peacock JA and Postle BR (2011) Increased alpha-band power during the retention of shapes and shape-location associations in visual short-term memory. Front. Psychology 2:128. doi: 10.3389/fpsyg.2011.00128
Received: 11 March 2011;
Accepted: 01 June 2011;
Published online: 10 June 2011.
Edited by:
Gregor Thut, University of Glasgow, UKCopyright: © 2011 Johnson, Sutterer, Acheson, Lewis-Peacock and Postle. This is an open-access article subject to a non-exclusive license between the authors and Frontiers Media SA, which permits use, distribution and reproduction in other forums, provided the original authors and source are credited and other Frontiers conditions are complied with.
*Correspondence: Jeffrey S. Johnson, Department of Psychiatry, University of Wisconsin – Madison, 6001 Research Park Boulevard, Madison, WI 53719, USA. e-mail: jsjohnson3@wisc.edu