- 1 Department of Psychology, Beckman Institute, University of Illinois at Urbana–Champaign, Champaign, IL, USA
- 2 Department of Psychology, The City College of the City University of New York, New York, NY, USA
Alpha oscillations are ubiquitous in the brain, but their role in cortical processing remains a matter of debate. Recently, evidence has begun to accumulate in support of a role for alpha oscillations in attention selection and control. Here we first review evidence that 8–12 Hz oscillations in the brain have a general inhibitory role in cognitive processing, with an emphasis on their role in visual processing. Then, we summarize the evidence in support of our recent proposal that alpha represents a pulsed-inhibition of ongoing neural activity. The phase of the ongoing electroencephalography can influence evoked activity and subsequent processing, and we propose that alpha exerts its inhibitory role through alternating microstates of inhibition and excitation. Finally, we discuss evidence that this pulsed-inhibition can be entrained to rhythmic stimuli in the environment, such that preferential processing occurs for stimuli at predictable moments. The entrainment of preferential phase may provide a mechanism for temporal attention in the brain. This pulsed inhibitory account of alpha has important implications for many common cognitive phenomena, such as the attentional blink, and seems to indicate that our visual experience may at least some times be coming through in waves.
Introduction
The bombardment of stimulation hitting our primary sensory areas necessitates selective enhancement of relevant information and suppression of whatever is irrelevant or interfering. Theories posit that salient properties inherent to the stimulus, top-down interventions, and fluctuations in attentional focus over time can modulate sensory processing, biasing it in favor of salient and task-relevant information at the expense of irrelevant or actively ignored stimuli (e.g., Desimone and Duncan, 1995; Corbetta and Shulman, 2002; Luck, 2009). Although a great deal of research has focused on understanding how top-down signals select task-relevant information, the specific mechanisms by which task irrelevant information is filtered out is less well understood. Recently, there has been growing evidence that alpha oscillations (8–12 Hz) can play a significant role in modulating input information by inhibiting visual and other neural processing.
In this paper we review evidence in support of three main theoretical points: (a) The role of the alpha rhythm goes beyond that of a mechanism for sensory disengagement, as it is also involved in cognitive control and attention through modulatory influences; (b) Alpha oscillations act as a pulsed-inhibition of neural processing, with synchronized oscillations in a brain area leading to periodic inhibition on every cycle, so that the phase of alpha is critical in determining whether or not processing is suppressed; (c) The brain exploits the alpha rhythm to appropriately time microstates of excitation and/or inhibition so as to be optimally ready to process or inhibit incoming information.
Alpha Power, Attention, and Awareness
When large ensembles of neurons fire in synchrony, an electric field is produced that is large enough to propagate through the brain and skull and can be measured with electrodes on the scalp. These ensembles of neurons fire at particular frequencies depending on their function and activity level. Thus, electroencephalographic (EEG) recordings provide data about the functional state of the neurons. Neural activity in the alpha range (8–12 Hz) has been shown to reflect the state of awareness of an individual. In the original EEG recordings by Berger (1929), and in subsequent work by Adrian and Matthews (1934), alpha oscillations were shown to vary as a function of the level of attention paid by the subject to the visual environment. As subjects began to lose attentional focus, increased amplitude of alpha oscillations was observed, with the largest alpha amplitude occurring when the subjects’ eyes were closed. For many years it was thus thought that alpha represented an idling process in the visual system, such that the amplitude of alpha oscillations in a given task could be used as an index of one’s general level of arousal or focus. More recently, alpha oscillations have begun to be viewed in a different light: that of an attention mechanism (e.g., Thut et al., 2006) or as a general inhibitory mechanism in the brain (e.g., Klimesch et al., 2007; Jensen and Mazaheri, 2010).
Oscillations in the alpha band (8–12 Hz) are a ubiquitous characteristic of neural activity. Alpha activity was the name given to synchronized 10 Hz activity measured in the EEG signal because it was the first, largest, and most easily identifiable signal in the single trace recorded by Berger’s primitive equipment (Berger, 1929). Subcortical recordings have revealed that this synchronization of 8–12 Hz neural firing is subserved by both (1) a thalamo-cortical loop involving the LGN, the pulvinar, and visual cortex (Steriade et al., 1990), and (2) interconnections between visual cortical regions (Lopes Da Silva et al., 1973, 1980; Lopes Da Silva, 1991). Intracranial recordings have further shown 8–12 Hz oscillations to be present throughout the cortex (Lopes Da Silva, 1991). At the scalp the largest alpha amplitude is observed over parietal and occipital areas (e.g., Johnson et al., 2010), although its frequency is slower and its distribution tends to be more frontal in older subjects (Gratton et al., 1992). Alpha is also evident over motor cortices in the form of mu oscillations (Pfurtscheller and Neuper, 1994)1.
Since this initial interpretation of alpha as a generic idling mechanism, increased focus has been placed on the specific influence of alpha oscillations on cognitive and neural processes (e.g., Klimesch et al., 2007; Jensen and Mazaheri, 2010). Spontaneous (i.e., not stimulus driven) increases in alpha oscillations have been shown to be associated with higher threshold detection rates across a wide range of stimulus types (e.g., Ergenoglu et al., 2004; Palva et al., 2005a; Romei et al., 2008a,b; van Dijk et al., 2008; Busch et al., 2009; Wyart and Tallon-Baudry, 2009) and with poorer performance on a number of cognitive tasks (e.g., Linkenkaer-Hansen et al., 2004; Mo et al., 2004; Babiloni et al., 2006; Del Percio et al., 2007; Hanslmayr et al., 2007; Mazaheri et al., 2009). For example, in a recent study, we showed that the detection rate of a near-threshold target stimulus that preceded a metacontrast mask was dependent upon the level of alpha power; detection rate increased monotonically as alpha power decreased (Figure 1; Mathewson et al., 2009).
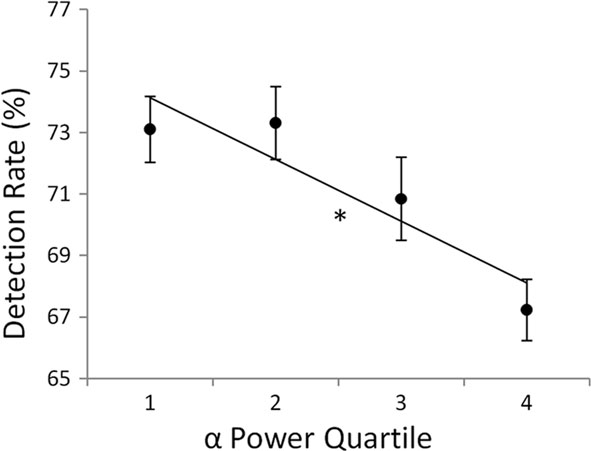
Figure 1. Detection rate of a metacontrast-masked-target decreased with increasing levels of pre-stimulus alpha power quartiles (1 = lowest, 4 = highest) measured on single-trials at electrode Pz. Error bars represent within-subject SE, *p < 0.05. (Figure adapted from Mathewson et al., 2009 reprinted with permission).
In other research, increases in alpha have been linked to the successful inhibition of distracter items to aid working memory representations (e.g., Hamidi et al., 2009; Sauseng et al., 2009). During a visual working memory task, for example, a set of four items was monitored for change after a brief blank period, while the visual distracter information on the opposite side of the screen was ignored (Sauseng et al., 2009). During the retention interval, the occipital alpha power over the side contralateral to the ignored visual stimuli increased. This asymmetric increase in alpha power was predictive of visual working memory performance across trials and subjects. The authors concluded that increased alpha activity allowed for more efficient suppression of the distracting visual stimuli in the unattended field. This result is consistent with the more general observation that an inward shift of attentional focus toward mental operations (as in mental rotation tasks, visual imagery, and other high-load working memory tasks) is typically accompanied by increases in posterior alpha power, suggesting that, in such cases, alpha might be working to inhibit sensory processing and decrease overall distractibility by sensory events (e.g., Jensen et al., 2002; Cooper et al., 2003).
More recently, studies have shown that the degree of alpha lateralization or its involvement in sensory inhibition is modulated by attention. Shifting or maintaining attention to one side of visual space leads to predictable fluctuations in the power and topography of alpha oscillations, which in general appear selectively increased ipsilaterally to the visual hemifield where the relevant information is presented (e.g., Worden et al., 2000). These controlled fluctuations in alpha have in turn been shown to influence target detection and visual performance (Sauseng et al., 2005; Kelly et al., 2006; Thut et al., 2006; Rihs et al., 2007, 2009; Capotosto et al., 2009; Wyart and Tallon-Baudry, 2009; Rajagovindan and Ding, 2010). A similar relationship has also been shown during shifts of lateralized auditory attention (Kerlin et al., 2010). Increased alpha oscillations also produce diminished transcranial magnetic stimulation (TMS) effects in both the visual (Romei et al., 2008b) and motor domains (Sauseng et al., 2009), indicating that alpha influences the excitability of the cortex.
Shifts in alpha power and/or scalp distribution have also been observed when participants are asked to attend to either the color or motion of visual stimuli (Min and Herrmann, 2007; Snyder and Foxe, 2010). Levels of alpha power have been shown to be modulated by temporal expectancy of stimulus onsets (Min et al., 2008), rightward shifts of attention during fatigue (Pérez et al., 2009) and following erroneous responses to visual stimuli (Carp and Compton, 2009), all indicating a role for alpha in selective neural processing. In animal studies using intracranial recordings, this relationship between decreased attention, increased alpha power, and selective processing has been shown to be present in areas V1, V2, and V4, whereas an opposite relationship has been measured in higher areas such as IT (Mo et al., 2011; Bollimunta et al., 2008, 2011).
In multimodal human studies combining EEG and fMRI, increased alpha oscillations have generally been shown to be related to decreases in the BOLD signal and cortical metabolism in sensory areas, and increased activity in so called default-mode areas (e.g., Moosmann, et al., 2003; Ben-Simon et al., 2008; Jann et al., 2010; Sadaghiani et al., 2010; Scheeringa et al., 2011). One must keep in mind when considering joint EEG–fMRI studies that the EEG power envelope measured on the scalp used to predict BOLD changes only represents a limited and spatially smeared window into the oscillatory activity of the entire brain – a window that is heavily weighted toward co-oriented sources close to the scalp.
Some past studies have shown that event-related brain potentials (ERPs) and subsequent BOLD signal measured concurrently are uninfluenced by the power of pre-stimulus alpha oscillations (Becker et al., 2008; Reinacher et al., 2009; Scheeringa et al., 2011). These findings have been taken as evidence against a proposal that ERPs are the result of ongoing alpha oscillations becoming phase reset to stimulus onset, since it would predict that trials with larger pre-stimulus power would have larger ERPs (Mazaheri and Jensen, 2006; Becker et al., 2008), although this debate continues (e.g., Klimesch et al., 2009; Risner et al., 2009). A recent finding of a U-shaped function between alpha power and ERP amplitude may explain these contradictory findings (Rajagovindan and Ding, 2010). It is likely that the relationship between alpha power and evoked activity is a complicated interaction between the stimulus modality and the predominant source of the surface-measured alpha activity. Recent advances in the use of multivariate variance decomposition techniques and source modeling are important for separating the various alpha generators from each other and from other neural activity (Snyder and Foxe, 2010; Scheeringa et al., 2011).
Klimesch et al. (2007) has recently proposed a theoretical framework for alpha oscillations. They propose that alpha represents a general inhibition mechanism across cortical areas, and emphasize the important and complementary role played by alpha oscillations in neural inhibition and timing. This framework not only explains the inhibitory modulations of alpha activity with attention and fatigue but also explains the role of alpha oscillations in other brain areas (e.g., Pfurtscheller and Neuper, 1994). The theory emphasizes the additional role played by alpha beyond a general inhibition of neural activity in constraining the timing of neural firing. Importantly, this theory makes some predictions about tasks in which increased focus of attention should actually increase alpha oscillations, when important inhibitory or timing processes are needed, which has indeed been observed (e.g., Ray and Cole, 1985; Sauseng et al., 2009). It is proposed that these timing mechanisms are subserved by the excitability cycle of alpha oscillations. It is also often observed that the alpha power differs between the two frontal hemispheres; a large amount of literature has investigated “frontal alpha asymmetry” as a marker of depression. This asymmetry may reflect an unbalance in the level of control exerted by frontal regions on ongoing goals, tasks, and emotionality (for a review see Coan and Allen, 2004). A general role for alpha as a control mechanism across cortical areas also explains large decreases in alpha power, referred to as either alpha desynchronization, alpha suppression, or alpha blocking, during important mental and sensory processing (e.g., Klimesch et al., 1998).
In support of the general role played by alpha in many brain areas, in a recent EEG study of video game training, we have shown predominant changes in alpha activity over the course of learning (Figure 2A). Subjects spent 20 h learning the complex video game Space Fortress, in which a player controls a ship with the goal of destroying a combative central fortress (Donchin et al., 1989). Subjects’ EEG activity was recorded during and outside of game play, before and after training. The amount of evoked alpha to relevant stimuli in the game increased as subjects learned to master the game (Figures 2B,C; Maclin et al., in press). Furthermore, the level of frontal alpha power during the first session playing the game could predict almost 50% of the variance in the rate of learning. Even the frontal alpha power measured outside of the game, prior to training, could predict subsequent learning rate. Furthermore, this effect could be dissociated from the mu-rhythm difference between left and right hemispheres associated with flying the ship, which was not predictive of subsequent performance. Finally, additional variance in game improvement was accounted for by event-related alpha desynchronization in response to successful hits on the fortress. Together, these results reveal a number of distinct types of alpha activity with different functional relevance for attention and performance. Importantly, these results suggest that frontal alpha plays an important role in cognitive/attentional control, which is critical in learning to perform optimally in a complex video game. Recently, it has also been shown that eye movements can be influenced by frontal alpha activity, and it has been proposed that frontal eye-field activity may be the source of these influences (Drews and VanRullen, 2011; Mazaheri et al., 2011). More research is needed to understand the difference between traditionally observed posterior alpha modulations and these recently observed frontal alpha effects. Additional research could also shed light on the relationship between frontal alpha modulations and the frontal theta differences reported in a number of studies (Cohen et al., 2007; Cavanagh et al., 2009). Finally, it is also important to note that cognitive control involves inhibition as well as activation. Therefore, this theory is consistent with the general idea that alpha is related to cortical inhibition.
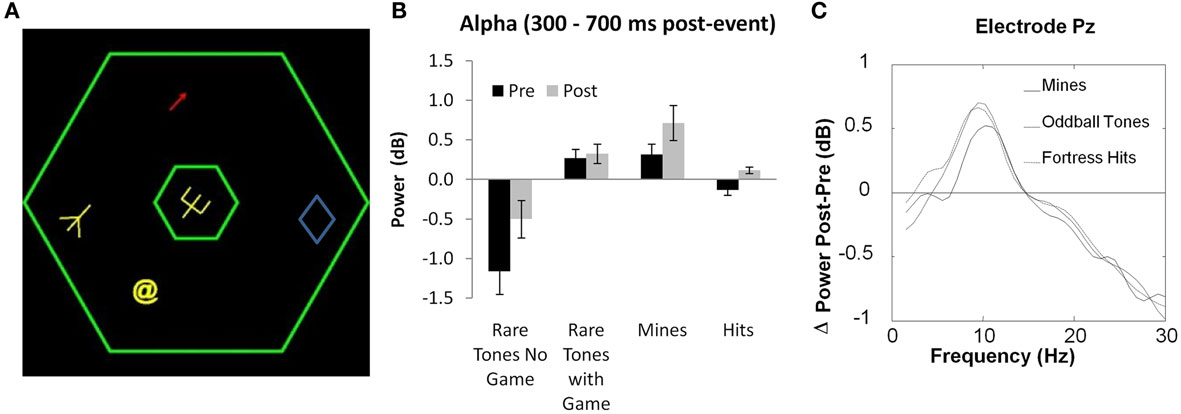
Figure 2. (A) Display for the game Space Fortress, on which subjects were trained for 20 h. (B) and (C) Changes in frontal and parietal mean evoked alpha oscillations between pre- and post-training EEG recording during the Space Fortress game, from 300 to 700 ms after stimulus onset. Error bars represent within-subject SE. (Figure adapted from Maclin et al., in press, reprinted with permission).
Alpha-Phase
Thus far, we have reviewed studies indicating the inhibitory role played by alpha activity in ongoing processing. Importantly these studies all make the assumption that modulation in alpha power represents an all-or-none brake applied to neural processing. By its very nature, however, alpha is an oscillatory phenomenon. Thus, is the inhibition indexed by alpha continuous or does it vary according to the peaks and troughs in the alpha cycle? An important corollary to this question is whether the presence of alpha is a correlate of inhibition or in fact a direct index of some of the mechanisms supporting inhibitory processes in the brain. Over the last century, interest has been directed toward understanding the cyclic nature of alpha oscillations. Speculations about the significance of different phases of the alpha cycle were already presented by Bishop (1933). These original speculations were echoed by Lindsley (1952), who proposed that the alpha rhythm “represented for the cell, or for the aggregates of cells with which it is associated, an oscillation in cortical excitability.” Lindsley’s prediction leads to the proposal that inhibitory effects should vary with the phase of alpha. Chang (1951) showed that the output of a frog neuron, given a constant level of stimulation oscillates at a frequency of roughly 10 Hz, indicating that the cell went through 10 Hz oscillations in its excitability. In subsequent decades, studies revealed an effect of the phase of the ongoing alpha activity on simple reaction time measures, with the slowest RTs for stimuli whose presentation was yoked to the scalp positive peak (Callaway and Yeager, 1960; Dustman and Beck, 1965). Recently, Hamm et al. (2010) reported a higher likelihood of saccades to a lateralized target in association with particular alpha-phases. Nunn and Osselton (1974) showed that masked visual presentation of the word “DANGER” led to skin conductance response changes that were modulated by the phase of ongoing alpha oscillations at the word onset, suggesting that this word was better processed at preferred phases of alpha oscillations.
The idea that alpha oscillations represent oscillations in cognitive processing has led some to speculate that the very nature of conscious visual perception occurs in perceptual moments or frames, and that perhaps a portion of the alpha cycle indexes the duration of these perceptual moments. Evidence for this has been provided in a study by Varela et al. (1981) in which subjects were presented with either simultaneous or sequential visual stimuli at either the peak of the trough of the scalp-measured EEG alpha oscillations. Visual events presented asynchronously together during the positive phase of an alpha oscillations were much more likely to be labeled incorrectly as simultaneous than those presented together during the troughs. However, this effect has since been hard to replicate (Gho and Varela, 1988; VanRullen and Koch, 2003).
More recent studies have further investigated the extent to which the processing of information varies as a function of the phase of ongoing local excitability cycles. Animal studies have shown that a subset of neurons preferentially fire during specific phases of the ongoing local field potential (LFP; Jacobs et al., 2007; Lakatos et al., 2008; Lörincz et al., 2009). A great deal of research has also shown that evoked responses (N1 and P1 components) to identical visual stimulation vary as a function of the phase of ongoing oscillations at the time of stimulation (Trimble and Potts, 1975; Brandt et al., 1991; Jansen and Brandt, 1991; Barry et al., 2003, 2004; Kruglikov and Schiff, 2003; Sachdev et al., 2004; Lakatos et al., 2008; Mathewson et al., 2009; or Haig and Gordon, 1998 who found later P3 differences). It is important to note, however, that evidence that these evoked potentials are not independent of the ongoing EEG phase cannot be considered as evidence that ERPs are merely artifacts of phase resetting, since additional ERP variance is unexplained by ongoing oscillatory activity (Kruglikov and Schiff, 2003; Mazaheri and Jensen, 2006; Becker et al., 2008). This debate is beyond the scope of the current review, but is germane to its thesis since Klimesch et al. (2007) have proposed that this phase resetting would serve to re-align the preferential processing phase of the alpha cycle to maximize stimulus processing. Recently, evoked BOLD responses to brief fixation events have been shown to vary as a function of the phase but not the power of independent alpha components of the simultaneously recorded EEG (Scheeringa et al., 2011), indicating that these phase effects can even have metabolic consequences. Together, these findings provide support for the view that alpha oscillations represent fluctuations in the ongoing excitability of the underlying cortical areas (Lindsley, 1952).
The interpretation of alpha-phase as representing different levels of cortical excitability leads to the prediction that identical visual stimuli may engender different perceptual representations depending on their synchronicity with the alpha-phase (Lindsley, 1952). Indeed, an early study found that identification of an auditory stimulus amongst noise differed as a function of the ongoing alpha-phase (Rice and Hagstrom, 1989).
In order to directly test this hypothesis, in a recent study we presented participants with brief targets that were followed closely in time by a metacontrast mask (Figure 3; Mathewson et al., 2009). We adjusted the timing and stimulus conditions such that, on average, our subjects detected about half of the targets that were presented, while maintaining low levels of false alarms on catch trials. This balance allowed us to directly compare targets that were and were not detected. We were specifically interested in the time period before the onset of the target, and the relationship between the alpha oscillations during this time period and subsequent awareness. Crucially, we showed for the first time that on trials with high levels of alpha power over posterior areas, the phase of the alpha activity preceding the stimulus could predict the subsequent detection of the masked-target. Targets presented in one half of the phase cycle were much less likely to be detected than targets presented at the opposite phase or on trials with low-alpha power (Figure 3A). This seems to indicate that the modulation is specific to a particular phase of the alpha cycle when alpha is high. Thus, there are two routes to detection: the first is to decrease alpha power, which increases cortical excitability and permits a consistent level of processing of the target; the second, when attention wanes and alpha power is high (or if a part of visual space is being inhibited and alpha power is high in the area of cortex processing that target), then certain phases of this increased alpha activity inhibit processing. Therefore, on average, targets will be less likely to be detected with larger levels of alpha power and/or when their timing is out of when their timing is in-phase with the inhibitory portion of the alpha cycle.
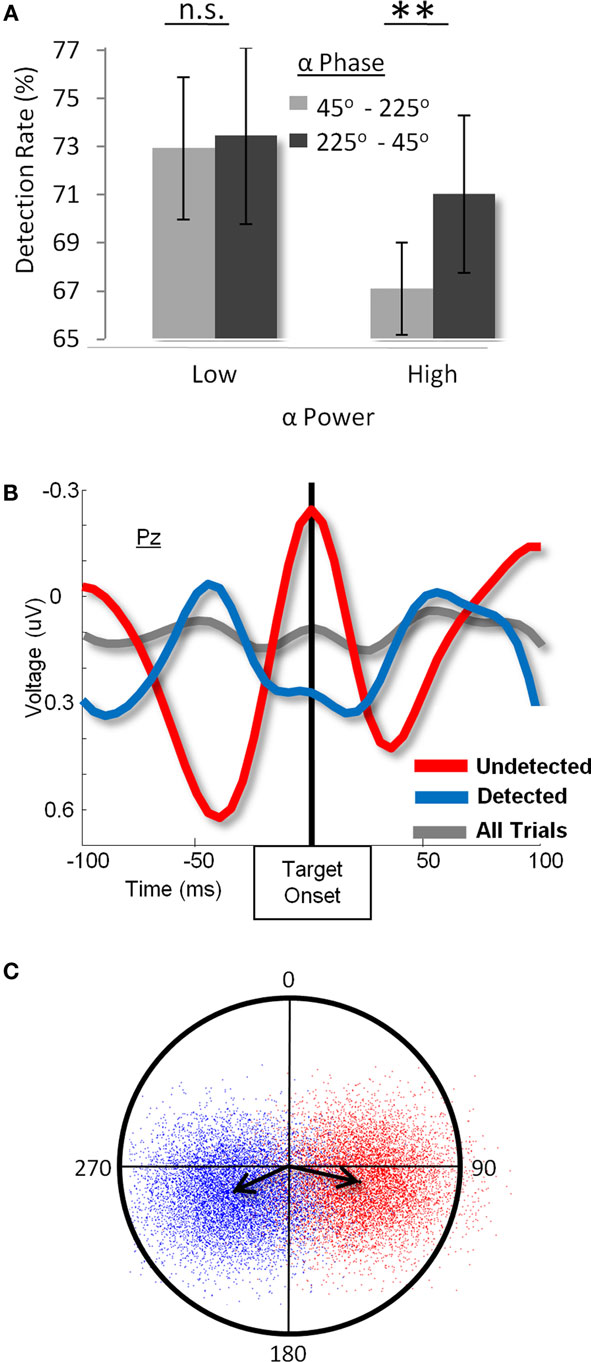
Figure 3. (A) Detection rate as a function of alpha power and phase before stimulus onset. When alpha power is low (left bar graph), there is no difference in masked-target detection as a function of pre-target alpha-phase. When alpha power is high (right bar graph), however, not only is detection lower overall, but it differs between opposite alpha-phases. (B). Grand-average ERP at the Pz electrode for detected (blue), undetected (red), and all (gray) targets. Results show the presence of counter-phase alpha oscillations between detected and undetected targets, whereas the overall average is flat, indicating that subjects did not phase lock to the stimulus before its onset. (C) Polar plot of a bootstrap-derived distribution of the average phase (angle) and amplitude (distance from origin) of pre-target 10-Hz oscillations for detected (red) and undetected (blue) targets. Each dot is the grand-average phase over the 12 subjects for one of 10,000 equally sized random samples from the two conditions. The arrows represent the centroids of the distribution of mean phases. (Figure adapted from Mathewson et al., 2009, reprinted with permission).
A possible methodological issue with the finding of an interaction between alpha power and phase effects on visual awareness is that on low-alpha trials the signal-to-noise ratio of the phase measurement will be inevitably lower. Thus any differences in the alpha-phase/detection relationship observed between high- and low-power trials may be due to increased error in-phase estimation for low-power trials. From a statistical standpoint it is very difficult to tease apart the influences of phase and power, as the reliability of the phase measurement inevitably varies as a function of the power level. Note, however, that our theory stems not only from the interaction of power and phase but also from the main effect of power. A crucial finding of the Mathewson et al. (2010) paper (see Figure 3A) is that detection rate is higher overall for low- than for high-alpha power trials. The most parsimonious account of these two effects (the interaction of power and phase, and the main effect of power) is to propose that the observed effects of phase and power on detection are linked by a single common mechanism; cortical excitability. In particular, awareness occurs when a threshold of cortical excitability is reached, be that during a certain phase when alpha is high or when alpha is low overall. When alpha is low, and thus cortical excitability is high, detection will be high regardless of the phase of alpha. An alternative account may propose that the influences of phase on neural activity are independent and dissociable from the influence of alpha power on the same activity. However, such an account is less satisfying as it is difficult to imagine independent effects of phase and power on neural activity and performance, given what we know about how synchronized fluctuations of neural activity give rise to the oscillatory activity we measure.
The finding that visual awareness fluctuates as a function of the phase of ongoing oscillations in the EEG was corroborated by a subsequent study that analyzed the temporal and frequency breadth of this effect for threshold-level targets (Busch et al., 2009). In their study, the targets, instead of being masked, were rendered difficult to detect by reducing their presentation time with a staircase procedure, such that each subject detected about half of them. Again, differences in the EEG preceding detected and undetected targets were examined. The authors considered a broad range of times and frequencies, and summarized the difference in-phase between the two conditions using a succinct statistic, the phase bifurcation index (PBI), which measured simultaneously the phase consistency and phase separation between two conditions. They predicted that if the phase of EEG oscillations differed between detected and undetected targets, then PBI should be high in that frequency, as each condition should have a preferential and opposite phase. Indeed, it was found that around 100 ms before the onset of the stimulus, 7–10 Hz oscillations in EEG had opposite phases for detected and undetected targets, thus replicating the core finding of the earlier Mathewson et al. (2009) study in the same journal, Interestingly, the authors found that the site of the maximal phase difference was frontal (Busch and VanRullen, 2010; Drews and VanRullen, 2011). The discrepancy in peak location of the phase effect between our study and those of the VanRullen group may be due to the different visual stimuli used in the tasks. Metacontrast masks are believed to limit visibility of the target by interrupting feedback information from higher visual areas, blocking any prospect of widespread reverberation of target information (Ro et al., 2003). In our paradigm, this would have limited the representation of the target to early visual areas. Not having masked the target, and thus not limiting its neural representation, Busch et al. (2009) may have maximized the influence of oscillatory activity occurring within higher-level visual areas on target detection. Furthermore, it is important to keep in mind that scalp topography can misrepresent the distribution of underlying sources. It is especially important to consider the reference to which each channels voltage is compared, which is unfortunately not reported in either the Busch and VanRullen’s or Drews and VanRullen’s papers (for reviews of the influence of EEG phase on visual detection, see Wyart and Sergent, 2009; Hoogenboom and Romei, 2010).
In a review recently published in this journal, VanRullen et al. (2011) provide a brief overview of their own research on the influence of EEG phase on visual perception and reaction time, as well as the relationship between these processes and attention. Unfortunately, the author’s characterization of the results of Mathewson et al. (2009) contains a number of factual errors. First, VanRullen et al. (2011) claim that the data presented in Mathewson et al. (2009; reproduced in Figure 3B of the current paper) show the band-passed time-average EEG activity when in fact the data depict the broadband ERP signal, indicating a large and obvious difference in-phase between detected and undetected targets. Second, the authors claim that our fixed inter-target interval may have introduced overall phase-locking to stimulus onset; however, the grand-average ERP across all trials (i.e., detected and undetected) also presented in the same figure, clearly shows no phase-locking to the onset of the target, indicating that phase-locking was not a generalized phenomenon (see gray line in Figure 3B). Lastly, the authors claim that we restricted our analyses to a time-averaged inspection of the waveforms. However, Mathewson et al. (2009) presented the results of five other analyses on the single trial phase and amplitude of alpha, all corroborating the finding of a difference in alpha-phase between detected and undetected items (here see Figures 3A,C for examples). In sum, our careful and extensive analyses clearly show the influence alpha-phase on visual awareness, and rules out a number of alternative explanations for the effects.
In summary, a number of studies have shown that the phase of ongoing alpha oscillations can influence the fine-grained timing of perception. These findings have received further support by Lörincz et al. (2009) who correlated the scalp-measured EEG in behaving cats with the local field oscillations (LFO) in the LGN, the first relay node on the main visual pathway. They showed that the output of thalamo-cortical relay neurons from the LGN is phase-locked to ongoing alpha oscillations in the LGN itself. The authors argue that the strong correlation between these two oscillations justifies the use of in vitro LGN preparations, which spontaneously sustain their alpha rhythm. In their cellular and network analyses the authors reported that the phasic output of the relay-mode thalamo-cortical neurons was locked to the phase of the ongoing LFO, with two groups of cells preferring to fire at the positive and negative alpha peaks, respectively.
Pulsed-Inhibition
Based on this converging evidence we propose that alpha oscillations represent a pulsed-inhibition of ongoing visual processing. As we have shown, when alpha oscillations are high in power, their phase has an influence on subsequent visual awareness. Brief visual events occurring in a particular phase of ongoing oscillations do not reach awareness, while those in the opposite phase do (Figure 3; Mathewson et al., 2009). This view is presented in Figure 4.
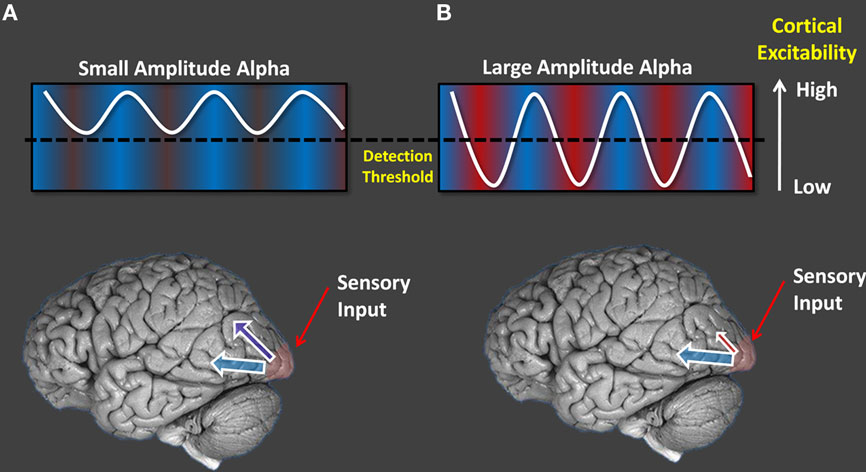
Figure 4. A schematic of the pulsed-inhibition account of alpha oscillations. [(A), left column] When alpha amplitude is low, cortical excitability is sufficiently high to produce consistently high levels of processing, and constant detection regardless of phase. [(B), right column] When alpha power is high, certain periods of its phase are inhibitory for visual processing and target detection differs as a function of the phase at which the target was presented.
When inhibition of some part of visual space, some part of time, or some visual feature is needed, top-down signals from fronto-parietal areas control the level of alpha oscillations (e.g., Sauseng et al., 2005; Capotosto et al., 2009). These ongoing oscillations can also fluctuate as a function of the current level of task engagement and vigilance (e.g., Mathewson et al., 2009). When alpha power is low in cortical areas, or during evoked alpha desynchronization, the excitability of that area is relatively high and above a threshold of awareness, leading to uniformly high performance. When alpha power is increased, the excitability becomes, on average, lower, leading to decreased performance. Inhibition is not constant, however, but is in fact pulsed as a function of the phase of the alpha oscillations, with periods of punctuate inhibition alternating with others of relatively intact processing (this is indicated in Figure 4A by the alpha wave oscillating around the threshold). In other words, phase and power are interdependent, with two possible states determined by power and with phase becoming important only when the power is high.
The proposal that alpha represents a pulsed-inhibition of ongoing brain activity is consistent with both Klimesch et al.’s (2007) theory that alpha reflects an inhibitory timing mechanism based on excitability cycles, as well as Jensen and Mazaheri’s (2010) recent proposal that alpha represents pulses of inhibitory gating of neural processing. The pulsed-inhibition mechanism we propose is similar to the asymmetric modulations in alpha that have been suggested by Mazaheri and Jensen (2010). The authors propose that alpha oscillations represent bouts of inhibition induced by GABAergic inter-neurons on the co-aligned pyramidal dendrites. Increases in this inhibitory activity would only modulate the peaks and not the troughs of alpha activity, since these dendritic currents are predominantly unidirectional. It has further been shown that these asymmetric modulations in alpha can account for some commonly observed slow ERP potentials (Mazaheri and Jensen, 2008; Van Dijk et al., 2010). Asymmetric modulations in alpha amplitude can also account for the fact that increases in alpha power lead to decreases in overall target detection, averaged across different phases.
Despite this common ground, there are also some differences across these accounts of the role of alpha oscillations. In our theory we stress an additional mechanism by which oscillatory alpha activity inhibits sensory processing. We have already considered the large amount of evidence for oscillations representing fluctuations in the excitability of the underlying cells. Increases in EEG alpha are associated with larger populations of neurons firing in synchrony with one another (e.g., Bollimunta et al., 2011). During periods of large-scale alpha synchrony, any given cell has less of a chance of firing during particular phases of the cycles. It is during these brief periods of widespread inhibition that alpha comes to exert its inhibitory effect on processing.
Thus, we propose that scalp-recoded alpha occurs when the excitability or inhibitory periods become synchronized over large populations of neurons. When there is less synchronization, these inhibitory periods are random and signals processed in the area can stand out against the noise. However, when oscillations become highly synchronized, periods of inhibition occur simultaneously across the population of cells, drowning out any signal representation. Interestingly, this theory has much in common with recent theories of attention from single cell recordings, where it has been shown that attention acts by decorrelating low-frequency noise in sensory areas (Mitchell et al., 2009).
To portray the theory in a metaphor, we imagine the oscillatory activity in a processing area as a large crowd at a football stadium. When the individual fans cheer at random times, any loud person can be heard over the hum of the crowd (e.g., “COLD BEER!”). However, when the same applause becomes synchronized in a unified cheer, brief periods of widespread sound drown out any other important sounds. Similarly, we propose that inhibition acts on sensory areas by synchronizing the oscillatory excitability cycles of neurons in those areas, drowning out incoming signals.
As mentioned, Jensen and Mazaheri (2010) propose that inhibitory GABA activity in sensory areas manifests itself as an asymmetric increase in alpha power due to its unidirectional current flow. In other words, they emphasize cellular-level mechanisms that may explain the same phenomena addressed by our model at a more global level of analysis. As such, the two theories are complimentary. However, these theories do lead to different predictions that can be tested experimentally. For instance, our theory would predict that any source of synchronized neuronal oscillations in sensory areas would inhibit processing, not just inhibitory GABAergic coupling. For instance, entrainment of cortical oscillations with TMS, or transcranial current stimulation would synchronize oscillatory activity presumably through electrical induction to a greater extent than by interneuron connections. Such data (see Thut and Miniussi, 2009) provide support for our theory, demonstrating that phase effects on cortical processing are observed for entrained brain oscillations (see below for a review that entrainment of oscillatory brain activity obtained with rhythmic visual stimulation can also have similar effects as spontaneous alpha in sensory processing).
Importantly, our pulsed-inhibition account of alpha can consolidate disparate theories of alpha activity. Palva and Palva (2007) have proposed that increases in alpha can also represent important active control processes, and emphasize the phase synchrony between frontal and parietal brain networks in the alpha band. In our model increases in alpha synchrony would not only be associated with widespread inhibitory pulses, but also with brief synchronized excitable periods in counter-phase. Thus, active cognitive and inhibitory roles of alpha oscillations may be two sides of the same pulsating coin. Importantly the Palva and Palva (2007) proposal also emphasizes the phase-amplitude coupling of alpha oscillations with higher frequency (e.g., gamma) neural activity. In other words, increased amplitude of high-frequency activity is seen during certain phases of lower-frequency alpha activity (Palva et al., 2005, 2010; Cohen, 2008; Osipova et al., 2008; Voytek et al., 2010). Given the enhanced and coordinated processing known to be associated with gamma activity (e.g., Tallon-Baudry and Bertrand, 1999), phase-amplitude coupling would provide an important strengthening of any low-frequency phase interactions.
An important feature of this conceptualization of alpha as a pulsed-inhibition of visual processing is its relation to top-down attention. Busch and VanRullen (2010) have investigated the relationship between the phase effects on visual awareness and attention. They first cued subjects to monitor a continuous stream of possible targets on one side of the visual field. However, subjects were also told to respond to targets presented in the uncued visual field. The authors found that the phase of EEG oscillations could predict target detection, but only when targets were presented on the cued side of the visual field. They interpret this finding as calling into question our proposal that increased alpha power and alpha-phase effects go hand in hand. Their reasoning is that, since increased attention should decrease alpha power, the pulsed-inhibition theory of alpha should predict that effects would be highest when alpha is high and targets are presented at an unattended location in the visual field (e.g., Thut et al., 2006; Mathewson et al., 2009). Importantly, the latter inference is predicated on the assumption that targets presented at the uncued location should be processed in a cortical region where alpha is particularly large. However, Busch and VanRullen (2010) did not measure the lateralization of alpha oscillations induced by the cue, and how the phase effects interacted with alpha power on a trial by trial basis. Furthermore, the data do indeed show a trend toward an effect of alpha-phase for targets presented at the unattended location, almost as large as that for those presented at the attended one, though not reaching significance. A further problem with their interpretation comes from the nature of the task itself, which in fact requires participants to respond to targets appearing at the “unattended” location. Thus, one may question to what extent that location was truly unattended, which, in turn, may have limited the extent to which alpha power was increased at that location; a strong inhibition of processing for stimuli presented at the unattended location should have produced large decreases in subjects’ performance, which did not occur.
Recently Capotosto et al. (2009) have begun to elucidate the brain networks responsible for top-down control of alpha variations. When repetitive TMS (rTMS) was applied to the FEFs and the IPS, the modulation of alpha activity as a function of attention leading up to the target onset diminished. Further, the alpha asymmetry elicited by spatial attention was also affected by rTMS and subjects no longer showed the benefits of spatial attention typically observed in a Posner cueing task. This provided the first causal evidence that attentional orienting by the FEF and IPS, in preparation of upcoming visual processing, is modulated by alpha oscillations. Indeed, Hamidi et al. (2009) also found that 10-Hz rTMS to IPS and FEF biased alpha oscillations and influenced target detection. Furthermore, simultaneous EEG and fMRI recordings reveal that activity in the dorsal attention network is negatively correlated with alpha power (Sadaghiani et al., 2010). We have recently combined the use of fast optical imaging of neuronal activity using the event-related optical signal (EROS; Gratton and Fabiani, 2010) and EEG to investigate the regions associated with changes in alpha activity. We found areas in both frontal and parietal regions whose activity correlated with changes in alpha power (Mathewson et al., in preparation).
In summary, we propose that alpha oscillations represent a pulsed-inhibition on ongoing processing. The power of these alpha oscillations can be controlled by top-down attention from fronto-parietal structures with the goal of biasing ongoing processing in favor of the task-relevant or attended stimulus. Indeed, the FEF and IPS are thought to bias visual processing largely through the pulvinar thalamic nucleus (Corbetta et al., 2008), which in turn has been shown to account for a significant proportion of the variance in posterior cortical alpha power (Steriade et al., 1990). These controlled modulations of alpha power then influence cortical excitability, inhibiting the processing of unattended or actively ignored items. When alpha power is high, the phase of the ongoing alpha oscillation can predict amount of processing, target detection, and response time.
Entrainment of Temporal Attention
Our proposal that alpha oscillations represent a pulsed-inhibition of ongoing processing would predict that if one were able to control the phase of these oscillations, one could manipulate these fluctuating periods of enhanced and inhibited firing. Indeed, research has shown that the phase of ongoing oscillations in the EEG can become automatically entrained to rhythmic stimuli in the environment (Adrian and Matthews, 1934; Walter and Walter, 1949). This so called steady-state visual evoked potential (SS-VEP) has been shown for a range of frequencies from 1 to 100 Hz (Herrmann, 2001; Pastor et al., 2002, 2003) and attention enhances this effect (Morgan et al., 1996; Rosenfeld et al., 1997; Müller et al., 1998; Andersen et al., 2008; Lakatos et al., 2008), suggesting that entrained oscillations in cortical excitability may represent a mechanism for temporal attention to rhythmic environmental events (Large and Jones, 1999; Coull et al., 2004; Buhusi and Meck, 2005; Schroeder and Lakatos, 2009). It is unclear, however, if entrained oscillations in the cortex have the same functional impact as do endogenously controlled changes in cortical oscillations.
Manipulations and theories of visuo-spatial attention are ubiquitous in the cognitive psychology literature (e.g., Posner and Petersen, 1990; Corbetta and Shulman, 2002; Reynolds and Heeger, 2009). However, only a small amount of research has extended these constructs into the temporal domain, investigating how attention to sensory objects fluctuates with time (Coull and Nobre, 1998; Large and Jones, 1999; Coull et al., 2004; Schroeder and Lakatos, 2009). Many parallels can be drawn between what can be characterized as visual attention and temporal attention, respectively. Both consist of mechanisms by which processing of a stimulus is enhanced at the expense of that of other stimuli (i.e., James, 1890; Posner and Peterson, 1990; Levinthal and Lleras, 2008; Yashar and Lamy, 2010). Theorizing about attention in the temporal domain has been limited (but see Jones, 1976; Coull and Nobre, 1998; Coull et al., 2004; Schroeder et al., 2008). In our day-to-day conversations, we are bombarded with rhythmic auditory information, which we can parse into individual sound units or phonemes, whose serial order can be distinguished with delays as short as 30 ms (Schmidt-Kassow and Kotz, 2008). Our ability to successfully attend to the relevant features of sound or speech developing over time thus relies on some sort of internal clock mechanism, which is able to predict from the ongoing information the timing of important upcoming events.
Jones (1976) proposed that, “…organisms are basically rhythmical and possess their own temporal structures which are manifested psychologically in a series of tunable perceptual rhythms…” In a more recent quantitative explication of the theory, Large and Jones (1999) posit the presence of internal oscillators representing pulses of “attention energy” that can be entrained to the timing of external rhythms. In their theoretical framework, attention allows for these internal oscillators to adjust their parameters in order to time their pulses of “attention energy” with relevant environmental stimuli, in a sense coupling the internal rhythms with those in the environment. Both the phase and the period of the internal oscillations become coupled to the phase and period of oscillations in the environment. Thus the future onsets of environmental stimuli will occur at times predicted by the entrained internal oscillations. This will allow the pulse of attention energy to enhance processing. To test this proposal, Jones et al. (2002) presented subjects with a standard auditory tone followed after a set of rhythmic distracters by a comparison tone. Subjects were best at comparing the two tones when the comparison tone was presented in-phase with an embedded rhythmicity in the irrelevant distracter tones. This was taken as evidence that waves of attentional energy can be built up to preferentially process temporally predictable stimuli.
To extend these series of studies to the visual modality we asked whether we could see evidence of entrainment for visual stimuli presented in the alpha range. We conducted the first behavioral test of this hypothesis as depicted in Figure 5 (Mathewson et al., 2010). We presented 8, 4, or 2 annuli prior to the onset of the target. These annuli, identical to the subsequent mask, were present at regularly spaced intervals, every 83 ms, leading to a presentation rate of 12 Hz, within the normal range of alpha oscillations. After the onset of the final entrainer, we presented the target at various lags with respect to the timing of the previous entraining stimuli. Targets were either presented in-phase with the preceding entrainment, out-of-phase with the entrainment, or in between. We predicted a quadratic effect as a function of lag after entrainment, with a peak in detection for targets in-phase with the entrainment, when the optimal phase of their excitability cycle would occur due to the processing of the previous stimuli.
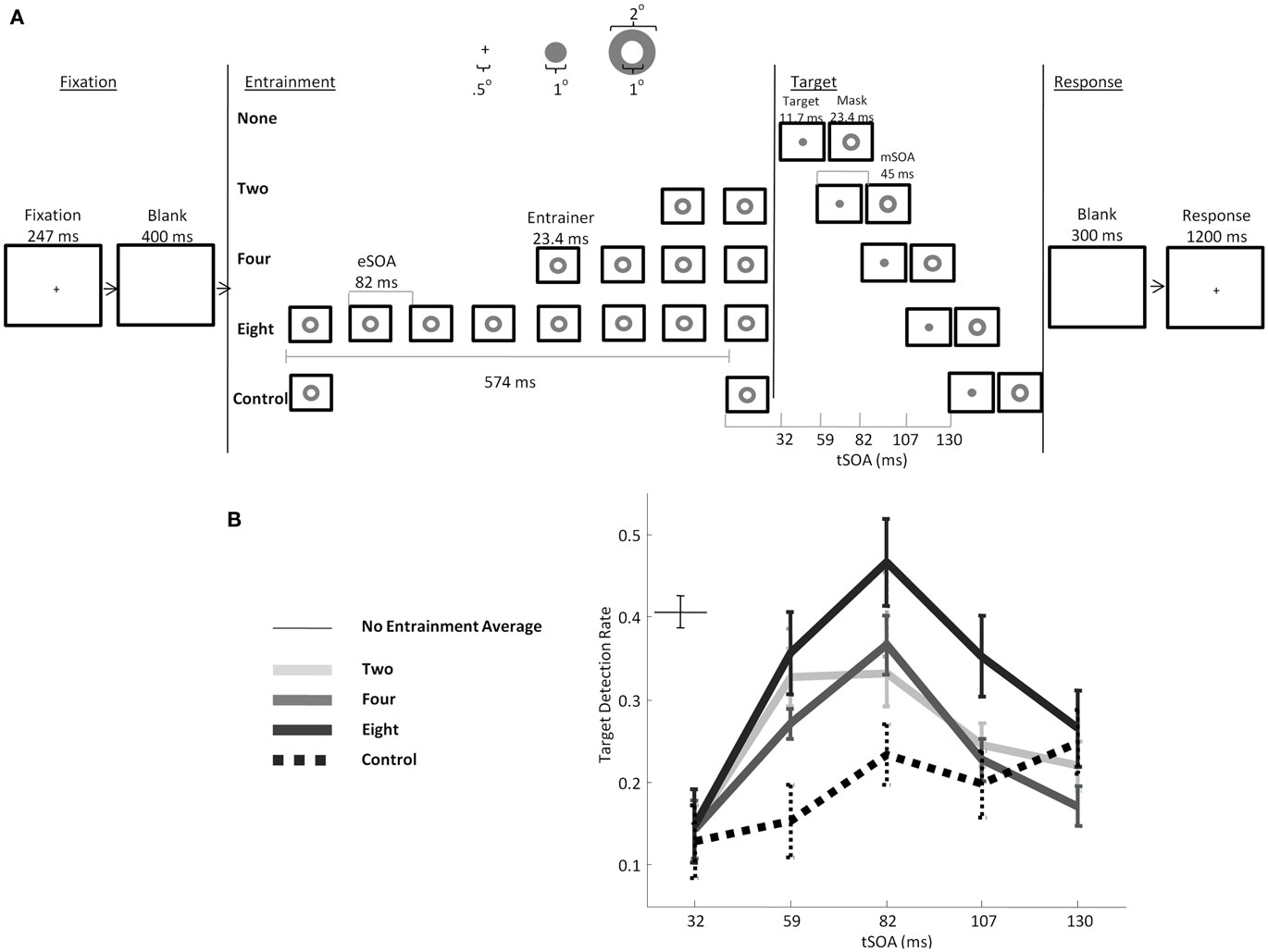
Figure 5. (A) Schematic of the trial timeline and stimulus dimensions from Mathewson et al. (2010). Subjects were presented with a masked visual target at various lags after a series of periodic preceding annuli. (B) Targets were better detected if they occurred in synchrony with the preceding rhythmic flashes, and this effect scaled as a function of the number of pre-target periodic stimuli. (Figure adapted with permission from Mathewson et al., 2010).
As can be seen in Figure 5B, we indeed found an effect of the entrainment, such that targets presented in-phase were better detected than targets presented out-of-phase. Furthermore, this effect scaled as a function of the number of entrainers, indicating that the entrainment process takes time to build up. When only two annuli were presented, but with a long gap in between to control for the length of the eight-entrainer-foreperiod condition (book-end condition), only a linear increase in detection was observed, with no peak in detection at 83 ms. In other words, in the absence of rhythmic entrainment, a single annulus preceding the target actually decreases target visibility (so called forward masking). Yet, when this last annulus is one of several in a rhythmic sequence, targets are released from forward masking and visibility changes in a phase-dependent manner. Importantly only the peak detection at 83 ms in the eight-entrainer condition was greater than the baseline detection rate with no preceding stimuli (Figure 5B), indicating that this rhythmic stimulation predominantly resulted in poorer detection for out-of-phase targets, but little or no enhanced processing for in-phase targets. This is consistent with the view that alpha is a pulsed-inhibition on visual processing, in that the majority of the change from the control condition was inhibitory and resulted in poorer detection.
The results of Mathewson et al. (2010) provide strong support for Jones et al.’s (2002) proposal, and reveal that detection of visual stimuli is influenced by their temporal predictability with respect to preceding visual events. Relatedly, Schmidt-Kassow et al. (2009) found that the P3b ERP response was earlier in response to stimuli in a regular sequence compared to a random sequence, indicating faster processing. In keeping with this hypothesis, in non-human primates, low-frequency (1.5 Hz) LFP oscillations can become entrained to external stimulus periodicity in an attended modality (Lakatos et al., 2007). The phase of these entrained oscillations then influences both the evoked response and RTs (Lakatos et al., 2008). This same effect has recently been replicated in humans (Stefanics et al., 2010) and has been shown in intercranial recordings in human patients to be enhanced by both attention and the predictability of stimuli (Besle et al., 2011). Further effects of temporal expectancies and entrainment of oscillations have been shown in the gamma range (over 30 Hz). Gamma flickering that is undetectable by subjects has nonetheless been shown to influence attentional orienting performance, thus indirectly influencing visual detection (Bauer et al., 2009). Finally, gamma has been driven by optogenetic stimulation, affecting sensory responses (Cardin et al., 2009). Rhythmic stimulation of the whiskers of a rat has also been shown to entrain related sensory areas (e.g., Tamereanca et al., 2008), as does sound stimulation (Gao et al., 2009). Somewhat frightening, Williams et al. (2004) have shown that the refresh rate of video displays such as computer monitors can entrain neural oscillations.
In order to make the crucial link between the EEG alpha-phase effects we observed in Mathewson et al. (2009), and the behavioral influence of entrainment at these same alpha frequencies on subsequent timing of visual awareness (Mathewson et al., 2010), we next recorded EEG activity in a modified version of the entrainment paradigm. We predicted that a rhythmic visual sequence in the alpha range would not only entrain alpha but at the same time entrain visual awareness. In the study, we asked if the hypothesized pulsed-inhibition represented by the phase of alpha oscillations could be controlled. Specifically, we predicted that stimuli presented at 12 Hz would entrain brain oscillations. We presented eight entraining stimuli directly before the onset of a metacontrast-masked visual stimulus identical to that used in Mathewson et al. (2009). We compared the eight-entrainer condition from the previous study to both the same book-end condition and an additional condition in which we added variability to the rhythmicity of our entrainment sequence. On variable entrainment trials, the same overall sequence length as the eight-entrainer condition was used, but the timing of the entrainers was jittered such that they were not presented at regular intervals. On each trial, one of these three pre-stimulus conditions was presented, followed by the target. The target was presented at one of seven lags after the onset of the final entrainer. This way, we had two target presentations that were in-phase with the preceding entrainment (first and second peak of the presumed oscillation), two that were out-of-phase, and three intermediaries.
To what extent are these induced fluctuations in awareness dependent on induced oscillations in the brain? First, entrainment led to increased phase-locking to the rhythmic stimuli compared to the variable condition, the degree of which predicted detection rate across subjects. Second, entrainment led to differences in the phase of 12-Hz oscillations over parietal areas between in-phase and out-of-phase targets, the size of which predicted the difference in detection between in- and out-of-phase targets. Finally, we found an analogous difference in alpha-phase between detected and undetected targets, replicating our previous findings (Mathewson et al., 2009). Interestingly, these effects were partially dependent on both pre-trial and pre-experiment oscillations in the alpha range, providing further evidence of a link between alpha and the entrainment of awareness.
These entrainment data can be accounted for by an expansion of the thalamo-cortical interaction model proposed by Lörincz et al. (2009). In their model, the output of the feed-forward neurons transmitting information from the retina to the cortex is modulated by a set of inhibitory inter-neurons in the LGN whose activity occurs in 10-Hz bursts, which act as pace-makers for the cortical alpha rhythm. Recently it has even been shown that the oscillating electrical fields created by neural populations can entrain the firing of local neurons through ephaptic coupling of gap junctions (Anastassiou et al., 2011). In the entrainment condition, we may assume that the interneuron activity is itself modulated by the feed-forward sensory input. This would cause the interneuron activity to be phase-locked with the entrainers. As a consequence, cortical alpha activity would also be entrained. Thus, modulated signals arrive in V1 already under the influence of modulatory oscillations, and these modulatory oscillations also are represented at later visual stages where they can continue to influence perception (Figure 4B). This synchronization suggests a mechanism for how specific phases with respect to stimulus onset lead to better performance on a wide range of cognitive tasks (e.g., Jansen and Brandt, 1991; Haig and Gordon, 2008; Mathewson et al., 2009).
These studies of the entrainment of ongoing oscillations have been supported by recent work using various transcranial stimulation protocols, and together with previous results attest to the causal role played by the power and phase of alpha oscillations on visual processing. Entrainment of beta oscillations over motor areas by transcranial alternating current stimulation (tACS) increased beta oscillations and in turn slowed movements (Pogosyan et al., 2009). rTMS at alpha frequencies has been shown to bias alpha oscillations in working memory tasks, leading to effects very much like those observed for spontaneous alpha (Hamidi et al., 2009; Sauseng et al., 2009). Both tACS and rTMS at 10 Hz have been shown to increase alpha oscillations (Johnson et al., 2010; Romei et al., 2010; Zaehle et al., 2010) and lead to analogous effects on visual detection as endogenous alpha oscillations (e.g., Romei et al., 2010), although specific entrainment of the phase of ongoing oscillations such that behavior is influenced has yet to be empirically shown. tACS has also been shown to entrain oscillations in animals (Ozen et al., 2010). However, given the highly unnatural nature of tACS, it is likely that entrainment by TMS or tACS will have different and possibly more unpredictable consequences on underlying EEG oscillations and behavior.
Entrainment of the phase of ongoing oscillations may explain some important and pervasive effects in common psychological tasks. For instance, when distracters are presented at fixation in a rapid serial visual presentation (RSVP, most often at 10 Hz; Raymond et al., 1992) task, targets embedded in the regular sequence are usually easily detected, with some important exceptions. Participants’ ability to identify the target in the rapid sequence increases monotonically as a function of position in the series, from about 70% for position 2 to above 90% for position eight, after which performance asymptotes (Ariga and Yokosawa, 2008; Ambinder and Lleras, 2009). This increase in performance as a function of position in the stream has been labeled “attentional awakening” to indicate that temporal attention to the sequence is becoming entrained to the rhythm of the RSVP, as the stream unfolds. This effect is very much consistent with the data reported by Mathewson et al. (2010), where increasing the number of pre-target entrainers increased detection monotonically from 0 to two to four to eight entrainers. Thus, even though the targets in the RSVP sequences were all in-phase with the distracters, this preferential phase-locking requires time to build up (Large and Jones, 1999; Mathewson et al., 2010). Indeed, when the timing of the distracters in the RSVP sequence is jittered such that their sequence is not perfectly rhythmic, initial target detection decreases (Martin et al., 2011).
A common manipulation in RSVP paradigms is to insert a second target (T2) into the sequence and investigate how its visibility is influenced by its temporal position with respect to the first target (T1). It is typically found that T2 accuracy is diminished when it follows T1 by 2–7 items, an effect referred to as the attentional blink. One possibility for this effect is that the entrainment of alpha-phase by the distracters is interrupted by the processing of the target (Arend et al., 2006). A phase interruption would seem to be necessary in order to stop the enhanced processing of the subsequent distracters from drowning out the target information. This preferential phase would then need to be reestablished by another gradual entrainment, explaining the similar time course of the attentional awakening and attentional blink (but see Ambinder and Lleras, 2009). Martin et al. (2011) found that the attentional blink was also decreased if jitter was added to the distracters prior to its presentation, indicating that the regular distracter intervals are an important precursor to the blink.
Summary and Conclusion
This review highlights the important role that alpha oscillations have in modulating sensory input. More generally, it suggests that alpha oscillations may be an important mechanism by which inhibitory influence and attentional control are exerted over different cortical activities. Alpha oscillations are in large part determined by interactions between thalamo-cortical and intra-cortical neuronal populations. Specifically, they may be due to the activity of GABAergic inhibitory inter-neurons, which may themselves receive input from excitatory output neurons. The manifestation of this circuitry is oscillatory activity, which modulates cortical excitability. This mechanism may be ubiquitous throughout the cortex, although the frequency of the oscillation may perhaps vary from area to area. According to this view, the appearance of the alpha rhythm is not merely a correlate of a state of low cortical activation, but rather a mechanism itself, by which low cortical excitability is enabled. Maintaining low excitability in an extended portion of the cortex probably serves a very important adaptive role, in that it allows for important information processing to occur undisturbed by irrelevant and secondary processes. At the same time, the oscillatory nature of the alpha rhythm allows for some of the unattended information to filter through; this may be of critical importance in cases in which the unattended information may be valuable. This is the sense in which we have drawn an analogy between the alpha-based mechanism for dealing with irrelevant information and the “anti-lock brake” (ABS) system of a car, in which some level of contacts with the road surface (in our case, the external environment) is maintained by applying pulses of braking rather than by braking continuously.
Our most recent data suggest that this pulsed-inhibition can become entrained to rhythmic external stimulation. This can be easily accounted for by the neuronal mechanisms that we have postulated to be at the bases of alpha. Interestingly, they suggest that alpha oscillations may be part of a general temporal tuning mechanism, by which our brain can exploit regularities in the environment to optimize processing.
A number of questions, however, remain open. For instance, what is the relationship between alpha and other types of oscillatory activity described in the brain? Electrophysiologists have long described a number of other rhythms characterized by other frequencies (such as beta, theta, gamma, and delta), and often correlated them with similar concepts such as perception, attention, and consciousness (Monto et al., 2008). The literature suggests that our brains can pick up on this wide range of frequencies. A question then follows: Is alpha special, or just one of the many rhythms at which cell ensembles can operate? Or, are different types of cell ensembles involved in different oscillations, and, if so, do they bring forth special types of brain states when their oscillations are triggered?
What is the relationship between brain oscillations and consciousness? James (1890) wrote that consciousness: “…does not appear to itself be chopped up in bits. Such words as ‘chain’ or ‘train’ do not describe it fitly … It is nothing jointed … it flows. A ‘river’ or a ‘stream’ are the metaphors by which it most naturally described.” Since James’ (1890) proposal that our conscious stream is not jointed but flows, many have proposed that in fact our perception of the world is more discrete and quantized (Efron 1970a,b; Dehaene, 1993; VanRullen and Koch, 2003; Smith et al., 2006; Pöppel, 2009). Some have proposed that this quantized nature may account for some common illusions. The discrete frames of our visual perception, perhaps related to the phase of ongoing excitability cycles, may create an aliasing during certain motion perception (Andrews and Purves, 2005; Simpson et al., 2005; VanRullen et al., 2005, 2006). Indeed, fluctuations in the illusory motion percepts are shown to correlate with changes in alpha oscillations (VanRullen et al., 2006; but see Kline et al., 2004, for an alternative account). We predict that the pulsed-inhibition of alpha oscillations may be the underlying cause of these so called perceptual moments.
The present review article summarized the evidence that, at least in some cases (such as when alpha power is high) our perception of the visual world may be discontinuous in nature. Specifically, during particular phases of the alpha oscillations, information is shown to be very poorly processed, while at other times information processing is enhanced. We summarized the evidence that sensory processing is gated by the phase of ongoing oscillations in baseline brain activity, creating oscillating periods of high and low neural excitability. We also described how these oscillations in cortical excitability are associated with concomitant fluctuations in our visual awareness; targets presented at particular phases of these oscillations do not reach awareness. We propose that these cycles of excitability act as a pulsed-inhibition on ongoing processing and that this inhibitory processing mode is common across many brain areas. Finally we reviewed evidence that the timing of the preferential phases of processing can be entrained to rhythmic stimuli in the environment, providing a possible mechanism for temporal attention in the brain.
Conflict of Interest Statement
The authors declare that the research was conducted in the absence of any commercial or financial relationships that could be construed as a potential conflict of interest.
Acknowledgments
The research reviewed here was supported by a Natural Science and Engineering Research Council of Canada (NSRC) Fellowship to Kyle E. Mathewson, Office of Naval Research MURI grant to Art Kramer, by a National Science Foundation grant #0843148 to Tony Ro, and by a National Institute of Mental Health grant # MH080182 to Gabriele Gratton.
Footnote
- ^It is important to note that not all cortical regions are equally likely to generate large scalp alpha activity. Certain brain areas are located far from the surface of the head, or their orientation is not conducive to generate large surface activities. Finally, some adjacent regions may generate counter-phase activity, which may cancel out. As a consequence, the amplitude of alpha oscillations may possess a very specific scalp distribution.
References
Adrian, E. D., and Matthews, B. H. C. (1934). The Berger rhythm: potential changes from the occipital lobes in man. Brain 4, 355–385.
Ambinder, M. S., and Lleras, A. (2009). Temporal tuning and attentional gating: two distinct attentional mechanisms on the perception of rapid serial visual events. Atten. Percept. Psychophys. 71, 1495–1506.
Anastassiou, C. A., Perin, R., Markram, H., and Koch, C. (2011). Ephaptic coupling of cortical neurons. Nat. Neurosci. 14, 217–224.
Andrews, T., and Purves, D. (2005). The wagon-wheel illusion in continuous light. Trends in Cogn. Sci. 9, 261–263.
Andersen, S. K., Hillyard, S. A., and Müller, M. M. (2008). Attention facilitates multiple stimulus features in parallel in human visual cortex. Curr. Biol. 18, 1006–1009.
Arend, I., Johnston, S., and Shapiro, K. L. (2006). Task-irrelevant visual motion and flicker attenuate the attentional blink. Psychon. Bull. Rev. 13, 600–607.
Ariga, A., and Yokosawa, K. (2008). Attentional awakening: gradual modulation of temporal attention in rapid serial visual presentation. Psychol. Res. 72, 192–202.
Babiloni, C., Vecchio, F., Bultrini, A., Luca Romani, G., and Rossini, P. M. (2006). Pre- and poststimulus alpha rhythms are related to conscious visual perception: a high-resolution EEG study. Cereb. Cortex 16, 1690–1700.
Barry, R. J., de Pascalis, V., Hodder, D., Clarke, A. R., and Johnstone, S. J. (2003). Preferred EEG brain states at stimulus onset in a fixed interstimulus interval auditory oddball task, and their effects on ERP components. Int. J. Psychophysiol. 47, 197–198.
Barry, R. J., Rushby, J. A., Johnstone, S. J., Clark, A. R., Croft, R. J., and Lawrence, C. A. (2004). Event-related potentials in the auditory oddball as a function of EEG alpha phase at stimulus onset. Clin. Neurophysiol. 115, 2593–2601.
Bauer, F., Cheadle, S. W., Parton, A., Muller, H. J., and Usher, M. (2009). Gamma flicker triggers attentional selection without awareness. Proc. Natl. Acad. Sci. U.S.A. 106, 1666–1671.
Becker, R., Ritter, P., and Villringer, A. (2008). Influence of ongoing alpha rhythm on the visual evoked potential. Neuroimage 39, 707–716.
Ben-Simon, E., Podlipsky, I., Arieli, A., Zhdanov, A., and Hendler, T. (2008). Never resting brain: simultaneous representation of two alpha related processes in humans. PLoS ONE 3, e3984. doi: 10.1371/journal.pone.0003984
Berger, H. (1929). über das Elektroenkephalogramm des Menschen. Arch. Psychiatr. Nervenkr. 87, 527–570.
Besle, J., Schevon, C. A., Mehta, A. D., Lakatos, P., Goodman, R. R., McKhann, G. M., Emerson, R. G., and Schroeder, C. E. (2011). Tuning of the human neocortex to the temporal dynamics of attended events. J. Neurosci. 31, 3176–3185.
Bishop, G. H. (1933). Cyclic change in the excitability of the optic pathway of the rabbit. Am. J. Physiol. 103, 213–224.
Bollimunta, A., Chen, Y., Schroeder, C. E., and Ding, M. (2008). Neuronal mechanisms of cortical alpha oscillations in awake-behaving macaques. J. Neurosci. 28, 9976–9988.
Bollimunta, A., Mo, J., Schroeder, C. E., and Ding, M. (2011). Neuronal mechanisms and attentional modulation of corticothalamic alpha oscillations. J. Neurosci. 31, 4935–4943.
Brandt, M. E., Jansen, B. H., and Carbonari, J. P. (1991). Pre-stimulus spectral EEG patterns and the visual evoked response. Electroencephalogr. Clin. Neurophysiol. 80, 16–20.
Buhusi, C. V., and Meck, W. H. (2005). What makes us tick? Functional and neural mechanisms of interval timing. Nat. Rev. Neurosci. 6, 755–765.
Busch, N. A., Dubois, J., and VanRullen, R. (2009). The phase of ongoing EEG oscillations predicts visual perception. J. Neurosci. 29, 7869–7876.
Busch, N. A., and VanRullen, R. (2010). Spontaneous EEG oscillations reveal periodic sampling of visual attention. Proc. Natl. Acad. Sci. U.S.A. 107, 16048–16053.
Callaway, E. III, and Yeager, C. L. (1960). Relationship between reaction time and electroencephalographic alpha phase. Science 132, 1765–1766.
Capotosto, P., Babiloni, C., Romani, G. L., and Corbetta, M. (2009). Frontoparietal cortex controls spatial attention through modulation of anticipatory alpha rhythms. J. Neurosci. 29, 5863–5872.
Cardin, J. A., Cerlén, M., Meletis, K., Knoblich, U., Zhang, F., Deisseroth, K., Tsai, L. H., and Moore, C. I. (2009). Driving fast-spiking cells induces gamma rhythm and controls sensory responses. Nature 458, 663–667.
Carp, J., and Compton, R. J. (2009). Alpha power is influenced by performance errors. Psychophysiology 46, 336–343.
Cavanagh, J. F., Cohen, M. X., and Allen, J. J. B. (2009). Prelude to and resolution of an error: EEG phase synchrony reveals cognitive control dynamics during action monitoring. J. Neurosci. 29, 98–105.
Chang, H. T. (1951). Changes in excitability of cerebral cortex following single electric shock applied to cortical surface. J. Neurophysiol. 14, 95–111.
Coan, J. A., and Allen, J. J. B. (2004). Frontal EEG asymmetry as a moderator and mediator of emotion. Biol. Psychol. 67, 7–49.
Cohen, M. X. (2008). Assessing transient cross-frequency coupling in EEG data. J. Neurosci. Methods 168, 494–499.
Cohen, M. X., Elger, C. E., and Ranganath, C. (2007). Reward expectation modulates feedback-related negativity and EEG spectra. Neuroimage 35, 968–978.
Cooper, N. R., Croft, R. J., Dominey, S. J. J., Burgess, A. P., and Gruzelier, J. H. (2003). Paradox lost? Exploring the role of alpha oscillations during externally vs. internally directed attention and the implications for idling and inhibition hypotheses. Int. J. Psychophysiol. 47, 65–74.
Corbetta, M., Patel, P., and Shulman, G. L. (2008). The reorienting system of the human brain: from environment to theory of mind. Neuron 58, 306–324.
Corbetta, M., and Shulman, G. L. (2002). Control of goal-directed and stimulus-driven attention in the brain. Nat. Rev. Neurosci. 3, 201–215.
Coull, J. T., and Nobre, A. C. (1998). Where and when to pay attention: the neural systems for directing attention to spatial locations and to time intervals as revealed by both PET and fMRI. J. Neurosci. 18, 7426–7435.
Coull, J. T., Vidal, F., Nazarian, B., and Macar, F. (2004). Functional anatomy of the attentional modulation of time estimation. Science 303, 1506–1508.
Del Percio, C., Marzano, N., Tilgher, S., Fiore, A., Di Ciolo, E., Aschieri, P., Lino, A., Toràn, G., Babiloni, C., and Eusebi, F. (2007). Pre-stimulus alpha rhythms are correlated with post-stimulus sensorimotor performance in athletes and non-athletes: a high-resolution EEG study. Clin. Neurophysiol. 118, 1711–1720.
Desimone, R., and Duncan, J. (1995). Neural mechanisms of selective visual attention. Annu. Rev. Neurosci. 18, 193–222.
Donchin, E., Fabiani, M., and Sanders, A. F. (eds). (1989). The Learning Strategies Program: An Examination of the Strategies in Skill Acquisition. Amsterdam: Elsevier. [Published as Vol. 71 of Acta Psychologica].
Drews, J., and VanRullen, R. (2011). This is the rhythm of your eyes: the phase of ongoing electroencephalogram oscillations modulates saccadic reaction time. J. Neurosci. 31, 4698–4708.
Dustman, R. E., and Beck, E. C. (1965). Phase of alpha brain waves, reaction time and visually evoked potentials. Electroencephalogr. Clin. Neurophysiol. 1965, 433–440.
Efron, R. (1970b). The relationship between the duration of a stimulus and the duration of a perception. Neuropsychologia 8, 37–55.
Ergenoglu, T., Demiralp, T., Bayraktaroglu, Z., Ergen, M., Beydagi, H., and Uresin, Y. (2004). Alpha rhythm of the EEG modulates visual detection performance in humans. Brain Res. Cogn. Brain Res. 20, 376–383.
Gao, L., Meng, X., Ye, C., Zhang, H., Liu, C., Dan, Y., Poo, M.-M., He, J., and Zhang, X. (2009). Entrainment of slow oscillations of auditory thalamic neurons by repetitive sound stimuli. J. Neurosci. 29, 6013–6021.
Gho, M., and Varela, F. J. (1988). A quantitative assessment of the dependency of the visual temporal frame upon the cortical rhythm. J. Physiol. (Paris) 83, 95–101.
Gratton, G., and Fabiani, M. (2010). Fast optical imaging of human brain function. Front. Hum. Neurosci. 4:52. doi: 10.3389/fnhum.2010.00052
Gratton, G., Villa, A. E. P., Fabiani, M., Colombis, G., Palin, E., Bolcioni, G., and Fiori, M. G. (1992). Functional correlates of a three-component spatial model of the alpha rhythm. Brain Res. 582, 159–162.
Haig, A. R., and Gordon, E. (1998). EEG alpha phase at stimulus onset significantly affects the amplitude of the P3 ERP component. Int. J. Neurosci. 93, 101–116.
Hamidi, M., Slagter, H. A., Tononi, G., and Postle, B. R. (2009). Repetitive transcranial magnetic stimulation affects behavior by biasing endogenous cortical oscillations. Front. Integr. Neurosci. 3:14. doi: 10.3389/neuro.07.014.2009
Hamm, J. P., Dyckman, K. A., Ethridge, L. E., McDowell, J. E., and Clementz, B. A. (2010). Preparatory activations across a distributed cortical network determine production of express saccades in humans. J. Neurosci. 30, 7350–7357.
Hanslmayr, S., Aslan, A., Staudigl, T., Klimesch, W., Herrmann, C. S., and Bäuml, K.-H. (2007). Prestimulus oscillations predict visual perception performance between and within subjects. Neuroimage 37, 1465–1473.
Herrmann, C. S. (2001). Human EEG responses to 1-100 Hz flicker: resonance phenomena in visual cortex and their potential correlation to cognitive phenomena. Exp. Brain Res. 137, 346–353.
Hoogenboom, N., and Romei, V. (2010). Probing different time-scales of oscillatory fluctuations in visual awareness: from behavior to phase. Front. Hum. Neurosci. 4:178. doi: 10.3389/fnhum.2010.00178
Jacobs, J., Kahana, M. J., Ekstrom, A. D., and Fried, I. (2007). Brain oscillations control timing of single-neuron activity in humans. J. Neurosci. 27, 3839–3844.
Jann, K., Koenig, T., Dierks, T., Boesch, C., and Federspiel, A. (2010). Association of individual resting state EEG alpha frequency and cerebral blood flow. Neuroimage 51, 365–372.
Jansen, B. H., and Brandt, M. E. (1991). The effect of the phase of prestimulus alpha activity on the averaged visual evoked response. Electroencephalogr. Clin. Neurophysiol. 80, 241–250.
Jensen, O., Gelfand, J., Kounios, J., and Lisman, J. E. (2002). Oscillations in the alpha band (9-12 Hz) increase with memory load during retention in a short-term memory task. Cereb. Cortex 12, 877–882.
Jensen, O., and Mazaheri, A. (2010). Shaping functional architecture by oscillatory alpha activity: gating by inhibition. Front. Hum. Neurosci. 4:186. doi: 10.3389/fnhum.2010.00186
Johnson, J. S., Hamidi, M., and Postle, B. R. (2010). Using EEG to explore how rTMS produces its effects on behavior. Brain Topogr. 22, 281–293.
Jones, M. R. (1976). Time, our lost dimension: toward a new theory of perception, attention, and memory. Psychol. Rev. 83, 323–355.
Jones, M. R., Moynihan, H., MacKenzie, N., and Puente, J. (2002). Temporal aspects of stimulus driven attending in dynamic arrays. Psychol. Sci. 13, 313–319.
Kelly, S. P., Lalor, E. C., Reilly, R. B., and Foxe, J. J. (2006). Increases in alpha oscillatory power reflect an active retinotopic mechanism for distracter suppression during sustained visuospatial attention. J. Neurophysiol. 95, 3844–3851.
Kerlin, J. R., Shahin, A. J., and Miller, L. M. (2010). Attentional gain control of ongoing cortical speech representations in a “cocktail party.” J. Neurosci. 30, 620–628.
Klimesch, W., Doppelmayr, M., Russegger, H., Pachinger, T., and Schwaiger, J. (1998). Induced alpha band power changes in the human EEG and attention. Neurosci. Lett. 224, 73–76.
Klimesch, W., Sauseng, P., and Gruber, W. (2009). The functional relevance of phase reset: a comment to Risner et al. (2009): the visual evoked potential of surface alpha rhythm phase. Neuroimage 47, 5–7.
Klimesch, W., Sauseng, P., and Hanslmayr, S. (2007). EEG alpha oscillations: the inhibition-timing hypothesis. Brain Res. Rev. 53, 63–88.
Kline, K., Holcombe, A., and Eagleman, D. M. (2004). Illusory motion reversal is caused by rivalry, not by perceptual snapshots of the visual field. Vision Res. 44, 2653–2658.
Kruglikov, S. Y., and Schiff, S. J. (2003). Interplay of electroencephalogram phase and auditory-evoked neural activity. J. Neurosci. 23, 10122–10127.
Lakatos, P., Chen, C.-M., O’Connell, M. N., Mills, A., and Schroeder, C. E. (2007). Neuronal oscillations and multisensory interaction in primary auditory cortex. Neuron 53, 279–292.
Lakatos, P., Karmos, G., Mehta, A. D., Ulbert, I., and Schroeder, C. E. (2008). Entrainment of neuronal oscillations as a mechanism of attentional selection. Science 320, 110–113.
Large, E. W., and Jones, M. R. (1999). The dynamics of attending: how people track time-varying events. Psychol. Rev. 106, 119–159.
Levinthal, B. R., and Lleras, A. (2008). Context-free inhibition: attentional biases transfer strongly across temporal and spatial search tasks. Vis. cogn. 16, 1119–1123.
Lindsley, D. B. (1952). Psychological phenomena and the electroencephalogram. Electroencephalogr. Clin. Neurophysiol. 4, 443–456.
Linkenkaer-Hansen, K., Nikulin, V. V., Palva, S., Ilmoniemi, R. J., and Palva, J. M. (2004). Prestimulus oscillations enhance psychophysical performance in humans. J. Neurosci. 24, 10186–10190.
Lopes Da Silva, F. H. (1991). Neural mechanisms underlying brain waves: from neural membranes to networks. Electroencephalogr. Clin. Neurophysiol. 79, 81–93.
Lopes Da Silva, F. H., Van Lierop, T. H., Schrijer, C. F., and Van Leeuwen, W. S. (1973). Organization of thalamic and cortical alpha rhythms: spectra and coherences. Electroencephalogr. Clin. Neurophysiol. 35, 627–639.
Lopes Da Silva, F. H., Vos, J. E., Mooibroek, J., and Van Rotterdam, A. (1980). Relative contributions of intracortical and thalamo-cortical processes in the generation of alpha rhythms, revealed by partial coherence analysis. Electroencephalogr. Clin. Neurophysiol. 50, 449–456.
Lörincz, M. L., Kékesi, K. A., Juhász, G., Crunelli, V., and Hughes, S. W. (2009). Temporal framing of thalamic relay-mode firing by phasic inhibition during the Alpha rhythm. Neuron 63, 683–696.
Luck, S. J. (2009). “The spatiotemporal dynamics of visual-spatial attention,” in From Attention to Goal-Directed Behavior: Neurodynamical, Methodological, and Clinical Trends, eds F. Aboitizand and D. Cosmelli (Berlin: Springer), 51–66.
Maclin, E., Mathewson, K. E., Low, K. A., Boot, W. R., Kramer, A. F., Fabiani, M., and Gratton, G. (in press). Learning to multitask: electrophysiological indices of attention during video game training. Psychophysiology. doi: 10.1111/j.1469-8986.2011.01189.x
Martin, E. W., Enns, J. T., and Shapiro, K. L. (2011). Turning the attentional blink on and off: opposing effects of spatial and temporal noise. Psychon. Bull. Rev. 18, 295–301.
Mathewson, K. E., Fabiani, M., Gratton, G., Beck, D. M., and Lleras, A. (2010). Rescuing stimuli from invisibility: inducing a momentary release from visual masking with pre-target entrainment. Cognition 115, 186–191.
Mathewson, K. E., Gratton, G., Fabiani, M., Beck, D. M., and Ro, T. (2009). To see or not to see: Prestimulus alpha phase predicts visual awareness. J. Neurosci. 29, 2725–2732.
Mazaheri, A., DiQuattro, N. E., Bengson, J., and Geng, J. J. (2011). Pre-stimulus activity predicts the winner of top-down vs. bottom-up attentional selection. PLoS ONE 6, e16243. doi: 10.1371/journal.pone.0016243
Mazaheri, A., and Jensen, O. (2006). Posterior alpha activity is not phase reset by visual stimuli. Proc. Natl. Acad. Sci. U.S.A. 103, 2948–2952.
Mazaheri, A., and Jensen, O. (2008). Asymmetric amplitude modulations of brain oscillations can generate slow evoked responses. J. Neurosci. 28, 7781–7787.
Mazaheri, A., and Jensen, O. (2010). Rhythmic pulsing: linking ongoing brain activity with evoked responses. Front. Hum. Neurosci. 4:177. doi: 10.3389/fnhum.2010.00177
Mazaheri, A., Nieuwenhuis, I. L. C., Van Dijk, H., and Jensen, O. (2009). Prestimulus alpha and mu activity predicts failure to inhibit motor responses. Hum. Brain Mapp. 30, 1791–1800.
Min, B.-K., and Herrmann, C. S. (2007). Prestimulus EEG alpha activity reflects prestimulus top-down processing. Neurosci. Lett. 422, 131–135.
Min, B.-K., Park, J. Y., Kim, E. J., Kim, J. I., Kim, J.-J., and Park, H.-J. (2008). Prestimulus EEG alpha activity reflects temporal expectancy. Neurosci. Lett. 438, 270–274.
Mitchell, J. F., Sundberg, K. A., and Reynolds, J. H. (2009). Spatial attention decorrelates intrinsic activity fluctuations in macaque area V4. Neuron 63, 879–888.
Mo, J., Schroeder, C. E., and Ding, M. (2011). Attentional modulation of alpha oscillations in macaque inferotemporal cortex. J. Neurosci. 31, 878–882.
Monto, S., Palva, S., Voipio, J., and Palva, J. M. (2008). Very slow EEG fluctuations predict the dynamics of stimulus detection and oscillation amplitudes in humans. J. Neurosci. 28, 8268–8272.
Moosmann, M., Ritter, P., Krastel, I., Brink, A., Thees, S., Blankenburg, F., Taskin, B., Obrig, H., and Villringer, A. (2003). Correlates of alpha rhythm in functional magnetic resonance imaging and near infrared spectroscopy. Neuroimage 20, 145–158.
Morgan, S. T., Hansen, J. C., and Hillyard, S. A. (1996). Selective attention to stimulus location modulates the steady-state visual evoked potential. Proc. Natl. Acad. Sci. U.S.A. 93, 4770–4774.
Müller, M. M., Teder-Sälejärvi, W., and Hillyard, S. (1998). The time course of cortical facilitation during cued shifts of spatial attention. Nat. Neurosci. 7, 631–634.
Nunn, C. M., and Osselton, J. W. (1974). The influence of the EEG alpha rhythm on the perception of visual stimuli. Psychophysiology 11, 294–303.
Osipova, D., Hermes, D., and Jensen, O. (2008). Gamma power is phase-locked to posterior alpha activity. PLoS ONE 3, e3990. doi: 10.1371/journal.pone.0003990
Ozen, S., Sirota, A., Belluscio, M. A., Anastassiou, C. A., Stark, E., Koch, C., and Buzsáki, G. (2010). Transcranial electric stimulation entrains cortical neuronal populations in rats. J. Neurosci. 30, 11476–11485.
Palva, J. M., Monto, S., Kulashekhar, S., and Palva, S. (2010). Neuronal synchrony reveals working memory networks and predicts individual memory capacity. Proc. Natl. Acad. Sci. U.S.A. 107, 7580–7585.
Palva, S., Linkenkaer-Hansen, K., Näätänen, R., and Palva, J. M. (2005a). Early neural correlates of conscious somatosensory perception. J. Neurosci. 25, 5248–5258.
Palva, J. M., Palva, S., and Kaila, K. (2005b). Phase synchrony among neuronal oscillations in the human cortex. J. Neurosci. 25, 3962–3972.
Palva, S., and Palva, J. M. (2007). New vistas for a-frequency band oscillations. Trends Neurosci. 30, 150–158.
Pastor, M. A., Artieda, J., Arbizu, J., Marti-Climent, J. M., Penuelas, I., and Masdeu, J. C. (2002). Activation of human cerebral and cerebellar cortex by auditory stimulation at 40 Hz. J. Neurosci. 22, 10501–10506.
Pastor, M. A., Artieda, J., Arbizu, J., Valencia, M., and Masdeu, J. C. (2003). Human cerebral activation during steady-state visual-evoked responses. J. Neurosci. 23, 11621–11627.
Pérez, A., Peers, P. V., Valdés-Sosa, M., Galán, L., García, L., and Martínez-Montes, E. (2009). Hemispheric modulations of alpha-band power reflect the rightward shift in attention induced by enhanced attentional load. Neuropsychologia 47, 41–49.
Pfurtscheller, G., and Neuper, C. (1994). Event-related synchronization of mu rhythm in the EEG over the cortical hand area in man. Neurosci. Lett. 174, 93–96.
Pogosyan, A., Gaynor, L. D., Eusebio, A., and Brown, P. (2009). Boosting cortical activity at beta-band frequencies slows movements in humans. Curr. Biol. 19, 1637–1641.
Pöppel, E. (2009). Pre-semantically defined temporal windows for cognitive processing. Philos. Trans. R. Soc. Lond. B Biol. Sci. 364, 1887–1896.
Posner, M. I., and Petersen, S. E. (1990). The attention system of the human brain. Annu. Rev. Neurosci. 13, 25–42.
Rajagovindan, R., and Ding, M. (2010). From prestimulus alpha oscillation to visual-evoked response: an inverted-u function and its attentional modulation. J. Cogn. Neurosci. 16, 1379–1394.
Ray, W. J., and Cole, H. W. (1985). EEG alpha activity reflects attentional demands, and beta activity reflects emotional and cognitive processes. Science 228, 750–752.
Raymond, J. E., Shapiro, K. L., and Arnell, K. M. (1992). Temporary suppression of visual processing in an RSVP task: an attentional blink? J. Exp. Psychol. Hum. 18, 849–860.
Reinacher, M., Becker, R., Villringer, A., and Ritter, P. (2009). Oscillatory brain states interact with late cognitive components of the somatosensory evoked potential. J. Neurosci. Methods 183, 49–56.
Rice, D. M., and Hagstrom, E. C. (1989). Some evidence in support of a relationship between human auditory signal-detection performance and the phase of the alpha cycle. Percept. Mot. Skills 69, 451–457.
Rihs, T., Michel, C. M., and Thut, G. (2007). Mechanisms of selective inhibition in visual spatial attention are indexed by alpha-band EEG synchronization. Eur. J. Neurosci. 25, 603–610.
Rihs, T., Michel, C. M., and Thut, G. (2009). A bias for posterior alpha-band power suppression versus enhancement during shifting versus maintenance of spatial attention. Neuroimage 44, 190–199.
Risner, M. L., Aura, C. J., Black, J. E., and Gawne, T. J. (2009). The visual evoked potential is independent of surface alpha rhythm phase. Neuroimage 45, 463–469.
Ro, T., Breitmeyer, B., Burton, P., Singhal, N. S., and Lane, D. (2003). Feedback contributions to visual awareness in human occipital cortex. Curr. Biol. 13, 1038–1041.
Reynolds, J. H., and Heeger, D. J. (2009). The normalization model of attention. Neuron 61, 168–185.
Romei, V., Brodbeck, V., Michel, C., Amedi, A., Pascual-Leone, A., and Thut, G. (2008a). Spontaneous fluctuations in posterior alpha-band EEG activity reflect variability in excitability of human visual areas. Cereb. Cortex 18, 2010–2018.
Romei, V., Rihs, T., Brodbeck, V., and Thut, G. (2008b). Resting EEG alpha-power over posterior sites indexes baseline visual cortex excitability. Neuroreport 19, 203–208.
Romei, V., Gross, J., and Thut, G. (2010). On the role of prestimulus alpha rhythms over occipito-parietal areas in visual input regulation: correlation or causation? J. Neurosci. 30, 8692–8697.
Rosenfeld, J. P., Reinhart, A. M., and Srivastava, S. (1997). The effects of alpha (10-Hz) and beta (22-Hz) “entrainment” stimulation on the alpha and beta EEG bands: individual differences are critical to prediction of effects. Appl. Psychophysiol. Biofeedback 22, 3–20.
Sachdev, R. N. S., Ebner, F. F., and Wilson, C. J. (2004). Effect of subthreshold up and down states on the whisker-evoked response in somatosensory cortex. J. Neurophysiol. 92, 3511–3521.
Sadaghiani, S., Scheeringa, R., Lehongre, K., Morillon, B., Giraud, A. L., and Kleinschmidt, A. (2010). Intrinsic connectivity networks, alpha oscillations, and tonic alertness: a simultaneous electroencephalography/functional magnetic resonance imaging study. J. Neurosci. 30, 10243–10250.
Sauseng, P., Klimesch, W., Gerloff, C., and Hummel, F. C. (2009). Spontaneous locally restricted EEG alpha activity determines cortical excitability in the motor cortex. Neuropsychologia 47, 284–288.
Sauseng, P., Klimesch, W., Stadler, W., Schabus, M., Doppelmayr, M., Hanslmayr, S., Gruber, W. R., and Birbaumer, N. (2005). A shift of visual spatial attention is selectively associated with human EEG alpha activity. Neuroscience 22, 2917–2926.
Scheeringa, R., Mazaheri, A., Bojak, I., Norris, D. G., and Kleinschmidt, A. (2011). Modulation of visually evoked cortical fMRI responses by phase of ongoing occipital alpha oscillations. J. Neurosci. 31, 3813–3820.
Schmidt-Kassow, M., and Kotz, S. A. (2008). Entrainment of syntactic processing? ERP-responses to predictable time intervals during syntactic reanalysis. Brain Res. 1226, 144–155.
Schmidt-Kassow, M., Schubotz, R. I., and Kotz, S. A. (2009). Attention and entrainment: P3b varies as a function of temporal predictability. Neuroreport 20, 31–36.
Schroeder, C. E., and Lakatos, P. (2009). Low-frequency neuronal oscillations as instruments of sensory selection. Trends Neurosci. 32, 9–18.
Schroeder, C. E., Lakatos, P., Kajikawa, Y., Partan, S., and Puce, A. (2008). Neuronal oscillations and visual amplification of speech. Trends Cogn. Sci. 12, 106–113.
Simpson, W., Shahani, U., and Manahilov, V. (2005). Illusory percepts of moving patterns due to discrete temporal sampling. Neurosci. Lett. 375, 23–27.
Smith, M. L., Gosselin, F., and Schyns, P. G. (2006). Perceptual moments of conscious visual experience inferred from oscillatory brain activity. Proc. Natl. Acad. Sci. U.S.A. 103, 5626–5231.
Snyder, A. C., and Foxe, J. J. (2010). Anticipatory attentional suppression of visual features indexed by oscillatory alpha-band power increases: a high-density electrical mapping study. J. Neurosci. 30, 4024–4032.
Stefanics, G., Hangya, B., Hernádi, I., Winkler, I., Lakatos, P., and Ulbert, I. (2010). Phase entrainment of human delta oscillations can mediate the effects of expectation on reaction speed. J. Neurosci. 30, 13578–13585.
Steriade, M., Gloor, P., Llinas, R., Lopes Da Silva, F., and Mesulam, M. (1990). Basic mechanisms of cerebral rhythmic activities. Electroencephalogr. Clin. Neurophysiol. 76, 481–508.
Tallon-Baudry, C., and Bertrand, O. (1999). Oscillatory gamma activity in humans and its role in object representation. Trends Cogn. Sci. 3, 151–162.
Temereanca, S., Brown, E. N., and Simons, D. J. (2008). Rapid changes in thalamic firing synchrony during repetitive whisker stimulation. J. Neurosci. 28, 11153–11164.
Thut, G., and Miniussi, C. (2009). New insights into rhythmic brain activity from TMS–EEG studies. Trends Cogn. Sci. 13, 182–189.
Thut, G., Nietzel, A., Brandt, S., and Pacual-Leone, A. (2006). Alpha-band electroencephalographic (EEG) activity over occipital cortex indexes visuospatial attention bias and predicts visual target detection. J. Neurosci. 26, 9494–9502.
Trimble, J. L., and Potts, A. M. (1975). Ongoing occipital rhythms and the VER. I. Stimulation at peaks of the alpha-rhythm. Invest. Ophthalmol. 14, 537–546.
Van Dijk, H., Schoffelen, J.-M., Oostenveld, R., and Jensen, O. (2008). Prestimulus oscillatory activity in the alpha band predicts visual discrimination ability. J. Neurosci. 28, 1816–1823.
Van Dijk, H., Van Der Werf, J., Mazaheri, A., Medendorp, W. P., and Jensen, O. (2010). Modulations in oscillatory activity with amplitude asymmetry can produce cognitively relevant event-related responses. Proc. Natl. Acad. Sci. U.S.A. 107, 900–905.
VanRullen, R., and Koch, C. (2003). Is perception discrete or continuous? Trends Cogn. Sci. 7, 207–213.
VanRullen, R., Busch, N. A., Drewes, J., and Dubois, J. (2011). Ongoing EEG phase as a trial-by-trial predictor of perceptual and attentional variability. Front. Psychology 2:60. doi: 10.3389/fpsyg.2011.00060
VanRullen, R., Reddy, L., and Koch, C. (2005). Attention-driven discrete sampling of motion perception. Proc. Natl. Acad. Sci. U.S.A. 102, 5291–5296.
VanRullen, R., Reddy, L., and Koch, C. (2006). The continuous wagon wheel illusion is associated with changes in electroencephalogram power at approximately 13 Hz. J. Neurosci. 26, 502–507.
Varela, F. J., Toro, A., John, E. R., and Schwartz, E. L. (1981). Perceptual framing and cortical alpha rhythm. Neuropsychologia 19, 675–686.
Voytek, B., Canolty, R. T., Shestyuk, A., Crone, N. E., Parvizi, J., and Knight, R. T. (2010). Shifts in gamma phase-amplitude coupling frequency from theta to alpha over posterior cortex during visual tasks. Front. Hum. Neurosci. 4:191. doi: 10.3389/fnhum.2010.00191
Walter, V. J., and Walter, W. G. (1949). The central effects of rhythmic sensory stimulation. Electroencephalogr. Clin. Neurophysiol. 1, 57–86.
Williams, P. E., Mechler, F., Gordon, J., Shapley, R., and Hawken, M. J. (2004). Entrainment to video displays in primary visual cortex of macaque and humans. J. Neurosci. 24, 8278–8288.
Worden, M. S., Foxe, J. J., Wang, N., and Simpson, G. V. (2000). Anticipatory biasing of visuospatial attention indexed by retinotopically specific alpha-band electroencephalography increases over occipital cortex. J. Neurosci. 20, RC63.
Wyart, V., and Sergent, C. (2009). The phase of ongoing eeg oscillations uncovers the fine temporal structure of conscious perception. J. Neurosci. 29, 12839–12841.
Wyart, V., and Tallon-Baudry, C. (2009). How ongoing fluctuations in human visual cortex predict perceptual awareness: baseline shift versus decision bias. J. Neurosci. 29, 8715–8725.
Yashar, A., and Lamy, D. (2010). Intertrial repetition facilitates selection in time: common mechanisms underlie spatial and temporal search. Psychol. Sci. 21, 243–251.
Keywords: alpha, oscillation, phase, pulsed-inhibition, EEG
Citation: Mathewson KE, Lleras A, Beck DM, Fabiani M, Ro T and Gratton G (2011) Pulsed out of awareness: EEG alpha oscillations represent a pulsed-inhibition of ongoing cortical processing. Front. Psychology 2:99. doi: 10.3389/fpsyg.2011.00099
Received: 15 March 2011;
Accepted: 03 May 2011;
Published online: 19 May 2011.
Edited by:
Gregor Thut, University of Glasgow, UKReviewed by:
Michael X. Cohen, University of Amsterdam, NetherlandsAli Mazaheri, University of California Davis, USA
Copyright: © 2011 Mathewson, Lleras, Beck, Fabiani, Ro and Gratton. This is an open-access article subject to a non-exclusive license between the authors and Frontiers Media SA, which permits use, distribution and reproduction in other forums, provided the original authors and source are credited and other Frontiers conditions are complied with.
*Correspondence: Kyle E. Mathewson, Department of Psychology, Beckman Institute, University of Illinois at Urbana-Champaign, 405 North Mathews Avenue, Urbana, IL 61801, USA. e-mail: kylemath@gmail.com