- 1 Department of Psychology, Tufts University, Medford, MA, USA
- 2 Department of Psychology, The University of Memphis, Memphis, TN, USA
- 3 Department of Brain and Cognitive Sciences, University of Rochester, Rochester, NY, USA
Recently, researchers have measured hand movements en route to choices on a screen to understand the dynamics of a broad range of psychological processes. We review this growing body of research and explain how manual action exposes the real-time unfolding of underlying cognitive processing. We describe how simple hand motions may be used to continuously index participants’ tentative commitments to different choice alternatives during the evolution of a behavioral response. As such, hand-tracking can provide unusually high-fidelity, real-time motor traces of the mind. These motor traces cast novel theoretical and empirical light onto a wide range of phenomena and serve as a potential bridge between far-reaching areas of psychological science – from language, to high-level cognition and learning, to social cognitive processes.
Traditional theories once viewed the mind’s cognitive and motor systems as functionally independent. A motor movement, therefore, was thought to be the uninteresting end-result of cognitive processing. It is becoming increasingly clear, however, that the dynamics of action do not simply reside in the aftermath of cognition. Rather, they are part and parcel with cognition, and the cognitive and motor systems are far more coextensive than previously imagined. The implication, then, is that the rich dynamics of a simple bodily movement, such as a hand movement, can leave powerful traces of internal cognitive processes.
The existence of such motor traces of the mind would represent an important advance because researchers have too often been forced to view the “black box” of cognition through off-line, indirect observation. By and large, we use inferences from stimulus characteristics and discrete behavioral outcomes, such as reaction times and errors, to generate rich accounts of mental phenomena. Although quite valuable, a major drawback to this approach is that behavioral outcomes provide relatively little information about how cognitive processing evolves over time and how multiple processes may temporally converge to drive ultimate responses. This presents a serious quandary because competing theoretical accounts in many domains make fine-grained predictions about what ought to be taking place across the time-course of mental activity – from perception, to language and high-level cognition, to social cognitive processes.
Movements of the hand, however, offer continuous streams of output that can reveal ongoing dynamics of processing, potentially capturing the mind in motion with fine-grained temporal sensitivity. Tracking eye movements has long been used for this purpose (e.g., Tanenhaus et al., 1995), but eye-tracking and hand-tracking each have their advantages (see Magnuson, 2005). For example, hand movements offer continuous streams of motor output whereas eye movements are typically comprised of discrete saccades. Here, we explain how continuous hand movements provide a powerful – yet cheap and readily obtainable – index of underlying cognitive processing as it unfolds across time. Unlike eye movements, hand movements are also able to occupy graded locations amidst multiple response locations at the same time. These fortuitous qualities of hand movements led Magnuson (2005, p. 9996), in a commentary on the seminal article by Spivey et al. (2005), to portend that such movements could be able to “address…some of the biggest questions facing cognitive science.”
The Link between Manual and Mental Dynamics
Classically, motor responses were seen as the end-result of a one-way route from perception → cognition → action; thus, they were thought to reveal little about cognitive processing (see Rosenbaum, 2005, for discussion). However, substantial behavioral evidence now indicates that cognition does not discretely collapse its processing onto movement execution. Instead, movement is continually updated by cognitive processing over time (Goodale et al., 1986). This is buttressed by neurophysiological evidence. Studies in humans, for example, suggest that the process of categorizing a visual stimulus immediately shares its ongoing results with the motor cortex to continuously guide a hand-movement response over time (e.g., Freeman et al., 2011). Further, human reaching movements suggest that multiple motor plans are prepared in parallel, and that these cascade over time into visually guided action (Song and Nakayama, 2006, 2008). Monkey studies show that the hand’s position and velocity are tightly yoked to ongoing changes in the firing of population codes within motor cortex (Paninski et al., 2004), and when a monkey must generate a hand movement based on a perceptual decision, these motor-cortical population codes are yoked to the evolution of the decision process (Cisek and Kalaska, 2005). These findings suggest that manual dynamics are intimately coextensive with mental dynamics, and therefore, hand movements that are sampled fast enough could potentially provide real-time read-outs of internal cognitive processing.
The recent tracking of hand movement en route to choices on a screen has opened up new avenues of investigation into a wide range of psychological phenomena. Hand trajectories recorded by a computer mouse, or sometimes a wireless remote or position sensor, have proven able to sensitively track participants’ tentative commitments to various choice alternatives over hundreds of milliseconds. As such, the emergence of a behavioral response can be sampled anywhere from 40 to 120 times per second. This permits insights into fine-grained temporal dynamics, providing unusually high-fidelity, real-time motor traces of the mind. Its sensitivity to these dynamics can reveal “hidden” cognitive states that are otherwise not availed by traditional measures (Song and Nakayama, 2006, 2008, 2009). More broadly, these real-time dynamics may be used to assess how multiple processes temporally interact and how multiple information sources weigh in on processing across hundreds of milliseconds, thereby resolving critical empirical questions and theoretical impasses across psychology (e.g., see Spivey, 2007).
Doing Psychology by Hand
Language
In language research, debate continues as to whether the language system is a discrete-symbolic architecture or whether it integrates multiple sources of information in parallel to continually constrain its operation. Considerable evidence for the parallel, integrative approach came from eye movements, but still some researchers argued that the discontinuity of eye-movement patterns could be accommodated by discrete-symbolic accounts. The link between manual and mental dynamics has recently served as compelling evidence that language processing is indeed, at multiple levels, gradient and parallel.
Spivey et al. (2005) asked participants to move the computer mouse from the bottom-center of the screen to the top-left or top-right corners. For instance, a picture of a candle appeared in the top-left and a picture of a candy appeared in the top-right while a spoken phrase was heard (e.g., “click the candle”). When the distractor object corresponded to a word whose initial set of phonemes overlapped with the spoken word (e.g., a picture of candy, for the spoken word, candle), hand movements showed a continuous attraction toward the distractor before settling into the correct alternative. Thus, the ongoing accrual of the spoken word partially activated multiple lexical representations (e.g., both candle and candy), causing movements to partially gravitate toward candy before settling into candle. This showed that the language system continually integrates the acoustic-phonetic input of a spoken word, triggering parallel competing representations that resolve over time. This permitted a discrimination among theoretical models previously not possible.
In some cases, however, comprehending spoken language is more difficult and would benefit from help of the visual system. Consider the following syntactically ambiguous sentence:
Put the apple on the towel in the box.
Typically, listeners resolve such ambiguities by incorporating available visual cues (e.g., is there an apple on a towel or an apple by itself?). In a series of studies, participants were given several objects on a screen while they heard a sentence instructing them to move one object to another. For example, hearing the above sentence, participants would use a computer mouse to click on an image displaying an apple on a towel, and to then drag it onto an image of a box. Before reaching the box, however, participants’ hand movements exhibited a continuous attraction toward the object associated with the incorrect syntactic parse (in this case, an empty towel). Consistent with analogous eye-tracking studies (e.g., Tanenhaus et al., 1995), the attraction effect was not seen when a second relevant object was present (e.g., an apple on a napkin). This provided strong support for constraint-based accounts of language processing by demonstrating partially active syntactic alternatives competing over time with a weighing-in of visual, contextual, and linguistic factors (Farmer et al., 2007a,b). Importantly, hand movements were able to reveal competition on a trial-by-trial basis, thereby evading longstanding concerns with analogous eye-movement results that were limited by discrete oculomotor output.
In some contexts, however, sentence processing may not flow so smoothly. For instance, Dale and Duran (2011) had participants verify simple statements by clicking on a “true” or “false” response. Statements involving a negation (e.g., “cats are not dogs”) led hand movements to exhibit sharp shifts in direction, initially pursuing one response and then rapidly redirecting toward the other, especially for negated true statements. Thus, during sentence processing, participants experienced an abrupt shift in their evolving decision. Classic work suggests that negation is an operator that rapidly reverses the truth/falsity of an interpretation and predicts shifts in cognitive dynamics, consistent with other work showing that hand movement may index such rapid transitions (e.g., Resulaj et al., 2009). These rapid cognitive shifts, however, could not be evidenced by traditional measures.
Social Cognition
Hand movements have also been critical in uncovering the temporal dynamics of social cognition. For example, they have provided new insights into social categorization. In one series of studies, participants were presented with sex-typical (e.g., masculine male) and sex-atypical (e.g., feminine male) faces and asked to categorize the target’s sex by clicking on a “male” or “female” response on the screen. During categorization of sex-atypical faces, hand trajectories were continuously attracted to the opposite sex-category before settling into the correct response. This was evidence that social categorization involves dynamic competition, where partially active category representations (male and female) continuously compete over time to stabilize onto one categorical outcome (Freeman et al., 2008; also see Dale et al., 2007). These findings led to a new model of social categorization, proposing that all available facial, vocal, and bodily cues (among other constraints) simultaneously weigh in on multiple partially active category representations, and that these dynamically evolve over time into stable categorical perceptions (Freeman and Ambady, 2011b).
This model was supported by further evidence from a similar paradigm with race categorization. Hand movements again showed continuous-attraction effects for atypical targets, but also revealed important dissociations in how the processing of White-specifying and Black-specifying cues evolves across the categorization time-course. Moreover, as the amount of racial ambiguity increased, hand trajectories showed corresponding increases in attraction toward the unselected race-category and increases in motor complexity. This showed that the competition inherent to social categorization sensitively increases and decreases with the amount of ambiguity challenging the perceptual system (Freeman et al., 2010). Further, hand movements have revealed not only that multiple facial cues are dynamically integrated, but also that cues across sensory modalities (facial and vocal cues) are dynamically integrated as well (Freeman and Ambady, 2011a). See Figure 1. Hand movements have also been used to temporally dissociate the integration of specific cues, such as facial shape and pigmentation (Freeman and Ambady, in press).
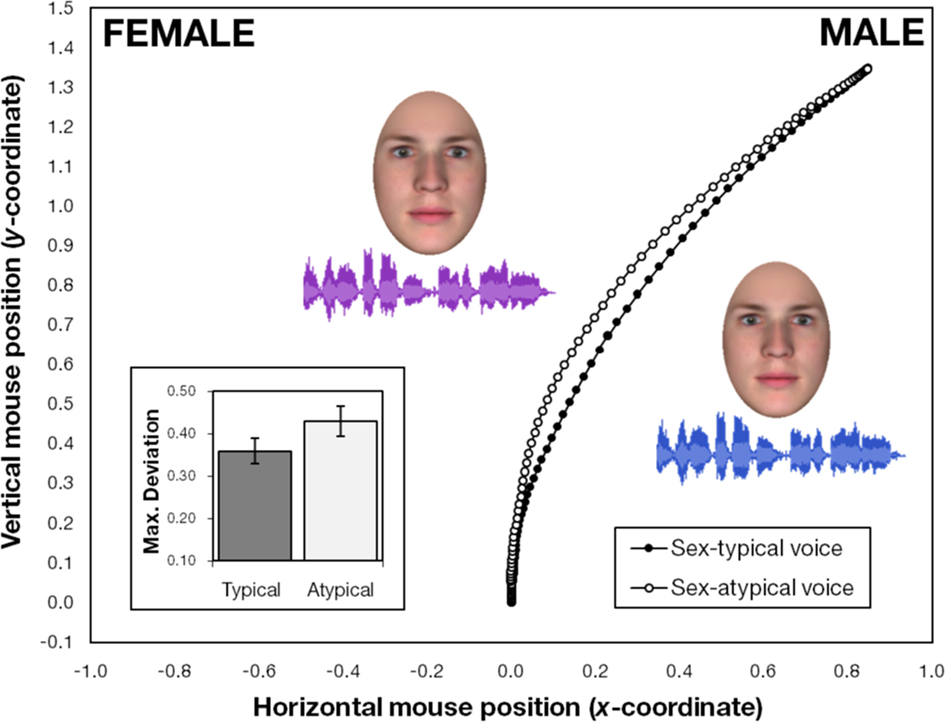
Figure 1. Hand movements reveal temporally dynamic face-voice integration. Freeman and Ambady (2011a) found that when categorizing a face’s sex, the simultaneous processing of a sex-atypical voice led participants’ mouse trajectories to be continuously attracted to the opposite sex-category response before settling into the response consistent with the face’s correct sex. Mean mouse trajectories from one study are depicted (aggregated across male and female targets). In this figure, trajectories for all targets were remapped rightward, with the opposite sex-category on the left and the sex-category consistent with the face’s sex on the right. A sample male face stimulus is displayed (all male and female face stimuli were somewhat sex-ambiguous). A voice stimulus typical for the face’s sex (masculine) is shown on the right (audio waveform depicted in blue), next to the mean trajectory for sex-typical trials. Its atypical (feminine) counterpart is shown on the left, next to the mean trajectory for sex-atypical trials (audio waveform depicted in purple). During an actual trial, a single face was centered at the bottom of the screen while the voice stimulus played. The bar graph shows trajectories’ maximum deviation toward the opposite sex-category from a direct line between trajectories’ start and end points (error bars denote SE of the mean). The continuous-attraction effect shows that ongoing voice-processing results were dynamically integrated with ongoing face-processing results over time. Adapted from Freeman and Ambady (2011a).
The competition during categorization can also bear downstream effects. For instance, Freeman and Ambady (2009) found that, before participants clicked on a stereotypically masculine attribute (e.g., aggressive) for male targets or stereotypically feminine attribute (e.g., caring) for female targets, their hands partially swerved toward the attribute stereotypically associated with the opposite sex when facial cues partly overlapped with that sex (e.g., masculine women and feminine men). Thus, not only do facial cues associated with an alternate category trigger a partially active representation of that category, but this partial activation cascades into the triggering of associated stereotypes. Hand movements can also reveal other types of partial parallel activations otherwise not seen by traditional measures. For example, although White participants almost always explicitly reported liking Black people, an inspection of the hand trajectories leading up to those “like” judgments revealed a parallel consideration to indicate that they “dislike” them (Wojnowicz et al., 2009). Such a parallel activation of multiple attitudes that continually coalesce into explicit judgments had important theoretical implications for models of social evaluation.
High-Level Cognition and Learning
Even high-level decision-making processes can be revealed in manual dynamics. McKinstry et al. (2008) had participants judge the truth of questions by moving their mouse to “yes” or “no” response options. Questions of greater uncertainty were answered with greater fluctuation than ones with greater certainty. Thus, hand movements revealed parallel competition across the process of high-level decision-making and showed how this competition is affected by certainty in graded fashion. Hand movements also revealed a strong confirmation bias throughout the response, because answers of low probability had overall slower trajectories.
Duran et al. (2010) used the same basic design, and revealed that this “truth bias” persists when people answer autobiographical questions falsely. After participants were presented with the final word, they were cued to respond either falsely or truthfully. Trajectories during false responses had greater sample entropy, reached peak velocity later in the movement, and had a steeper curve toward the competing option while en route to the false response. As such, hand trajectories revealed the ongoing process of “overcoming the truth” during deceptive responding. See Figure 2.
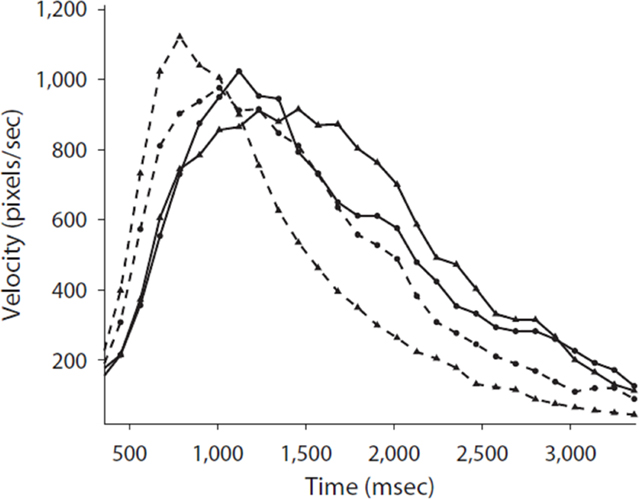
Figure 2. Hand movements provide insights into deception. Duran et al. (2010) had participants answer autobiographical questions either truthfully or falsely by clicking a “yes” or “no” response on the screen. Trajectories’ velocity profiles are plotted as a function of time for truthful responses clicking “no” (dashed line with circles), truthful responses clicking “yes” (dashed line with triangles), false responses clicking “no” (solid line with circles), and false responses clicking “yes” (solid line with triangles). Hand trajectories during false responses reached peak velocity later in the movement. This, among other differences between false and truthful responses in movement complexity and curvature toward the opposite response, suggests the presence of a truth bias that pulls processing off course during the production of a deceptive response. Adapted from Duran et al. (2010).
Finally, at an even longer timescale than decisions, Dale et al. (2008) studied how growing knowledge, across many trials, covaries with manual dynamics during a learning task. They had participants learn random pairs of shapes in a paired-associate memory design. Participants were shown a shape at the bottom of the screen, and instructed to pick one of two in the upper-most corners that was associated with that bottom shape. They were given feedback and gradually learned the pairings. The researchers found that hand movements (measured with a wireless Nintendo Wii remote) covaried in a gradient way with emerging knowledge of the pairs. Moreover, growing knowledge correlated with stronger force responding on the remote, and when new shapes appeared (unbeknownst to participants) this “bodily confidence” was modulated in response. Thus, hand movements revealed the longer timescale dynamics of the knowledge itself, as the participants exhibited learning across the task. Interestingly, at even longer timescales (e.g., hours), gesturing patterns of hand movement have long been used to index learning and transitions of knowledge, especially in children (Goldin-Meadow and Alibali, 2002).
Further Questions and Conclusions
The work discussed above suggests that the intimate link between manual and mental dynamics may be used to provide critical insights into psychological phenomena. It also emphasizes an integrative approach to these phenomena by demonstrating a deep coextension of processes typically investigated independently, such as action, perception, and both basic and social cognition. Insights from simple manual actions may therefore serve as an unforeseen bridge between far-reaching areas of psychological science.
This action-dynamics approach to the mind holds particular promise given the practicality of measuring hand movements. There now exists freely available, user-friendly software to run and analyze data from computer mouse-tracking experiments (Freeman and Ambady, 2010). Thus, rather than measure an ultimate response time, researchers can easily open up such responses into continuous streams of rich cognitive output through nothing more than a computer and mouse. Mouse-tracking also permits the recording of online mental processing in young children at an age where online data are often difficult to obtain (Anderson et al., in press). With the capturing of hand movements now just as available and affordable as recording the classic response time, we are hopeful that a growing number of studies will employ the methodology.
In this action-dynamics approach, researchers are afforded many ways to measure and analyze hand trajectories. The computer mouse is by far the most practical, but other options include a wireless remote (e.g., Dale et al., 2008) or a position sensor (e.g., Song and Nakayama, 2008). Although the techniques are quite similar, one notable difference is that mouse-tracking captures only 2D movement, whereas the others could potentially capture 3D movement. The techniques also provide different motoric experiences; for example, tasks using a wireless remote can measure large, free-ranging arm movements. Another consideration is that, in mouse-tracking, hand movements are stabilized by a surface. Moreover, mouse-tracking can be carried out through online data collection systems like Amazon Mechanical Turk (Dale and Duran, 2011). Once trajectories are recorded, several measures may be computed, including maximum deviation toward an unselected response (e.g., distractor), curvature area, switches in direction, or movement complexity. The time-course of the hand’s position, velocity, acceleration, or proximity to a selected or unselected response, can also be examined. Each analysis carries its own advantages with respect to the theoretical and practical interests at hand. Freeman and Ambady (2010) provide a more complete discussion of measures and analytic techniques for trajectory data. They also describe a user-friendly software package that permits the recording, measurement, and analysis of mouse trajectories from highly customizable experimental tasks.
Despite the headway made by the work reviewed here, however, the moving hand still has much to reveal about the mind. For example, in a recent critique of the method and its interpretation, van der Wel et al. (2009) discussed whether a model of discrete processing could produce, through (continuous) linear combinations of motor plans, the evidence for continuous processing often obtained from hand-tracking experiments. The authors argued that the inference of processing continuity from hand-tracking data is not yet fully warranted. In response, Spivey et al. (2010) argued that the measures drawn from hand trajectories are of sufficient richness that the proposed model of van der Wel et al. (2009) does not capture their full range of nuances. Nevertheless, both sets of authors agree that such debate is central to further understanding the manner in which cognition flows into action.
There is also not yet a clear understanding of the particular cognitive processes directly indexed by hand movements. For instance, several of the studies described here reported effects of motor competition, but one might wonder whether the competition may be mediated by attentional mechanisms (e.g., see Rizzolatti et al., 1987). Recent mouse-tracking work has found that such competitive effects can also reflect embodied cognitive processes leaking out into the motor system (Miles et al., 2010). In addition, one might wonder whether average graded attraction effects, like that seen in Figure 1, reflect parallel competing responses (e.g., parallel pursuits toward both “female” and “male”) or perhaps strategic response switching (e.g., an initial pursuit toward “female,” which is then corrected by a later pursuit toward “male”). Indeed, previous work found that hand movements can distinguish between these two possibilities. For example, Farmer et al. (2007b) demonstrated distinctly different hand-trajectory distributions for scenarios involving parallel competing responses versus scenarios involving strategic midflight corrections (see Figure 3). Freeman et al. (2008) provided a second demonstration of this in the more typical two-choice mouse-tracking design as well. In short, more work is needed to provide a comprehensive mapping of hand movements in these paradigms with specific underlying cognitive processes. Nevertheless, at this early stage of the action-dynamics approach, hand movements do show an unusually sensitive ability to tap into real-time cognition.
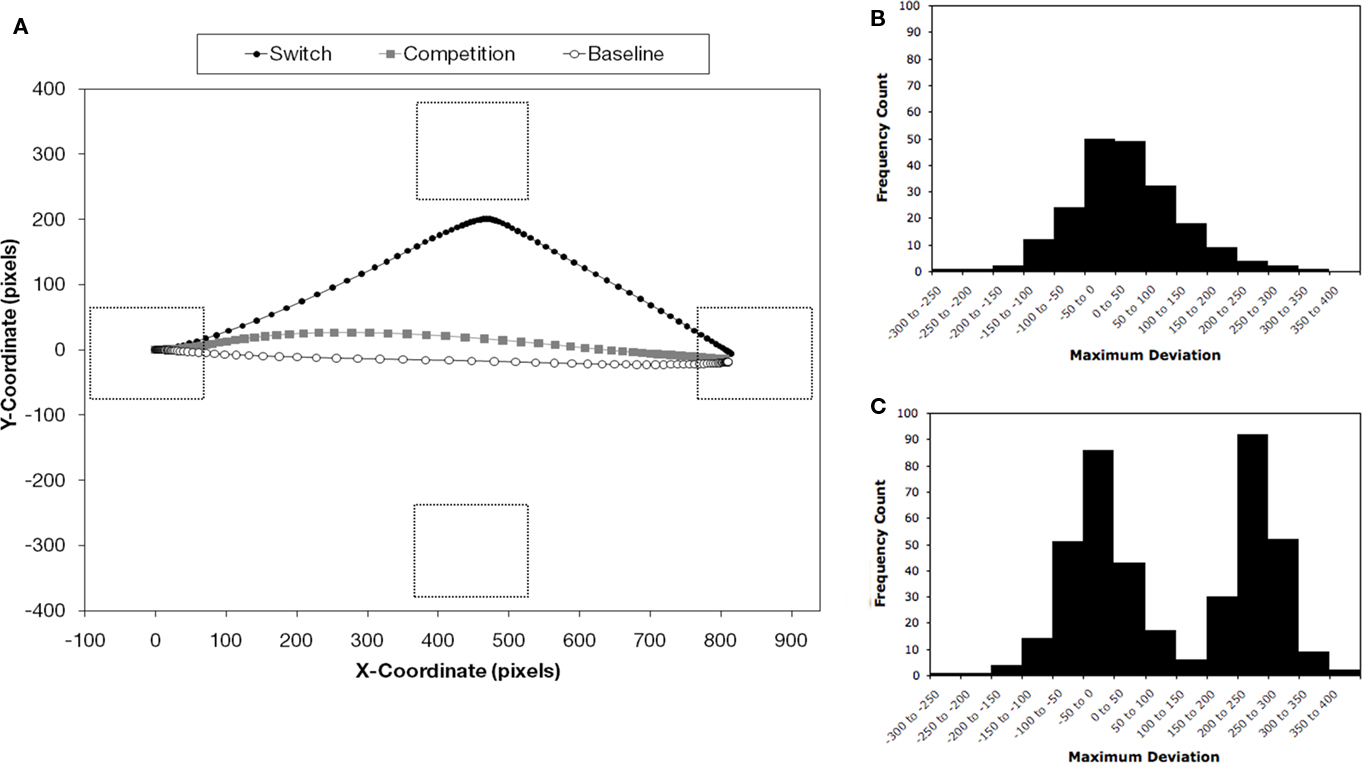
Figure 3. Parallel competition vs. strategic response switching. Farmer et al. (2007a) conducted an additional study where participants were presented with three colored squares and asked to click on the green square. In the baseline condition, the top and bottom squares were red and the rightward square was green, corresponding to a condition where no attraction to another object on the screen would be predicted. In the switch condition, the green square originally appeared at the top-center, with the other two squares being red. Once the cursor exited the leftward “Start” box, however, the green square turned red, and the leftward square that was originally red became green. This simulates a situation where participants initially commit to an incorrect response, and then subsequently switch their commitment to the correct response. In the competition condition, the bottom square was red, the rightward square was green, and the top-center square was a blue-green. Here, the blue-green square served as a distractor. (A) shows average trajectories. In the baseline condition, the average trajectory showed a direct pursuit toward the correct response. In the switch condition, the average trajectory showed a strong initial movement toward the original location of the green square, followed by a sharp redirection toward the ultimate location of the green square. In the competition condition, the average trajectory was smooth and showed graded attraction toward the location of the distractor. (B) shows the distribution of trial-by-trial deviations for trajectories in the competition condition, illustrating unimodality. (C) shows the distribution of trial-by-trial deviations for trajectories pooled across the switch and baseline conditions, illustrating bimodality. The average trajectories and distributions elicited by these conditions show how the hand-tracking methodology can identifiably tap into a variety of cognitive processes (e.g., parallel competition, strategic response switching). Adapted from Farmer et al. (2007a).
Another issue is to relate this methodological approach to the long-established precedent of reaction times and reaction time distributions (e.g., Van Zandt, 2000). The reaction time is still the most widely used across psychological studies, and because of its ease of measurement and interpretation, we would not argue that hand-tracking should serve as any full substitute. Instead, approximately continuous hand-tracking data from an unfolding decision provides a unique behavioral junction point for certain theoretical debates. For example, theoretical debate anchored methodologically in reaction time eventually sought mitigation in reaction time distribution analyses (e.g., Ratcliff et al., 1999). Dynamic data from an action trajectory may, in some cases, more directly reveal the cognitive processes under dispute. These same points may be made about eye-tracking, now widely used as an ongoing measure of cognitive processing (e.g., Tanenhaus et al., 1995). The same caveats hold: Future work should tease apart the relative merits of all these methodological approaches and determine where they are most usefully employed.
A final pressing question is how fine-grained mental dynamics provided by hand movements relate to higher-level phenomena, such as behavior, educational and clinical outcomes, or social interaction. More work is needed to tie together data across these multiple time scales. Moreover, future research would benefit from combining hand movements with eye-movement and electroencephalography (EEG) data for a more complete characterization of the time-course of perception, cognition, and action, and their many interactions. Ultimately, this work could be used to bridge diverse domains of psychological science and help form truly integrative accounts of the mind.
Conflict of Interest Statement
The authors declare that the research was conducted in the absence of any commercial or financial relationships that could be construed as a potential conflict of interest.
Acknowledgments
This work was supported by NIH fellowship (F31-MH092000) to Jonathan Freeman and NSF research grants (BCS-0826825 and BCS-0720322) to Rick Dale. The authors thank Nalini Ambady, Ken Nakayama, and Phillip Holcomb for helpful insights.
References
Anderson, S.E., Farmer, T.A., Goldstein, M., Schwade, J., and Spivey, M. (in press). “Individual differences in measures of linguistic experience account for variability in the sentence processing skill of five-year-olds,” in How children make linguistic generalizations: Experience and variation in first language acquisition, eds I. Arnon and E. V. Clark (Amsterdam: Benjamins).
Cisek, P., and Kalaska, J. F. (2005). Neural Correlates of Reaching Decisions in Dorsal Premotor Cortex: specification of multiple direction choices and final selection of action. Neuron 45, 801–814.
Dale, R., and Duran, N. D. (2011). The cognitive dynamics of negated sentence verification. Cogn. Sci. doi: 10.1111/j.1551-6709.2010.01164.x
Dale, R., Kehoe, C., and Spivey, M. J. (2007). Graded motor responses in the time course of categorizing atypical exemplars. Mem. Cognit. 35, 15–28.
Dale, R., Roche, J., Snyder, K., and McCall, R. (2008). Exploring action dynamics as an index of paired-associate learning. PLoS ONE 3, e1728. doi: 10.1371/journal.pone.0001728
Duran, N. D., Dale, R., and McNamara, D. S. (2010). The action dynamics of overcoming the truth. Psychon. Bull. Rev. 17, 486–491.
Farmer, T. A., Anderson, S. E., and Spivey, M. J. (2007a). Gradiency and visual context in syntactic garden-paths. J. Mem. Lang. 57, 570–595.
Farmer, T. A., Cargill, S., Hindy, N., Dale, R., and Spivey, M. J. (2007b). Tracking the continuity of language comprehension: computer-mouse trajectories suggest parallel syntactic processing. Cogn. Sci. 31, 889–909.
Freeman, J. B., and Ambady, N. (2009). Motions of the hand expose the partial and parallel activation of stereotypes. Psychological Science 20, 1183–1188.
Freeman, J. B., and Ambady, N. (2010). MouseTracker: software for studying real-time mental processing using a computer mouse-tracking method. Behav. Res. Methods 42, 226–241.
Freeman, J. B., and Ambady, N. (2011a). When two become one: temporally dynamic integration of the face and voice. J. Exp. Soc. Psychol. 47, 259–263.
Freeman, J. B., and Ambady, N. (2011b). A dynamic interactive theory of person construal. Psychol. Rev. 118, 247–279.
Freeman, J. B., and Ambady, N. (in press). Hand movements reveal the time-course of shape and pigmentation processing in face categorization. Psychonomic Bulletin and Rev. doi: 10.3758/s13423-011-0097-6.
Freeman, J. B., Ambady, N., Midgley, K. J., and Holcomb, P. J. (2011). The real-time link between person perception and action: brain potential evidence for dynamic continuity. Soc. Neurosci. 6, 139–155.
Freeman, J. B., Ambady, N., Rule, N. O., and Johnson, K. L. (2008). Will a category cue attract you? Motor output reveals dynamic competition across person construal. J. Exp. Psychol. Gen. 137, 673–690.
Freeman, J. B., Pauker, K., Apfelbaum, E. P., and Ambady, N. (2010). Continuous dynamics in the real-time perception of race. J. Exp. Soc. Psychol. 46, 179–185.
Goldin-Meadow, S., and Alibali, M. W. (2002). “Looking at the hands through time: a microgenetic perspective on learning and instruction,” in Microdevelopment: Transition Processes in Development and Learning, eds N. Granott and J. Parziale (Cambridge: Cambridge University Press), 80–105.
Goodale, M. A., Pelisson, D., and Prablanc, C. (1986). Large adjustments in visually guided reaching do not depend on vision of the hand or perception of target displacement. Nature 320, 748–750.
Magnuson, J. S. (2005). Moving hand reveals dynamics of thought. Proc. Natl. Acad. Sci. U.S.A. 102, 9995–9996.
McKinstry, C., Dale, R., and Spivey, M. J. (2008). Action dynamics reveal parallel competition in decision making. Psychol. Sci. 19, 22–24.
Miles, L. K., Betka, E., Pendry, L. F., and Macrae, C. N. (2010). Mapping temporal constructs: actions reveal that time is a place. Q. J. Exp. Psychol. (Colchester) 63, 2113–2119.
Paninski, L., Fellows, M. R., Hatsopoulos, N. G., and Donoghue, J. P. (2004). Spatiotemporal tuning of motor cortical neurons for hand position and velocity. J. Neurophysiol. 91, 515–532.
Ratcliff, R., Van Zandt, T., and McKoon, G. (1999). Connectionist and diffusion models of reaction times. Psychol. Rev. 106, 261–300.
Resulaj, A., Kiani, R., Wolpert, D. M., and Shadlen, M. N. (2009). Changes of mind in decision-making. Nature 461, 263–266.
Rizzolatti, G., Riggio, L., Dascola, I., and Umilta, C. (1987). Reorienting attention across the horizontal and vertical meridians: evidence in favor of a premotor theory of attention. Neuropsychologia 25, 31–40.
Rosenbaum, D. A. (2005). The Cinderella of psychology: the neglect of motor control in the science of mental life and behavior. Am. Psychol. 60, 308–317.
Song, J. H., and Nakayama, K. (2006). Role of focal attention on latencies and trajectories of visually guided manual pointing. J. Vis. 6, 982–995.
Song, J. H., and Nakayama, K. (2008). Target selection in visual search as revealed by movement trajectories. Vision Res. 48, 853–861.
Song, J. H., and Nakayama, K. (2009). Hidden cognitive states revealed in choice reaching tasks. Trends Cogn. Sci. (Regul. Ed.) 13, 360–366.
Spivey, M. J., Dale, R., Knoblich, G., and Grosjean, M. (2010). Do curved reaching movements emerge from competing perceptions? A reply to van der Wel et al. (2009). J. Exp. Psychol. Hum. Percept. Perform. 36, 251–254.
Spivey, M. J., Grosjean, M., and Knoblich, G. (2005). Continuous attraction toward phonological competitors. Proc. Natl. Acad. Sci. 102, 10393–10398.
Tanenhaus, M. K., Spivey-Knowlton, M. J., Eberhard, K. M., and Sedivy, J. C. (1995). Integration of visual and linguistic information in spoken language comprehension. Science 268, 1632–1634.
van der Wel, R. P. R. D., Eder, J. R., Mitchel, A. D., Walsh, M. W., and Rosenbaum, D. A. (2009). Trajectories emerging from discrete versus continuous processing models in phonological competitor tasks: a commentary on Spivey, Grosjean, and Knoblich (2005). J. Exp. Psychol. Hum. Percept. Perform. 35, 588–594.
Keywords: hand, motor, real-time, temporal dynamics, time-course, mouse-tracking
Citation: Freeman JB, Dale R and Farmer TA (2011) Hand in motion reveals mind in motion. Front. Psychology 2:59. doi: 10.3389/fpsyg.2011.00059
Received: 08 February 2011; Accepted: 24 March 2011;
Published online: 20 April 2011.
Edited by:
Anna M. Borghi, University of Bologna, ItalyReviewed by:
Ruud Custers, Utrecht University, NetherlandsMichael Kaschak, Florida State University, USA
Copyright: © 2011 Freeman, Dale and Farmer. This is an open-access article subject to a non-exclusive license between the authors and Frontiers Media SA, which permits use, distribution and reproduction in other forums, provided the original authors and source are credited and other Frontiers conditions are complied with.
*Correspondence: Jonathan B. Freeman, Department of Psychology, Tufts University, 490 Boston Avenue, Medford, MA 02155, USA. e-mail: jon.freeman@tufts.edu