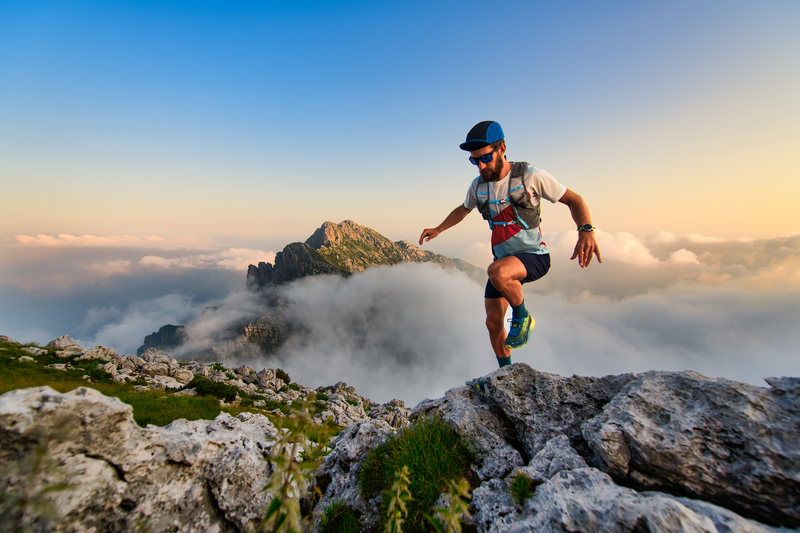
95% of researchers rate our articles as excellent or good
Learn more about the work of our research integrity team to safeguard the quality of each article we publish.
Find out more
ORIGINAL RESEARCH article
Front. Psychol. , 08 December 2010
Sec. Cognition
Volume 1 - 2010 | https://doi.org/10.3389/fpsyg.2010.00221
This article is part of the Research Topic Embodied and Grounded Cognition View all 24 articles
This work presents a connectionist model of the semantic-lexical system based on grounded cognition. The model assumes that the lexical and semantic aspects of language are memorized in two distinct stores. The semantic properties of objects are represented as a collection of features, whose number may vary among objects. Features are described as activation of neural oscillators in different sensory-motor areas (one area for each feature) topographically organized to implement a similarity principle. Lexical items are represented as activation of neural groups in a different layer. Lexical and semantic aspects are then linked together on the basis of previous experience, using physiological learning mechanisms. After training, features which frequently occurred together, and the corresponding word-forms, become linked via reciprocal excitatory synapses. The model also includes some inhibitory synapses: features in the semantic network tend to inhibit words not associated with them during the previous learning phase. Simulations show that after learning, presentation of a cue can evoke the overall object and the corresponding word in the lexical area. Moreover, different objects and the corresponding words can be simultaneously retrieved and segmented via a time division in the gamma-band. Word presentation, in turn, activates the corresponding features in the sensory-motor areas, recreating the same conditions occurring during learning. The model simulates the formation of categories, assuming that objects belong to the same category if they share some features. Simple exempla are shown to illustrate how words representing a category can be distinguished from words representing individual members. Finally, the model can be used to simulate patients with focalized lesions, assuming an impairment of synaptic strength in specific feature areas.
Traditional theories on human language and semantic memory assume that cognition consists in the manipulation of abstract symbols, separate from the modal system for perception and action. More specifically, word meaning in these theories is often represented as a vector in a multidimensional space, with the elements of this vector consisting of abstract features. This traditional point of view, however, fails to take into account that the meaning of concrete objects is strongly grounded in daily experience and exploits the perceptual modalities.
Recently, various theories in different domains (including linguistics, psychology, cognitive neuroscience, and robotics) rejected the idea that semantic memory merely relies upon abstract symbols, and emphasized the importance of concrete experience in the formation and retrieval of object meaning. “Grounded cognition” or “embodied cognition” (Gibbs, 2003; Barsalou, 2008; Borghi and Cimatti, 2010) assumes that our concepts consist of “perceptual symbols,” and that the retrieval of concept meaning is a form of re-activation of past sensory-motor and introspective experience.
Neuroimaging studies support the idea that simulation of past experience plays a pivotal role in conceptual processing. Dealing with information on food or smell activates gustatory and olfactory areas, respectively (Simmons et al., 2005; Gonzalez et al., 2006). Regions of the posterior temporal cortex involved in object representation become active during conceptual processing of pictures and words, as well as during auditory sentence comprehension (Davis and Johnsrude, 2003; Giraud et al., 2004; Rodd et al., 2005). Answering questions concerning visual, auditory, tactile, or taste properties activates regions involved in each of these modalities (Goldberg et al., 2006). Reading action words activates regions in the premotor cortex that are active when the subject performs the corresponding movement (Hauk et al., 2004). These results support the idea that conceptual processing is largely based on simulation of past experience, and that this experience provides part of the fundamental grounding for the construction of semantic memory and the use of language (the interested reader can find more details in excellent review papers on the subject such as Martin and Chao, 2001; Martin, 2007; Barsalou, 2008).
Several qualitative theories of semantic memory proposed in recent years may be reconciled with the grounded cognition viewpoint (see Hart et al., 2007). Most of them assume that a concept is described in a semantic memory as a collection of sensory-motor features, which spread over different cortical areas (Warrington and Shallice, 1984; Warrington and McCarthy, 1987; Caramazza and Shelton, 1998; Gainotti, 2000; Humphreys and Forde, 2001). These models are motivated by results on neurologically damaged patients showing selective impairment in category representation (for instance in recognition of animate vs. inanimate items).
Among others, a few conceptual models merit a brief description due to their analogy with some aspects of the model presented herein. Tyler and collaborators (Tyler et al., 2000; Tyler and Moss, 2001) developed a model named “Conceptual Structure Account.” This model assumes that semantic memory is organized as a distributed network of features. An important aspect is that objects may share certain features: common features would indicate a category membership, whereas members in the same category can be distinguished by distinctive features. Hence, peculiar features are essential to identify individual objects in the same category.
Barsalou and Simmons (Barsalou et al., 2003; Simmons and Barsalou, 2003) proposed a model (named the “Conceptual Topography Theory”) in which features are organized according to a topographical principle: the spatial proximity of neurons reflects similarity in the features they encode. According to this model, groups of features send their information to other convergence neurons, which replicate the concept of “convergence zone” originally proposed by Damasio (1989).
Hart et al. (2002) and Kraut et al. (2004) proposed the “Neural Hybrid Model of Semantic Object Memory” in which different regions encode not only sensorimotor but also high-order cognitive information (language, emotion, …). This information is then bound by synchronization of gamma rhythms modulated by the thalamus.
The previous models, however, were merely qualitative or conceptual. As Barsalou (2008) pointed out, the wealth of well-documented experimental data now urges the development of computational models to formalize the qualitative theories and inspire experimental tests. Unlike classical artificial intelligence methods which manipulate abstract symbols, the grounded cognition assumption should adopt the formalism of dynamic systems and neural network architectures to implement its basic ideas in computational models (Barsalou, 2008).
Several connectionist models have been developed in recent years, using attractor networks. Most of them are aimed at analyzing how the statistical relationships between features and categories can be incorporated in a semantic memory model, to explore the consequences for language processing (for instance, semantic priming) and to simulate semantic deficits by damage to network connection weights. Some models also investigated modal vs. amodal representation, and the role of distinctive vs. shared features.
Among others, Farah and McClelland (1991) developed a model in which differences between living and non-living things were simulated using a different number of functional and perceptual features for each concept. The model was able to explain selective category impairment by removing some features. Hinton and Shallice (1991) and Plaut and Booth (2000) used a back-propagation network with a feed-forward from orthography to semantics, and a feedback loop from semantics to hidden units. The damaged network exhibited behavior emulating phenomena found in deep dyslexia. McGuire and Plaut (1997) trained a network to map a visual or tactile representation onto phonology and action units via a common set of intermediate units. Plaut (2002) further developed this model assuming that connections among hidden units are constrained to favor short connections. An important result is that hidden units develop a graded degree of modality (i.e., while some regions in the hidden units network are mainly unimodal (visual or tactile) other regions are multimodal). The model was then used to simulate optic aphasia.
Rogers et al. (2004) developed a model in which perceptual, linguistic, and motor representations communicate via a heteromodal region (probably located in the anterior temporal cortex), which encodes semantic aspects and recalls the “convergence zone” hypothesized by Damasio (Damasio et al., 1996). The model has been used to differentiate between semantic dementia (which causes a generalized semantic impairment) and other pathologies characterized by category-specific deficits (Lambon Ralph et al., 2007).
Vigliocco et al. (2004) developed a model assuming two levels of semantic representations: conceptual features and lexico-semantic representations. The first is assumed to be organized according to modality. An important aspect of their model is that the lexico-semantic space is trained in an unsupervised manner to develop a self-organizing map. This map allows a similarity to be built between lexical representations.
Other authors focused on the role of distinctive and shared features. Randall et al. (2004) trained a feed-forward three-layer back-propagation model to map words from semantic features, and studied the role of shared and distinctive features. Their results suggest that distinctive features are vulnerable due to weak correlation with other features. Cree et al. (1999) developed an attractor network with three sets of features (word-form, semantic features, and hidden units) trained with the back-propagation through time learning algorithm. Cree et al. (2006) used a variation of this model, in which semantic units were directly connected by reciprocal weights, to investigate the role of distinctive features in the computation of word meaning. Contrary to Randall et al. (2004), their results suggest that distinctive features have a privileged role in the computation of word meaning.
Alternative models used attractor networks trained by the Hebb rule to simulate phenomena such as semantic priming in normal subjects and in schizophrenia patients (Siekmeier and Hoffman, 2002) or to study the type of errors made by dyslexic patients (McRae et al., 1997) and by patients with dementia (Gonnerman et al., 1997).
Miikkulainen (1993, 1997) developed a model consisting of two self-organizing maps (one for lexical symbols and the other for word meaning) and of associative connections between them based on Hebbian learning. This model is perhaps the most similar to the model presented in this work.
The brief overview presented above highlights the increasing impact of computational models on the study of semantic and lexical aspects. This study describes a new model of the lexical-semantic memory, which is coherent with the grounded cognition hypothesis. Characteristics shared with previous models are: (i) a distinction between a conceptual representation (based on features) and a lexical store; (ii) the conceptual store is divided into distinct areas, which may be devoted to different modalities; (iii) the lexical aspects are implemented in a “convergence zone”; (iv) concepts are retrieved using attractor dynamics.
Original aspects of the model, not incorporated in previous studies are the following. (i) The use of oscillatory units in the gamma-band. Hence, attractors are not steady states but synchronized oscillations among neurons participating in the same object representation. This solution allows several objects to be retrieved simultaneously in memory and correctly segmented via temporal phase separation. This may allow the realization of more sophisticated semantic memories in which several concomitant objects concur to form a complex scene. Indeed, in many cognitive problems, several representations in memory may need to be maintained to have a complete understanding of the scene or realize a complex task (let us consider, for instance, sentence comprehension, or working memory tasks). The role of gamma-band synchronization in object recognition is supported by many data in the neurophysiological literature, not only in relation to perceptual problems, but also for high-level cognitive tasks (Pulvermüller et al., 1996; Tallon-Baudry et al., 1997, 1998; Bhattacharya et al., 2001; Salinas and Sejnowski, 2001; Osipova et al., 2006; Melloni et al., 2007). (ii) Features in the semantic space have a topological organization typical of modal representations in the cortex (for instance in motor, tactile and auditory areas, and in many visual areas). With the exception of the model by Miikkulainen (1993, 1997), other models do not use topological maps to describe the conceptual aspects of their objects. A self-organizing map was used by Vigliocco et al. (2004) but was devoted to lexical aspects. (iii) A different and more physiological rule (i.e., a time dependent Hebbian rule) is used to train synapses, employing both potentiation and depression, whereas most models used a back-propagation supervised algorithm to train synapses. In particular, our rules spontaneously lead to a clear distinction between the role of shared and distinctive features in the conceptual network. Although other models analyzed this distinction (Cree et al., 2006), our structure of synapses is new, as shown in the Section “Results” and analyzed in the Section “Discussion.”
A simpler version of our current model, limited to the semantic aspects, was outlined in a recent work (Ursino et al., 2009). The previous version, however, had several limitations: (i) each object could be represented by a fixed number of features, whereas, according to Pexman et al. (2002, 2003) the number of features plays an important role in object recognition. In particular, these authors observed that words with a higher number of features respond more quickly than words with fewer features, a result which supports a distributed representation of meaning; (ii) there was no clear difference between distinctive features and shared features, to represent objects in the same class; (iii) there was no link between the semantic and lexical aspects.
Aim of this paper is to present an upgraded version of the model, and test it with a few examples on simulated objects, emphasizing the analogy with the grounded cognition assumption. The main improvements are: (i) the model can manage objects with a different number of features; (ii) it clearly differentiates between the role of distinctive and shared features in object recognition; (iii) it includes a lexical area for the representation of lemmas (or word-forms); (iv) the model learns the relationships between features of the same object (i.e., object semantics), and its word-form from exempla, using physiological learning rules.
Simulations are presented to show how the model can evoke correct words from a partial cue in the semantic area, and how a word can reconstruct the conceptual representation of the object in the semantic network, spreading over different feature areas. Model limits and lines for future investigation are then discussed.
The model incorporates two neuronal networks, as illustrated in the schematic diagram of Figure 1. The first is devoted to representing the conceptual meaning of objects, described as a collection of features (semantic network). The second represents word-forms or lemmas (lexical network). The semantic network is explained first, including mechanisms for learning the object conceptual meaning from exempla. Then the lexical network is introduced, explaining mechanisms to link semantic and lexical aspects. Equations for the new (lexical and training) aspects are given in the Appendix, together with parameter numerical values.
Figure 1. Schematic diagram describing the general structure of the network. The model presents a “semantic network” and a “lexical network.” The semantic network consists of nine distinct Feature Areas (upper shadow squares), each composed of 20 × 20 neural oscillators. Each oscillator is connected with other oscillators in the same area via lateral excitatory and inhibitory intra-area synapses, and with oscillators in different areas via excitatory inter-area synapses. The lexical area consists of 20 × 20 elements (lower shadow square), whose activity is described via a sigmoidal relationship. Moreover, elements of the feature and lexical networks are linked via recurrent synapses (WF, WL).
The first network, named “semantic network,” is devoted to a description of objects represented as a collection of sensory-motor features. These features are assumed to spread along different cortical areas (in both the sensory and motor cortex and perhaps also in other areas, for instance emotional) and are organized topologically according to a similarity principle. This means that two similar features activate proximal neural groups in the network.
The network is composed of F distinct cortical areas (see Figure 1). Each area, in turn, consists of a lattice of neural oscillators. An oscillator may be silent, if it does not receive enough excitation, or may oscillate in the γ-frequency band (30–70 Hz) if excited by sufficient input. Oscillator dynamics is realized via the local feedback connection of an excitatory and an inhibitory population. This arrangement can be seen as a simple description of a cortical column or of a cortico-thalamic circuit. An oscillatory activity in this network allows different objects to be simultaneously held in memory (i.e., it favors the solution of the binding and segmentation problem) via γ-band synchronization (see Ursino et al., 2009). Oscillators representing the properties of the same object should oscillate in phase, whereas oscillators representing properties of different objects should oscillate with a different phase. Gamma-band synchronization has been proposed as an important mechanism in high-level cognitive tasks, including language recognition and semantic processing (Steriade, 2000; Slotnick et al., 2002; Kraut et al., 2004).
During the simulation, a feature is represented by a single input localized at a specific coordinate of the network, able to trigger the oscillatory activity of the corresponding unit. We assume that this input is the result of an upstream processing stage that extracts the main sensory-motor properties of the objects. In previous works (Ursino et al., 2009), we assumed that each object is described by a fixed number of features. Conversely, we now consider that the number of features can vary from one object to the next. The way these features are extracted and represented in the sensory and motor areas is beyond the aim of the present model. The use of authentic objects with realistic features may represent a further evolution of this model.
The present network has a maximum of nine features: this constraint was imposed merely to reduce the simulation computational cost.
A topological organization in each cortical area is realized assuming that each oscillator is connected with the others in the same area via lateral excitatory and inhibitory synapses (intra-area synapses). The synapses have a Mexican hat disposition, i.e., proximal neurons excite reciprocally and inhibit more distal ones. This disposition produces an “activation bubble” in response to a single localized feature input: not only is the neural oscillator representing that individual feature activated, but also the proximal ones linked via sufficient lateral excitation. This has important consequences for object recognition: neural oscillators in proximal positions share a common fate during the learning procedure and are subject to a common synapse reinforcement. Hence, they participate in the representations of the same objects. In this way, an object can be recognized even when some of its features are slightly altered (similarity principle).
Throughout the present paper, the lateral intra-area synapses and the topological organization will not be trained, i.e., they are assigned “a priori.” This choice is convenient to maintain a clear separation between different processes in our model (i.e., implementation of the similarity principle on the one hand and implementation of object semantics on the other). Of course, topological maps can also be learned through experience (Hertz et al., 1991), but this mechanism probably develops in early life and precedes object semantic learning.
Besides the intra-area synapses, we also assumed the existence of excitatory long-range synapses between different cortical areas in the semantic network (inter-area synapses). These are subject to a training phase (see below) and implement the conceptual (i.e., semantic) knowledge of the object.
The inter-area synapses connecting different features are trained in a first phase, in which single objects (described by all their features) are presented to the network one by one. We assume that synapses are reinforced based on the correlation between the activity in the post-synaptic unit, and the activity in the pre-synaptic unit mediated over a previous 20 ms time window (Markram et al., 1997; Abbott and Nelson, 2000). However, reinforcement alone would produce a symmetric pattern of synapses, whereas an asymmetric pattern of synapses may be useful in case of objects with common features. Let us consider an object sharing common features with other objects but with some distinctive features (examples will be considered in the Results). It can be expected that distinctive features are highly important to recognize an object and evoke the remaining features (including all common ones). Conversely, it can be expected that common features (shared with other objects) do not evoke distinctive features. To obtain this behavior, asymmetrical synapses are needed: synapses from common features to distinctive features must be weaker, whereas synapses from distinctive features to common features must be stronger. This asymmetrical pattern of synapses is obtained assuming that a synapse weakens when the pre-synaptic neuron is active and the post-synaptic neuron is inhibited (homosynaptic depression). In this way, synapses from common to distinctive features weaken at any presentation of a new object sharing the same common features. An example of the synapses obtained after presentation of two objects, each with seven features (four common features and three distinctive features) is given in Figure 2.
Figure 2. An example of the synapses connecting features in the semantic network after training. The network was trained using obj3 and obj4 described in Table 1 (other objects are not included to simplify the analysis). The color in each figure represents the strength of the synapses reaching a given post-synaptic neuron, coming from different pre-synaptic neurons. The position of the post-synaptic neuron is marked with a gray circle. Arrows have been included for clarity. Two exempla are reported in the present figure. The left panel describes the strength of the synapses reaching a neuron coding for a common feature (this is the neuron at position 15, 5, whose feature is common for obj3 and obj4). The right panel describes the synapses reaching a neuron coding for a distinctive feature (this is the neuron at position 30, 30, whose feature is distinctive for obj4). The figure content can be explained as follows: (i) a common feature (left panel) receives synapses from all features in obj3 and obj4. The synapses coming from the other three common features are stronger (red color) than those coming from the six distinctive features (green color), since common features are more often encountered during training; (ii) a distinctive feature (right panel) receive synapses only from the other six features of the same object (obj4). Synapses from the distinctive features have the same strength as in the left panel (green color), whereas synapses from common to distinctive features are weaker (cyan color) as a consequence of depression (see text for details). Hence, common features are strongly interconnected with other common features, but do not evoke distinctive features. Distinctive features are moderately interconnected, and can evoke both other common features and other distinctive features. Finally, it is worth noting that a neuron receives synapses not only from neurons coding for the “exact” feature of the same object, but also from proximal neurons, thereby constituting an “activation bubble.” This implements a similarity principle.
After training, the semantic network exhibits the typical behavior of an auto-associative memory, i.e., it can reconstruct the overall conceptual information of an object starting from a partial content. However, several aspects differentiate this network from classic auto-associative nets (such as the Hopfield net, see Hertz et al., 1991). First, thanks to the topological implementation, an object can be reconstructed even after moderate changes in a few features (similarity principle). Second, thanks to the forgetting factor included via the homosynaptic depression, distinctive features of an object play a greater role than shared features. Lastly, oscillatory activity allows multiple objects to be simultaneously held in memory.
To represent lexical aspects, the model includes a second layer of neurons, denoted “lexical network.” Each computational unit in this network codes for a lemma or a word-form and is associated with an individual object representation. In this case too, the input must be considered the result of an upstream processing stage, which recognizes the individual words from phonemes or from orthographic analysis. Description of this processing stream is well beyond the aim of this model: some exempla can be found in recent works by others (see Hopfield and Brody, 2001) for word recognition from phonemes, and (Farah and McClelland, 1991; Hinton and Shallice, 1991) for orthographic processing models.
Of course, units in this network can also be stimulated through long-range synapses coming from the semantic network: in this regard, the lexical network constitutes an amodal convergence zone, as hypothesized in the anterior temporal lobe (Damasio, 1989; Gainotti, 2005). Long-range synapses between the lexical and the semantic networks are subjected to learning (see below) and may be either excitatory or inhibitory.
For the sake of simplicity, computational units in this network are not described as oscillators. Hence, if stimulated with a constant input, they reach a given steady-state activation value after a transient response (but, of course, they oscillate if stimulated with an oscillating input coming from the semantic network).
In order to associate words with their object representations, we performed a second training phase in which the model receives a single input to the lexical network (i.e., a single word is detected) together with the features of a previously learned object. Synapses linking the objects with words in both directions (i.e., from the lexical network to the feature network and vice versa) are then trained.
While synapses from words to features ( in Figure 1) are simply excitatory and are trained on the basis of the pre- and post-synaptic correlation, when computing the synapses from features to words (
in Figure 1) we tried to address a fundamental requirement: a word must not be evoked if spurious features (not originally belonging to the prototypical object) are active. This situation may occur when two or more concomitant objects are not correctly segmented, and some of their features pop up together. Hence, this requirement corresponds to a correct solution of the segmentation problem. This requirement also avoids a member of a category evoking the word representing the whole category (assuming that features in a category are shared by its members).
To address this requirement, we assumed that before training all units in the feature network send strong inhibitory synapses to units in the lexical network. Hence, activation of any feature potentially inhibits lexical units. These synapses are then progressively withdrawn during the training phase, and excitatory synapses are formed on the basis of the correlation between activity in the feature unit and in the lexical unit. Moreover, we assumed that after sufficient training the sum of all excitatory synapses reaching a word must be constant, irrespective of the number of features (i.e., we adopted a normalization of synaptic weights). The consequence of this choice is that after training a word receives inhibition from all features that do not belong to its semantic representation, but receives excitation from its own feature units. When all features are present, without spurious features, the neuron coding for the specific word is excited above a threshold and switches from the off to the on state. Switching of neurons has been mimicked using a sharp sigmoidal relationship.
After training, a word can reconstruct its conceptual representation in the semantic network by evoking the same cortical activity present during object learning, in agreement with the grounded cognition assumption (“word recognition task”). Similarly, reconstruction of a complete object representation in the semantic network from a partial cue evokes the corresponding word in the lexical area (“object recognition task”). Finally, the network can distinguish between a category and its individual members (and evokes the corresponding words) on the basis of the shared and distinctive features.
Simulations shown in this work were performed using six distinct objects: two of them (named obj1 and obj2) have five features, the others (obj3, obj4, obj5, and obj6) have seven features. Moreover, obj3 and obj4, are correlated, i.e., they exhibit four shared features plus three distinctive features. We assume that the four shared features of obj3 and obj4 denote a category (say “ctg1”) (just to fix our ideas, obj3 can be “dog,” obj4 can be “cat,” and the four shared features may represent the category “pet”). Another two objects (obj5 and obj6) also exhibit four shared features, which denote a new category (say “ctg2”). Moreover, obj3, obj4, obj5, and obj6 have two shared features that identify a wider category (say “ctg3”) incorporating “ctg1” and “ctg2.” To fix our ideas, obj5 and obj6 may be “cattle” and “goat,” “ctg2” the category “ruminant” and “ctg3” the category “animal.” During a first training period, the objects were given to the network several times in a random fashion. The learning rate and the number of iteration steps during the training phase were chosen so that at the end of training the objects could be reconstructed giving about one half of their features. Subsequently, during a second training period the objects (including the categories) were given to the semantic network together with the corresponding word in the lexical network, and the association objects-words was created. The position of the individual features in the semantic network, and the position of the corresponding word-forms in the lexical network are given in Table 1.
Table 1. Position of the features in the semantic network for the six simulated objects (second column), and position of the corresponding word-forms in the lexical network (third column).
Other objects and categories, uncorrelated with the previous ones, with a number of features ranging between two and nine, were also tested. They behave substantially like those presented here.
Figure 3 shows the example of a concomitant “object recognition” and “word recognition” task. In this simulation, a word (obj3) was given to the lexical network, and just four features of a second object (obj1) were given to the semantic network. As the figure clearly shows, after a short transient period the semantic network is able to recover the missing feature of obj1; the overall conceptual meaning of obj1 then evokes the corresponding word in the lexical area but almost completely inhibits activity of the word coding for obj3. After a short period of time, when the conceptual representation of obj1 is turned off, the word representing obj3 evokes its conceptual representation in the semantic network. The semantic representations of obj3 and obj1 then oscillate in time division. It is worth noting that the network recreates the same cortical representations in the semantic network that were originally present during the learning of obj1 and obj3, according to the grounded cognition hypothesis.
Figure 3. Simulation of a “word recognition task” and an “object recognition task” performed simultaneously. Four features of obj1 were given as input to the semantic network, while the word denoting obj3 was given to the lexical network. The four lines represent activity in the semantic network (left panels) and in the lexical network (right panels) at steps 136, 156, 199, and 217 of the simulation (duration of each step: 0.2 ms). At step 136 (first line) obj1 is completely reconstructed in the semantic network, the word coding for obj1 is activated in the lexical network, while the word coding for obj3 is inhibited. At step 156 (second line), when the conceptual representation of obj1 has been switched off, the word coding for obj3 evokes its conceptual representation in the semantic network. Then, the two representations alternate, oscillating in the gamma range.
Figures 4 and 5 describe the results of two distinct object recognition tasks, in which the subject must recognize a single category (Figure 4) or a member from a category (Figure 5) starting from partial cues in the semantic network. More particularly, in Figure 4 three features shared by obj3 (dog) and obj4 (cat) are given to the semantic network. The model reconstructs the fourth shared feature, and the word denoting the category “pet” is finally evoked in the lexical area, without evoking the words denoting individual members. In Figure 5, the semantic network receives two distinctive features of obj3 (“dog”) and one shared feature of “pet.” The semantic network now recovers all seven features of “dog,” and the word denoting the individual member is correctly evoked in the lexical area. These simulations clearly disclose the different role of shared and distinctive features in object recognition.
Figure 4. Simulation of an object recognition task in which the subject must recognize a category. Three properties common to the dog and cat are given to the semantic network. The four lines represent activity in the semantic network (left panels) and in the lexical network (right panels) at steps 67, 68, 69, and 71 of the simulation (duration of each step: 0.2 ms). As evident in the left panels, the three features progressively evoke the fourth common feature in the semantic network; when the overall conceptual representation of the category is reconstructed, the word coding for that category (“pet”) is activated in the lexical area (fourth line). It is worth noting that the distinctive properties of dog and cat are not evoked, and so individual members of the category do not appear in the lexical network.
Figure 5. Simulation of an object recognition task in which the subject must recognize a member of a category. Two distinctive properties of dog and one common property shared by dog and cat are given to the semantic network. The four lines represent activity in the semantic network (left panels) and in the lexical network (right panels) at steps 131, 133, 135, and 137 of the simulation (duration of each step: 0.2 ms). As shown in the left panels, the three features progressively evoke the four remaining features of dog in the semantic network; when the overall conceptual representation is reconstructed, the word coding for “dog” is activated in the lexical area (fourth line). It is worth noting that the distinctive properties of cat are not evoked. Neither the word coding for “cat,” nor the word naming for the category (“pet”) appear in the lexical network.
Figure 6 shows the case in which all 10 features of obj3 and obj4 (i.e., cat and dog) are given to the semantic network. The two objects are correctly segmented (despite the presence of the four common features) and the two words are evoked in the lexical area. It is worth noting that some isolated features appear at some instants during the simulation, but do not evoke any response in the lexical area.
Figure 6. Simulation of an object recognition task in which the subject must recognize two members of the same category. All 10 properties of dog and cat (four shared properties and three distinctive properties for each object) are given to the semantic network. The six lines represent activity in the semantic network (left panels) and in the lexical network (right panels) at steps 11, 133, 176, 239, 285, and 347 of the simulation (duration of each step: 0.2 ms). As the figure shows, the conceptual representations of the two objects are correctly reconstructed in the semantic network, and the corresponding words (“dog” and “cat”) evoked in the lexical network, despite the presence of four shared features (see the second, fourth, and sixth lines). The word designating the category (“pet”) does not appear in the lexical network. It is worth noting that some isolated features appear at some instants during the simulation (third and fifth lines), but do not evoke any response in the lexical area.
Finally, Figure 7 shows the result obtained by giving the lexical network two words of the same category (the word “dog” and the word “cat”). If the two words are given simultaneously, the behavior of the semantic network is remarkable. The four common features oscillate in synchronism, and the word “pet” appears in the lexical network. Hence, the model is able to generalize from two words to a category. The three distinctive features of cat, and the three distinctive features of dog oscillate independently, without a synchronization with the remaining four features.
Figure 7. Simulation of a word recognition task in which the subject must recognize two words from the same category. The two words naming “dog” and “cat” are given to the semantic network. The six lines represent activity in the semantic network (left panels) and in the lexical network (right panels) at steps 12, 32, 60, 81, 100, and 120 of the simulation (duration of each step: 0.2 ms). As the figure shows, the two words initially evoke the four shared properties in the semantic network and consequently the word denoting the category (“pet”) is activated in the lexical area (third line). Hence, the network can generalize from the two members of the category to the category name. The three distinctive features of the two objects oscillate in time division (fourth and fifth lines) causing the momentarily inhibition of the alternative word. Hence, the three words (two members and their category) oscillate in time division in the gamma range.
In the previous exempla we used just a small number of objects and categories (three objects and just one category). An important problem is whether the network can manage a larger number of objects representing many categories, and can manage a taxonomy of categories. As further commented in the Section “Discussion,” we can store additional objects in the network without altering its behavior, provided the new objects are uncorrelated to the existing ones. We tested this aspect by storing new objects (not shown in Table 1 for brevity) with the following characteristics: (i) new objects with distinct features (from two to nine) but no correlation with the others. They can be retrieved correctly; (ii) pairs of objects with shared features, but no correlation with previous objects; they generate new categories and behave like obj3 and obj4.
A more complex condition may occur if we consider the case of three or more objects within the same category, or objects which involve a taxonomy of categories. To test this condition, we incorporated two new objects in the network (obj5 and obj6, see Table 1). Objects now generated a simple taxonomy: a category (say “animal”) now includes four objects (dog, cat, cattle, and goat) with two shared features; another two categories (say “pet” and “ruminant”), included within “animal,” have two objects each with four shared features. All objects were first trained separately in the semantic network, then objects and categories were associated with word-forms in the lexical network (see Table 1).
Simulations show that the model can correctly discriminate between these categories and individual objects, and correctly evoke the corresponding words. In particular, a word in the lexical network evokes the correct conceptual representation in the semantic network, both if the word represents an object and when it represents a category. For instance, the word “goat” evokes all seven features; the word “ruminant” evokes the four features common to cattle and goat only; the word “animal” evokes just the two features shared by dog, cat, cattle, and goat. Similarly, providing a number of features in the semantic net causes the correct object reconstruction (without confounding objects and categories) and evokes the corresponding word. An example is given in Figure 8. The upper panel shows a simulation when five features of goat were given to the network (all four shared features in ruminant and one feature distinctive of goat); the remaining two features are evoked and the correct word denoting “goat” appears in the lexical area. The mid panel shows the case when the semantic net receives one feature in animal, together with two features in ruminant (these are distinctive features for this category); the fourth feature is evoked, together with the word-form denoting “ruminant.” Finally, the bottom panel shows the case when only two features of animal, shared by four objects, are given. In this case, in the absence of any distinctive feature, the larger category (“animal”) is evoked in the lexical net.
Figure 8. Simulation of three object recognition tasks (performed separately) involving a taxonomy of categories. The upper panel shows a single snapshot obtained when the four properties of ruminant are given to the semantic network, together with one distinctive feature of goat. The network restores the two remaining features of goat, and the correct word (“goat”) is evoked in the lexical area. The mid panel shows a single snapshot obtained when the two shared features of animal are given to the semantic network, together with a feature of ruminant. The fourth feature of ruminant is restored and the word “ruminant” evoked in the lexical area. Finally, the bottom panel shows a single snapshot obtained by giving the two features of animal to the semantic network. This information does not spread toward other features and the word “animal” is evoked in the lexical network.
A common assumption to explain selective impairment in category representation is that different categories exploit different subsets of features (for instance, sensory features are essential to recognize animate objects, whereas motor features are essential to recognize tools). Hence, a lesion damaging a specific zone of the cortex would cause a selective impairment only for those categories which intensively exploit critical features in that zone.
In order to simulate selective impairment with our model, we considered the first two objects in Table 1 (obj1 and obj2) which have five features each, without common features. We chose to compare two objects with the same number of features, so that any difference can be ascribed to the position of features rather than to the complexity of the semantic representation. To simulate a semantic deficit, we assumed that a given percentage of synapses emerging from neurons in a given region (see Figure 9, upper panels) are damaged in a random fashion (i.e., they have been randomly set at zero). This may reflect a loss of neurons caused by a local lesion. As shown in the upper panels of Figure 9, the first object has four features in the damaged region. Conversely, the second object has just one feature in this region. For each level of synapse damage (from 0 to 40%) we repeated 10 simulations for each object presentation, and computed the response of the corresponding word in the lexical area. A response is assumed to be correct if presentation of the object is able to evoke a sufficient activity (above a given threshold) in the lexical area. The bottom panels of Figure 9 show the percentage of correct responses for the two objects, as a function of the percentage of synapse impairment, and using two different thresholds for detection (one very small, 0.1 and the other quite high, 0.4). It is clear that if the percentage of synapse damage increases above 20–30%, the network frequently fails to recognize obj1 until, for a percentage of synapse damage as high as 40%, it almost completely loses the possibility to evoke a correct word. Conversely, recognition of obj2 is almost unaffected by the synapse damage, despite the presence of one feature in the damaged area. The four remaining features of obj2 are able to restore the fifth one, and recover the correct word irrespective of the local damage.
Figure 9. Simulation of lexical deficit. The upper panels show the representations of two distinct objects in the semantic network (obj1 and obj2 in Table 1), with five features each, used to simulate selective impairment in category representation. The dashed area denotes the lesioned region, where a given percentage of synapses has been removed randomly. Obj1 has four features in the lesioned region, whereas obj2 has just one feature in that region. The bottom panels represent the percentage of success in recognition of obj1 and obj2, as a function of the percentage of damaged synapses. Two different thresholds for word recognition in the lexical area were used. It is worth noting that obj1 is frequently missed for a percentage of synapse damage greater than 20%, whereas the recognition of obj2 is quite robust despite synapse damage.
Modern theories on grounded cognition assign a pivotal role to “simulation” in the formation of the conceptual meaning of objects. Although the term “simulation” can have different meanings (see the recent paper by Borghi and Cimatti, 2010 for a clear analysis), a typical shared viewpoint considers simulation a form of re-activation of past experience. This may consist in the recruitment of the same neural networks involved in perception and action (Jeannerod, 2007) and in excitation of the same neural groups which coded for sensory and motor experience (Barsalou, 1999).
Our model implements grounded cognition by exploiting a few fundamental assumptions. Most of these find significant support in the recent neurophysiological and cognitive literature.
(i) The semantic and lexical aspects of declarative knowledge are stored in two distinct networks. Moreover, the semantic network spreads over different areas and exploits a distributed representation to describe object meaning. These ideas are frequently accepted in the neurocognitive literature, and are supported by analysis of bilingual subjects (Potter et al., 1984; Kroll and Stewart, 1994; McRae et al., 1997) who exhibit a common semantic representation but distinct lexical items.
(ii) The semantic network works like a classic auto-associative circuit, i.e., it can restore the overall past experience starting from a partial cue. Nevertheless, our semantic net differs from classic auto-associative networks in many important instances: it implements a similarity principle (an object can be restored even if it is just similar to a previous one) and can manage multiple objects simultaneously by means of rhythm synchronization in the gamma-band. The first aspect is realized through a topological organization of features, as in Barsalou and Simmons (Barsalou et al., 2003; Simmons and Barsalou, 2003). Of course, a modified version of our model can be imagined in which just some features (like colors, sounds, motion) have a topological organization and exploit a similarity principle, while other features are not topologically organized. This may be the subject of future more realistic model versions. The role of gamma-band synchronization in high-level cognition is well-documented, and is the subject of active research (the interested reader can find more references in Engel and Singer, 2001; Kraut et al., 2004; Fries et al., 2007). A further important aspect differentiating our model from classic auto-associative nets is the presence of asymmetric synapses. While shared features do not evoke distinctive features, distinctive features are able to evoke shared features thereby leading to a complete object reconstruction. This aspect implements a different role for distinctive and shared features in the recognition of categories or members within a category, on the basis of synapse weights after training. A similar analysis of weights is reported in Cree et al. (2006) but with a noteworthy difference from our approach. Cree et al.’s model differentiates between shared and distinctive features by using the weights between words and features. The authors reported that weights from words to distinctive features are significantly higher than the weights to shared features. Similarly, weights leaving the distinctive features to words are higher than those leaving the shared features. As a consequence, distinctive features are more active than shared features and more strongly involved in word activation. Our results differ since differences between distinctive and shared features are implemented in the synapses within the semantic net (see Figure 2): distinctive features send stronger synapses to shared features, and receive smaller synapses from them. Our model first reconstructs an object completely in the semantic net and then associates it with the corresponding word-form. Conversely, the interaction between words and features is essential for object reconstruction in Cree et al.’s (2006) model. Both strategies may be implemented in real semantic memories.
(iii) The lexical network represents a sort of amodal convergence zone (Damasio, 1989). Neurons in this zone become active if and only if the overall semantic representation of the corresponding object is simultaneously active in the semantic net. The presence of a convergence zone supports the idea of “gamma-based computation” (Fries et al., 2007): if all features of the object are oscillating in phase, the convergence zone receives enough input excitation to trigger the word-coding neuron. Conversely, if features oscillate out of phase, neurons in the convergence zone do not receive enough excitation to become active. The lexical zone, however, does not only receive converging inputs (thanks to entering synapses), but also sends information back to cortical areas (thanks to outgoing synapses) thus enabling the re-creation of past experience. When a word is given to the lexical net, its emerging synapses recover the same activity in the semantic network that was present at the moment of object learning. If this activity is assumed to be motor, perceptual or emotional in type, the grounded experience of that object is recreated.
(iv) Features and word-forms in our model are assumed to be the result of upstream processing networks, which extract these features from previous sensory or motor information (for the semantic net) or from phonological or orthographic information (for the lexical net). A description of these processing stages is beyond the aim of the present study, but may be integrated in the model (possibly starting from already existing models) in future versions. One consequence is that neural activity, recovered to represent the conceptual meaning of objects, is not present in the primary motor and/or perceptual cortices, but is a kind of neural information processed in higher cortical areas (for instance, in the temporal visual pathway for visual information). This aspect agrees with the notion of “perceptual symbols” put forward by Barsalou (1999) and may explain the difference between merely perceiving an object and recreating its conceptual meaning. Object perception also involves activation in the primary cortical areas, while recreating the conceptual meaning of objects mainly activates higher areas involved in feature representation. Neuroimaging data in favor of this idea can be found in Martin (2007).
(v) Past experience is stored in model synapses using physiological learning rules. These exploit the correlation (or anti-correlation) of pre-synaptic and post-synaptic activities over a time window (10–20 ms) compatible with gamma-band activity (Markram et al., 1997; Abbott and Nelson, 2000). The model, however, assumes different versions for the learning rule to build synapses within the semantic network and to build lexical-semantic connections. These are essential to reach a correct model behavior (i.e., to have a different role for shared and distinctive features in the semantic net, and to represent objects in the lexical net with a different number of features). These rules may be the subject of ad hoc testing on the basis of available experimental data in the literature or new experiments on synaptic plasticity.
After implementing the previous basic ideas, the model can cope with word recognition and object recognition tasks quite well, also involving multiple objects, categories, and words. Furthermore, the model can distinguish the members of a category, and evoke a category from multiple members, exploiting the differences between shared and distinctive features. A particular behavior of the model is the response to two simultaneous words, representing two members of the same category. The model is able to generalize from members to the category by isolating the shared features from the distinctive features of the two objects (see Figure 7) and activating the three words (the two denoting members and the third denoting the category) in time division. We do not know if this model response is correct from a cognitive perspective, but it certainly represents an interesting emergent behavior. Finally, the present model implementation represents a simple and straightforward way to look at selective impairment in category representation. As shown in Figure 9, selective random damage to synapses in a zone of the semantic network naturally leads to a deficit in recognition of objects whose conceptual meaning exploits many features in that zone.
The present work considered just six different objects and three categories adopting a single hierarchical level between categories (i.e., we have one larger category which contains two smaller ones). Of course, the number of objects and categories can be increased without significant deterioration in model performance provided the new objects and the new categories are uncorrelated to the older ones (i.e., if patterns are orthogonal). This is a well-known property of auto-associative networks (Hertz et al., 1991). Indeed, we trained the network with some additional uncorrelated objects (with a number of features ranging between 2 and 9), and with further objects having some shared features (which implement new categories uncorrelated to previous categories) and the network functions correctly in storing and retrieving these objects.
A more complex problem may occur if the number of correlated objects increases, i.e., trying to store many objects with shared features, since this may cause a deterioration in the performance of auto-associative networks. The present work had a maximum of four correlated objects (i.e., a maximum of four objects within the same category) with a moderate correlation (2/7). Two pairs of objects have a greater correlation (4/7). Studying the capacity of the network to manage a larger number of correlated objects may be the focus of subsequent works. However, storing a large number of correlated objects in the network (to simulate a realistic data set) may require an increase in network size since the capacity of an auto-associative network depends on the ratio between the number of objects and the number of neurons (Hertz et al., 1991). The present study kept the number of neurons and feature areas quite low to contain the size of the synapse matrix and avoid an excessive computational charge.
A possibility offered by the model in future works is to study the potential occurrence of under-generalization or over-generalization during training. The present work used a similar learning rate for the different objects, and all objects were trained using a complete set of their features to obtain a correct behavior during word recognition and object recognition tasks. Over-generalization might occur if one object is stored much more strongly than other objects in the same category (for instance due to longer training or a higher learning rate). Conversely, under-generalization might occur when a specific feature (not really belonging to a category) is erroneously associated with the word representing the category. All these occurrences may be investigated with the model, studying its behavior during the training period as a function of the parameters used (learning rate, duration of the inputs) and the statistics of the input features.
Finally, it is important to stress aspects of the present model which deserve further investigation and may be the subject of future research.
In the introduction, we stressed that our model agrees with the “grounded” or “embodied” cognition viewpoint. Indeed, it may be objected that it is not easy to distinguish between features in our approach and symbols normally used in amodal computation, and so there is no real embodiment in the model. Conversely, we think that the present model contains some significant embodiment aspects, especially in the way features are represented and organized. First, as stressed above, in our model the features exhibit a topological organization, i.e., they are organized in maps resembling those found in many cortical regions within the motor and perceptual areas. Due to computational limits, each map is represented by means of a 20 × 20 lattice (i.e., we just have 400 variations of the same feature) but, of course, a much finer map could be constructed in which features exhibit minimal nuances (such as in real cortical maps). We claim that this aspect of the model clearly differentiates it from a symbolic representation. The topological representation of features allows implementation of a similarity principle. Indeed, each feature excites an “activation bubble,” i.e., a group of neurons which respond to a similar attribute. This aspect again makes the model suitable to simulate perceptual or motor modalities, instead of abstract symbols. We are not aware of previous semantic models (with the exception of Miikkulainen, 1993, 1997) which implement this topological organization in the feature areas, although previous models have implemented a topology in the lexical area to organize words (Vigliocco et al., 2004). Another important aspect embodied in the model is that word recognition occurs by recreating over different cortical areas the same representation present when the object was originally learnt. Of course, we are aware that, at this level of modelization, our features cannot be completely distinguished from amodal symbols and in this regard our features resemble the “perceptual symbols” proposed by Barsalou. Future model application with more realistic data sets will replace the present schematic objects with real ones, using modal features in the different areas. This may allow more model predictions to be formulated and challenged against real data.
A further limitation of the present model is that we used just a localist representation of word-forms, whereas previous models included connections in the lexical area (see Miikkulainen, 1993, 1997; Vigliocco et al., 2004). In our model, possible connections among words occur just indirectly, i.e., are mediated by a correlation in the conceptual representation within the semantic net. It is likely that direct connections among words in the lexical network may be created by experience, especially if words occur frequently together or in close temporal proximity, even in the absence of a clear semantic correlation. Of course, a relationship among words may be of the utmost value to implement syntactic aspects in the model. The use of a localist representation of word-forms has been introduced to lay emphasis only on the semantic aspects. A more sophisticated description of lexical aspects (including a distributed representation of the activity in the net and connections among words) will be the object of future model improvement.
In conclusion, the present model provides a theoretical framework for the formalization of recent theories on the semantic-lexical memory system based on a grounded cognition approach. Original aspects consist in the possibility to manage multiple objects and words, and to distinguish between categories and individual members by learning distinctive and shared features on the basis of past experience. Although the present version only deals with simulated objects, it points out important aspects which may drive future research. These are especially concerned with the organization of the network and with the learning rules included. Subsequent versions of the model should consider the possibility to represent real objects and to simulate results of cognitive tests. This may permit the validation or rejection of hypotheses, a comparison with existing data, and the design of new tests.
The authors declare that the research was conducted in the absence of any commercial or financial relationships that could be construed as a potential conflict of interest.
Abbott, L. F., and Nelson, S. B. (2000). Synaptic plasticity: taming the beast. Nat. Neurosci. 3, 1178–1183.
Barsalou, L. W., Kyle Simmons, W., Barbey, A. K., and Wilson, C. D. (2003). Grounding conceptual knowledge in modality-specific systems. Trends Cogn. Sci. 7, 84–91.
Bhattacharya, J., Petsche, H., and Pereda, E. (2001). Long-range synchrony in the gamma band: role in music perception. J. Neurosci. 21, 6329–6337.
Borghi, A. M., and Cimatti, F. (2010). Embodied cognition and beyond: acting and sensing the body. Neuropsychologia 48, 763–773.
Caramazza, A., and Shelton, J. R. (1998). Domain-specific knowledge systems in the brain the animate-inanimate distinction. J. Cogn. Neurosci. 10, 1–34.
Cree, G. S., McNorgan, C., and McRae, K. (2006). Distinctive features hold a privileged status in the computation of word meaning: implications for theories of semantic memory. J. Exp. Psychol. Learn. Mem. Cogn. 32, 643–658.
Cree, G. S., McRae, K., and McNorgan, C. (1999). An attractor model of lexical conceptual processing: simulating semantic priming. Cogn. Sci. 23, 371–414.
Damasio, A. R. (1989). Time-locked multiregional retroactivation: a systems level proposal for the neural substrates of recall and recognition. Cognition 33, 25–62.
Damasio, H., Grabowski, T. J., Tranel, D., Hichwa, R. D., and Damasio, A. R. (1996). A neural basis for lexical retrieval. Nature 380, 499–505.
Davis, M. H., and Johnsrude, I. S. (2003). Hierarchical processing in spoken language comprehension. J. Neurosci. 23, 3423–3431.
Engel, A. K., and Singer, W. (2001). Temporal binding and the neural correlates of sensory awareness. Trends Cogn. Sci. 5, 16–25.
Farah, M. J., and McClelland, J. L. (1991). A computational model of semantic memory impairment: modality specificity and emergent category specificity. J. Exp. Psychol. Gen. 120, 339–357.
Gainotti, G. (2000). What the locus of brain lesions tells us about the nature of the cognitive defect underlying category-specific disorders: a review. Cortex 36, 539–559.
Gainotti, G. (2005). The influence of gender and lesion location on naming disorders for animals, plants and artefacts. Neuropsychologia 43, 1633–1644.
Giraud, A. L., Kell, C., Thierfelder, C., Sterzer, P., Russ, M. O., Preibisch, C., and Kleinschmidt, A. (2004). Contributions of sensory input, auditory search and verbal comprehension to cortical activity during speech processing. Cereb. Cortex 14, 247–255.
Goldberg, R. F., Perfetti, C. A., and Schneider, W. (2006). Perceptual knowledge retrieval activates sensory brain regions. J. Neurosci. 26, 4917–4921.
Gonnerman, L. M., Andersen, E. S., Devlin, J. T., Kempler, D., and Seidenberg, M. S. (1997). Double dissociation of semantic categories in Alzheimer’s disease. Brain Lang. 57, 254–279.
Gonzalez, J., Barros-Loscertales, A., Pulvermuller, F., Meseguer, V., Sanjuan, A., Belloch, V., and Avila, C. (2006). Reading cinnamon activates olfactory brain regions. Neuroimage 32, 906–912.
Hart, J., Anand, R., Zoccoli, S., Maguire, M., Gamino, J., Tillman, G., King, R., and Kraut, M. A. (2007). Neural substrates of semantic memory. J. Int. Neuropsychol. Soc. 13, 865–880.
Hart, J., Moo, L. R., Segal, J. B., Adkins, E., and Kraut, M. A. (2002). “Neural substrates of semantics,” in Handbook of Language Disorders, ed. A. Hillis (Philadelphia, PA: Psychology Press), 207–228.
Hauk, O., Johnsrude, I., and Pulvermüller, F. (2004). Somatotopic representation of action words in human motor and premotor cortex. Neuron 41, 301–307.
Hertz, J., Krogh, A., and Palmer, R. G. (1991). Introduction to the Theory of Neural Computation. Redwood City, CA: Addison-Wesley Publishing Company.
Hinton, G. E., and Shallice, T. (1991). Lesioning an attractor network: investigations of acquired dyslexia. Psychol. Rev. 98, 74–95.
Hopfield, J. J., and Brody, C. D. (2001). What is a moment? Transient synchrony as a collective mechanism for spatiotemporal integration. Proc. Natl. Acad. Sci. U.S.A. 98, 1282–1287.
Humphreys, G. W., and Forde, E. M. E. (2001). Hierarchies, similarity, and interactivity in object recognition: “category-specific” neuropsychological deficits. Behav. Brain Sci. 24, 453–476.
Kraut, M. A., Pitcock, J. A., and Hart, J. (2004). Neural mechanisms of semantic memory. Curr. Neurol. Neurosci. Rep. 4, 461–465.
Kroll, J. F., and Stewart, E. (1994). Category interference in translation and picture naming: evidence for asymmetric connections between bilingual memory representations. J. Mem. Lang. 33, 149–174.
Lambon Ralph, M. A., Lowe, C., and Rogers, T. T. (2007). Neural basis of category-specific demantic deficits for living things: evidence from semantic dementia, HSVE and a neural network model. Brain 130, 1127–1137.
Markram, H., Lubke, J., Frotscher, M., and Sakmann, B. (1997). Regulation of synaptic efficacy by coincidence of postsynaptic APs and EPSPs. Science 275, 213–215.
Martin, A. (2007). The representation of object concepts in the brain. Annu. Rev. Psychol. 58, 25–45.
Martin, A., and Chao, L. L. (2001). Semantic memory and the brain: structure and processes. Curr. Opin. Neurobiol. 11, 194–201.
McGuire, S., and Plaut, D. C. (1997). Systematicity and Specialization in Semantics: A Computational Account of Optic Aphasia. Hillsdale, NJ: Lawrence Erlbaum Associates, Inc., 502–507.
McRae, K., de Sa, V. R., and Seidenberg, M. S. (1997). On the nature and scope of featural representations of word meaning. J. Exp. Psychol. Gen. 126, 99–130.
Melloni, L., Molina, C., Pena, M., Torres, D., Singer, W., and Rodriguez, E. (2007). Synchronization of neural activity across cortical areas correlates with conscious perception. J. Neurosci. 27, 2858–2865.
Miikkulainen, R. (1993). Subsymbolic Natural Language Processing: An Integrated Model of Scripts, Lexicon, and Memory. Cambridge, MA: MIT Press.
Miikkulainen, R. (1997). Dyslexic and category-specific aphasic impairments in a self-organizing feature map model of the Lexicon. Brain Lang. 59, 334–366.
Osipova, D., Takashima, A., Oostenvald, R., Fernández, G., Maris, E., and Jensen, O. (2006). Theta and gamma oscillations predict encoding and retrieval of declarative memory. J. Neurosci. 26, 7523–7531.
Pexman, P. M., Holyk, G. G., and Monfils, M. H. (2003). Number-of-features effects and semantic processing. Mem. Cogn. 31, 842–855.
Pexman, P. M., Lupker, S. J., and Hino, Y. (2002). The impact of feedback semantics in visual word recognition: number-of-features effects in lexical decision and naming tasks. Psychon. Bull. Rev. 9, 542–549.
Plaut, D. C. (2002). Graded modality-specific specialisation in semantics: a computational account of optic aphasia. Cogn. Neuropsychol. 19, 603–639.
Plaut, D. C., and Booth, J. R. (2000). Individual and developmental differences in semantic priming: empirical and computational support for a single-mechanism account of lexical processing. Psychol. Rev. 107, 786–823.
Potter, M. C., So, K. F., Eckardt, B. V., and Feldman, L. B. (1984). Lexical and conceptual representation in beginning and proficient bilinguals. J. Verbal Learn. Verbal Behav. 23, 23–38.
Pulvermüller, F., Preissl, H., Lutzenberger, W., and Birbaumer, N. (1996). Brain rhythms of language: nouns versus verbs. Eur. J. Neurosci. 8, 937–941.
Randall, B., Moss, H. E., Rodd, J. M., Greer, M., and Tyler, L. K. (2004). Distinctiveness and correlation in conceptual structure: behavioral and computational studies. J. Exp. Psychol. Learn. Mem. Cogn. 30, 393–406.
Rodd, J. M., Davis, M. H., and Johnsrude, I. S. (2005). The neural mechanisms of speech comprehension: fMRI studies of semantic ambiguity. Cereb. Cortex 15, 1261–1269.
Rogers, T. T., Lambon Ralph, M. A., Garrard, P., Bozeat, S., McClelland, J. L., Hodges, J. R., and Patterson, K. (2004). Structure and deterioration of semantic memory: a neuropsychological and computational investigation. Psychol. Rev. 111, 205–235.
Salinas, E., and Sejnowski, T. J. (2001). Correlated neuronal activity and the flow of neural information. Nat. Rev. Neurosci. 2, 539–550.
Siekmeier, P. J., and Hoffman, R. E. (2002). Enhanced semantic priming in schizophrenia: a computer model based on excessive pruning of local connections in association cortex. Br. J. Psychiatry 180, 345–350.
Simmons, W. K., and Barsalou, L. W. (2003). The similarity-in-topography principle: reconciling theories of conceptual deficits. Cogn. Neuropsychol. 20, 451–486.
Simmons, W. K., Martin, A., and Barsalou, L. W. (2005). Pictures of appetizing foods activate gustatory cortices for taste and reward. Cereb. Cortex 15, 1602–1608.
Slotnick, S. D., Moo, L. R., Kraut, M. A., Lesser, R. P., and Hart, J. (2002). Interactions between thalamic and cortical rhythms during semantic memory recall in human. Proc. Natl. Acad. Sci. U.S.A. 99, 6440–6443.
Steriade, M. (2000). Corticothalamic resonance, states of vigilance and mentation. Neuroscience 101, 243–276.
Tallon-Baudry, C., Bertrand, O., Delpuech, C., and Pernier, J. (1997). Oscillatory gamma-band (30–70 Hz) activity induced by a visual search task in humans. J. Neurosci. 17, 722–734.
Tallon-Baudry, C., Bertrand, O., Peronnet, F., and Pernier, J. (1998). Induced gamma-band activity during the delay of a visual short-term memory task in humans. J. Neurosci. 18, 4244–4254.
Tyler, L. K., and Moss, H. E. (2001). Towards a distributed account of conceptual knowledge. Trends Cogn. Sci. 5, 244–252.
Tyler, L. K., Moss, H. E., Durrant-Peatfield, M. R., and Levy, J. P. (2000). Conceptual structure and the structure of concepts: a distributed account of category-specific deficits. Brain Lang. 75, 195–231.
Ursino, M., Magosso, E., and Cuppini, C. (2009). Recognition of abstract objects via neural oscillators: interaction among topological organization, associative memory and gamma band synchronization. IEEE Trans. Neural Netw. 20, 316–335.
Vigliocco, G., Vinson, D. P., Lewis, W., and Garrett, M. F. (2004). Representing the meaning of objects and action words: the featural and unitary semantic space hypothesis. Cogn. Psychol. 48, 422–488.
Warrington, E. K., and McCarthy, R. A. (1987). Categories of knowledge: further fractionations and an attempted integration. Brain 110, 1273–1296.
Keywords: gamma-band oscillations, synchronization, Hebbian rules, word production, word recognition, object recognition, lexical deficit, categorization
Citation: Ursino M, Cuppini C and Magosso E (2010) A computational model of the lexical-semantic system based on a grounded cognition approach. Front. Psychology 1:221. doi: 10.3389/fpsyg.2010.00221
Received: 15 July 2010;
Accepted: 20 November 2010;
Published online: 08 December 2010.
Edited by:
Anna M. Borghi, University of Bologna and Institute of Cognitive Sciences and Technologies, ItalyReviewed by:
Ken Mcrae, University of Western Ontario, CanadaCopyright: © 2010 Ursino, Cuppini and Magosso. This is an open-access article subject to an exclusive license agreement between the authors and the Frontiers Research Foundation, which permits unrestricted use, distribution, and reproduction in any medium, provided the original authors and source are credited.
*Correspondence: Mauro Ursino, Department of Electronics, Computer Science and Systems, University of Bologna, Viale Risorgimento 2, I40136 Bologna, Italy. e-mail:bWF1cm8udXJzaW5vQHVuaWJvLml0
Disclaimer: All claims expressed in this article are solely those of the authors and do not necessarily represent those of their affiliated organizations, or those of the publisher, the editors and the reviewers. Any product that may be evaluated in this article or claim that may be made by its manufacturer is not guaranteed or endorsed by the publisher.
Research integrity at Frontiers
Learn more about the work of our research integrity team to safeguard the quality of each article we publish.