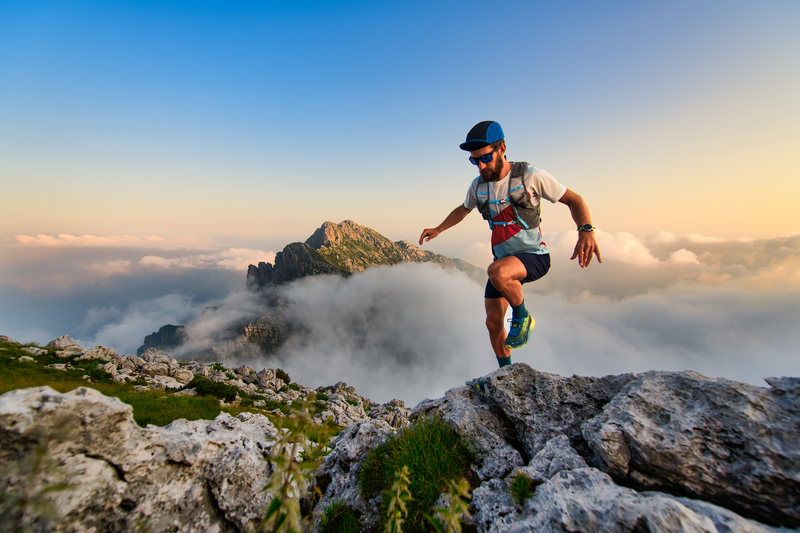
95% of researchers rate our articles as excellent or good
Learn more about the work of our research integrity team to safeguard the quality of each article we publish.
Find out more
REVIEW article
Front. Polit. Sci. , 20 March 2025
Sec. Politics of Technology
Volume 7 - 2025 | https://doi.org/10.3389/fpos.2025.1561776
This article is part of the Research Topic Human Rights and Artificial Intelligence View all articles
Frontier AI systems, including large-scale machine learning models and autonomous decision-making technologies, are deployed across critical sectors such as finance, healthcare, and national security. These present new cyber-risks, including adversarial exploitation, data integrity threats, and legal ambiguities in accountability. The absence of a unified regulatory framework has led to inconsistencies in oversight, creating vulnerabilities that can be exploited at scale. By integrating perspectives from cybersecurity, legal studies, and computational risk assessment, this research evaluates regulatory strategies for addressing AI-specific threats, such as model inversion attacks, data poisoning, and adversarial manipulations that undermine system reliability. The methodology involves a comparative analysis of domestic and international AI policies, assessing their effectiveness in managing emerging threats. Additionally, the study explores the role of cryptographic techniques, such as homomorphic encryption and zero-knowledge proofs, in enhancing compliance, protecting sensitive data, and ensuring algorithmic accountability. Findings indicate that current regulatory efforts are fragmented and reactive, lacking the necessary provisions to address the evolving risks associated with frontier AI. The study advocates for a structured regulatory framework that integrates security-first governance models, proactive compliance mechanisms, and coordinated global oversight to mitigate AI-driven threats. The investigation considers that we do not live in a world where most countries seem to be wishing to follow European Union ideals, and in the wake of this particular trend, this research presents a regulatory blueprint that balances technological advancement with decentralised security enforcement.
Artificial intelligence (AI) has become an integral part of modern technology, influencing various sectors and prompting a critical examination of its applications and implications. AI technologies have changed our interaction with digital intelligence, and created new ethical, privacy, and security challenges.
These challenges are pervasive, affecting numerous aspects of human life, and require new regulatory frameworks. The development and deployment of AI technologies, including Generative AI, present complex ethical, privacy concerns, and security risks.
The regulation of AI must be viewed through an ethical and idealistic lens, but also as a strategic challenge situated within a global context of geopolitical tensions, economic competition, and adversarial uses of AI. The notion that AI regulation can be implemented in a uniform and cooperative manner is complicated by the reality that many nations prioritise competitiveness, sovereignty, and national security over collective governance efforts. As such, AI regulation should integrate mechanisms to reduce (or even eliminate) risks stemming from disinformation, cybersecurity threats, and the weaponisation of AI.
This requires risk-based governance models alongside aspirational ethical frameworks. The dual-use nature of AI, where technologies designed for beneficial applications can also be repurposed for malicious use, requires adaptability in regulatory frameworks. Countries may need to balance transparency requirements with national security considerations, as complete openness regarding AI decision-making mechanisms could expose vulnerabilities that adversaries might exploit.
Furthermore, AI systems can exhibit near-untraceable behaviours, particularly when using advanced deep learning and adversarial learning techniques. Existing explainability methods (e.g., LIME, SHAP) provide limited insight into these systems, as complex neural networks often lack interpretability beyond localised approximations. More comprehensive strategies, including the development of new traceability metrics and governance mechanisms, are necessary to ensure oversight without stifling innovation. The efforts by INAIT and similar initiatives in enhancing traceability should be further explored as potential models for embedding greater transparency into AI governance.
As new technologies advance, they require legal and regulatory compliance frameworks to ensure ethical use, privacy, and security. The new regulations and guidelines include global regulations such as the EU’s Artificial Intelligence Act (European Parliament, 2023) and the USA’s National AI Initiative Act (The White House, 2023), which focus on establishing a legal framework for AI systems based on risk, AI research and development, and ethical guidelines. China’s New Data Security Law emphasises data security, user privacy, and the role of AI in national security. There are sector-specific guidelines, such as HIPAA (1996) in the USA, designed for healthcare, which may evolve to address AI in patient data handling. The guidelines for AI in algorithmic trading and risk assessment are different in finance. The ethical frameworks for legal and regulatory compliance for AI include UNESCO’s Recommendation on the Ethics of AI, which offers a global standard for ethical AI, and IEEE’s Ethically Aligned Design, which prioritises human rights in AI. The privacy regulations include updates in the General Data Protection Regulation (GDPR) (ICO, 2018; GDPR, 2018), considering AI’s role in data processing, and the CCPA (2018), which includes AI data handling provisions.
Nations need to establish clear ethical guidelines and standards to govern the development and use of AI. These guidelines should address various concerns, including privacy, transparency, bias, and accountability. The GDPR is a prime example of a framework for managing personal data, which can be applied to AI. The GDPR is intended to give individuals more control over their personal data, while ensuring that companies that collect and use such data are held accountable for their actions. By adopting similar ethical guidelines and standards, countries can ensure that AI is developed and employed fairly, openly, and responsibly. Such guidelines can be critical in the development public confidence in AI and promoting its responsible use. In Table 1, the AI uses cases are categorised and analysed according to use cases.
Regulations governing the use of AI must be personalised to specific sectors, given the extensive range of its applications—see Table 1. For example, in the healthcare sector, stringent regulations concerning AI’s role in diagnosis and the handling of patient data are crucial. Similarly, in the finance sector, regulations for AI should focus on detecting fraud and algorithmic trading. Governments can establish oversight committees to monitor AI research and development, including funding ethical AI research and promoting practices that prevent the creation of harmful AI technologies.
Establishing global standards for AI, like the Paris Agreement for climate change, is the next step in ensuring AI is safe and ethical use. These standards should address issues such as the AI arms race, autonomous weapons, and global surveillance systems. Encouraging international cooperation in AI research and focusing on the ethical and safe development of AI technologies is essential. Sharing best practices, research findings, and ethical guidelines can facilitate collaboration and lead to more effective outcomes. Since AI systems often rely on data that crosses borders, it is vital to establish international agreements on data governance, privacy, and security. The EU-US Privacy Shield (European Court of Justice, 2020) can be a starting point for these agreements. However, as a result of the Schemes II decision, the EU-U.S. Privacy Shield Framework is no longer a valid mechanism to comply with EU data protection requirements when transferring personal data from the European Union to the United States.
AI cybersecurity capacity-building is a priority for nations and organisations looking to establish secure AI-driven infrastructures. The integration of AI across critical domains, such as healthcare, financial systems, autonomous systems, and national security, requires a targeted investments in AI-specific cybersecurity frameworks. Countries with advanced AI capabilities must lead global initiatives to develop standardised security benchmarks, model resilience assessment protocols, and AI incident response mechanisms.
The European Union’s Artificial Intelligence Act and the US AI Executive Order both recognise the need for enhanced AI security measures, yet there remains a lack of international coordination in AI cybersecurity policy. The establishment of an AI Cybersecurity Capacity Centre, modeled after cybersecurity capacity-building initiatives like the UK’s Global Cybersecurity Capacity Centre (n.d.), could serve as a dedicated global effort to strengthen AI security policies, research funding, and defensive AI strategies. AI regulatory frameworks should incentivise investment in research areas such as:
AI threat intelligence to monitor and predict adversarial AI attack trends.
Secure AI software supply chains to prevent exploitation of AI models through dependencies.
Trusted AI hardware solutions that mitigate risks from hardware-based attacks.
Automated AI security audits that continuously validate AI model integrity.
Such investments would ensure that AI development aligns with security principles while maintaining interoperability across global AI governance frameworks.
Regulations must be flexible enough to accommodate new technologies that may emerge. However, divergent international interests make it challenging to reach a global agreement, as different countries have varying priorities and ethical standards. Additionally, technologically advanced nations may have different interests than developing countries, making enforcing regulations locally and internationally difficult.
AI systems, particularly large-scale models such as generative AI and reinforcement learning systems, face unique cybersecurity threats that extend beyond traditional software vulnerabilities. Adversarial machine learning (AML) attacks, such as evasion attacks, model inversion, data poisoning, and backdoor attacks, present new risks to AI reliability, security, and integrity. For instance, AI systems used in fraud detection, medical diagnosis, and national security can be manipulated through adversarial perturbations, which subtly alter inputs to cause incorrect or misleading outputs without being detected.
Understanding AI attack surfaces is pre-requirement for securing AI-driven ecosystems. Attack vectors can exploit weaknesses in model architecture, training data provenance, and inference-time decision-making. Deep learning-based AI systems, for example, are susceptible to gradient-based perturbation attacks (Naqvi et al., 2024) that force models to misclassify data (Lung, 2023) while remaining undetected (Naqvi et al., 2024). Moreover, model inversion attacks threaten privacy by reconstructing sensitive training data (Fredrikson et al., 2015), exposing personal information embedded within AI models (He et al., 2019). Addressing these risks requires AI governance frameworks that mandate security-by-design principles (CISA, 2023), continuous monitoring, and adversarial testing during AI deployment.
AI governance does not exist in a vacuum, it operates within a competitive and adversarial global context where national interests, geopolitical rivalries, and economic competitiveness shape regulatory efforts. While ideal governance frameworks emphasise ethical principles and collective responsibility, real-world implementation faces significant resistance from nations prioritising AI supremacy, national security, and economic dominance.
One of the major challenges in AI governance is the strategic use of AI for disinformation, cyber warfare, and economic manipulation. The role of AI in hybrid warfare, where adversarial states deploy AI-powered misinformation campaigns and exploit AI-driven vulnerabilities in cybersecurity infrastructures, highlights the necessity of regulation that is not just ethical but also strategically resilient. The EU AI Act and similar governance efforts must be adaptive to real-world power struggles, incorporating defensive mechanisms that ensure compliance without stifling innovation.
Additionally, AI’s near-untraceable decision-making processes pose unique challenges. Deep learning models, particularly adversarial networks (Goodfellow et al., 2014), can operate in ways that evade traditional explainability techniques (Ozdag, 2018). Existing methods such as LIME and SHAP provide limited traceability, necessitating more advanced solutions such as adversarial robustness testing, causal inference models, and cryptographic audit trails. The INAIT initiative and emerging AI transparency methodologies are promising, but broader interdisciplinary research is required to balance AI explainability with performance efficiency.
To avoid overly idealistic regulatory proposals, a more pragmatic governance approach must include staged implementation strategies, international negotiation mechanisms, and sector-specific compliance pathways. A key lesson from cybersecurity policy development is that rigid, one-size-fits-all regulations fail in dynamic adversarial environments. AI governance should therefore embrace flexible, incentive-driven policies that encourage compliance while accounting for competitive realities.
The digital divide (Whyte, 2018) remains a major challenge in AI governance, as access to AI-driven technologies is largely dictated by financial resources, digital literacy, and regional infrastructure. AI regulation cannot be effective unless it considers the socio-economic barriers that prevent equitable access to AI benefits. In developing nations, limited AI research capabilities, inadequate computing infrastructure, and restricted AI education create a significant gap in AI adoption. Even within developed countries, lower-income populations face exclusion from AI-driven economic opportunities due to lack of access to computational resources.
AI regulation should therefore include provisions for reducing the digital divide by incentivising AI education programs, funding community-based AI research, and mandating inclusive AI development policies. Governments and AI firms should collaborate to establish AI accessibility initiatives that prioritise digital equity, ensuring that underprivileged groups can benefit from AI advancements without being disproportionately affected by AI-induced economic disruptions.
Creating AI governance committees and conducting regular system audits can help ensure accuracy, mitigate bias, and guarantee ethical alignment. The AI governance committee should comprise experts in AI/ML, data privacy, and ethics. The committee should be responsible for monitoring the use of AI/ML systems in the organisation, addressing ethical concerns, and creating guidelines for their use. Regular system audits can help identify issues and ensure they perform as intended.
Organisations must also comply with data privacy laws when implementing AI/ML systems. This involves using data anonymisation techniques and adhering to regulatory requirements like GDPR. Data anonymisation techniques can help protect sensitive information, such as personal data while allowing the AI/ML system to perform its intended function.
Regular assessments should be conducted to reduce potential risks associated with AI/ML systems, and plans should be implemented to address any potential risks. This includes assessing the accuracy of the system’s outputs, identifying and mitigating any biases, and ensuring that the system aligns with ethical standards. Regular training sessions should be provided to ensure that employees are knowledgeable about AI ethics and legal obligations. This training should cover AI ethics, data privacy, and regulatory requirements. Public awareness campaigns should also be launched to educate the public about the capabilities and limitations of AI systems. This can help address concerns about AI’s impact on jobs, privacy, and security.
AI systems do not operate in isolation, they exist within complex ecosystems of cloud infrastructures, data pipelines, federated learning networks, and API-driven architectures. The interdependency of AI systems introduces cascading cybersecurity risks, where vulnerabilities in one model or dataset can propagate across supply chains. For example, data poisoning in a foundational model can lead to compromised downstream AI services, impacting multiple sectors that rely on the model’s outputs. Similarly, supply chain attacks on pre-trained models, where adversaries embed undetectable manipulations, can result in the silent exploitation of AI systems in finance, healthcare, and critical infrastructure.
To manage these risks, regulatory frameworks must incorporate AI security standards that enforce stringent vetting of AI models, continuous adversarial robustness assessments, and secure model distribution policies. AI security capacity-building efforts should prioritise defensive mechanisms such as adversarial training, differential privacy, homomorphic encryption, and federated trust frameworks to prevent risk propagation across AI-driven supply chains.
The GDPR (2018) is a crucial piece of legislation in the European Union and the United Kingdom (ICO, 2018) that focuses on data protection and privacy. After Brexit, the UK retained GDPR in domestic law as the UK GDPR. However, worth mentioning that the UK has the independence to keep the framework under review. The UK GDPR (ICO, 2018) is integrated with an amended version of the Data Protection Act 2018 (GOV.UK, 2018).
The GDPR has significant implications for AI and ML systems, particularly in how they process, store and use personal data. To comply with GDPR regulations, companies are modifying their AI systems in several ways. They are redesigning AI systems to collect only the data required for their specific purpose, following the GDPR principle of data minimisation. Companies also ensure that their AI systems are transparent about the data they collect and process, aligning with the purpose limitation principle of GDPR. To further enhance data subject rights, companies are implementing mechanisms that facilitate user rights under GDPR, such as the right to access, the right to be forgotten, and the right to data portability. They are also developing AI solutions that can efficiently handle requests for data erasure or modification.
GDPR restricts automated decision-making that significantly impacts individuals. Companies are incorporating human oversight into AI decision-making processes to comply with this. They are also developing explainable AI models to provide transparency and understanding of decision-making. Companies are conducting Data Protection Impact Assessments (DPIA) for AI projects to identify and mitigate data protection risks. They also ensure that DPIAs are integral to the AI development lifecycle. To comply with GDPR regulations, companies are utilising advanced data anonymisation techniques to ensure that AI systems do not unintentionally reveal personal data. They are also balancing the need for high-quality data in AI with the privacy requirements of GDPR.
The impact of GDPR on AI-driven businesses is multifaceted and complex. One of the most significant impacts has been the increased compliance costs for companies. These costs include investing in legal, technical, and operational measures to ensure GDPR compliance, which can be a significant financial burden, particularly for small and medium-sized enterprises. The GDPR has also presented innovation challenges for some AI initiatives, particularly those in data-intensive areas like machine learning. Companies may need to adjust their AI initiatives to meet the stringent requirements of GDPR. However, it is worth noting that these challenges could also present opportunities for innovation by fostering more transparent, accountable, and ethical AI systems.
One of the key benefits of GDPR compliance is the competitive advantage it offers. Companies that ensure GDPR compliance, can gain consumer trust and market reputation. GDPR has set a benchmark for data privacy laws globally, and companies operating in multiple jurisdictions might adopt GDPR-compliant practices as a standard, influencing AI development worldwide.
Another significant impact of GDPR on AI-driven businesses is the enhanced consumer trust it fosters. By adhering to GDPR, companies can enhance their credibility and build trust with consumers increasingly concerned about data privacy. This can also lead to increased customer loyalty and brand reputation. GDPR is also pushing companies to consider the ethical implications of AI, fostering a more responsible approach to AI development. This approach can help mitigate the risks associated with AI, such as biased or discriminatory outcomes, and ensure that AI is developed in a way aligned with societal values and expectations.
Machine Learning for Governance, Automated Compliance Monitoring is a new field where ML algorithms can be trained to monitor and report on compliance with regulatory requirements. In the financial sector, ML can detect anomalies that indicate non-compliance with regulations such as anti-money laundering laws. Similarly, Predictive Analysis for Treaty Compliance involves using ML to analyse vast amounts of data to predict potential treaty violations. This is particularly useful in environmental treaties where ML can forecast environmental impacts or in arms control treaties to monitor prohibited activities.
Another area is Natural Language Processing (NLP) for Legal Analysis, where NLP techniques can automate the interpretation of legal texts and treaties, making it easier to understand compliance requirements and facilitating faster regulatory reviews.
There are also new Computing Hardware Advancements, such as High-Performance Computing (HPC), which can process enormous datasets necessary for comprehensive compliance monitoring. This is crucial in sectors like climate science, where large-scale simulations are essential for treaty compliance. Another similar technology is Quantum Computing (Mallow et al., 2022; Marais et al., 2022; Sevilla and Moreno, 2019; Awan et al., 2022; Alyami et al., 2021; Gupta et al., 2023), which, although still in its early stages, promises unprecedented capabilities in analysing and monitoring treaty compliance.
A more developed technology is Edge Computing for Real-Time Monitoring, which is used for deploying edge computing devices to enable real-time monitoring and data processing at the source. This is crucial for immediate compliance enforcement in industries like manufacturing and energy.
Cryptographic techniques such as blockchain (He et al., 2022; Hazra et al., 2022; Wylde et al., 2022; Androulaki et al., 2018; Dong et al., 2018), secure multi-party computation, homomorphic encryption, and zero-knowledge proofs can be applied for effective AI governance. These technologies provide powerful tools for technical governance that can significantly enhance the ability of governments and regulatory bodies to monitor compliance, predict potential violations, and enforce regulations and treaties more effectively.
For example, blockchain technology can provide a transparent and immutable ledger that is useful for tracking compliance in supply chains and international trade. Similarly, Secure Multi-Party Computation allows multiple parties to jointly compute a function over their inputs while keeping those inputs private, beneficial in scenarios where data sharing is sensitive but necessary for compliance.
Homomorphic Encryption (HE) (Nita and Mihailescu, 2023) can enable computations on encrypted data, allowing regulatory bodies to verify compliance without compromising the privacy of the underlying data. Zero-Knowledge Proofs, on the other hand, can prove the compliance of an entity without revealing the actual data, maintaining privacy while ensuring regulatory oversight.
However, the deployment of these technologies must be balanced with ethical considerations and privacy protection, ensuring that governance is efficient and respectful of individual rights and freedoms. It is crucial to consider ethical implications and privacy concerns when implementing these technologies, especially in areas like surveillance and personal data processing.
To effectively use these technologies, interoperability standards are needed to ensure that systems can communicate and share data securely. Developing integrated platforms that combine ML, advanced computing, and cryptographic techniques can offer comprehensive solutions for monitoring and ensuring compliance.
Homomorphic Encryption (HE) is a method which allows calculations to be carried out on encrypted data (Nita and Mihailescu, 2023), producing an encrypted output that mirrors the result of operations performed on the original unencrypted data. This feature of HE makes it an incredibly valuable tool for conducting privacy-preserving computations in regulatory compliance.
HE finds numerous applications in governance, such as in data privacy during compliance audits. For instance, financial institutions can use HE to demonstrate compliance with regulatory requirements without sacrificing the confidentiality of individual customer data. What’s more, regulatory bodies frequently require aggregated data from multiple sources for compliance monitoring, and HE can securely aggregate this data while ensuring that individual data points remain encrypted and safeguarded. HE can also be particularly useful in situations where data needs to be shared across borders for treaty compliance, as it ensures that data remains encrypted throughout the entire process, thereby enabling compliance with data protection laws like GDPR. Additionally, HE enables machine learning models to be trained using encrypted data, which can be a boon for regulatory bodies that use machine learning for compliance monitoring but are constrained by privacy concerns.
However, HE is computationally intensive, which can impede its widespread adoption in real-time compliance monitoring. Additionally, implementing HE solutions can be complex and requires specialised knowledge, which can challenge regulatory bodies with limited technical expertise.
While cryptographic solutions such as homomorphic encryption and zero-knowledge proofs enhance AI privacy and compliance, they also introduce security risks in the absence of an internationally standardised identity management system. Anonymisation mechanisms can be exploited by malicious actors, including cybercriminals and state-sponsored hackers, to evade legal scrutiny. The dark web and cybercrime networks have already begun using cryptographic AI tools for untraceable transactions, illicit data trading, and adversarial AI deployment.
AI governance must therefore balance the benefits of cryptographic security with the risks of unchecked anonymity. One potential solution is the implementation of multi-tiered encryption policies, where regulatory bodies retain conditional oversight over AI systems handling sensitive national security data. Additionally, international cooperation is required to establish ethical AI cryptographic norms that prevent adversarial exploitation while safeguarding individual privacy rights. National security-driven AI regulations should integrate threat intelligence mechanisms that proactively monitor AI-driven cyber risks while ensuring that encryption standards do not enable undetectable AI misuse.
Homomorphic encryption can be categorised into three types: Partially Homomorphic Encryption (PHE), Somewhat Homomorphic Encryption (SWHE), and Fully Homomorphic Encryption (FHE).
Partially Homomorphic Encryption (PHE) supports a single type of operation, such as only addition or multiplication, on encrypted data. This limited functionality, represented in the blue box of Table 2, is particularly suitable for specific applications that require simple arithmetic on encrypted data. Examples include secure voting systems and data anonymisation, where basic operations on data are sufficient to achieve the desired outcomes without compromising data security.
Somewhat Homomorphic Encryption (SWHE), depicted in the green box, extends the capabilities of PHE by supporting addition and multiplication operations, though the number of these operations is limited. This type of encryption allows for a sequence of arithmetic operations on encrypted data, making it useful for more complex applications like encrypted search and basic data analytics. SWHE strikes a balance between functionality and efficiency, enabling more intricate computations while maintaining a degree of operational simplicity.
Fully Homomorphic Encryption (FHE), illustrated in the red box, represents the most advanced form of homomorphic encryption (Gentry et al., 2012). FHE supports an unlimited number of operations, including any number of additions and multiplications, on encrypted data. This capability allows for the performance of complex arithmetic and algorithms directly on encrypted data, making FHE ideal for sophisticated applications such as complex data analytics and machine learning. The ability to conduct comprehensive analyses and develop models on encrypted data without compromising privacy is a significant advantage of FHE.
The distinctions between PHE, SWHE, and FHE highlight the trade-offs between functionality, complexity, and computational overhead. While PHE and SWHE offer more efficient solutions for specific tasks with lower computational requirements, FHE provides unparalleled flexibility and security for applications demanding extensive data manipulation and analysis. Table 2 describes the differences between PHE, SWHE, and FHE. Understanding these types of homomorphic encryption and their respective applications is crucial for selecting the appropriate method to ensure data privacy and security in various contexts.
Table 2 explains that homomorphic encryption enables different levels of secure data processing while preserving privacy. As the need for data security continues to grow, the application of homomorphic encryption will become increasingly vital in areas ranging from secure voting systems to advanced machine learning. Table 2 summarises these types and their respective applications, providing a clear overview of the capabilities and potential uses of homomorphic encryption in maintaining data security and privacy.
Zero-knowledge proofs (ZKPs) (Yang and Li, 2020) are a cryptographic technique that allow one party to prove the truth of a statement to another party without revealing any additional information beyond the fact that the statement is true (Zhang et al., 2021), making them a powerful tool for enhancing governance (Liu et al., 2024).
ZKPs have a wide range of potential applications, especially in regulatory compliance. For instance, in industries where sensitive or proprietary information is maintained, ZKPs can be used to demonstrate compliance with regulatory requirements without revealing confidential data. Similarly, in financial regulation, ZKPs can be used to prove the legitimacy of transactions without disclosing sensitive details, thereby supporting efforts to combat financial crimes such as money laundering.
Another significant advantage of ZKPs is that they enable secure, privacy-preserving compliance checks between organisations. This can be particularly useful in collaborative projects or joint ventures where sensitive information cannot be fully shared. By using ZKPs, organisations can ensure that each party meets its regulatory obligations without compromising the confidentiality of any data.
However, implementing ZKPs in governance can be challenging. ZKPs are complex and require significant computational resources, making them difficult to use in large-scale applications. Moreover, integrating ZKP solutions into existing regulatory compliance systems can be challenging and may require substantial modifications.
Advanced cryptographic techniques like Homomorphic Encryption (Gentry, 2009) and Zero-Knowledge Proofs present a promising opportunity to enhance privacy and security in regulatory compliance. While they offer solutions for securely handling sensitive data, their complexity and computational demands pose challenges that require attention. As these technologies continue to evolve and become more accessible, we can expect to see increased adoption in technical governance, providing more efficient and privacy-respecting methods for ensuring compliance. These mechanisms are essential for mitigating risks and ensuring the ethical and safe development and deployment of these technologies across various jurisdictions.
These mechanisms are essential for mitigating risks and ensuring the ethical and safe development and deployment of these technologies across various jurisdictions. The establishment of global industrial and commercial standards is vital for ensuring efficient operations. Prominent international bodies, including the ISO (2017) and the International Electrotechnical Commission (IEC) (Shaaban et al., 2018), have been vital in creating standards across numerous industries, including AI and cybersecurity. The International Telecommunication Union (2018) has similarly contributed by setting worldwide standards for telecommunications and IT, with a significant focus on cybersecurity and AI.
In the United States, the National Institute of Standards and Technology (2023) plays a crucial role in developing frameworks that often achieve international adoption. Another key player is the Institute of Electrical and Electronics Engineers (2023), which works to develop global standards that influence the design and implementation of AI and computing technologies. Monitoring and enforcement of these standards are critical for ensuring compliance. Various United Nations agencies, such as UNESCO, lead the way in establishing ethical standards for AI, while the ITU focuses on telecommunication and cyber norms. The UN Group of Governmental Experts (GGE) contributes by creating international norms and monitoring aspects like cyber warfare, AI, and lethal autonomous weapons systems (LAWS).
To promote responsible behaviour in cyberspace, international cybersecurity alliances such as the Paris Call for Trust and Security in Cyberspace are instrumental in establishing global norms. Collaborative research initiatives, like Horizon Europe, foster joint AI and cybersecurity research, promoting shared standards and ethical guidelines. Bodies like the European AI Alliance facilitate international collaboration in AI research and policy-making. Partnerships between universities, research institutes, and industries across countries help in establishing common research agendas and ethical guidelines. Arms control remains a critical issue within international relations. Frameworks such as the UN Conference on Disarmament play a key role in negotiating international treaties regarding emergent warfare technologies, including cyber weapons and autonomous weapons systems. However, there is a growing need for new treaties and agreements that specifically address issues like cyber warfare and autonomous weapons, akin to the Chemical Weapons Convention.
Despite progress, several challenges and future directions persist in developing effective international governance structures. Harmonising diverse interests remains a significant challenge, with different countries having varying policies and priorities. The fast pace of technological advancement makes it difficult for international norms and institutions to keep up. Enforcement mechanisms for international agreements, particularly in areas like cybersecurity, are complex and often lack clear jurisdictional authority. Ensuring broad participation, including from developing countries, is essential for establishing truly global governance of emerging technologies. International norms and institutions are critical in mitigating risks associated with AI, cybersecurity, and related technologies across jurisdictions. While significant progress has been made, ongoing efforts are required to adapt to the rapidly evolving technological landscape, harmonise diverse global interests, and develop robust and enforceable international frameworks. The future of international governance in technology will likely involve a combination of evolving existing institutions and norms and creating new ones specifically tailored to address the unique challenges posed by these advanced technologies.
In the context of AI governance, several criteria for model access decisions must be established. The intended use of the AI model must align with ethical guidelines and legal frameworks. Access should be granted based on the purpose’s legitimacy, considering factors such as societal benefit, scientific research, or compliance with regulatory standards. This criterion ensures that AI models are used in a manner that promotes positive outcomes and adheres to the overarching principles of responsible AI use. For instance, using an AI model for medical research aimed at improving patient outcomes would be considered a legitimate and beneficial purpose, whereas utilising the same model for unethical surveillance would not meet this criterion. Furthermore, entities seeking access to AI models must adhere to strict data privacy and security standards, particularly when the AI model involves personal or sensitive data. This requirement ensures that data subjects’ rights are protected and that the integrity and confidentiality of the data are maintained. Organisations must demonstrate their ability to implement robust data protection measures, such as data anonymisation, encryption, and secure data handling protocols, to prevent unauthorised access and data breaches.
Entities must also be willing to maintain transparency about how the AI model is used and be accountable for the outcomes. Transparency involves providing clear and accessible information about the model’s functioning, decision-making processes, and the purposes for which it is used. Accountability entails that organisations take responsibility for the model’s impacts, ensuring that any negative consequences are addressed and mitigated. This criterion helps build trust and ensures that AI models are used ethically and responsibly. Additionally, the entity seeking access must possess or have access to the necessary technical expertise to understand and properly use the AI model. This ensures that the model is employed effectively and safely, reducing the risk of misuse or suboptimal performance. Organisations must demonstrate their technical capabilities, including knowledge of AI principles, model operation, and troubleshooting, to ensure they can handle the complexities of the AI system. Lastly, adherence to established ethical guidelines, such as fairness, non-discrimination, and human oversight, should be a prerequisite for access. This ensures that the use of AI models aligns with societal values and ethical norms, promoting fairness and justice. Organisations must commit to principles like equitable treatment of all individuals, avoiding biases in AI outputs, and maintaining human oversight to intervene, when necessary, thereby ensuring the ethical use of AI technologies.
Institutions responsible for making model access decisions play a key role in ensuring these criteria are met. Independent AI auditing bodies, for example, could be specialised institutions established specifically for AI governance, operating independently to assess and make decisions on AI model access. These bodies can provide unbiased evaluations based on set criteria and ensure that access decisions are made transparently and fairly, thereby maintaining objectivity and public trust in AI governance. National or international regulatory bodies with mandates covering technology, data protection, and AI could also oversee access to AI models. These agencies can enforce compliance with legal standards and ethical guidelines, ensuring that AI model use is regulated effectively. Their involvement ensures that access decisions are grounded in legal authority and public policy.
Ethics committees within organisations or independent ethics boards can oversee decisions, ensuring alignment with ethical norms and societal values. These committees can review access requests, evaluate the ethical implications, and make recommendations based on a thorough ethical analysis, thus promoting responsible AI usage. Collaborative groups comprising industry experts, academia, and other stakeholders can be formed to make informed decisions on model access. These industry consortia can leverage diverse perspectives and expertise to assess access requests, ensuring that decisions are well-rounded and consider various aspects of AI deployment. Additionally, organisations like ISO or IEEE can play a role in setting global standards for model access and contributing to decision-making processes. Their involvement ensures that access criteria are consistent with international best practices and standards, facilitating global cooperation and interoperability.
Public-private partnerships between government bodies and private sector entities can bring together regulatory oversight and industry expertise. These collaborations can create a balanced approach to model access, using the strengths to ensure effective and responsible AI governance. Balancing interests and maintaining transparency in model access decisions requires stakeholder engagement. It is crucial to involve various stakeholders, including public representatives, in the decision-making process, ensuring that diverse perspectives are considered. Stakeholder engagement helps in understanding the broader impacts of AI models and ensures that access decisions reflect the interests and concerns of different groups, promoting inclusivity and fairness.
There should also be mechanisms for appealing decisions and for independent oversight of the decision-making process to maintain trust and accountability. An appeals process allows entities to challenge access decisions they perceive as unfair, while oversight ensures that the decision-making process remains transparent, unbiased, and aligned with established criteria and ethical standards.
There are several key avenues through which AI firms can collaborate for the public good, each focusing on different aspects of societal improvement.
One of the most effective ways for AI firms to contribute to public benefit is through open-source initiatives. By sharing code, datasets, and research findings, AI firms democratise access to cutting-edge technologies and accelerate innovation across the field. Platforms like GitHub host numerous collaborative projects where firms contribute to widely used tools such as TensorFlow and PyTorch, facilitating advancements in AI that benefit a broader community. Additionally, AI firms can engage in joint research efforts, often in partnership with academic institutions, to address critical societal challenges. These initiatives might focus on healthcare, climate change, or education, pooling resources and expertise to tackle complex problems that require interdisciplinary approaches. Such collaborations drive technological progress and ensure that AI innovations have a meaningful impact on pressing global issues.
By participating in forums like the Partnership on AI, firms can agree on shared ethical principles that guide AI development and deployment, ensuring technologies are designed with fairness, transparency, and accountability in mind. Furthermore, AI firms can implement self-regulation frameworks within their operations to promote responsible AI development. These frameworks can include measures for privacy protection, bias mitigation, and transparency, helping to ensure that AI technologies are used ethically and do not perpetuate existing inequalities or create new ones.
Creating data pools accessible to researchers and organisations working on societal issues is another way AI firms can contribute to the public good. By sharing anonymised datasets, especially in fields like healthcare, firms can aid in the development of predictive models that improve disease prevention and treatment. Establishing data trusts managed by independent third parties ensures that shared data is used responsibly and for the public benefit. These trusts can help balance the need for data access with privacy concerns, fostering a more ethical and effective use of data in AI research and development.
AI firms can facilitate knowledge exchange through joint training programmes, workshops, and conferences. These initiatives promote continuous learning and skill development in the AI field, ensuring that professionals stay abreast of the latest advancements and ethical considerations. Collaborating with universities to develop talent and support AI curriculum is another avenue. Offering internships, sponsoring research, and providing real-world problems for academic exploration help cultivate the next generation of AI experts and ensure that educational programmes are aligned with industry needs.
AI firms can work together to advocate for policies that encourage innovation while protecting public interests. By engaging with policymakers and regulatory bodies, firms can contribute to the development of informed governance frameworks that balance technological advancement with societal wellbeing. Joint efforts to raise public awareness about AI, its benefits, and ethical use are essential. Transparency campaigns that explain AI technologies and their societal impacts help clarify AI for the general public and build trust in these technologies.
Established AI firms can support startups and SMEs by setting up or contributing to incubators and accelerators. These programmes can provide mentorship, funding, and access to technology resources, helping smaller entities innovate and grow within the AI space. Collaborating on AI solutions for global challenges, such as poverty, hunger, and disaster response, is a powerful way for AI firms to make a positive impact. AI technologies can be used for more effective resource allocation during humanitarian crises, enhancing the ability to respond to and mitigate the effects of disasters.
For AI firms, cooperating for the public benefit involves looking beyond commercial interests to consider the broader societal implications of their technologies. This cooperation requires a commitment to shared goals, transparency, and ethical practices.
AI systems must adhere to data protection regulations such as the GDPR, which stipulates specific guidelines for handling personal data. Non-compliance with these regulations can result in severe legal penalties and a significant loss of public trust. Ensuring compliance is therefore a fundamental aspect of responsible AI development. Obtaining explicit and informed consent from individuals before using their data in AI systems is imperative, particularly in sensitive domains like healthcare. Additionally, the principle of data minimisation should be followed, where only the data necessary for the intended purpose is collected. This reduces the risk of privacy breaches and ensures that personal data is handled responsibly.
Techniques such as data masking, pseudonymisation, and aggregation are employed to minimise identification risks. While these methods are effective in reducing privacy risks, they must be implemented carefully to prevent re-identification. The balance between maintaining data utility and ensuring privacy must be managed meticulously. Privacy considerations should be integrated into AI systems from the design stage, rather than being an afterthought. This holistic approach includes assessing privacy impacts, implementing robust data protection measures, and ensuring ongoing compliance throughout the AI system’s lifecycle. By embedding privacy into the design, AI developers can proactively address potential privacy issues.
While GDPR and other data protection frameworks provide strong guidelines for data privacy, emerging AI-specific security threats necessitate adaptations to existing regulatory mechanisms. AI models are increasingly capable of data reconstruction attacks, where an adversary exploits access to model outputs to infer sensitive information from training data. This poses a challenge to data anonymisation techniques, as modern deep learning models can re-identify individuals from seemingly anonymised data sets.
Additionally, shadow models (unauthorised copies of AI models trained through API-based data extraction) raise concerns about intellectual property theft, bias replication, and lack of accountability in AI decision-making. Traditional privacy laws do not explicitly address these risks, and we need new AI governance policies that integrate model-specific access controls, differential privacy enforcement, and cryptographic AI access verification protocols.
The regulatory landscape must also evolve to incorporate continuous AI privacy assessments, ensuring that AI systems undergo periodic audits to validate compliance with data protection principles. Enforcing such measures would enhance AI accountability while preventing unintended data security breaches arising from model vulnerabilities.
Balancing innovation with privacy is guided by ethical frameworks that ensure AI technologies are used for societal benefits without compromising individual privacy. Transparency in data usage and AI decision-making processes is essential for building trust. Clear communication about how data is used and the reasoning behind AI decisions helps in fostering public confidence in AI systems. In real-world applications, sectors such as finance, healthcare, and e-commerce are increasingly employing AI while navigating complex privacy landscapes.
Understanding bias in AI involves recognising the various sources from which it can stem. Data bias arises from unrepresentative or prejudiced data sets, which can skew the outcomes of AI models. Algorithmic bias occurs when algorithms make decisions based on flawed patterns or rules, often reflecting the biases present in the training data. Societal bias mirrors existing societal prejudices and stereotypes, which can be inadvertently encoded into AI systems. These biases can lead to unfair outcomes, such as discrimination and the unfair treatment of certain groups and can erode public confidence in AI technologies when biases are perceived or realised.
Fairness in AI systems requires an understanding of key concepts. Equality involves treating all individuals the same, while equity involves adjusting treatment to achieve fair outcomes. Contextual fairness acknowledges that definitions of fairness may vary depending on the application domain and cultural context. Metrics and techniques for ensuring fairness include demographic parity, which ensures decisions are independent of sensitive attributes like race or gender, and equal opportunity and equalised odds, which strive for equal predictive performance across different groups. Incorporating individual fairness considerations into decision-making processes also plays a crucial role.
Mitigating bias in AI systems involves several strategies. Diverse data collection ensures that data sets are representative of all relevant groups, reducing the risk of biased outcomes. Algorithmic auditing involves regularly reviewing algorithms to detect and correct biases. Human oversight is also essential, as human judgement can identify and correct biases that algorithms might overlook. These measures collectively help in developing fairer AI systems.
However, achieving fairness in AI presents several challenges. Trade-offs often need to be made between fairness and other objectives, such as accuracy or privacy. Measuring fairness can be difficult, especially in complex or subjective contexts. Additionally, societal norms and definitions of fairness are continually evolving, necessitating ongoing adaptation and vigilance.
Practical applications and case studies illustrate the importance of addressing bias and fairness in AI. In healthcare, ensuring that AI diagnostic tools do not perpetuate biases against certain patient groups is critical for equitable healthcare delivery. In recruitment, avoiding AI tools that might favour certain demographics helps in maintaining fair hiring processes. In the criminal justice system, addressing biases in predictive policing or risk assessment tools is vital for ensuring justice and fairness.
Bias and fairness in AI require a comprehensive approach that includes diverse data, ethical AI design, continuous monitoring, and the incorporation of societal values. As AI systems become more prevalent, ensuring their fairness is crucial for their acceptability and success. Ensuring fairness in AI systems fosters public trust and supports the broader goal of applying AI for societal benefit.
Figure 1 highlighting the sources and types of bias, their impacts, and the measures needed to ensure fairness. It summarises the origins of bias, including data collection, model training, and algorithm design, and categorises bias into sampling, algorithmic, and cultural forms. The figure also describes the detrimental effects of bias, such as unfair treatment, misrepresentation, and decision inaccuracies, which can undermine public trust in AI technologies. To counter these biases, it emphasises the importance of diverse data sets, fairness metrics, and ethical guidelines, along with a feedback loop that supports continuous monitoring and adaptive adjustments, ensuring AI systems are equitable and just.
Figure 1 summarises bias and fairness in AI and ML systems, highlighting the sources, types, impacts of bias, and measures for ensuring fairness.
The figure identifies three primary sources of bias in AI systems. Data bias stems from unrepresentative or prejudiced data sets that can skew AI outputs. Algorithmic bias occurs when algorithms make decisions based on flawed patterns or rules, often reflecting biases present in the training data. Societal bias, on the other hand, reflects existing societal prejudices and stereotypes that can be inadvertently encoded into AI systems. These biases can significantly impact the performance and fairness of AI applications.
Bias in AI can manifest in several forms. Sampling bias arises when the data sample used to train the AI system does not represent the entire population accurately. Algorithmic bias occurs when the algorithms themselves are flawed or are trained on biased data. Cultural bias reflects broader societal prejudices and stereotypes that can be embedded in AI systems, perpetuating existing inequities. Recognising these types of biases is crucial for developing strategies to mitigate their effects.
The implications of bias in AI are profound. Biased AI systems can lead to unfair treatment, misrepresentation, and decision inaccuracies. Unfair outcomes occur when certain groups are discriminated against due to biased AI decisions. Misrepresentation can happen when AI systems incorrectly portray information about individuals or groups. Decision inaccuracy refers to the incorrect or suboptimal decisions made by AI systems due to underlying biases. These impacts can erode public trust in AI technologies, making it essential to address bias comprehensively.
To ensure fairness in AI systems, diverse data sets should be used to train models, representing all relevant groups accurately. Fairness metrics, such as demographic parity, ensure that decisions are independent of sensitive attributes like race or gender. Techniques such as equal opportunity and equalised odds aim to provide equal predictive performance across different groups. Ethical guidelines must be established and followed to ensure AI systems are designed and used responsibly. Incorporating individual fairness considerations into decision-making processes is also crucial for achieving fairness.
A continuous feedback loop is essential for maintaining fairness in AI systems. This involves regular monitoring and adaptive adjustments to the AI models. Continuous monitoring helps detect biases as they emerge, allowing for timely interventions. Adaptive adjustments ensure that AI systems remain fair and effective over time, adapting to new data and societal changes. Human oversight is integral to this process, as human judgement can identify and correct biases that automated systems might overlook.
In healthcare, AI diagnostic tools must be scrutinised to ensure they do not perpetuate biases against certain patient groups. In recruitment, avoiding AI tools that might favour specific demographics is essential for maintaining fair hiring processes. In the criminal justice system, addressing biases in predictive policing or risk assessment tools is critical for ensuring justice and fairness. These practical applications illustrate the importance of addressing bias and fairness in AI.
Achieving fairness in AI is challenging due to several factors. There are inherent trade-offs between fairness and other objectives like accuracy or privacy. Measuring fairness is complex, especially in subjective or multifaceted contexts. Additionally, societal norms and definitions of fairness are continually evolving, requiring AI systems to adapt constantly.
Understanding transparency in AI involves recognising the importance of clarity and openness in communicating an AI system’s capabilities, decision-making processes, and limitations. Transparency is key to building user trust and understanding, which are essential for the widespread acceptance of AI systems. Techniques for achieving transparency include Explainable AI (XAI) methods (Pawar et al., 2020) such as LIME (Local Interpretable Model-agnostic Explanations) and SHAP (SHapley Additive exPlanations), which provide insights into how AI models make decisions. These techniques are particularly valuable in sectors like finance and healthcare, where understanding AI decisions is critical.
However, achieving transparency presents several challenges. Balancing the complexity of AI models with the need for understandable explanations is a significant hurdle. Additionally, navigating the tension between protecting proprietary models and the need for openness poses another challenge. Despite these difficulties, ensuring transparency is crucial for fostering trust and enabling the ethical use of AI.
Accountability in AI systems involves the assignment of responsibility for the outcomes of these systems, including the obligation to report, explain, and amend mistakes. This concept encompasses ethical and legal implications, ensuring AI systems are used responsibly and ethically, with mechanisms in place to address negative outcomes. Regulatory frameworks such as the EU AI Act provide guidelines for accountability in AI, outlining the standards that AI developers and users must adhere to. Compliance and enforcement mechanisms are essential for ensuring adherence to these standards.
Human oversight is a critical component of accountability. Ensuring human involvement in AI decision-making, particularly in critical areas like judicial systems and healthcare, is vital for maintaining ethical standards. Training and awareness programmes for AI practitioners and users are also necessary to educate them on their responsibilities and the ethical use of AI.
Practical applications and case studies highlight the importance of transparency and accountability. Instances where a lack of these principles led to issues, such as biased decision-making in recruitment AI systems, underscore their necessity. Navigating the trade-offs between transparency, privacy, and commercial interests is a delicate balancing act that requires careful consideration. Furthermore, the rapidly evolving nature of AI technologies necessitates continuous updates to regulations and standards.
To address the challenge of assessing transparency and explainability in AI systems, recent EU initiatives provide frameworks for regulatory and technical oversight. The European Centre for Algorithmic Transparency (ECAT), inaugurated in April 2023, aims to provide scientific and technical support for the enforcement of the EU Digital Services Act, particularly concerning algorithmic accountability. Additionally, the Joint Research Centre (JRC) has been instrumental in developing methodologies for ensuring trustworthy AI, offering insights into bias detection, risk assessment, and explainability techniques. The Humaint project further contributes to this discourse by examining the cognitive and social impact of AI, reinforcing the need for rigorous interpretability mechanisms. While these initiatives mark significant progress, they also underscore the persistent difficulties in achieving full transparency, particularly in black-box AI models and deep learning architectures. This highlights the necessity for a combined approach, integrating regulatory oversight with advancements in explainable AI (XAI) techniques to enhance the interpretability of complex AI systems while maintaining security and efficiency.
Beyond the ethical considerations explicitly addressed in this study, additional factors such as environmental sustainability and digital inequality must be incorporated into AI regulatory frameworks. The exponential increase in computational demands associated with generative AI models has significant energy and resource implications, leading to concerns about carbon footprints and water consumption. Some nations are already considering nuclear energy as a potential solution to meet the energy demands of AI infrastructure, highlighting the scale of this challenge. Regulatory frameworks must address data privacy and algorithmic fairness and consider policies that promote energy-efficient AI development. This could include incentives for research into low-energy AI architectures and promoting federated learning techniques that distribute computational loads more efficiently.
One of the most overlooked yet critical ethical dimensions of AI regulation is the environmental impact of large-scale AI systems. Generative AI models, such as large language models (LLMs), require extensive computational resources, leading to significant carbon and hydric footprints. The exponential increase in AI-driven energy demands has prompted discussions about alternative power sources, including nuclear energy, to sustain AI infrastructure. Without clear regulatory guidelines, AI development could exacerbate climate change through unchecked energy consumption.
To address this, regulatory frameworks should incorporate sustainability metrics into AI governance. Policymakers should incentivise the development of energy-efficient AI architectures, promote research into quantum AI for reduced energy expenditure, and mandate carbon transparency for AI firms. Additionally, federated learning and decentralised AI models can reduce data transfer costs and lower overall energy consumption, aligning AI development with sustainability goals.
Regulatory efforts must also consider the supply chain effects of AI computing hardware. The environmental cost of AI extends beyond energy usage, encompassing rare earth metal extraction, electronic waste, and hazardous material disposal. Future AI regulation should integrate sustainability audits for AI hardware production, ensuring that AI-driven advancements do not compromise environmental resilience.
Additionally, the digital divide presents a critical ethical dimension. While AI regulation aims to foster responsible and fair technology use, its effectiveness depends on equitable access to digital resources. Many populations, particularly in the Global South and marginalised communities in developed nations, lack the financial means, education, or digital literacy necessary to benefit from AI-driven innovations. If regulatory policies fail to account for these disparities, they risk exacerbating social and economic inequalities. Ensuring that AI governance incorporates strategies to bridge the digital divide, such as funding initiatives for AI education and prioritising accessibility in AI tool design, is essential for equitable progress.
Bias mitigation efforts should also extend beyond gender and race to include underrepresented cultural minorities, elderly populations, and lower socio-economic groups. For instance, AI-driven healthcare systems must account for the disparities in life expectancy and access to medical services among different socio-economic groups. Algorithmic fairness should encompass broader considerations of social inequality to prevent the reinforcement of systemic disadvantages.
One of the primary aspects of this framework is ensuring privacy and data protection. AI systems must adhere to existing data protection laws, such as the GDPR, which advocate for data minimisation and explicit consent. Techniques like data masking, pseudonymisation, and aggregation are crucial for protecting individual privacy while maintaining data utility. Integrating advanced privacy-preserving methods, such as differential privacy and federated learning, can further enhance the efficacy of these measures.
Many regulatory frameworks were conceived before the widespread adoption of generative models, which raise unique issues such as intellectual property rights over training datasets, misinformation risks, and the monopolisation of computational resources. While privacy rights are well-established in AI governance discussions, the legal status of datasets used for training large models remains ambiguous. The regulation of proprietary AI models should consider fair data usage principles, ensuring that training data adheres to ethical collection practices and respects copyright laws.
Another crucial aspect is the increasing divergence between large-scale AI models and alternative approaches. While most discourse focuses on US-centric large language models, alternative strategies, such as small language models (SLMs) and decentralised AI frameworks, are gaining traction. These models offer advantages in terms of computational efficiency and localised adaptation but require distinct regulatory considerations, particularly in data governance and security. AI regulation should govern the dominant models developed by major corporations but also consider the implications of smaller-scale and decentralised AI solutions.
The intensifying investments in generative AI also present a sovereignty issue. With AI R&D concentrated among a handful of dominant firms and nations, disparities in AI access and capabilities are widening. This dynamic has strategic implications, as AI regulation cannot be decoupled from discussions on technological sovereignty and economic power imbalances. Policies promoting open AI ecosystems, international research collaborations, and equitable AI access can help mitigate this concentration of power.
While privacy rights have been a central focus of AI regulation, a major gap exists in the legal treatment of training datasets and model-generated content. Current legal frameworks struggle to define the ownership rights of datasets used in AI model training, particularly when copyrighted materials are scraped from the internet without explicit consent. The issue extends to AI-generated content, where determining authorship and intellectual property rights remains legally ambiguous.
The debate surrounding the fair use of training data has intensified with legal cases against major AI firms accused of using copyrighted datasets without permission. AI regulation should establish clearer IPR guidelines for generative AI, ensuring fair compensation for content creators while maintaining access to public domain resources for AI training.
AI governance should address the monopolisation risks posed by dominant AI firms that control access to computational power and proprietary datasets. Open-source AI initiatives should be incentivised to ensure that AI development remains decentralised and accessible to a broader research community.
Ethical considerations and bias mitigation are equally critical in the responsible deployment of AI technologies. Diverse data collection is essential to create representative datasets, reducing the risk of bias. Regular algorithmic audits can help identify and rectify biases. Implementing fairness-aware machine learning techniques and bias mitigation algorithms ensures that AI systems treat all individuals equitably. Human oversight is vital, particularly in sensitive applications such as healthcare and judicial systems, to correct biases that automated systems might overlook. Training programmes for AI practitioners on ethical AI usage and bias mitigation are necessary to support this oversight.
Transparency and accountability are fundamental principles that underpin public trust in AI systems. XAI techniques, such as LIME and SHAP, enhance the interpretability of AI models, making their decision-making processes more understandable. More sophisticated methods like causal inference and counterfactual explanations provide deeper insights into AI decisions, complementing transparency strategies. Establishing clear accountability frameworks, including compliance with the EU AI Act and other relevant regulations, ensures that AI systems are used responsibly. Mechanisms for reporting, explaining, and amending mistakes must be in place and functional.
International and domestic regulatory frameworks are crucial for harmonising AI governance. Global cooperation is essential for establishing standards that address issues such as AI arms races, autonomous weapons, and cross-border data governance. Proposals for creating international organisations, akin to a ‘World AI Organisation’, highlight the need for a unified global approach. Domestically, regulations must be tailored to specific sectors, such as healthcare and finance, to address unique ethical and security concerns. Oversight committees and public awareness campaigns play vital roles in promoting informed decision-making and fostering public confidence in AI.
Technical approaches to governance, such as homomorphic encryption and zero-knowledge proofs, are vital for ensuring privacy and security in AI systems. These cryptographic techniques allow for secure data processing, preserving privacy while enabling comprehensive data analysis. The global absence of a unified identity management system means that encryption-based anonymity protections can be exploited for illicit activities, including cybercrime and darknet operations. AI regulation must balance privacy protections with security considerations by embedding safeguards that prevent abuse while maintaining individual rights. This requires international agreements on AI-enabled security threats, cybersecurity frameworks that account for AI’s evolving capabilities, and collaborative monitoring initiatives to detect and mitigate risks. Blockchain technology for example, provides transparent and immutable ledgers, useful for tracking compliance in various domains. Secure multi-party computation can facilitate collaborative data analysis while maintaining data privacy. This highlights the need for these advanced technical solutions, and in Table 3, we can see the emerging framework.
Despite the simplicity of the approach presented in Table 3, significant progress, challenges in AI governance persist. Harmonising diverse international interests and keeping pace with rapid technological advancements are ongoing issues. Measuring fairness and balancing it with other objectives, such as accuracy and privacy, remains complex. Continuous assessment, adaptation, and collaboration among stakeholders are essential to address these challenges effectively. Engaging various stakeholders, including public representatives, in the decision-making process ensures diverse perspectives are considered, promoting inclusivity and fairness.
A crucial oversight in the proposed framework in Table 3, are the existing discussion on AI regulation is the role of standardisation efforts. The EU AI Act, for example, relies heavily on standardisation processes facilitated by public bodies such as CEN-CENELEC (2025). Similarly, global AI governance efforts must acknowledge the increasing involvement of the United Nations High-level Advisory Body on Artificial Intelligence, which is actively shaping AI policy discussions at an international level. By ignoring these official standardisation bodies, governance discussions risk being detached from the institutional realities shaping regulatory enforcement.
To enhance regulatory effectiveness, AI governance frameworks should incorporate multi-stakeholder perspectives, including the roles of industry leaders, academic researchers, and civil society organisations. While the current discourse on AI governance tends to focus on regulatory bodies and state actors, industry-led initiatives play a critical role in shaping de facto standards. For instance, voluntary AI ethics frameworks developed by leading technology firms influence global AI governance in ways that are sometimes more immediate than formal legislative processes. Incorporating these perspectives ensures that AI regulation remains practical and adaptable to real-world deployment challenges.
Similarly, the inclusion of NGOs and advocacy groups in AI governance discussions is essential to maintain a balance between commercial interests and societal impact. Many AI-related risks, such as algorithmic bias and digital inequality, are best addressed through collaborative governance models that leverage expertise from diverse sectors.
The framework in, and all other AI regulatory frameworks, must incorporate structured AI incident response protocols to address security breaches, adversarial attacks, and model failures. Unlike traditional cybersecurity incidents, AI security breaches can result in cascading failures where adversarial manipulations propagate through interconnected AI models.
A comprehensive AI incident response strategy should include:
AI-specific threat intelligence sharing between regulatory bodies and industry stakeholders.
Automated detection of adversarial AI attacks using anomaly detection and adversarial retraining.
Rapid response measures to mitigate AI-induced misinformation, fraud, or operational failures.
Regulatory enforcement of AI security auditing to pre-emptively identify vulnerabilities.
These provisions would enhance the resilience of AI-driven ecosystems and ensure that regulatory efforts remain proactive in mitigating AI security threats. Building upon the proactive learning concept, the framework in also emphasises the importance of encouraging ethical innovation and research. Supporting initiatives that prioritise ethical considerations in AI development fosters a culture of responsibility. Collaboration with academic institutions can advance the understanding of AI ethics and governance, facilitating research projects that address real-world applications and implications. Policy advocacy plays a crucial role in developing comprehensive AI regulations that balance innovation with ethical considerations.
The findings of this study align closely with recent advancements and discussions in the field of AI regulation, particularly in areas such as privacy, ethics, transparency, and accountability.
Recent studies highlight the critical importance of privacy and data protection in AI systems, echoing our emphasis on compliance with regulations like the GDPR. The principle of data minimisation and the necessity for explicit consent are reiterated across contemporary literature, underscoring their fundamental role in mitigating privacy risks. However, recent research also explores more advanced techniques such as differential privacy, which offers robust methods for preserving individual privacy while allowing for useful data analysis. Integrating these advanced privacy-preserving techniques into regulatory frameworks could enhance the efficacy of privacy measures discussed in this study.
Ethical considerations, particularly concerning bias and fairness in AI systems, highlighted by this research, is also a significant focus in current literature. Recent studies suggest that in addition to diverse data collection and algorithmic auditing, incorporating fairness constraints during the model training process can mitigate biases more effectively. Techniques such as fairness-aware machine learning and bias mitigation algorithms are increasingly recognised as essential tools for developing equitable AI systems.
Transparency and accountability in AI systems remain critical for fostering public trust, as affirmed by our findings and recent standards like the EU AI Act. The use of XAI techniques such as LIME and SHAP, discussed in this paper, is widely endorsed in contemporary research for enhancing the interpretability of AI models. However, recent advancements propose more sophisticated methods like causal inference and counterfactual explanations, which provide deeper insights into AI decision-making processes. These methods could complement the transparency strategies outlined in this study, offering more robust solutions for understanding and overseeing AI systems.
The concept of establishing international organisations to oversee AI regulation, akin to the Paris Agreement for climate change, has gained traction in recent policy discussions. The proposed ‘World AI Organisation’ aligns with suggestions from recent studies advocating for a unified global approach to AI governance. Domestically, sector-specific regulations, such as those in healthcare and finance, continue to evolve, with recent guidelines emphasising the importance of dynamic and adaptable regulatory measures.
Technical approaches to governance, such as homomorphic encryption and zero-knowledge proofs, are increasingly recognised as vital tools for ensuring privacy and security in AI systems. Recent research supports the use of these cryptographic techniques for secure data processing, reinforcing their importance as discussed in this paper. However, advancements in quantum-safe encryption and secure multi-party computation offer additional layers of security and efficiency, suggesting further areas for integration into the technical governance frameworks proposed by this study.
Despite significant progress, challenges in AI governance persist. Harmonising diverse international interests and keeping pace with rapid technological advancements are ongoing issues, as noted in this study and recent literature. The complexity of measuring fairness and balancing it with other objectives, such as accuracy and privacy, remains a critical challenge.
This study examined the dimensions of regulating AI and ML systems, with particular attention to LLMs, such as ChatGPT. By addressing core pillars of privacy, ethics, fairness, transparency, accountability, and international regulatory frameworks, this research highlights the challenges and opportunities that define AI governance.
A primary conclusion of this work underscores the centrality of privacy and data protection within AI systems. Adherence to frameworks such as the GDPR remains a legal obligation and ethical requirement. Advanced privacy-preserving methodologies, including homomorphic encryption, federated learning, and differential privacy, offer a method to reconcile the tension between data utility and robust privacy protection. These techniques are foundational to the construction of AI systems that are secure and socially responsible.
Ethical considerations, particularly with respect to bias and fairness, emerge as non-negotiable in the deployment of AI technologies. This study has identified the pervasive and systemic nature of algorithmic bias, advocating for a multi-pronged approach that includes the adoption of fairness-aware machine learning techniques, regular algorithmic audits, and the cultivation of diverse and representative datasets. Moreover, understanding of fairness, encompassing concepts of equity, contextual fairness, and proportionality, is essential to ensure that AI applications meet ethical standards across diverse societal and cultural contexts.
Transparency and accountability represent foundational principles for fostering public trust in AI technologies. The study endorses the integration of Explainable AI (XAI) techniques, such as LIME and SHAP, alongside emerging methods including causal inference and counterfactual explanations, to enhance model interpretability. Equally, the establishment of accountability mechanisms, supported by legislative frameworks such as the EU AI Act, ensures that ethical responsibilities are embedded into the lifecycle of AI development and deployment.
On the international stage, this research has underscored the necessity of harmonised global regulatory frameworks to address transnational challenges, such as the proliferation of autonomous weapons and the complexities of cross-border data governance. The proposal for a ‘World AI Organisation’ seeks to provide a unified body to promote international cooperation, encourage best practices, and facilitate the equitable governance of AI technologies. Domestically, sector-specific regulations, tailored to the unique demands of fields such as healthcare and finance, are essential for mitigating risks and safeguarding trust in AI systems.
From a technical perspective, this study highlights the promise of cryptographic approaches, such as zero-knowledge proofs, blockchain technology, and secure multi-party computation, as essential tools for enabling privacy-preserving operations and ensuring compliance with regulatory standards. These techniques represent critical innovations for the responsible governance of AI, particularly in the context of increasingly complex and data-intensive applications.
Nevertheless, significant challenges persist. Foremost among these are the difficulties in aligning divergent international interests and the need to ensure that regulatory frameworks evolve in step with the rapid pace of technological development. The measurement of fairness, particularly when balancing competing objectives such as accuracy, privacy, and equity, remains an area of acute complexity.
This study offers a blueprint for AI governance that seeks to balance innovation with the ethical demands of accountability, transparency, and societal trust.
To strengthen the applicability of AI governance recommendations, future studies need to incorporate concrete case studies of successful regulatory frameworks and implementation strategies. Examples such as the AI auditing processes established by the UK’s Information Commissioner’s Office (ICO) and the real-world applications of algorithmic impact assessments in Canadian AI governance provide valuable insights into how AI regulation functions in practice.
Additionally, while the paper acknowledges that regulatory implementation is complex, more specific strategies for overcoming these challenges should be investigated. This includes proposing phased implementation approaches, sector-specific regulatory adaptations, and investment strategies to ensure that AI governance efforts are adequately resourced.
By incorporating these additional dimensions, future studies can bridge the gap between idealistic regulatory frameworks and the real-world constraints of AI governance, ensuring that recommendations remain ethically sound and strategically viable.
PR: Conceptualization, Data curation, Formal analysis, Investigation, Methodology, Software, Supervision, Validation, Visualization, Writing – original draft, Writing – review & editing.
The author(s) declare that financial support was received for the research and/or publication of this article. This work was supported by UK EPSRC [grant number EP/S035362/1].
The author declares that the research was conducted in the absence of any commercial or financial relationships that could be construed as a potential conflict of interest.
The author declares that Generative AI was used in the creation of this manuscript. Grammarly was used to proof check for spelling and grammar.
All claims expressed in this article are solely those of the authors and do not necessarily represent those of their affiliated organizations, or those of the publisher, the editors and the reviewers. Any product that may be evaluated in this article, or claim that may be made by its manufacturer, is not guaranteed or endorsed by the publisher.
Alyami, H., Nadeem, M., Alharbi, A., Alosaimi, W., Ansari, M. T. J., Pandey, D., et al. (2021). The evaluation of software security through quantum computing techniques: a durability perspective. Appl. Sci. 11:11784. doi: 10.3390/app112411784
Androulaki, E., Barger, A., Bortnikov, V., Muralidharan, S., Murthy, C., Nguyen, B., et al. Hyperledger fabric: a distributed operating system for permissioned blockchains. Proceedings of the thirteenth EuroSys conference. (2018).
Awan, U., Hannola, L., Tandon, A., Goyal, R. K., and Dhir, A. (2022). Quantum computing challenges in the software industry. A fuzzy AHP-based approach. Inf. Softw. Technol. 147:106896. doi: 10.1016/j.infsof.2022.106896
CCPA. State of California - department of justice - Office of the Attorney General [Internet]. (2018). Available online at: https://oag.ca.gov/privacy/ccpa (Accessed September 20, 2023).
CEN-CENELEC. The European Committee for Standardization [internet]. (2025). Available online at: https://www.cencenelec.eu/about-cen/ (Accessed February 15, 2025).
CISA. Shifting the balance of cybersecurity risk: Principles and approaches for security-by-design and -default [internet]. (2023). Available online at: http://www.cisa.gov/tlp/ (Accessed August 8, 2023).
Dong, Z., Luo, F., and Liang, G. (2018). Blockchain: a secure, decentralized, trusted cyber infrastructure solution for future energy systems. J. Mod. Power Syst. Clean Energy 6, 958–967. doi: 10.1007/s40565-018-0418-0
European Court of Justice. EU-U.S. privacy shield [internet]. (2020) Available online at: https://commission.europa.eu/law/law-topic/data-protection/international-dimension-data-protection/eu-us-data-transfers_en (Accessed February 15, 2025).
European Parliament. AI act: A step closer to the first rules on artificial intelligence. (2023). Available online at: https://www.europarl.europa.eu/news/en/press-room/20230505IPR84904/ai-act-a-step-closer-to-the-first-rules-on-artificial-intelligence (Accessed July 7, 2023).
Fredrikson, M., Jha, S., and Ristenpart, T. (2015). Model inversion attacks that exploit confidence information and basic countermeasures. Proceedings of the ACM Conference on Computer and Communications Security.
GDPR. What is GDPR, the EU’s new data protection law? (2018). Available online at: https://gdpr.eu/what-is-gdpr/ (Accessed July 7, 2023).
Gentry, C. Fully homomorphic encryption using ideal lattices. Proceedings of the Annual ACM Symposium on Theory of Computing [Internet]. (2009).
Gentry, C., Halevi, S., and Smart, N. P. (2012). Fully homomorphic encryption with Polylog overhead. Lecture notes in computer Science (including subseries lecture notes in artificial intelligence and lecture notes in bioinformatics) [internet]. LNCS 7237, 465–482. doi: 10.1007/978-3-642-29011-4_28
Global Cybersecurity Capacity Centre. Home page | global cyber security capacity Centre. Available online at: https://gcscc.ox.ac.uk/home-page (Accessed February 15, 2025).
Goodfellow, I., Pouget-Abadie, J., Mirza, M., Xu, B., Warde-Farley, D., Ozair, S., et al. (2014). Generative adversarial networks. Commun ACM 63, 139–144. doi: 10.1145/3422622
GOV.UK. Data protection act 2018 (2018). Available online at: https://www.legislation.gov.uk/ukpga/2018/12/contents (Accessed February 15, 2025).
Gupta, S., Modgil, S., Bhatt, P. C., Chiappetta Jabbour, C. J., and Kamble, S. (2023). Quantum computing led innovation for achieving a more sustainable Covid-19 healthcare industry. Technovation 120:102544. doi: 10.1016/j.technovation.2022.102544
Hazra, A., Alkhayyat, A., and Adhikari, M. (2022). “Blockchain for cybersecurity in edge networks,”in IEEE Consumer Electronics Magazine. Vol. 13. pp. 97–102 doi: 10.1109/MCE.2022.3141068
He, S., Ficke, E., Pritom, M. M. A., Chen, H., Tang, Q., Chen, Q., et al. (2022). Blockchain-based automated and robust cyber security management. J Parallel Distrib Comput 163, 62–82. doi: 10.1016/j.jpdc.2022.01.002
He, Z., Zhang, T., and Lee, R. B. Model inversion attacks against collaborative inference. ACM International Conference Proceeding Series. (2019).
HIPAA. Health insurance portability and accountability act of 1996. (1996). Available online at: https://www.cdc.gov/phlp/publications/topic/hipaa.html (Accessed July 7, 2023).
ICO. UK GDPR guidance and resources. (2018). Information Commissioner’s Office (ICO): The UK GDPR. Available online at: https://ico.org.uk/for-organisations/data-protection-and-the-eu/data-protection-and-the-eu-in-detail/the-uk-gdpr/ (Accessed July 8, 2023).
Institute of Electrical and Electronics Engineers. IEEE introduces new program for free access to AI ethics and governance standards. (2023). Available online at: https://standards.ieee.org/news/get-program-ai-ethics/#:~:text=PISCATAWAY%2C%20NJ%2C%2017%20January%202023,in%20AI%20Ethics%20and%20Governance (Accessed March 14, 2025).
ISO. ISO - International Organization for Standardization [internet]. (2017). Available online at: https://www.iso.org/home.html (Accessed December 26, 2017).
Liu, J., Kretz, I., Liu, H., Tan, B., Wang, J., Sun, Y., et al. Certifying zero-knowledge circuits with refinement types. (2024). Available online at: https://ieeexplore.ieee.org/document/10646715/ (Accessed September 19, 2024).
Lung, R. I., (2023). A game theoretic decision-making approach for fast gradient sign attacks. Elsevier [internet]. Available online at: https://www.sciencedirect.com/science/article/pii/S1877050923006762 (Accessed November 15, 2024).
Mallow, G. M., Hornung, A., Barajas, J. N., Rudisill, S. S., An, H. S., and Samartzis, D. (2022). Quantum computing: the future of big data and artificial intelligence in spine. Spine Surg. Relat. Res. 6:93. doi: 10.22603/ssrr.2021-0251
Marais, A., Adams, B., Ringsmuth, A. K., Ferretti, M., Gruber, J. M., Hendrikx, R., et al. Artificial intelligence computing at the quantum level. Data. (2022); 7::28. Available online at: https://www.mdpi.com/2306-5729/7/3/28/htm (Accessed October 6, 2023).
Naqvi, S., Shabaz, M., and Khan, M. (2024, 2023). Adversarial attacks on visual objects using the fast gradient sign method. J Grid Comput 21:684. doi: 10.1007/s10723-023-09684-9
National Institute of Standards and Technology (2023). AI Risk Management Framework. Available online at: https://www.nist.gov/itl/ai-risk-management-framework (Accessed April 18, 2023).
Nita, S. L., and Mihailescu, M. I. (2023). Homomorphic encryption. Adv. Homomor. Search. Encryp., 27–88. doi: 10.1007/978-3-031-43214-9_3
Ozdag, M. (2018). Adversarial attacks and defenses against deep neural networks: a survey. Procedia Comput. Sci. 140, 152–161. doi: 10.1016/j.procs.2018.10.315
Pawar, U., O’Shea, D., Rea, S., and O’Reilly, R. Explainable AI in healthcare. (2020) International conference on cyber situational awareness, Data Analytics and Assessment, Cyber SA.
Sevilla, J., and Moreno, P. (2019) Implications of quantum computing for artificial intelligence alignment research. Available at: https://arxiv.org/abs/1908.07613 (Accessed March 14, 2025).
Shaaban, A. M., Kristen, E., and Schmittner, C. (2018). “Application of IEC 62443 for IoT components” in Lecture notes in computer Science (including subseries lecture notes in artificial intelligence and lecture notes in bioinformatics) ed. G. Goos. (Springer Verlag), 214–223.
The International Telecommunication Union. X.1500: Overview of cybersecurity information exchange [internet]. (2018). Available online at: https://www.itu.int/rec/T-REC-X.1500 (Accessed July 25, 2023).
The White House. Fact sheet: Biden-Harris administration announces new actions to promote responsible AI innovation that protects Americans’ rights and safety. (2023). Available online at: https://bidenwhitehouse.archives.gov/briefing-room/statements-releases/2023/05/04/fact-sheet-biden-harris-administration-announces-new-actions-to-promote-responsible-ai-innovation-that-protects-americans-rights-and-safety/ (Accessed March 14, 2025).
Whyte, C. (2018). Crossing the digital divide: monism, dualism and the reason collective action is critical for cyber theory production. Politics Govern. 6, 73–82. doi: 10.17645/pag.v6i2.1338
Wylde, V., Rawindaran, N., Lawrence, J., Balasubramanian, R., Edmond, P., Jayal, A., et al. (2022). Cybersecurity, data privacy and Blockchain: a review. SN Comput. Sci. 3, 127–112. doi: 10.1007/s42979-022-01020-4
Yang, X., and Li, W. (2020). A zero-knowledge-proof-based digital identity management scheme in blockchain. Comput. Secur. 99:102050. doi: 10.1016/j.cose.2020.102050
Keywords: frontier AI regulation, AI security threats, adversarial risk, cryptographic governance, compliance enforcement, legal accountability, regulatory harmonisation, algorithmic oversight
Citation: Radanliev P (2025) Frontier AI regulation: what form should it take? Front. Polit. Sci. 7:1561776. doi: 10.3389/fpos.2025.1561776
Received: 16 January 2025; Accepted: 05 March 2025;
Published: 20 March 2025.
Edited by:
Ana Campina, Fernando Pessoa University, PortugalReviewed by:
Pierre Rossel, Inspiring Futures Sàrl, SwitzerlandCopyright © 2025 Radanliev. This is an open-access article distributed under the terms of the Creative Commons Attribution License (CC BY). The use, distribution or reproduction in other forums is permitted, provided the original author(s) and the copyright owner(s) are credited and that the original publication in this journal is cited, in accordance with accepted academic practice. No use, distribution or reproduction is permitted which does not comply with these terms.
*Correspondence: Petar Radanliev, cGV0YXIucmFkYW5saWV2QGNzLm94LmFjLnVr
Disclaimer: All claims expressed in this article are solely those of the authors and do not necessarily represent those of their affiliated organizations, or those of the publisher, the editors and the reviewers. Any product that may be evaluated in this article or claim that may be made by its manufacturer is not guaranteed or endorsed by the publisher.
Research integrity at Frontiers
Learn more about the work of our research integrity team to safeguard the quality of each article we publish.