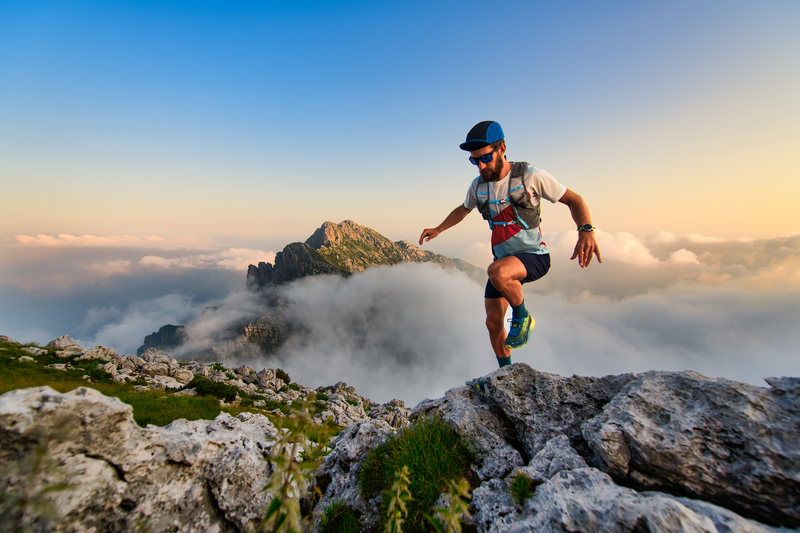
95% of researchers rate our articles as excellent or good
Learn more about the work of our research integrity team to safeguard the quality of each article we publish.
Find out more
ORIGINAL RESEARCH article
Front. Plant Sci. , 28 January 2025
Sec. Plant Abiotic Stress
Volume 16 - 2025 | https://doi.org/10.3389/fpls.2025.1527447
Introduction: Earlier sowing is a promising strategy of ensuring sufficiently high maize yields in the face of negative environmental factors caused by climate change. However, it leads to the low temperature exposure of maize plants during emergence, warranting a better understanding of their response and acclimation to suboptimal temperatures.
Materials and Methods: To achieve this goal, whole transcriptome sequencing was performed on two maize inbred lines – tolerant/susceptible to low temperatures, at the 5-day-old seedling stage. Sampling was performed after 6h and 24h of treatment (10/8°C). The data was filtered, mapped, and the identified mRNAs, lncRNAs, and circRNAs were quantified. Expression patterns of the RNAs, as well as the interactions between them, were analyzed to reveal the ones important for low-temperature response.
Results and Discussion: Genes involved in different steps of photosynthesis were downregulated in both genotypes: psa, psb, lhc, and cab genes important for photosystem I and II functioning, as well as rca, prk, rbcx1 genes necessary for the Calvin cycle. The difference in low-temperature tolerance between genotypes appeared to arise from their ability to mitigate damage caused by photoinhibition: ctpa2, grx, elip, UF3GT genes showed higher expression in the tolerant genotype. Certain identified lncRNAs also targeted these genes, creating an interaction network induced by the treatment (XLOC_016169-rca; XLOC_002167-XLOC_006091-elip2). These findings shed light on the potential mechanisms of low-temperature acclimation during emergence and lay the groundwork for subsequent analyses across diverse maize genotypes and developmental stages. As such, it offers valuable guidance for future research directions in the molecular breeding of low-temperature tolerant maize.
Negative environmental factors caused by climate change, such as extreme temperatures, and prolonged drought periods, continue to jeopardize global food security by impacting crop productivity (Pörtner et al., 2022; Benitez-Alfonso et al., 2023). Average temperatures on Earth are rapidly rising: an increase of 2.5-4.5°C is expected by the year 2100 (Wang et al., 2018), and this negatively impacts the plants’ carbon uptake, resulting in decreased crop yield (Wang et al., 2018). Maize is particularly affected since it is a C4 plant. Additionally, maize grown in temperate regions reaches the developmental stages most susceptible to heat and drought – flowering and grain filling (Deryng et al., 2014), during a time of year when these extreme weather events are most likely to occur. On average, a yield loss of 7.4% for every 1°C rise in the temperature is predicted for maize (Zhao et al., 2017). Some studies predicted even higher values: up to 23% in the United States (Xu et al., 2016) or 30% in Mexico (Murray-Tortarolo et al., 2018). Since maize is one of the most important crop species worldwide, increasing the crops’ adaptation to climate change, while ensuring sufficient maize yields to guarantee future food security, becomes extremely urgent (Farooq et al., 2023).
Crop adaptation approaches encompass increasing crops’ resilience to climate change factors (Benitez-Alfonso et al., 2023), but also strategies of avoidance, that include earlier sowing, adoption of later-maturing spring varieties, or adoption of winter cultivars in cooler environments (Liu et al., 2017; Collins and Chenu, 2021; Zhu et al., 2022). Some studies have shown that earlier sowing could positively impact maize yield, if implemented with necessary changes in some traits and management practices (Bassu et al., 2021; Zhu et al., 2022). In temperate areas, early sowing could ensure that flowering and grain-filling stages are completed before the periods of extreme heat and drought. However, as maize development is affected by temperatures lower than 15°C (Silva-Neta et al., 2015), earlier sowing results in the exposure of maize seedlings to suboptimal temperatures in the early development, during the emergence stage (VE). The VE stage is considered to be extremely thermo-sensitive, and low temperature (LT) stress at that stage does affect maize yield (Jiang et al., 2021; Beegum et al., 2023).
The effects of low temperatures on maize have been extensively studied over the years. It is known that temperatures below 10°C cause cellular and tissue injuries, protein denaturation, photosynthesis restriction, and oxidative damage (Frascaroli and Revilla, 2018; Zhou et al., 2022a). LT reduces the germination rate and seedling vigor, and leads to the inhibition of growth and development (Yang et al., 2011; Zhang et al., 2020). However, most studies focus on maize seedlings at later developmental stages, starting from the first leaf development (V1) (Waititu et al., 2021; Zhou et al., 2023; Gao et al., 2024). Data on the LT response in the VE stage is less frequent, but recently, more studies on this stage have been published (Li et al., 2021; Xuhui et al., 2022). Implementing early sowing in maize production requires further research of the response to low temperatures during the VE stage, as well as the uncovering of the possible methods of acclimating to this stress factor.
Evaluating gene expression patterns based on transcriptome sequencing (RNA-seq) data has been an effective way of analyzing the molecular mechanisms behind the LT response in plants and elucidating how they combine to establish tolerance (Dasgupta et al., 2020). RNA-seq methods have shown that genes involved in abiotic stress responses, including transcription factors, signal transduction molecules, and antioxidative enzymes, are induced under LT conditions (Mao et al., 2017; Sowiński et al., 2020). Additionally, non-coding RNAs (ncRNAs), such as long non-coding (lncRNAs) and circular RNAs (circRNAs), are involved in gene regulation in plants (Yu et al., 2019). lncRNAs comprise linear, ncRNAs, longer than 200 nucleotides (nt) (Waseem et al., 2021). These ncRNAs fulfill their roles in regulating gene expression through epigenetic modification, splicing regulation, or interaction with other RNA classes (Wang et al., 2023; Gonzales et al., 2024). The primary way lncRNAs regulate gene expression is through their interaction with microRNAs (miRNAs), as described by the ceRNA hypothesis (Salmena et al., 2011; He et al., 2020). miRNAs are known gene expression inhibitors, that act through RNA silencing of target mRNAs (Zhang et al., 2022). The ceRNA hypothesis states that lncRNAs can influence RNA silencing, as they have the same miRNA binding sites as the target mRNAs. By competing for the miRNAs with the target mRNAs, the lncRNAs can reduce the level of target mRNA inhibition and exert their influence on transcription. Circular RNAs are endogenous, single-stranded, covalently-closed RNA molecules, generated by back-splicing events of precursor mRNAs during post-transcriptional processes (Han et al., 2020). Similar to lncRNAs, circRNAs can act as miRNA sponges (Hansen et al., 2013), interact with proteins and transcription factors (Li et al., 2015b), alter genome structure (Dong et al., 2016), and guide protein translation (Yang et al., 2017). Both lncRNAs and circRNAs have been found to take part in the LT response and acclimation of plants (Biswas et al., 2021; Wang et al., 2024). For example, a novel cold-induced lncRNA, CIL1 was found to regulate the expression of multiple stress-related genes, and affect the plant cold tolerance in Arabidopsis (Liu et al., 2022a). Also, overexpression of the Vitis vinifera circATS1, improved cold tolerance in Arabidopsis (Gao et al., 2019). Screening for LT regulated genes and ncRNAs in the VE stage may help identify hub genes, ncRNAs, and the interaction networks that can be potential targets for breeding tolerant varieties, capable of survival and growth under LT conditions.
The aim of the research study was to examine the immediate (6h) and delayed (24h) responses to low temperatures in the 5-day-old maize seedlings (VE stage) of both the tolerant and sensitive maize genotype. This was carried out by applying RNA-seq technologies and analyzing the expression of genes and ncRNAs (lncRNAs, circRNAs, and miRNAs) involved in this response. The RNA expression profiles under optimal and LT conditions were compared and the interactions between the different RNA classes were analyzed, with the goal of revealing expression patterns and interaction networks important for establishing low-temperature tolerance in the selected developmental stage.
Two maize inbred lines of contrasting tolerance to LT stress, marked as LT, the tolerant, and LS, the susceptible inbred line, were exposed to low temperatures at the five-day-old (5d-old) seedling stage. The two maize lines were parental components of commercial ZP hybrids, developed in the Maize Research Institute Zemun Polje (MRIZP), and the seeds were obtained from the MRIZP institute. LS belonged to the Lancaster heterotic group, while LT was a semi-dent. The tolerance of the two lines was assessed in a previous experiment (Božić et al., 2024), and the selection was made based on seed vigor, survival rate, radicle and coleoptile length, as well as seedling fresh weight.
Seeds of both inbred lines were sterilized in 10% sodium hypochlorite (commercial bleach) and germinated in the dark, for five days, under optimal conditions (25/20°C – 12/12h period, 75% relative humidity) in a climate chamber (MLR-352H-PE, PHC Europe B.V.). Subsequently, the 5-day-old seedlings were subjected to the LT treatment (T) at 10/8°C for 6 and 24 hours, 12/12h light/dark photoperiod, and 700 µmolm-2s-1 light intensity. Samples of 30 maize seedlings per inbred line were taken after 6h and 24h for total RNA isolation. The sampled tissue was ground in liquid nitrogen and stored at -80°C until further use. Control plants (C) were grown under optimal conditions (25/20°C – 12/12h photoperiod, 700 µmolm-2s-1 light intensity, 75% relative humidity) in the same period and sampled at identical time points. There were eight samples in total: two samples per treatment condition (C, T), per time point (6h, 24h), and per inbred line (LS, LT).
Total RNA was extracted from ≈100 mg of frozen tissue per sample using the GeneJet™ RNA Purification kit (Thermo Scientific, USA) and purified by applying Ambion® DNA-free™ DNase I (Invitrogen, USA), according to the manufacturer’s instructions. Total RNA concentrations were first determined using the NanoPhotometer® spectrophotometer (IMPLEN, USA), while the RNA quality and contamination were screened through agarose gel electrophoresis. After the preliminary check, the 2100 Bioanalyzer and RNA Nano 6000 Assay Kit (Agilent®, CA, USA) were used to further determine the RNA integrity and quantity. All samples with RIN above six were chosen for the downstream analysis.
Library preparation and sequencing of the eight samples were carried out on the NovaSeq 6000 (Illumina®, USA) at the Novogene Bioinformatics Technology Co. Ltd., in Beijing, China. Firstly, ribosomal RNA, rRNA, was removed from the total RNA (1000 ng) using magnetic beads from the Ribo-Zero Plus rRNA Depletion Kit (Illumina®, USA), according to the manufacturer’s instructions. One library per sample was then generated by NEBNext® Ultra™ Directional RNA Library Prep Kit for Illumina® (NEB®, USA), following the manufacturer’s recommendations. The library quality was assessed on the Agilent Bioanalyzer 2100. After, the paired-end (PE) 2x150bp sequencing was performed on all eight libraries (LT-C-6, LT-C-24, LT-T-6, LT-T-24, LS-C-6, LS-C-24, LS-T-6, and LS-T-24).
The raw reads were first checked using FastQC (v0.12.1, Andrews, 2010), and then processed with Trimmomatic (v0.39-2, Bolger et al., 2014). Reads containing adapter and poly-N sequences and low quality reads (Phred score > 30, N% < 10%) were removed. Clean reads were mapped onto the Zea mays B73 NAM reference genome version 5.0 (https://plants.ensembl.org/Zea_mays/Info/Index; Accessed March 4, 2024) utilizing STAR (v2.7.11, Dobin et al., 2013) and the corresponding annotation file. The pipelines used for the analysis of all three classes of RNA molecules (mRNAs, lncRNAs, and circRNAs) are shown in Figure 1.
Figure 1. The RNA data analysis pipeline. Raw reads, acquired through 150 bp PE sequencing, were passed through quality control and processed to remove reads containing adapter and poly-N sequences and low quality reads (Phred score > 30, N% < 10%). The trimmed reads were then aligned onto the Zea mays B73 NAM 5.0 reference genome. mRNAs could be quantified after mapping, but lncRNAs and circRNAs required additional steps. To identify lncRNAs, the reads were assembled, and filtered (by length, expression level, exon number, and genome position), in addition to assessing the absence of coding potential (CPC2, CPAT, Plek). Additionally, the potential cis- and trans-targets of the identified lncRNAs were predicted. circRNA detection was accomplished through a custom pipeline in Circexplorer2. After all the coding and non-coding RNAs were identified, the quantification, differential expression (DE) analysis, and network construction were performed. Furthermore, functional enrichment (GO, KEGG) analysis was performed for the identified DE mRNAs. Bioinformatics tools used in each step are also shown next to the box containing the step name.
Fragments mapped to each gene were quantified with htseq (v2.0.5, Putri et al., 2022). Principal component analysis (PCA) was performed to calculate the distance between samples using factoextra (v1.0.7, Kassambara and Mundt, 2020) in R (v4.3.3, R Core Team, 2024). Hierarchical cluster analysis was performed in the cluster R package (v2.1.6, Maechler et al., 2023), using normalized read count values and the Euclidian distance method. Differential expression (DE) analysis was performed with edgeR (v4.0, Robinson et al., 2010) to identify significant DE genes between the control and treatment conditions in both LT and LS. The transcripts were filtered based on the expression level (minimal counts per sample > 15), library sizes were normalized and the differential expression was calculated. The statistical significance was determined using the negative binomial distribution test (Anders and Huber, 2010; Roberts et al., 2011). The p-value was adjusted according to the Bayesian interpretation (Storey, 2003), and the adjusted value, or the q-value was further used. Genes with the q-value < 0.01 and log2 fold change (FC) > 1 or < -1 between the control and treatment samples were considered differentially expressed.
The Gene Ontology (GO) enrichment analysis of the DEGs was conducted using clusterProfiler (v4.10.1, Wu et al., 2021) and biomartr database (Drost and Paszkowski, 2017). Significantly enriched GO terms were determined by the p-value < 0.05 with the Fisher’s exact test and the Bonferroni multi-test adjustment. The same package was used to test the statistical enrichment of the target gene candidates in KEGG metabolic pathways (Kanehisa et al., 2008) by applying the same criteria. Co-expression patterns and gene modules were identified based on the weighted gene co-expression network analysis (WGCNA), by utilizing the WGCNA package in R (v1.72, Langfelder and Horvath, 2008).
The mapped reads of each sample were assembled after alignment utilizing Cufflinks (v2.2.1, Trapnell et al., 2010) in a reference-based approach. The assembled transcripts were filtered based on their length (> 200 nt), expression level (FPKM > 0.1), and exon number (>1). After that was completed, transcripts that overlap with known protein-coding genes were also filtered out using a Cufflinks function, cuffcompare, and only the ones with class codes “u”, “i”, “o” and “x” were kept for further analysis. Then, by applying three different approaches: CPC2 (Kang et al., 2017), CPAT (Wang et al., 2013), and Plek (Li et al., 2014), only the lncRNAs determined to lack any coding potential by all three methods were selected. lncRNA-fragment quantification was performed with htseq and the DE analysis between the control and treatment conditions was carried out utilizing edgeR, adopting the same analysis parameters as those implemented in the mRNA study.
Potential lncRNA cis-targets were explored using bedtools (100 kb up- or downstream from the lncRNA coordinates) (v2.31.1, Quinlan and Hall, 2010), while the trans-targets were predicted with the LncTar software (ndG < -0.15, Li et al., 2015a). The co-expression patterns of lncRNAs were predicted by applying the WGCNA R package. Additionally, miRNAs expressed under chilling conditions of 5-d old seedlings (Božić et al., 2024) were selected for the miRNA-lncRNA target prediction, performed using psRobot (v1.2, Wu et al., 2012).
circRNA detection and identification of alternative back-splicing sites were accomplished through a custom computational pipeline in CIRCexplorer2 (v2.0.5, Zhang et al., 2016). It included circRNA fusion junction read alignment and parsing; de novo assembly for circular RNA transcripts using the Cufflinks reference annotation-based transcript (RABT), and characterization of alternative back-splicing sites, including both the 5’ and 3’ alternative back-splicing events. To quantitate circRNA expression, htseq was utilized using fragments that are mapped to the back-spliced exon-exon junction sites. DE analysis between the treated and control samples was performed in edgeR. microRNA target sites in exons of circRNA loci were identified using psRobot and compared to the miRNAs (Božić et al., 2024).
The results of the DE analysis and target prediction of mRNA, lncRNA, and circRNA, as well as of the previously identified miRNAs (Božić et al., 2024) were utilized in constructing complex miRNA-lncRNA, miRNA-circRNA, and miRNA-mRNA regulatory relationships. Furthermore, the regulatory relationships were then combined into a unique lncRNA/circRNA-miRNA-mRNA regulatory network, established using Cytoscape software (v3.10.2, Shannon et al., 2003).
Quantitative real-time PCR (qRT-PCR) was applied to validate the sequencing results. Genes and lncRNAs were chosen for validation based on the FC size and significance, ensuring they are present in most of the sequenced samples. Total RNA was extracted and treated with DNase I, as previously described, from all samples. Revert Aid First Strand cDNA synthesis kit with RNase inhibitor (Thermo Scientific™) was used to synthesize cDNA and the qRT-PCR analysis was carried out using cyclophilin (cyp) as the internal reference gene (Lin et al., 2014) and in three biological replicates for each sample for both mRNAs and lncRNAs. PCR reactions were performed on a StepOnePlus™ Real-Time PCR System (Applied Biosystems™, USA) with the HOT FIREPol® EvaGreen® qPCR Mix Plus (ROX) (Solis BioDyne™). The primers used were designed using Primer 3 (v0.4.0) online software (http://bioinfo.ut.ee/primer3-0.4.0) and checked in NCBI Primer-BLAST tool (https://www.ncbi.nlm.nih.gov/tools/primer-blast). The list of the primers is given in the Supplementary Table S1. Relative gene expression was calculated according to Livak and Schmittgen (2001) using efficiency correction according to Pfaffl (2003). Student t-test was carried out for mean comparison with a significance level at p < 0.05 for qRT-PCR validation results.
In total, 817.8 million raw reads were generated from the sequenced libraries, averaging ≈51.11 million reads per library. After processing the raw reads, 803.2 million high-quality reads remained (on average ≈50.2 million reads). The high-quality reads were then mapped, with the average alignment rate being 93.57% and the unique mapping rate amounting to 78.5%. The detailed information for each sample is listed in Supplementary Table S2.
To analyze the response of 5-d-old maize seedlings, the expression levels were compared between the control and treatment conditions at both time points (6h, 24h). The mRNA transcripts were quantified, filtered, and FPKM values were calculated. PCA analysis showed that more than 70% of the variability in gene expression abundance between the samples can be explained by the first three principal components (Figure 2A). Based on the expression levels, there was a clear separation into four different groups dependent both on the genetic background and experimental conditions (Figure 2B). Agglomerative hierarchical clustering analysis additionally confirmed that the samples were clustered firstly based on treatment conditions, and then based on the differences between LS and LT (Figure 2D). DE analysis was performed on 26,023 genes, and comparisons were made between the control and treatment conditions. In total, 508 genes were found to be DE (q-value < 0.01, -1 ≤ log2FC ≥ 1): 175 in LS after 6h, and 235 after 24h; while in LT 102 were DE after 6h and 163 after 24h (Figure 2C, Supplementary Table S3). Five DE genes were common for both genotypes and both time points (Zm00001eb106430, Zm00001eb113780, Zm00001eb161610, Zm00001eb101660, Zm00001eb325410). Common and unique DE genes for each comparison (LS 6h, LS 24h, LT 6h, and LT 24h) are shown in Figure 3A.
Figure 2. mRNA expression summary. (A) Percentage of explained variances of selected principal components (PCs). (B) PCA graph of individual libraries, explained by the first two PCs (PC1 and PC2). (C) mRNAs differentially expressed between the control and treatment, in the two genotypes (LS, LT), at the two time points (6h and 24h). Up-regulated mRNAs are shown in blue, while down-regulated are in orange. (D) Hierarchical cluster analysis, based on the expression patterns expressed in FPKM of 26,023 mRNAs across the eight libraries.
Figure 3. Differentially expressed (DE) genes. (A) Venn diagram showing the common and unique differentially expressed (DE) genes for each comparison: LS 6h is shown in orange, LS 24h in yellow, LT 6h in blue, and LT 24h in green. (B) Expression profiles of selected DE genes for each comparison (LS 6h, LS 24h, LT 6h, and LT 24h). Fold change is shown as its log 2 value (log2FC), in the range from -10 (blue), over 0 (white), to 10 (red).
Most of the DE genes encoded proteins involved in several aspects of photosynthesis. Genes necessary for photosystem I (PSI) assembly – psa genes (psaD, psaE, psaG, psaH, psaL, psaN), as well as those needed for the assembly of photosystem II (PSII) – psb genes (psbP, psbQ-1), were down-regulated in both genotypes at either of the time points. Genes important for the stability of the light-harvesting complex (LHC) were also down-regulated – lhcA-p4, lhcB5, cab1, cab7, cab48. Additionally, genes involved in the Calvin cycle and ribulose bisphosphate carboxylase/oxygenase, Rubisco, activity regulation were also found to be DE: rca, rbcx2, and prk. Several genes important for photoinhibition protection were up-regulated: ctpa2, elip1, elip2, grxs5, grxs17, psy2, thi1, UF3GT, and pds. On the other hand, many genes encoding heat shock proteins (HSP) and heat stress transcription factors (HSF) were down-regulated. The hsp/HSF genes, down-regulated after 24h in LS, included hsp16, hsp17.4, hsp17.5, hsp17.6, hsp18, hsp21, hsp23, hsp26, hsp70-8, hsp70-17, hsp82, HSFB2a, and HSFB2b. The only exception was hsp17, up-regulated in LS after 24h. On the other hand, only hsp16, HSFA6b, HSFB2a, and HSFB2b were down-regulated in LT after 24h. Details of the expression profiles of the selected DE genes are shown in Figure 3B.
GO enrichment analysis revealed the DE genes were mainly enriched in two processes: “response to abiotic stimulus” (16 mRNAs) and “photosynthesis” (9 mRNAs). mRNAs enriched in “response to abiotic stimulus” could be further categorized into those enriched in “response to temperature stimulus” (heat, cold), “response to oxidative stress”, but also “response to light stimulus” (Figure 4, Supplementary Table S4). The KEGG pathway results of the enrichment analyses revealed that the DE mRNAs were mostly enriched in the pathways of “Energy metabolism” (Circadian rhythm) and “Environmental adaptation” (Photosynthesis) (Supplementary Table S4).
Figure 4. Functional enrichment of differentially expressed mRNAs. (A) Bar chart showing the results of over-representation analysis (ORA) for the Biological Process GO class. (B) Emap plot showing the results of over-representation analysis (ORA) for the Biological Process GO class.
There were six significant gene clusters (p < 0.05) associated with the LT treatment identified in the WGCNA analysis: three positively and three negatively correlated. Additionally, five gene clusters were significantly associated with the tolerant genotype, LT. Among the gene clusters associated with the LT treatment or the tolerant genotype, the genes were filtered based on the module membership and the significance of the expression of the gene in question to the associated trait. The resulting genes and modules are shown in Supplementary Table S5. GO enrichment analyses were performed on these genes, showing that genes of three modules associated with the LT treatment were significantly enriched in peptide biosynthetic process and nucleosome organization. On the other hand, genes belonging to modules associated with the tolerant genotype were functionally enriched in response to water deprivation, response to increased salt levels, and nucleosome organization.
After the transcripts were assembled, they were filtered and only the ones longer than 200 nt, with the expression level higher than 0.1, containing more than one exon and not overlapping with known protein-coding genes were kept, leaving only the 24,677 transcripts with class codes “u”, “i”, “o” and “x”. Three different approaches were then applied to identify lncRNAs among those transcripts: CPC2, CPAT, and Plek. Only the 786 transcripts determined to lack any coding potential by all three methods were considered as reliably expressed lncRNAs (Figure 5A, Supplementary Table S6). Comparisons were made between the lncRNA and coding gene positions in the genome showing that 66.8% were intergenic lncRNAs (lincRNAs) (Figure 5B). Also, comparing lncRNA to coding genes revealed that lncRNA genes had fewer exons than the coding ones. Single-exon lncRNA genes comprised 40.1% of all lncRNA genes, while those with two exons took up 55.2%. On the other hand, coding genes with one and/or two exons made up only 37.2%, while genes with three or more exons took up 62.8%. Additionally, 62.9% of lncRNAs were <500 nt, and mRNAs were of similar length – 54.4% were <500 nt. However, the percentage of those longer than 1000 nt was higher in the mRNA group (17.5%), compared to lncRNAs (4.2%).
Figure 5. Summary of the lncRNA identification and quantification. (A) Potential lncRNAs identified through the three different approaches: CPC2 (yellow), CPAT (orange), and Plek (blue); and the identified lncRNAs common for different pairs and all three approaches. (B) Classification of identified lncRNAs into four classes: intergenic (orange), intronic (purple), sense (blue), and antisense lncRNAs (yellow). (C) lncRNA FPKM distribution across the libraries (Ls_C_6, Ls_C_24, Ls_T_6, Ls_T_24, Lt_C_6, Lt_C_24, Lt_T_6, Lt_T_24).
Quantification of lncRNAs was performed in htseq and both raw counts and FPKM values were calculated (Figure 5C). DE lncRNAs under LT stress were calculated through the edgeR package and 63 lncRNAs, between the control and treatment samples, were identified across the two genotypes and time points (Figure 6A, Supplementary Table S7). Unlike the mRNAs, no lncRNA was common for both genotype and treatment duration (Figure 6B). More than half were found to be DE in only one of the time points in a single genotype. XLOC_000175 was the only lncRNA DE in both genotypes after 6h: LT-6h logFC = 2.13; LS-6h logFC = 2.28. On the other hand four DE lncRNAs were common for both genotypes after 24h: XLOC_001043 (LT logFC = 2.38, LS logFC = 3.83), XLOC_006714 (LT logFC = 11.65, LS logFC = 4.67), XLOC_015129 (LT logFC = 2.88, LS logFC = 3.08), and XLOC_016664 (LT logFC = 6.20, LS logFC = 3.69). WGCNA analysis showed a single cluster of 153 lncRNAs associated with the LT treatment, in which 41 lncRNAs showed high intramodular connectivity (p < 0.05, Supplementary Table S8). Among the DE lncRNAs, eight were found to be potential hub genes in the module associated with the LT treatment (XLOC_000816, XLOC_001043, XLOC_008440, XLOC_009662, XLOC_010836, XLOC_011976, XLOC_012259, XLOC_016214).
Figure 6. Differentially expressed lncRNA. (A) lncRNAs differentially expressed between the control and treatment, in the two genotypes (LS, LT), at the two time points (6h and 24h). Up-regulated lncRNAs are shown in blue, while down-regulated are in orange. (B) Unique and common differentially expressed lncRNAs between the two genotypes (LS, LT) and time points (6h and 24h). Ls_6h is shown in orange, Ls_24h in yellow, while Lt_6h is presented in blue, and Lt_24h in green.
Gene targets of lncRNA were identified using two methods: potential cis-targets were searched for 100 kb up- or downstream using bedtools, and the trans-targets were predicted with the LncTar software. A total of 362 potential cis-targets of 63 DE lncRNA were discovered (Supplementary Table S9), with targets of only five lncRNAs being DE: XLOC_009238-Zm00001eb069040, XLOC_016169-Zm00001eb164390, XLOC_010836-Zm00001eb113210, XLOC_006594-Zm00001eb420380, XLOC_015072-Zm00001eb180830. Additionally, only three had Uniprot characterizations: rca (Zm00001eb164390), tic32 (Zm00001eb420380), and sweet (Zm00001eb180830). XLOC_016169 appeared to have a negative effect on the expression of rca in LT, after 24h of LT exposure; while XLOC_006594 and XLOC_015072 showed a positive correlation with their target genes, tic32 and sweet, respectively – both pairs were down-regulated after 24h. On the other hand, 191 potential trans-targets of 22 DE lncRNAs were discovered (Supplementary Table S10). Only 66 of the potential target mRNAs were DE. Most significantly, the target analysis showed that XLOC_012388 positively influenced the expression of hsp17. Also, elip2 was shown to be targeted by three different lncRNAs (XLOC_016783, XLOC_002167, and XLOC_006091) in the two genotypes. These lncRNAs were upregulated after 6h, and seem to positively influence elip2 expression as well. psRobot was used to identify potential lncRNA targets of previously identified miRNAs expressed in LT conditions of 5-d old seedlings (Božić et al., 2024), and it has been identified that nine miRNAs may potentially have an effect on seven lncRNAs (Supplementary Table S11). Only XLOC_009553, targeted by zma-miR166k-5p, was DE. However, zma-miR166k-5p was not shown to be DE in Božić et al. (2024).
The circRNAs involved in the LT response in 5d-old seedlings were identified, along with the alternative back-splicing sites using CIRCexplorer2. Additionally, the circRNAs were quantified through htseq and only the ones with FPKM > 0.1 in at least one library were considered for further analysis. These included 135 identified circRNAs (Figure 7A, Supplementary Table S12). Most of the identified circRNAs were 200-800 nt in length (Figure 7B). Exonic circRNAs made up the most of expressed circular RNAs (46.94%), while the intronic and intergenic comprised 32.43% and 20.63%, respectively. Nearly 83% were single-exon circRNAs, while the rest contained up to six internal exons (Figure 7C). Also, 86.67% of circRNAs were produced by a single parent gene. Two circRNAs from one gene accounted for 10.37%, and three different circRNAs made up 1.48%. Only two genes were responsible for forming more than three circRNA isoforms: Zm00001eb224050 generated four circRNAs, while Zm00001eb420520 held six different circRNAs (Figure 7D). edgeR was used to detect DE circRNAs between the treated and control samples, but none were found. Additionally, 11 microRNAs were found to potentially target 11 circRNAs (Supplementary Table S13).
Figure 7. Identified circRNAs. (A) circRNA FPKM distribution across the samples (Ls_C_6, Ls_C_24, Ls_T_6, Ls_T_24, Lt_C_6, Lt_C_24, Lt_T_6, Lt_T_24) of the selected 135 circRNAs. (B) Length distribution across the eight libraries of the selected 135 circRNAs. (C) Exon number of the selected 135 circRNAs. 83% of circRNAs had one exon and are shown in blue, those with two accounted for 14% and are shown in orange, while the rest of circRNAs were represented with 1% in each category: circRNAs with three exons shown in yellow, those with four in purple and six in light blue. (D) The number of parent genes (y-axis) that generated different numbers of circRNAs (x-axis).
Non-coding RNAs have the ability to target coding RNA molecules and regulate their expression through different mechanisms. The lncRNA/circRNA-miRNA-mRNA networks can be constructed based on these interactions, so first each of the individual interactions between RNA classes and their correlations had to be predicted. Potential lncRNA-miRNA, lncRNA-mRNA, and circRNA-miRNA interactions were already described in previous sections. Potential mRNA-miRNA interactions were also analyzed in Božić et al., 2024.
Due to the lack of differentially expressed target RNAs (circRNAs and lncRNA-targets of miRNAs), the only parts of the network that could be predicted were lncRNA-mRNA and miRNA-mRNA. The network showed that the expression of 19 lncRNAs was correlated to the expression patterns of 41 target mRNA (Figure 8). XLOC_012388 acted as a hub-regulator, affecting the largest number of target genes. Most of the XLOC_012388 target genes had no functional Uniprot annotation, but the ones that did were involved in growth and development (protodermal factor 1; zinc finger protein 2; small auxin up-regulated protein 37, SAUR37), photosynthesis (oxygen-evolving enhancer protein 3-1, sedoheptulose-1,7-bisphosphatase), and abiotic stress response (hsp17, hsp26). The elip2 gene (Zm00001eb301270) was possibly positively regulated by three different lncRNAs: XLOC_016783, XLOC_002167 and XLOC_006091. Giberellin-regulated protein 1 gene’s (Zm00001eb051810) expression was also modified by three lncRNAs: XLOC_012259, XLOC_007807, XLOC_012388. Still, most of the genes targeted by more than one lncRNA, such as Zm00001eb069040, Zm00001eb215270, Zm00001eb293270, Zm00001eb156200, did not have functional annotations.
Figure 8. The lncRNA-mRNA coexpression network. Orange nodes represent the lncRNAs, while the blue rectangles represent the target mRNAs. Positive correlation between the lncRNA and mRNA expression is shown with a green arrow, while the negative is shown with a red one. The network showed that the expression of 19 lncRNAs was correlated to the expression patterns of 41 target mRNA.
A total of 1822 genes were identified as targets for both known and novel miRNAs. Seven miRNAs and the target mRNAs were found to have opposite expression patterns, pointing to the possible role of the miRNAs in gene expression regulation (Supplementary Table S14). Two potentially novel miRNAs seemed to target genes important for the Calvin cycle: down-regulation of novel_452 had a positive effect on the rbcx2 expression, while novel_696 was up-regulated leading to decreased expression levels of prk. On the other hand, some known miRNAs seem to be directly involved in the LT response. zma-miR164a-3p was upregulated after 24h in LS, and the predicted target gene, HSFBa, was significantly down-regulated.
Sequencing results were validated through qRT-PCR analysis of the selected DE mRNAs and lncRNAs. Six mRNAs (ADO3, THI1, AED1, nat4, pds, rca) and five lncRNAs (XLOC_012565, XLOC_000175, XLOC_001043, XLOC_006714, XLOC_016783) were selected for the analysis. In general, the expression patterns obtained through qRT-PCR matched those obtained through NGS sequencing, with small inconsistencies (for example, in LT-6h, changes in expression of aed1 and thi1 were detected in qRT-PCR, but not in sequencing results). Results of the qRT-PCR validation of mRNAs are shown in Supplementary Figure S1, while lncRNA validation results are shown in Supplementary Figure S2.
Photosynthesis is one of the most important metabolic processes in plants, and is crucial for successful growth and development, as well as the plant yield (Muhammad et al., 2021). However, it is significantly affected by various abiotic stressors, including low temperatures (Gururani et al., 2015). This is particularly true for C-4 plants, such as maize, that have a more sensitive photosynthetic apparatus (Bilska-Kos et al., 2018). Low temperatures affect photochemical efficiency of the PSI and PSII, chlorophyll biosynthesis, Calvin cycle enzyme activity, particularly Rubisco (Muhammad et al., 2021). Suboptimal temperatures also lead to the generation of reactive oxygen species (ROS) (Nishiyama and Murata, 2014), which cause further oxidative damage to the photosystems and inhibition of PSII repair (Nishiyama et al., 2011; Gururani et al., 2015). This research found, that by affecting the expression of certain genes, the LT treatment in 5-day-old seedlings had an effect on the PSI and PSII assembly, light-harvesting complex (LHC) stability, and Rubisco activity. Additionally, the treatment temperatures activated certain defensive responses when it comes to oxidative damage caused by the destabilization of the photosynthetic apparatus.
LT affected the integrity of both photosystems in the 5d-old maize seedlings. Genes encoding structural proteins of both PSI and PSII reaction centers, and the components of the antenna complexes were down-regulated in both genotypes. The down-regulated genes included: psa genes necessary for PSI reaction center assembly (Ozakca, 2013); psb genes required for PSII assembly and stability (Pagliano et al., 2013); and several genes responsible for the expression of chloroplastic chlorophyll a-b binding (CAB) proteins of the LHC. CAB proteins comprise the antenna LHC of both PSI and PSII (Zhao et al., 2020). LHC are necessary for the initiation of photochemical reactions, maintaining thylakoid membrane structure, and regulating excitation energy distribution between PSII and PSI (Gan et al., 2019). Down-regulation of the psa, psb, lhc, and cab genes in LT conditions has been reported before in various plant species, including maize (Velitchkova et al., 2020; Yu et al., 2021; Banović Đeri et al., 2022; Balassa et al., 2022), implying that the light-dependent reactions are particularly sensitive to LT.
Several genes involved in the regulation of the Calvin cycle and Rubisco activity were found to be DE. Rubisco activity is a key factor in determining the rate and proper functioning of photosynthesis under both optimal and LT conditions, particularly in C4 plants. C4 plants have lower levels and capacities of Rubisco compared to C3 plants (Salesse-Smith et al., 2020). Therefore, maintaining adequate Rubisco activity is essential for sustaining the photosynthesis rates needed for survival under environmental stress. Rubisco activase (RCA), encoded by the rca gene, is the enzyme responsible for regulating Rubisco (Bracher et al., 2017). Up-regulation of rca leads to higher photosynthesis rates and productivity (Zhang et al., 2019c; Feng et al., 2023). Data on the effect of low temperatures on rca expression is lacking, but under other abiotic stressors, rca expression was increased in maize (Salesse-Smith et al., 2018; Sparrow-Muñoz et al., 2023). Here, in LT conditions, rca was down-regulated after 24h in both genotypes. Interestingly, rbcx2 gene, encoding one of the proteins necessary for the Rubisco assembly, chloroplastic chaperonin-like RbcX protein 2 was upregulated after 6h of treatment in both genotypes. The RbcX proteins are known to have chaperone activity during the assembly of Rubisco in cyanobacteria (Li et al., 2022), but their homologues were found in higher plants as well, where they fulfill a similar role (Kolesiński et al., 2011). To the authors’ knowledge this is the first note of any rbcx gene being DE under abiotic stress conditions. Additionally, phosphoribulokinase gene, prk, was down-regulated after 24h. PRKs are a part of the regeneration phase of the Calvin cycle (Gurrieri et al., 2021). Again, the data on prk expression levels under abiotic stress in maize is lacking, but the gene’s down-regulation was reported in cold-treated Iranian wheat (Rinalducci et al., 2011). prk was also down-regulated in salt and drought-treated wheat (reviewed in Kosová et al., 2015).
The results show that LT negatively affected the light-independent phase of photosynthesis in 5-day-old maize seedlings, by limiting carbon fixation and ribulose regeneration in the Calvin cycle, regardless of genotype. However, in the first 6h of treatment, a course of action was taken to alleviate the described negative effects by intensifying the assembly of Rubisco, through rbcx2 up-regulation. The expression levels of rbcx2 were significantly higher in LT, offering a possible explanation for a better recovery of the Calvin cycle after 24h in the tolerant genotype.
The damages to both photosystems and the reduction of the Calvin cycle enzyme activity, can lead to notable decreases in photosynthetic efficiency. Lowered photosynthetic efficiency results in inhibited electron transport and an increase in ROS levels and photoinhibition (Gan et al., 2019). This further leads to the over-excitation and loss of structural integrity of PSII (Tikkanen and Aro, 2014), as well as to the hindrance of PSII damage repair (Nishiyama and Murata, 2014). Protein responsible for the PSII repair, protein D1, is encoded by the psbA gene (Gururani et al., 2015). Interestingly, while the psbA gene was not found to be DE, a gene encoding the carboxyl-terminal-processing peptidase 2 (CTPA2), necessary for the C-terminal processing and activation of the D1 protein (Che et al., 2013) was upregulated in both genotypes after 6h. This finding suggests that, while the synthesis of protein D1 did not differ between the control and treatment conditions, it was activated only under low-temperature conditions as a way of ensuring damage repair of PSII.
Besides the PSII repair, additional protective measures were likely employed to alleviate the harmful effects associated with ROS and photoinhibition. For example, early light-inducible proteins (ELIPs) protect chloroplasts from photodamage, by facilitating energy dissipation that protects PSII from photoinhibition (Liu et al., 2020). Low temperatures were reported to lead to ELIP up-regulation in Arabidopsis (Hayami et al., 2015), and Medicago sativa (Zhuo et al., 2013), but no data exists for maize. Herein, elip2 was up-regulated in LS and both elip1 and elip2 in LT after 6h of treatment. Rizza et al. (2011) showed that in Arabidopsis, elip1 and elip2 were independently regulated under abiotic stress conditions. This coincides with the results in 5d-old seedlings, since elip1 was upregulated only in LT and suggests a factor possibly involved in establishing low temperature tolerance in maize seedlings. Additionally, two glutaredoxin genes (GRX) were upregulated after 6h: GRXS17 in LS, and GRXS5 and GRXS17 in LT. Glutaredoxins (GRXs) are oxidoreductases that are involved in controlling the redox potential and ROS regulation (Meyer et al., 2009). GRXS17 genes were found to be upregulated in Arabidopsis during heat stress (Martins et al., 2020; Rao et al., 2023), and AtGRXS17 overexpression in tomato lead to enhanced chilling tolerance (Hu et al., 2015). GRXS5 was also involved in abiotic stress response in the fern species Pteris vittata (Sundaram et al., 2008), and overexpression of PvGRXS5 in Arabidopsis increased the plants’ tolerance to heat stress and reduced oxidative damage to proteins (Sundaram and Rathinasabapathi, 2010). It appears that GRXs fulfill a similar role in 5d-old maize seedlings, suggesting a possible role in modulating responses to extreme temperatures in different crops. The difference in their expression levels between LS and LT again alludes to the possible role of antioxidative responses being important for establishing chilling tolerance. To the author’s knowledge, this is the first report on their differential expression in maize under low-temperature conditions.
Another activated photoprotective measure was the antioxidative pigment accumulation, including carotenoids and anthocyanins. Carotenoid levels are increased during abiotic stress periods as a way to limit the damages of ROS (Uarrota et al., 2018). Various plant species displayed increased carotenoid levels under low temperatures (Guo et al., 2022; He et al., 2023), including maize (Obeidat et al., 2018; Waititu et al., 2021). Several enzymes involved in the carotenoid biosynthetic pathway were up-regulated in both genotypes of 5-d old seedlings. The psy2 gene is one of the three phytoene synthases found in maize, and it was upregulated in both genotypes after 6h. The PSY2 enzyme is responsible for carotenoid accumulation in green tissues where the photosynthetic processes occur (Zhou et al., 2022b). The pds gene encoding the 15-cis-phytoene desaturase, that yields 9,15,9’-tri-cis-ζ-carotene (Koschmieder et al., 2017), was up-regulated in both genotypes after 24h. An interesting difference between the genotypes was the apparent increase in anthocyanin content only in LT, through the up-regulation of an enzyme involved in the biosynthetic pathway: anthocyanidin 3-O-glucosyltransferase (UF3GT). UF3GT expression was increased in the treatment conditions at both time points in LT. Anthocyanin accumulation was connected to enhanced protection from photoinhibition in maize leaves at low temperatures (Rodríguez et al., 2014; Yu et al., 2022).
In conclusion, the findings of changes in expression regarding photosynthesis-related genes, show that LT significantly affected the photosynthetic process regardless of genotype and their susceptibility to this stress factor: by affecting the PS assembly and their photorecepting units (LHC), as well as the activity of crucial enzymes, like Rubisco. However, the difference in low-temperature susceptibility between the genotypes in the VE stage could be attributed to the courses of action taken to limit the damage caused by LT-induced ROS accumulation and photoinhibition. LT seemed to be more efficient in handling the oxidative damages: with higher expression levels of proteins and enzymes with antioxidative roles, such as CTPA2, ELIPs, GRXs, UF3GT (Supplementary Figure S3).
GO analysis showed that the DE genes enriched in the response to abiotic stimuli, encompassed reactions to both heat and cold. Most of the genes belonged to the HSP and HSF family. Interestingly, nearly every identified hsp or HSF gene was down-regulated. The only exception was hsp17, up-regulated in LS after 24h. Still, a larger number of down-regulated hsp/HSF genes were identified in LS, rather than LT. LT is most often associated with the accumulation of HSPs, as a way to decrease protein dysfunction and denaturing (Hlaváčková et al., 2013; ul Haq et al., 2019), but this is not the case for every plant species. There are reports of both HSPs and HSFs up-regulation (Kollipara et al., 2002; Meng et al., 2022), as well as their down-regulation in maize (Li et al., 2020; Sowiński et al., 2020). Sowiński et al. (2020) compared several independent transcriptomic research studies focusing on the response of maize seedlings to moderate (12–15°C) or severe cold stress (below 8°C). They found that in both cases hsp genes were mostly down-regulated, but the number of DE hsp genes was higher in maize plants subjected to severe cold conditions. Unlike LT, expression patterns in LS were more similar to those found in studies with maize subjected to temperatures lower than 8°C, suggesting the temperatures applied in this research affected the susceptible genotype more gravely. Since the up-regulation of HSPs is often considered a crucial aspect of the plant’s response to any abiotic stressor (Janmohammadi et al., 2015), it would be safe to assume that the inability to limit protein denaturation and dysfunction, can be a significant factor in maize susceptibility to low temperatures, as well as in the differences in susceptibility between the different maize genotypes.
Two DE genes were enriched for response to cold: thiamine thiazole synthase, THI1, and the previously mentioned, pds, involved in carotenoid biosynthesis. THI1 is involved in the biosynthesis of thiazole, a precursor of thiamine (vitamin B1). Thiamine is involved in various metabolic processes such as glycolysis, pentose phosphate pathway, and the tricarboxylic acid cycle (Yusof, 2019), but also abiotic stress response (Yee et al., 2016). THI1 levels were shown to be increased under drought response in Arabidopsis (Li et al., 2016), and Medicago sativa (Yin et al., 2022). The down-regulation of THI1 only in LS can also be a factor in the difference in LT susceptibility between genotypes. To the author’s knowledge, this is the first description of THI1 involvement in LT response in maize.
lncRNAs are known to have important roles in the growth and development of plants, as well as responses to environmental changes, including low temperatures (Biswas et al., 2021). Many lncRNAs have been identified under LT stress in numerous plant species: Arabidopsis (Calixto et al., 2019), rice (Leng et al., 2020), wheat (Lu et al., 2020). lncRNAs expressed under abiotic stresses have been researched in maize as well: under conditions of drought (Pang et al., 2019), heat (Hu et al., 2022), nitrogen deficiency (Ma et al., 2021b). The involvement of lncRNAs in the low temperature response has also been studied in V6 maize seedlings (Waititu et al., 2021) and primary root tips of 8-day-old seedlings (Xuhui et al., 2022). To the author’s knowledge, this is the first study of lncRNAs in 5-day-old maize seedlings.
Herein, 786 transcripts were determined to satisfy the necessary criteria to be considered lncRNAs. A large number of the transcripts were designated as lincRNAs, each less than 1000 nucleotides in length, which corresponds with the findings of Xuhui et al. (2022). Additionally, lncRNA genes with 1-2 exons comprised more than 95% of all expressed lncRNAs, a much higher ratio than in mRNA genes (37%). Similar results were reported in other works related to maize lncRNAs under abiotic stress (Yu et al., 2020; Hu et al., 2022; Liu et al., 2022b). Of the 63 DE lncRNAs, more than half were found to be DE in only one of the time points in a single genotype and none were common for both genotypes and time-points. Also, the lncRNA expression levels were significantly lower than the levels of expression of mRNAs, as reported in previous studies. Further characterization of identified and DE lncRNAs was made difficult due to the lack of specialized databases for plant lncRNAs. For example, the most widely used database PLncDB (Jin et al., 2021), contains more than 30,000 entries for maize but only 26 are validated.
lncRNA research is still in the phase of large-scale identification, rather than functional characterization (Gonzales et al., 2024). Still, some of their functions, particularly through their interaction with other RNA classes, such as miRNAs, are known. Since none of the miRNA-lncRNA pairs were DE, making any conclusions about the interaction is difficult. lncRNAs can also directly target the expression of genes positioned in the vicinity of their transcription sites in a cis-manner, regulating genes found at or near the same genomic locus, or in a trans-manner, at independent chromosomal loci (Fatica and Bozzoni, 2014; Waseem et al., 2021). Regarding cis-targets, XLOC_016169 negatively impacted rca expression in LT, suggesting its role in regulating Rubisco activity. XLOC_006594 seemed to positively influence tic32 (chloroplastic protein TIC32) expression; while XLOC_015072 also showed positive expression correlation with target gene sweet (bidirectional sugar transporter, SWEET). TIC32 is a short-chain dehydrogenase, part of the TIC (translocon of the inner chloroplast envelopes) complex, essential for chloroplast biogenesis (Hörmann et al., 2004). There is no data on tic32 expression under low-temperature conditions, but under heat stress, it was reduced in pea (Dutta et al., 2009). SWEET has been shown to have an important role in low-temperature tolerance in several plant species (Miao et al., 2017; Zhang et al., 2019a). Their down-regulation in LS only may point to their role in establishing low temperature tolerance in maize lines.
Potential DE trans-mRNA targets were involved in growth and development (germination, tissue differentiation; Casparian strip development), abiotic stress response (heat shock proteins, antioxidative response), and protein degradation. This result suggests the possibility of maize lncRNAs being directly involved in the plants’ response to LT, by affecting the expression of genes involved in the stress response (rca, elip2, hsp17), but confirmation of this assumption would require further characterization of the lncRNAs and exploration of their role in this pathway.
circRNAs have been identified in various plant species, such as Arabidopsis (Ye et al., 2015), rice (Zhou et al., 2021), wheat (Han et al., 2021), and maize (Han et al., 2020; Xu et al., 2024). circRNAs have been shown to be involved in the response of maize plants to drought (Zhang et al., 2019b; Xu et al., 2024), increased salt levels (Liu et al., 2022b), soil nitrogen deficiency (Ma et al., 2021a). The involvement of circRNAs in the maize cold response was described only in a meta-analysis done by Tang et al. (2018). To the authors’ knowledge this is the first description of their identification and expression in 5-day-old maize seedlings.
Initial identification revealed 6951 circRNAs. However, nearly 91% had expression levels lower than the set threshold, meaning they were present with less than 10 reads across all libraries. To ensure reliable expression analysis, such transcripts were not considered as expressed circRNAs with high confidence and were not regarded in downstream analyses, leaving 135 circRNAs for further analyses. Most of the identified circRNAs were 200-800 nt in length, which is in line with other research works regarding maize circular RNAs, as well as those of other plant species (Han et al., 2020; Ma et al., 2021a). On the other hand, the largest ratio of the expressed circRNAs was exonic circular RNAs. In maize, the classification of circRNAs based on the positional relationship between the circRNAs and their parent gene is not uniform. There are reports of different ratios of exonic, intronic, and intergenic circRNAs, but in all circRNAs are mostly of exonic origin, with intronic and intergenic circRNAs varying in proportion (Ma et al., 2021a; Xu et al., 2024). Alternative back-splicing is a process in which multiple circRNAs are derived from the same back-splice site in a single gene (Tang et al., 2018). Despite detecting 63 unique back-splice junctions in the 5d-old maize seedlings, nearly 90% of circRNAs were produced from a single gene. Parent genes of multiple circRNAs were responsible for the formation of less than 15% of all circRNAs. Again, there are multiple reports of alternative back-splicing events in maize, with the percentage of single-gene-origin circRNAs covering different proportions of total circRNAs. However, findings similar to the ones from this research were reported by Luo et al. (2019) and Liu et al. (2022b). When it comes to the involvement of identified circRNAs in the LT response, no DE circRNAs were detected in the 5d-old maize seedlings. Also, despite identifying eleven miRNAs potentially targeting identified circRNAs, the target circRNAs, nor their parent genes, were DE. For this reason, no conclusions could be drawn about the role of miRNAs in regulating circRNAs under chilling conditions.
Circular RNAs are known to be expressed at levels lower than linear RNAs in plants and animals (Wang et al., 2014; Liu et al., 2023). Still, expression levels detected in this research are even lower than those reported in existing literature. For example, Han et al. (2020) showed that in V3 and V5/V6 maize seedlings ≈70% of circRNAs were expressed with less than ten reads across all libraries, which is lower than the ratio identified in this experiment (91%). A possible explanation for this finding could be the examined vegetative stage: five days post-germination did not seem to be enough time for circRNAs to accumulate and be effectively included in the low-temperature response.
Potential interactions between the lncRNAs and other RNA classes (miRNA, mRNA) needed for the lncRNA/circRNA-miRNA-mRNA network construction, were already described in previous chapters, just like circRNA-miRNA associations.
zma-miR164a-3p negatively impacted the HSFBa expression. miR164a-3p were previously identified to participate in the salt treatment response in maize (Fu et al., 2017) and tomato (Wang et al., 2021). However, miR164a-3p were down-regulated in both plant species under the salt treatment. To the authors’ knowledge, this is the first time their role in targeting heat stress factors under the chilling treatment was described in maize. Despite finding significant miRNA-mRNA interactions, the target pairs did not form a network, but were all separate entities: there were no individual miRNAs found that affect multiple genes, nor target genes regulated by several miRNAs.
The lncRNA-mRNA coexpression network comprised of 19 lncRNAs and 41 target mRNAs (Figure 8). As previously stated, lncRNAs can affect target genes through multiple mechanisms, including directly binding to mRNAs to regulate the mRNA stability, interfering with transcription, as well as acting as miRNA sponges as explained in the ceRNA hypothesis (He et al., 2020), resulting in different co-expression patterns, seen here as well. lncRNA XLOC_012388 acted as a hub-regulator, affecting the largest number of target genes. lncRNAs affected the expression of genes involved in growth and development, photosynthesis, and abiotic stress response. Still, most of the genes targeted by the lncRNAs, did not have functional annotations, which made drawing any conclusions about the proposed regulatory network more difficult.
Due to the lack of DE circRNAs and the lncRNA-targets of miRNAs not being DE, constructing the complete lncRNA/circRNA-miRNA-mRNA network was difficult, despite many potential cases. For example, the target prediction showed that several lncRNAs (XLOC_005314, XLOC_010450) and circRNAs (bna_circ_824, bna_circ_1505) have binding sites for zma-miR169i-3p, which they share with two target genes (Zm00001eb339510, Zm00001eb286120). This suggests a possible role of those non-coding RNAs in modulating the expression of target mRNAs, but it could not be confirmed due to the lack of expression data. A possible explanation could be the analyzed developmental stage in which lower expression levels, particularly of the noncoding RNA molecules, were detected.
The impact of low temperatures on the levels of coding and long non-coding RNAs in 5-day-old maize seedlings, during the VE stage, is substantial, allowing for conclusions to be drawn regarding the plants’ responses and the distinctions between tolerant and susceptible genotypes. According to the results, photosynthesis is the process most affected by low temperatures. The LT treatment had an effect on the integrity of the reaction centers and antenna complexes of both photosystems, as well as on the Calvin cycle enzyme activity and chloroplast assembly, by affecting the expression of various important genes (various psa, psb, lhc, cab genes; rca; prk; tic32). Since such a decrease in photosynthetic efficiency can lead to the generation of ROS and photoinhibition, it was not surprising that many genes involved in limiting the photooxidative damage were upregulated (ctpa2, grxS17, grxS5, elip1, elip2, UF3GT). It was in the expression of these genes where differences between the genotypes could be seen: according to the results, LT seemed better equipped at fighting photoinhibition through a more significant up-regulation of these genes. Additionally, the expression of many of the genes seemed to be regulated by non-coding RNA: XLOC_016169-rca; XLOC_006594-tic32; XLOC_016783-XLOC_002167-XLOC_006091-elip2; novel_452-rbcx2; novel_696-prk. However, the low levels of non-coding RNA expression in this developmental stage prevented the complete lncRNA/circRNA-miRNA-mRNA network from being formed. Certain genes’ roles in the low-temperature or abiotic stress response in maize weren’t previously described: rbcx, grxS17, grxS5, THI1; and neither were the interactions with lncRNAs.
This research is the first of its kind to be carried out during such an early developmental stage, such as VE, under LT conditions in maize. Additionally, to the authors’ knowledge it is the first study of lncRNAs and circRNAs during emergence in maize. Consequently, it sheds light on the responses of young maize plants to LT environments, specifically through changes in their transcriptomic expression. Insights from this study lay the groundwork for subsequent network analyses in later developmental phases and across diverse maize genotypes, as well as the potential mechanisms underlying the maize low-temperature tolerance/susceptibility. As such, it offers valuable guidance for future research directions in the molecular breeding of chilling-tolerant maize.
The datasets presented in this study can be found in online repositories. The names of the repository/repositories and accession number(s) can be found below: https://www.ebi.ac.uk/ena, PRJEB80094.
MB: Conceptualization, Data curation, Formal Analysis, Investigation, Validation, Writing – original draft, Writing – review & editing. DIM: Conceptualization, Investigation, Writing – review & editing. VA: Funding acquisition, Resources, Writing – review & editing. ND: Funding acquisition, Resources, Writing – review & editing. AN: Conceptualization, Investigation, Writing – review & editing.
The author(s) declare that financial support was received for the research, authorship, and/or publication of this article. This work was supported by Maize Research Institute Zemun Polje (internal research fund) and Ministry of Science, Technological Development and Innovation under Grant No. 451-03-66/2024-03/200040.
We would like to thank Dr. Jason Wallace, as well as the rest of The Wallace Lab at the University of Georgia (UGA), for the help and dedication provided to MB in mastering the bioinformatics techniques. Also, we wanted to thank the members of The Laboratory for Molecular Genetics and Physiology at the Maize Research Institute Zemun Polje for all their assistance during this research study.
The authors declare that the research was conducted in the absence of any commercial or financial relationships that could be construed as a potential conflict of interest.
The author(s) declare that no Generative AI was used in the creation of this manuscript.
All claims expressed in this article are solely those of the authors and do not necessarily represent those of their affiliated organizations, or those of the publisher, the editors and the reviewers. Any product that may be evaluated in this article, or claim that may be made by its manufacturer, is not guaranteed or endorsed by the publisher.
The Supplementary Material for this article can be found online at: https://www.frontiersin.org/articles/10.3389/fpls.2025.1527447/full#supplementary-material
Anders, S., Huber, W. (2010). Differential expression analysis for sequence count data. Genome Biol. 11, R106. doi: 10.1186/gb-2010-11-10-r106
Andrews, S.2010 Babraham Bioinformatics - FastQC A Quality Control tool for High Throughput Sequence Data. Available online at: https://www.bioinformatics.babraham.ac.uk/projects/fastqc/ (Accessed March 14, 2024).
Balassa, G., Oláh, C., Balassa, K., Rácz, I., Kátay, G., Kalapos, B., et al. (2022). Physiological and molecular background of maize cold-tolerance enhancement with S-methylmethionine salicylate. J. Plant Growth Regul. 41, 2073–2091. doi: 10.1007/s00344-022-10695-1
Banović Đeri, B., Božić, M., Dudić, D., Vićić, I., Milivojević, M., Ignjatović-Micić, D., et al. (2022). Leaf transcriptome analysis of Lancaster versus other heterotic groups’ maize inbred lines revealed different regulation of cold-responsive genes. J. Agron. Crop Sci. 208, 497–509. doi: 10.1111/jac.12529
Bassu, S., Fumagalli, D., Toreti, A., Ceglar, A., Giunta, F., Motzo, R., et al. (2021). Modelling potential maize yield with climate and crop conditions around flowering. Field Crops Res. 271, 108226. doi: 10.1016/j.fcr.2021.108226
Beegum, S., Walne, C. H., Reddy, K. N., Reddy, V., Reddy, K. R. (2023). Examining the corn seedling emergence–temperature relationship for recent hybrids: insights from experimental studies. Plants 12, 3699. doi: 10.3390/plants12213699
Benitez-Alfonso, Y., Soanes, B. K., Zimba, S., Sinanaj, B., German, L., Sharma, V., et al. (2023). Enhancing climate change resilience in agricultural crops. Curr. Biol. 33, R1246–R1261. doi: 10.1016/j.cub.2023.10.028
Bilska-Kos, A., Panek, P., Szulc-Głaz, A., Ochodzki, P., Cisło, A., Zebrowski, J. (2018). Chilling-induced physiological, anatomical and biochemical responses in the leaves of Miscanthus × giganteus and maize (Zea mays L.). J. Plant Physiol. 228, 178–188. doi: 10.1016/j.jplph.2018.05.012
Biswas, A., Sen, B., Bandyopadhyay, S., Mal, C. (2021). Co-regulatory functions of miRNA and lncRNA in adapting biotic and abiotic stress in economically important dicot plants. Plant Gene 26, 100275. doi: 10.1016/j.plgene.2021.100275
Bolger, A. M., Lohse, M., Usadel, B. (2014). Trimmomatic: a flexible trimmer for Illumina sequence data. Bioinformatics 30, 2114–2120. doi: 10.1093/bioinformatics/btu170
Božić, M., Ignjatović Micić, D., Delić, N., Nikolić, A. (2024). Maize miRNAs and their putative target genes involved in chilling stress response in 5-day old seedlings. BMC Genomics 25, 479. doi: 10.1186/s12864-024-10403-1
Bracher, A., Whitney, S. M., Hartl, F. U., Hayer-Hartl, M. (2017). Biogenesis and metabolic maintenance of rubisco. Annu. Rev. Plant Biol. 68, 29–60. doi: 10.1146/annurev-arplant-043015-111633
Calixto, C. P. G., Tzioutziou, N. A., James, A. B., Hornyik, C., Guo, W., Zhang, R., et al. (2019). Cold-dependent expression and alternative splicing of arabidopsis long non-coding RNAs. Front. Plant Sci. 10. doi: 10.3389/fpls.2019.00235
Che, Y., Fu, A., Hou, X., McDonald, K., Buchanan, B. B., Huang, W., et al. (2013). C-terminal processing of reaction center protein D1 is essential for the function and assembly of photosystem II in Arabidopsis. PNAS 110, 16247–16252. doi: 10.1073/pnas.1313894110
Collins, B., Chenu, K. (2021). Improving productivity of Australian wheat by adapting sowing date and genotype phenology to future climate. Clim Risk Manag 32, 100300. doi: 10.1016/j.crm.2021.100300
Dasgupta, P., Das, A., Datta, S., Banerjee, I., Tripathy, S., Chaudhuri, S. (2020). Understanding the early cold response mechanism in IR64 indica rice variety through comparative transcriptome analysis. BMC Genomics 21, 425. doi: 10.1186/s12864-020-06841-2
Deryng, D., Conway, D., Ramankutty, N., Price, J., Warren, R. (2014). Global crop yield response to extreme heat stress under multiple climate change futures. Environ. Res. Lett. 9, 34011. doi: 10.1088/1748-9326/9/3/034011
Dobin, A., Davis, C. A., Schlesinger, F., Drenkow, J., Zaleski, C., Jha, S., et al. (2013). STAR: ultrafast universal RNA-seq aligner. Bioinformatics 29, 15–21. doi: 10.1093/bioinformatics/bts635
Dong, R., Zhang, X.-O., Zhang, Y., Ma, X.-K., Chen, L.-L., Yang, L. (2016). CircRNA-derived pseudogenes. Cell Res. 26, 747–750. doi: 10.1038/cr.2016.42
Drost, H.-G., Paszkowski, J. (2017). Biomartr: genomic data retrieval with R. Bioinformatics 33, 1216–1217. doi: 10.1093/bioinformatics/btw821
Dutta, S., Mohanty, S., Tripathy, B. C. (2009). Role of temperature stress on chloroplast biogenesis and protein import in pea. Plant Physiol. 150, 1050–1061. doi: 10.1104/pp.109.137265
Farooq, A., Farooq, N., Akbar, H., Hassan, Z. U., Gheewala, S. H. (2023). A critical review of climate change impact at a global scale on cereal crop production. Agronomy 13, 162. doi: 10.3390/agronomy13010162
Fatica, A., Bozzoni, I. (2014). Long non-coding RNAs: new players in cell differentiation and development. Nat. Rev. Genet. 15, 7–21. doi: 10.1038/nrg3606
Feng, Y., Wu, H., Liu, H., He, Y., Yin, Z. (2023). Effects of osRCA overexpression on rubisco activation state and photosynthesis in maize. Plants 12, 1614. doi: 10.3390/plants12081614
Frascaroli, E., Revilla, P. (2018). “Genomics of cold tolerance in maize,” in The maize genome. Eds. Bennetzen, J., Flint-Garcia, S., Hirsch, C., Tuberosa, R. (Cham, Switzerland: Springer International Publishing), 287–303. doi: 10.1007/978-3-319-97427-9_17
Fu, R., Zhang, M., Zhao, Y., He, X., Ding, C., Wang, S., et al. (2017). Identification of Salt Tolerance-related microRNAs and Their Targets in Maize (Zea mays L.) Using High-throughput Sequencing and Degradome Analysis. Front. Plant Sci. 8. doi: 10.3389/fpls.2017.00864
Gan, P., Liu, F., Li, R., Wang, S., Luo, J. (2019). Chloroplasts— Beyond energy capture and carbon fixation: tuning of photosynthesis in response to chilling stress. Int. J. Mol. Sci. 20, 5046. doi: 10.3390/ijms20205046
Gao, Z., Li, J., Luo, M., Li, H., Chen, Q., Wang, L., et al. (2019). Characterization and cloning of grape circular RNAs identified the cold resistance-related vv-circATS1. Plant Physiol. 180, 966–985. doi: 10.1104/pp.18.01331
Gao, L., Pan, L., Shi, Y., Zeng, R., Li, M., Li, Z., et al. (2024). Genetic variation in a heat shock transcription factor modulates cold tolerance in maize. Mol. Plant 17, 1423–1438. doi: 10.1016/j.molp.2024.07.015
Gonzales, L. R., Blom, S., Henriques, R., Bachem, C. W. B., Immink, R. G. H. (2024). LncRNAs: the art of being influential without protein. Trends Plant Sci. 29, 770–785. doi: 10.1016/j.tplants.2024.01.006
Guo, R., Liu, T., Guo, C., Chen, G., Fan, J., Zhang, Q. (2022). Carotenoid biosynthesis is associated with low-temperature adaptation in Rhodosporidium kratochvilovae. BMC Microbiol. 22, 319. doi: 10.1186/s12866-022-02728-2
Gurrieri, L., Fermani, S., Zaffagnini, M., Sparla, F., Trost, P. (2021). Calvin–Benson cycle regulation is getting complex. Trends Plant Sci. 26, 898–912. doi: 10.1016/j.tplants.2021.03.008
Gururani, M. A., Venkatesh, J., Tran, L. S. P. (2015). Regulation of photosynthesis during abiotic stress-induced photoinhibition. Mol. Plant 8, 1304–1320. doi: 10.1016/j.molp.2015.05.005
Han, Y., Li, X., Yan, Y., Duan, M.-H., Xu, J.-H. (2020). Identification, characterization, and functional prediction of circular RNAs in maize. Mol. Genet. Genomics 295, 491–503. doi: 10.1007/s00438-019-01638-9
Han, Y., Zhao, Y., Wang, H., Zhang, Y., Ding, Q., Ma, L. (2021). Identification of ceRNA and candidate genes related to fertility conversion of TCMS line YS3038 in wheat. Plant Physiol. Biochem. 158, 190–207. doi: 10.1016/j.plaphy.2020.10.037
Hansen, T. B., Jensen, T. I., Clausen, B. H., Bramsen, J. B., Finsen, B., Damgaard, C. K., et al. (2013). Natural RNA circles function as efficient microRNA sponges. Nature 495, 384–388. doi: 10.1038/nature11993
Hayami, N., Sakai, Y., Kimura, M., Saito, T., Tokizawa, M., Iuchi, S., et al. (2015). The responses of arabidopsis early light-induced protein2 to ultraviolet B, high light, and cold stress are regulated by a transcriptional regulatory unit composed of two elements. Plant Physiol. 169, 840–855. doi: 10.1104/pp.15.00398
He, X., Guo, S., Wang, Y., Wang, L., Shu, S., Sun, J. (2020). Systematic identification and analysis of heat-stress-responsive lncRNAs, circRNAs and miRNAs with associated co-expression and ceRNA networks in cucumber (Cucumis sativus L.). Physiol. Plant 168, 736–754. doi: 10.1111/ppl.12997
He, J., Yao, L., Pecoraro, L., Liu, C., Wang, J., Huang, L., et al. (2023). Cold stress regulates accumulation of flavonoids and terpenoids in plants by phytohormone, transcription process, functional enzyme, and epigenetics. Crit. Rev. Biotech. 43, 680–697. doi: 10.1080/07388551.2022.2053056
Hlaváčková, I., Vítámvás, P., Santrůček, J., Kosová, K., Zelenková, S., Prášil, I. T., et al. (2013). Proteins involved in distinct phases of cold hardening process in frost resistant winter barley (Hordeum vulgare L.) cv Luxor. Int. J. Mol. Sci. 14, 8000–8024. doi: 10.3390/ijms14048000
Hörmann, F., Küchler, M., Sveshnikov, D., Oppermann, U., Li, Y., Soll, J. (2004). Tic32, an essential component in chloroplast biogenesis. J. Biol. Chem. 279, 34756–34762. doi: 10.1074/jbc.M402817200
Hu, X., Wei, Q., Wu, H., Huang, Y., Peng, X., Han, G., et al. (2022). Identification and characterization of heat-responsive lncRNAs in maize inbred line CM1. BMC Genomics 23, 208. doi: 10.1186/s12864-022-08448-1
Hu, Y., Wu, Q., Sprague, S. A., Park, J., Oh, M., Rajashekar, C. B., et al. (2015). Tomato expressing Arabidopsis glutaredoxin gene AtGRXS17 confers tolerance to chilling stress via modulating cold responsive components. Hortic. Res. 2, 1–11. doi: 10.1038/hortres.2015.51
Janmohammadi, M., Zolla, L., Rinalducci, S. (2015). Low temperature tolerance in plants: Changes at the protein level. Phytochem 117, 76–89. doi: 10.1016/j.phytochem.2015.06.003
Jiang, R., He, W., He, L., Yang, J. Y., Qian, B., Zhou, W., et al. (2021). Modelling adaptation strategies to reduce adverse impacts of climate change on maize cropping system in Northeast China. Sci. Rep. 11, 810. doi: 10.1038/s41598-020-79988-3
Jin, J., Lu, P., Xu, Y., Li, Z., Yu, S., Liu, J., et al. (2021). PLncDB V2.0: a comprehensive encyclopedia of plant long noncoding RNAs. Nucleic Acids Res. 49, D1489–D1495. doi: 10.1093/nar/gkaa910
Kanehisa, M., Araki, M., Goto, S., Hattori, M., Hirakawa, M., Itoh, M., et al. (2008). KEGG for linking genomes to life and the environment. Nucleic Acids Res. 36, D480–D484. doi: 10.1093/nar/gkm882
Kang, Y.-J., Yang, D.-C., Kong, L., Hou, M., Meng, Y.-Q., Wei, L., et al. (2017). CPC2: a fast and accurate coding potential calculator based on sequence intrinsic features. Nucleic Acids Res. 45, W12–W16. doi: 10.1093/nar/gkx428
Kassambara, A., Mundt, F. (2020). factoextra: extract and visualize the results of multivariate data analyses. Available online at: https://cran.r-project.org/web/packages/factoextra/index.html (Accessed April 30, 2024).
Kolesiński, P., Piechota, J., Szczepaniak, A. (2011). Initial characteristics of RbcX proteins from Arabidopsis thaliana. Plant Mol. Biol. 77, 447–459. doi: 10.1007/s11103-011-9823-8
Kollipara, K. P., Saab, I. N., Wych, R. D., Lauer, M. J., Singletary, G. W. (2002). Expression profiling of reciprocal maize hybrids divergent for cold germination and desiccation tolerance. Plant Physiol. 129, 974–992. doi: 10.1104/pp.000729
Koschmieder, J., Fehling-Kaschek, M., Schaub, P., Ghisla, S., Brausemann, A., Timmer, J., et al. (2017). Plant-type phytoene desaturase: Functional evaluation of structural implications. PloS One 12, e0187628. doi: 10.1371/journal.pone.0187628
Kosová, K., Vítámvás, P., Urban, M. O., Klíma, M., Roy, A., Prášil, I. T. (2015). Biological networks underlying abiotic stress tolerance in temperate crops—A proteomic perspective. Int. J. Mol. Scis 16, 20913–20942. doi: 10.3390/ijms160920913
Langfelder, P., Horvath, S. (2008). WGCNA: an R package for weighted correlation network analysis. BMC Bioinfo 9, 559. doi: 10.1186/1471-2105-9-559
Leng, Y., Sun, J., Wang, J., Liu, H., Zheng, H., Zhang, M., et al. (2020). Genome-wide lncRNAs identification and association analysis for cold-responsive genes at the booting stage in rice (Oryza sativa L.). Plant Genome 13, e20020. doi: 10.1002/tpg2.20020
Li, Z., Huang, C., Bao, C., Chen, L., Lin, M., Wang, X., et al. (2015b). Exon-intron circular RNAs regulate transcription in the nucleus. Nat. Struct. Mol. Biol. 22, 256–264. doi: 10.1038/nsmb.2959
Li, Q., Jiang, Y.-L., Xia, L.-Y., Chen, Y., Zhou, C.-Z. (2022). Structural insights into cyanobacterial RuBisCO assembly coordinated by two chaperones Raf1 and RbcX. Cell Discovery 8, 93. doi: 10.1038/s41421-022-00436-9
Li, J., Ma, W., Zeng, P., Wang, J., Geng, B., Yang, J., et al. (2015a). LncTar: a tool for predicting the RNA targets of long noncoding RNAs. Brief Bioinform. 16, 806–812. doi: 10.1093/bib/bbu048
Li, Y., Wang, X., Li, Y., Zhang, Y., Gou, Z., Qi, X., et al. (2020). Transcriptomic analysis revealed the common and divergent responses of maize seedling leaves to cold and heat stresses. Genes 11, 881. doi: 10.3390/genes11080881
Li, C.-L., Wang, M., Wu, X.-M., Chen, D.-H., Lv, H.-J., Shen, J.-L., et al. (2016). THI1, a thiamine thiazole synthase, interacts with ca2+-dependent protein kinase CPK33 and modulates the S-type anion channels and stomatal closure in arabidopsis. Plant Physiol. 170, 1090–1104. doi: 10.1104/pp.15.01649
Li, H., Yue, H., Xie, J., Bu, J., Li, L., Xin, X., et al. (2021). Transcriptomic profiling of the high-vigour maize (Zea mays L.) hybrid variety response to cold and drought stresses during seed germination. Sci. Rep. 11, 19345. doi: 10.1038/s41598-021-98907-8
Li, A., Zhang, J., Zhou, Z. (2014). PLEK: a tool for predicting long non-coding RNAs and messenger RNAs based on an improved k-mer scheme. BMC Bioinfo 15, 311. doi: 10.1186/1471-2105-15-311
Lin, Y., Zhang, C., Lan, H., Gao, S., Liu, H., Liu, J., et al. (2014). Validation of Potential Reference Genes for qPCR in Maize across Abiotic Stresses, Hormone Treatments, and Tissue Types. PloS One 9, e95445. doi: 10.1371/journal.pone.0095445
Liu, Y., Cai, Y., Li, Y., Zhang, X., Shi, N., Zhao, J., et al. (2022b). Dynamic changes in the transcriptome landscape of Arabidopsis thaliana in response to cold stress. Front. Plant Sci. 13. doi: 10.3389/fpls.2022.983460
Liu, G., Liu, F., Wang, Y., Liu, X. (2022a). A novel long noncoding RNA CIL1 enhances cold stress tolerance in Arabidopsis. Plant Sci. 323, 111370. doi: 10.1016/j.plantsci.2022.111370
Liu, R., Ma, Y., Guo, T., Li, G. (2023). Identification, biogenesis, function, and mechanism of action of circular RNAs in plants. Plant Comm 4, 100430. doi: 10.1016/j.xplc.2022.100430
Liu, D. L., Zeleke, K. T., Wang, B., Macadam, I., Scott, F., Martin, R. J. (2017). Crop residue incorporation can mitigate negative climate change impacts on crop yield and improve water use efficiency in a semiarid environment. Eur. Jl Agron. 85, 51–68. doi: 10.1016/j.eja.2017.02.004
Liu, X., Zhang, Y., Yang, H., Liang, Y., Li, X., Oliver, M. J., et al. (2020). Functional aspects of early light-induced protein (ELIP) genes from the desiccation-tolerant moss syntrichia caninervis. Int. J. Mol. Sci. 21, 1411. doi: 10.3390/ijms21041411
Livak, K. J., Schmittgen, T. D. (2001). Analysis of relative gene expression data using real-time quantitative PCR and the 2(-Delta Delta C(T)) Method. Methods 25, 402–408. doi: 10.1006/meth.2001.1262
Lu, Q., Xu, Q., Guo, F., Lv, Y., Song, C., Feng, M., et al. (2020). Identification and characterization of long non-coding RNAs as competing endogenous RNAs in the cold stress response of Triticum aestivum. Plant Biol. 22, 635–645. doi: 10.1111/plb.13119
Luo, Z., Han, L., Qian, J., Li, L. (2019). Circular RNAs exhibit extensive intraspecific variation in maize. Planta 250, 69–78. doi: 10.1007/s00425-019-03145-y
Ma, P., Gao, S., Zhang, H. Y., Li, B. Y., Zhong, H. X., Wang, Y. K., et al. (2021a). Identification and characterization of circRNAs in maize seedlings under deficient nitrogen. Plant Biol. (Stuttg) 23, 850–860. doi: 10.1111/plb.13280
Ma, P., Zhang, X., Luo, B., Chen, Z., He, X., Zhang, H., et al. (2021b). Transcriptomic and genome-wide association study reveal long noncoding RNAs responding to nitrogen deficiency in maize. BMC Plant Biol. 21, 93. doi: 10.1186/s12870-021-02847-4
Maechler, M., Rousseeuw, P., Strujf, A., Hubert, M., Hornik, K., Studer, M., et al. (2023). cluster: “Finding groups in data”: cluster analysis extended. Available online at: https://cran.r-project.org/web/packages/cluster/index.html (Accessed July 2, 2024). Rousseeuw et al.
Mao, J., Yu, Y., Yang, J., Li, G., Li, C., Qi, X., et al. (2017). Comparative transcriptome analysis of sweet corn seedlings under low-temperature stress. Crop J. 5, 396–406. doi: 10.1016/j.cj.2017.03.005
Martins, L., Knuesting, J., Bariat, L., Dard, A., Freibert, S. A., Marchand, C. H., et al. (2020). Redox modification of the iron-sulfur glutaredoxin GRXS17 activates holdase activity and protects plants from heat stress. Plant Physiol. 184, 676–692. doi: 10.1104/pp.20.00906
Meng, A., Wen, D., Zhang, C. (2022). Dynamic changes in seed germination under low-temperature stress in maize. Int. J. Mol. Sci. 23, 5495. doi: 10.3390/ijms23105495
Meyer, Y., Buchanan, B. B., Vignols, F., Reichheld, J.-P. (2009). Thioredoxins and glutaredoxins: unifying elements in redox biology. Annu. Rev. Gens 43, 335–367. doi: 10.1146/annurev-genet-102108-134201
Miao, H., Sun, P., Liu, Q., Miao, Y., Liu, J., Zhang, K., et al. (2017). Genome-wide analyses of SWEET family proteins reveal involvement in fruit development and abiotic/biotic stress responses in banana. Sci. Rep. 7, 3536. doi: 10.1038/s41598-017-03872-w
Muhammad, I., Shalmani, A., Ali, M., Yang, Q.-H., Ahmad, H., Li, F. B. (2021). Mechanisms regulating the dynamics of photosynthesis under abiotic stresses. Front. Plant Sci. 11. doi: 10.3389/fpls.2020.615942
Murray-Tortarolo, G. N., Jaramillo, V. J., Larsen, J. (2018). Food security and climate change: the case of rainfed maize production in Mexico. Agri ForMeteorol 253–254, 124–131. doi: 10.1016/j.agrformet.2018.02.011
Nishiyama, Y., Allakhverdiev, S. I., Murata, N. (2011). Protein synthesis is the primary target of reactive oxygen species in the photoinhibition of photosystem II. Physiol. Plant 142, 35–46. doi: 10.1111/j.1399-3054.2011.01457.x
Nishiyama, Y., Murata, N. (2014). Revised scheme for the mechanism of photoinhibition and its application to enhance the abiotic stress tolerance of the photosynthetic machinery. Appl. Microbiol. Biotechnol. 98, 8777–8796. doi: 10.1007/s00253-014-6020-0
Obeidat, W., Avila, L., Earl, H., Lukens, L. (2018). Leaf spectral reflectance of maize seedlings and its relationship to cold tolerance. Crop Sci. 58, 2569–2580. doi: 10.2135/cropsci2018.02.0115
Ozakca, D. U. (2013). “Effect of abiotic stress on photosystem I-related gene transcription in photosynthetic organisms,” in Photosynthesis (Rijeka, Croatia: IntechOpen). doi: 10.5772/55350
Pagliano, C., Saracco, G., Barber, J. (2013). Structural, functional and auxiliary proteins of photosystem II. Photosynth Res. 116, 167–188. doi: 10.1007/s11120-013-9803-8
Pang, J., Zhang, X., Ma, X., Zhao, J. (2019). Spatio-temporal transcriptional dynamics of maize long non-coding RNAs responsive to drought stress. Genes 10, 138. doi: 10.3390/genes10020138
Pfaffl, M. (2003). “Quantification strategies in real-time PCR,” in A-Z of quantitative PCR (International University Line (IUL, La Jolla, CA, USA), 87–112.
Pörtner, H.-O., Roberts, D. C., Tignor, M. M. B., Poloczanska, E. S., Mintenbeck, K., Alegría, A., et al. (2022). “Summary for policymakers,” in Climate change 2022: impacts, adaptation and vulnerability. Contribution of working group II to the sixth assessment report of the intergovernmental panel on climate change (Cambridge, UK: Cambridge University Press).
Putri, G. H., Anders, S., Pyl, P. T., Pimanda, J. E., Zanini, F. (2022). Analysing high-throughput sequencing data in Python with HTSeq 2.0. Bioinformatics 38, 2943–2945. doi: 10.1093/bioinformatics/btac166
Quinlan, A. R., Hall, I. M. (2010). BEDTools: a flexible suite of utilities for comparing genomic features. Bioinformatics 26, 841–842. doi: 10.1093/bioinformatics/btq033
Rao, X., Cheng, N., Mathew, I. E., Hirschi, K. D., Nakata, P. A. (2023). Crucial role of Arabidopsis glutaredoxin S17 in heat stress response revealed by transcriptome analysis. Funct. Plant Biol. 50, 58–70. doi: 10.1071/FP22002
R Core Team 2024 R: A language and environment for statistical computing. Available online at: https://www.R-project.org/ (Accessed February 28, 2024).
Rinalducci, S., Egidi, M. G., Karimzadeh, G., Jazii, F. R., Zolla, L. (2011). Proteomic analysis of a spring wheat cultivar in response to prolonged cold stress. Electrophoresis 32, 1807–1818. doi: 10.1002/elps.201000663
Rizza, A., Boccaccini, A., Lopez-Vidriero, I., Costantino, P., Vittorioso, P. (2011). Inactivation of the ELIP1 and ELIP2 genes affects Arabidopsis seed germination. New Phytol. 190, 896–905. doi: 10.1111/j.1469-8137.2010.03637.x
Roberts, A., Pimentel, H., Trapnell, C., Pachter, L. (2011). Identification of novel transcripts in annotated genomes using RNA-Seq. Bioinformatics 27, 2325–2329. doi: 10.1093/bioinformatics/btr355
Robinson, M. D., McCarthy, D. J., Smyth, G. K. (2010). edgeR: a Bioconductor package for differential expression analysis of digital gene expression data. Bioinformatics 26, 139–140. doi: 10.1093/bioinformatics/btp616
Rodríguez, V. M., Butrón, A., Rady, M. O. A., Soengas, P., Revilla, P. (2014). Identification of quantitative trait loci involved in the response to cold stress in maize (Zea mays L.). Mol. Breed. 33, 363–371. doi: 10.1007/s11032-013-9955-4
Salesse-Smith, C. E., Sharwood, R. E., Busch, F. A., Kromdijk, J., Bardal, V., Stern, D. B. (2018). Overexpression of Rubisco subunits with RAF1 increases Rubisco content in maize. Nat. Plants 4, 802–810. doi: 10.1038/s41477-018-0252-4
Salesse-Smith, C. E., Sharwood, R. E., Busch, F. A., Stern, D. B. (2020). Increased Rubisco content in maize mitigates chilling stress and speeds recovery. Plant Biotechnol. J. 18, 1409–1420. doi: 10.1111/pbi.13306
Salmena, L., Poliseno, L., Tay, Y., Kats, L., Pandolfi, P. P. (2011). A ceRNA hypothesis: the Rosetta Stone of a hidden RNA language? Cell 146, 353–358. doi: 10.1016/j.cell.2011.07.014
Shannon, P., Markiel, A., Ozier, O., Baliga, N. S., Wang, J. T., Ramage, D., et al. (2003). Cytoscape: A software environment for integrated models of biomolecular interaction networks. Genome Res. 13, 2498–2504. doi: 10.1101/gr.1239303
Silva-Neta, I. C., Pinho, E. V., Veiga, A. D., Pìnho, R. G., Guimarães, R. M., Caixeta, F., et al. (2015). Expression of genes related to tolerance to low temperature for maize seed germination. Genet. Mol. Res. 14, 2674–2690. doi: 10.4238/2015.March.30.28
Sowiński, P., Fronk, J., Jończyk, M., Grzybowski, M., Kowalec, P., Sobkowiak, A. (2020). Maize response to low temperatures at the gene expression level: A critical survey of transcriptomic studies. Front. Plant Sci. 11. doi: 10.3389/fpls.2020.576941
Sparrow-Muñoz, I., Chen, T. C., Burgess, S. J. (2023). Recent developments in the engineering of Rubisco activase for enhanced crop yield. Biochem. Soc. Trans. 51, 627–637. doi: 10.1042/BST20221281
Storey, J. D. (2003). The positive false discovery rate: a Bayesian interpretation and the q-value. AnnStat 31, 2013–2035. doi: 10.1214/aos/1074290335
Sundaram, S., Rathinasabapathi, B. (2010). Transgenic expression of fern Pteris vittata glutaredoxin PvGrx5 in Arabidopsis thaliana increases plant tolerance to high temperature stress and reduces oxidative damage to proteins. Planta 231, 361–369. doi: 10.1007/s00425-009-1055-7
Sundaram, S., Rathinasabapathi, B., Ma, L. Q., Rosen, B. P. (2008). An arsenate-activated glutaredoxin from the arsenic hyperaccumulator fern pteris vittata L. Regulates intracellular arsenite*. JBC 283, 6095–6101. doi: 10.1074/jbc.M704149200
Tang, B., Hao, Z., Zhu, Y., Zhang, H., Li, G. (2018). Genome-wide identification and functional analysis of circRNAs in Zea mays. PloS One 13, e0202375. doi: 10.1371/journal.pone.0202375
Tikkanen, M., Aro, E.-M. (2014). Integrative regulatory network of plant thylakoid energy transduction. Trends Plant Sci. 19, 10–17. doi: 10.1016/j.tplants.2013.09.003
Trapnell, C., Williams, B. A., Pertea, G., Mortazavi, A., Kwan, G., van Baren, M. J., et al. (2010). Transcript assembly and quantification by RNA-Seq reveals unannotated transcripts and isoform switching during cell differentiation. Nat. Biotechnol. 28, 511–515. doi: 10.1038/nbt.1621
Uarrota, V. G., Stefen, D. L. V., Leolato, L. S., Gindri, D. M., Nerling, D. (2018). “Revisiting carotenoids and their role in plant stress responses: from biosynthesis to plant signaling mechanisms during stress,” in Antioxidants and antioxidant enzymes in higher plants. Eds. Gupta, D. K., Palma, J. M., Corpas, F. J. (Cham, Switzerland: Springer International Publishing), 207–232. doi: 10.1007/978-3-319-75088-0_10
ul Haq, S., Khan, A., Ali, M., Khattak, A. M., Gai, W.-X., Zhang, H.-X., et al. (2019). Heat shock proteins: dynamic biomolecules to counter plant biotic and abiotic stresses. Int. J. Mol. Sci. 20, 5321. doi: 10.3390/ijms20215321
Velitchkova, M., Popova, A. V., Faik, A., Gerganova, M., Ivanov, A. G. (2020). Low temperature and high light dependent dynamic photoprotective strategies in Arabidopsis thaliana. Physiol. Plant 170, 93–108. doi: 10.1111/ppl.13111
Waititu, J. K., Cai, Q., Sun, Y., Sun, Y., Li, C., Zhang, C., et al. (2021). Transcriptome Profiling of Maize (Zea mays L.) Leaves Reveals Key Cold-Responsive Genes, Transcription Factors, and Metabolic Pathways Regulating Cold Stress Tolerance at the Seedling Stage. Genes 12, 1638. doi: 10.3390/genes12101638
Wang, P. L., Bao, Y., Yee, M.-C., Barrett, S. P., Hogan, G. J., Olsen, M. N., et al. (2014). Circular RNA is expressed across the eukaryotic tree of life. PloS One 9, e90859. doi: 10.1371/journal.pone.0090859
Wang, X., Fan, H., Wang, B., Yuan, F. (2023). Research progress on the roles of lncRNAs in plant development and stress responses. Front. Plant Sci. 14. doi: 10.3389/fpls.2023.1138901
Wang, H., Jia, Y., Bai, X., Gong, W., Liu, G., Wang, H., et al. (2024). Whole-transcriptome profiling and functional prediction of long non-coding RNAs associated with cold tolerance in japonica rice varieties. Int. J. Mol. Sci. 25, 2310. doi: 10.3390/ijms25042310
Wang, Z., Li, N., Yu, Q., Wang, H. (2021). Genome-Wide Characterization of Salt-Responsive miRNAs, circRNAs and Associated ceRNA Networks in Tomatoes. Int. J. Mol. Sci. 22, 12238. doi: 10.3390/ijms222212238
Wang, L., Park, H. J., Dasari, S., Wang, S., Kocher, J.-P., Li, W. (2013). CPAT: Coding-Potential Assessment Tool using an alignment-free logistic regression model. Nucleic Acids Res. 41, e74. doi: 10.1093/nar/gkt006
Wang, J., Vanga, S. K., Saxena, R., Orsat, V., Raghavan, V. (2018). Effect of climate change on the yield of cereal crops: A review. Climate 6, 41. doi: 10.3390/cli6020041
Waseem, M., Liu, Y., Xia, R. (2021). Long non-coding RNAs, the dark matter: an emerging regulatory component in plants. Int. J. Mol. Sci. 22, 86. doi: 10.3390/ijms22010086
Wu, T., Hu, E., Xu, S., Chen, M., Guo, P., Dai, Z., et al. (2021). clusterProfiler 4.0: A universal enrichment tool for interpreting omics data. Innovation 2, 100141. doi: 10.1016/j.xinn.2021.100141
Wu, H.-J., Ma, Y.-K., Chen, T., Wang, M., Wang, X.-J. (2012). PsRobot: a web-based plant small RNA meta-analysis toolbox. Nucleic Acids Res. 40, W22–W28. doi: 10.1093/nar/gks554
Xu, H., Twine, T. E., Girvetz, E. (2016). Climate change and maize yield in iowa. PloS One 11, e0156083. doi: 10.1371/journal.pone.0156083
Xu, J., Wang, Q., Tang, X., Feng, X., Zhang, X., Liu, T., et al. (2024). Drought-induced circular RNAs in maize roots: Separating signal from noise. Plant Physiol. 196, kiae229. doi: 10.1093/plphys/kiae229
Xuhui, L., Weiwei, C., Siqi, L., Junteng, F., Hang, Z., Xiangbo, Z., et al. (2022). Full-length transcriptome analysis of maize root tips reveals the molecular mechanism of cold stress during the seedling stage. BMC Plant Biol. 22, 398. doi: 10.1186/s12870-022-03787-3
Yang, Y., Fan, X., Mao, M., Song, X., Wu, P., Zhang, Y., et al. (2017). Extensive translation of circular RNAs driven by N6-methyladenosine. Cell Res. 27, 626–641. doi: 10.1038/cr.2017.31
Yang, G., Zou, H., Wu, Y., Liu, H., Yuan, Y. (2011). Identification and characterisation of candidate genes involved in chilling responses in maize (Zea mays L.). PCTOC 106, 127–141. doi: 10.1007/s11240-010-9900-8
Ye, C.-Y., Chen, L., Liu, C., Zhu, Q.-H., Fan, L. (2015). Widespread noncoding circular RNAs in plants. New Phytol. 208, 88–95. doi: 10.1111/nph.13585
Yee, W. S., Aziz, S. D. A., Yusof, Z. N. B. (2016). Osmotic stress upregulates the transcription of thiamine (vitamin B1) biosynthesis genes (THIC and THI4) in oil palm (Elaies guineensis). AJB 15, 1566–1574. doi: 10.5897/AJB2016.15222
Yin, H., Wang, Z., Li, H., Zhang, Y., Yang, M., Cui, G., et al. (2022). MsTHI1 overexpression improves drought tolerance in transgenic alfalfa (Medicago sativa L.). Front. Plant Sci. 13. doi: 10.3389/fpls.2022.992024
Yu, F., Tan, Z., Fang, T., Tang, K., Liang, K., Qiu, F. (2020). A comprehensive transcriptomics analysis reveals long non-coding RNA to be involved in the key metabolic pathway in response to waterlogging stress in maize. Genes 11, 267. doi: 10.3390/genes11030267
Yu, T., Zhang, J., Cao, J., Cai, Q., Li, X., Sun, Y., et al. (2021). Leaf transcriptomic response mediated by cold stress in two maize inbred lines with contrasting tolerance levels. Genomics 113, 782–794. doi: 10.1016/j.ygeno.2021.01.018
Yu, T., Zhang, J., Cao, J., Li, X., Li, S., Liu, C., et al. (2022). Metabolic insight into cold stress response in two contrasting maize lines. Life 12, 282. doi: 10.3390/life12020282
Yu, Y., Zhang, Y., Chen, X., Chen, Y. (2019). Plant noncoding RNAs: hidden players in development and stress responses. Annu. Rev. Cell DevBiol 35, 407–431. doi: 10.1146/annurev-cellbio-100818-125218
Yusof, Z. N. B. (2019). “Thiamine and its role in protection against stress in plants (Enhancement in thiamine content for nutritional quality improvement),” in Nutritional quality improvement in plants. Eds. Jaiwal, P. K., Chhillar, A. K., Chaudhary, D., Jaiwal, R. (Cham, Switzerland: Springer International Publishing), 177–186. doi: 10.1007/978-3-319-95354-0_7
Zhang, X.-O., Dong, R., Zhang, Y., Zhang, J.-L., Luo, Z., Zhang, J., et al. (2016). Diverse alternative back-splicing and alternative splicing landscape of circular RNAs. Genome Res. 26, 1277–1287. doi: 10.1101/gr.202895.115
Zhang, W., Wang, S., Yu, F., Tang, J., Shan, X., Bao, K., et al. (2019a). Genome-wide characterization and expression profiling of SWEET genes in cabbage (Brassica oleracea var. capitata L.) reveal their roles in chilling and clubroot disease responses. BMC Genomics 20, 93. doi: 10.1186/s12864-019-5454-2
Zhang, X., Wang, W., Zhu, W., Dong, J., Cheng, Y., Yin, Z., et al. (2019b). Mechanisms and functions of long non-coding RNAs at multiple regulatory levels. Int. J. Mol. Sci. 20, 5573. doi: 10.3390/ijms20225573
Zhang, F., Yang, J., Zhang, N., Wu, J., Si, H. (2022). Roles of microRNAs in abiotic stress response and characteristics regulation of plant. Front. Plant Sci. 13. doi: 10.3389/fpls.2022.919243
Zhang, H., Zhang, J., Xu, Q., Wang, D., Di, H., Huang, J., et al. (2020). Identification of candidate tolerance genes to low-temperature during maize germination by GWAS and RNA-seq approaches. BMC Plant Biol. 20, 333. doi: 10.1186/s12870-020-02543-9
Zhang, Y., Zhou, Y., Sun, Q., Deng, D., Liu, H., Chen, S., et al. (2019c). Genetic determinants controlling maize rubisco activase gene expression and a comparison with rice counterparts. BMC Plant Biol. 19, 351. doi: 10.1186/s12870-019-1965-x
Zhao, S., Gao, H., Luo, J., Wang, H., Dong, Q., Wang, Y., et al. (2020). Genome-wide analysis of the light-harvesting chlorophyll a/b-binding gene family in apple (Malus domestica) and functional characterization of MdLhcb4.3, which confers tolerance to drought and osmotic stress. Plant Physiol. Biochem. 154, 517–529. doi: 10.1016/j.plaphy.2020.06.022
Zhao, C., Liu, B., Piao, S., Wang, X., Lobell, D. B., Huang, Y., et al. (2017). Temperature increase reduces global yields of major crops in four independent estimates. Proc. Natl. Acad. Sci. U.S.A. 114, 9326–9331. doi: 10.1073/pnas.1701762114
Zhou, X., Muhammad, I., Lan, H., Xia, C. (2022a). Recent advances in the analysis of cold tolerance in maize. Front. Plant Sci. 13. doi: 10.3389/fpls.2022.866034
Zhou, X., Rao, S., Wrightstone, E., Sun, T., Lui, A. C. W., Welsch, R., et al. (2022b). Phytoene synthase: the key rate-limiting enzyme of carotenoid biosynthesis in plants. Front. Plant Sci. 13. doi: 10.3389/fpls.2022.884720
Zhou, R., Sanz-Jimenez, P., Zhu, X.-T., Feng, J.-W., Shao, L., Song, J.-M., et al. (2021). Analysis of rice transcriptome reveals the lncRNA/circRNA regulation in tissue development. Rice 14, 14. doi: 10.1186/s12284-021-00455-2
Zhou, Y., Zhang, H., Zhang, S., Zhang, J., Di, H., Zhang, L., et al. (2023). The G protein-coupled receptor COLD1 promotes chilling tolerance in maize during germination. Int. J. Biol. Macromol 253, 126877. doi: 10.1016/j.ijbiomac.2023.126877
Zhu, G., Liu, Z., Qiao, S., Zhang, Z., Huang, Q., Su, Z., et al. (2022). How could observed sowing dates contribute to maize potential yield under climate change in Northeast China based on APSIM model. Eur. J. Agron. 136, 126511. doi: 10.1016/j.eja.2022.126511
Keywords: maize, whole transcriptome profiling, low temperature stress, climate change, photosynthesis
Citation: Božić M, Ignjatović Micić D, Anđelković V, Delić N and Nikolić A (2025) Maize transcriptome profiling reveals low temperatures affect photosynthesis during the emergence stage. Front. Plant Sci. 16:1527447. doi: 10.3389/fpls.2025.1527447
Received: 13 November 2024; Accepted: 03 January 2025;
Published: 28 January 2025.
Edited by:
Dev Mani Pandey, Birla Institute of Technology, Mesra, IndiaReviewed by:
Klára Kosová, Crop Research Institute (CRI), CzechiaCopyright © 2025 Božić, Ignjatović Micić, Anđelković, Delić and Nikolić. This is an open-access article distributed under the terms of the Creative Commons Attribution License (CC BY). The use, distribution or reproduction in other forums is permitted, provided the original author(s) and the copyright owner(s) are credited and that the original publication in this journal is cited, in accordance with accepted academic practice. No use, distribution or reproduction is permitted which does not comply with these terms.
*Correspondence: Manja Božić, bWJvemljQG1yaXpwLnJz
Disclaimer: All claims expressed in this article are solely those of the authors and do not necessarily represent those of their affiliated organizations, or those of the publisher, the editors and the reviewers. Any product that may be evaluated in this article or claim that may be made by its manufacturer is not guaranteed or endorsed by the publisher.
Research integrity at Frontiers
Learn more about the work of our research integrity team to safeguard the quality of each article we publish.