- 1Departamento de Agronomia, Universidade Estadual de Maringá, Maringá, Paraná, Brazil
- 2Departamento de Agronomia, Universidade Estadual de Londrina, Londrina, Paraná, Brazil
- 3Departamento de Biologia, Universidade Estadual de Londrina, Londrina, Paraná, Brazil
- 4Área de Melhoramento Genético e Propagação Vegetal, Instituto de Desenvolvimento Rural do Paraná, Londrina, Paraná, Brazil
Phosphorus (P) is an essential macronutrient for maize (Zea mays L.) growth and development. Therefore, generating cultivars with upgraded P use efficiency (PUE) represents one of the main strategies to reduce the global agriculture dependence on phosphate fertilizers. In this work, genome-wide association studies (GWAS) were performed to detect quantitative trait nucleotide (QTN) and potential PUE-related candidate genes and associated traits in greenhouse and field trials under contrasting P conditions. The PUE and other agronomy traits of 132 maize inbred lines were assessed in low and normal P supply through the greenhouse and field experiments and Multi-locus GWAS was used to map the associated QTNs. Wide genetic variability was observed among the maize inbred lines under low and normal P supply. In addition, we confirm the complex and quantitative nature of PUE. A total of 306 QTNs were associated with the 24 traits evaluated using different multi-locus GWAS methods. A total of 186 potential candidate genes were identified, mainly involved with transcription regulator, transporter, and transference activity. Further studies are still needed to elucidate the functions and relevance of these genes regarding PUE. Nevertheless, pyramiding the favorable alleles pinpointed in the present study can be considered an efficient strategy for molecular improvement to increase maize PUE.
Introduction
The significant increase in maize yield is mainly attributed to genetic improvement, crop management, and fertilizer use (Byerlee, 2020). On the other hand, the dependence of modern agriculture on mineral fertilizers is alarming. The excessive and indiscriminate use of fertilizers has caused severe environmental problems such as water eutrophication, soil acidification, and air pollution (Seleiman et al., 2021). More than 20 million tons of phosphate fertilizers are applied in agriculture annually, and the global demand will be ~25 million tons by 2050 (Bindraban et al., 2020). Moreover, phosphate rocks are a finite resource, and their global reserves could be depleted in the next 300–400 years (Glaser and Lehr, 2019).
In Brazil, agriculture is extremely dependent on imported phosphate fertilizers, and their use has increased over the years (Withers et al., 2018). Most Brazilian soils are high-phosphorus (P) fixing soils, intensely weathered and rich in (hydro)oxides of iron and aluminum (Rodrigues et al., 2021). Thus, large amounts of phosphate fertilizers are needed to overcome the rapid immobilization of inorganic P (Pi) in these regions. In addition, the expansion of Brazilian agricultural lands by the conversion of degraded pastures or native savannas (Cerrado region) will require considerable amounts of phosphate fertilizers (Withers et al., 2018). In this context, developing cultivars with greater P use efficiency (PUE) represents one of the main strategies to reduce Brazilian agriculture's dependence on phosphate fertilizers and reduce the demand for P input (Pavinato et al., 2020) as well as one step further into sustainability.
Plants have developed several adaptive mechanisms to enhance Pi availability in the soil, along with better uptake, translocation, and use under limiting conditions of this nutrient (Wang et al., 2019a, 2021). For instance, root exudation of phosphatases and organic acids is key in improving soil Pi availability (Wang and Lambers, 2020). Plants can also modify root system architecture to increase Pi uptake under P-limiting conditions (Iqbal et al., 2020). Moreover, high-affinity Pi transporters are abundantly produced under P-deficient conditions to raise Pi root uptake and redistribution within the plant (Wang et al., 2021). Additionally, several alternative metabolic pathways and lipid membrane remodeling play a leading role in redistributing Pi from senescent to developing tissues to allow maximum biomass production (Dissanayaka et al., 2021).
Among the molecular improvement methods, genome-wide association studies (GWAS) have been widely adopted to identify quantitative trait nucleotides (QTN) in various crops worldwide (Li et al., 2019a; Cortes et al., 2021). Because PUE is a highly complex trait, strongly influenced by the environment and controlled by several genes with minor effects (Parentoni et al., 2010; Mendes et al., 2014; Meirelles et al., 2016; Bernardino et al., 2019), the use of multi-locus GWAS methods is better suited to dissect the genetic architecture of this trait in plants (Zhang et al., 2019). Multi-locus GWAS methods rely on a random-SNP-effect model where no multiple correction test is required. There are generally two steps in these models. Initially, a reduced number of molecular markers is selected using different algorithms. These markers are then used in multi-locus models to distinguish true signals (Zhang et al., 2019). Recently, several multi-locus GWAS methods have been developed and have shown increased detection power and accuracy to estimate QTN effects when compared to single-locus GWAS methods (Zhang et al., 2020).
Many quantitative trait loci (QTL) have already been identified in maize for low P tolerance, mainly related to root architecture system and biomass accumulation during early development stages (Zhang et al., 2014; Sahito et al., 2020). On the other hand, few studies were carried out in the field. Among them, studies that quantified PUE and its components are even scarcer, focusing more on grain yield components (Xu et al., 2018) and on root system architecture (Gu et al., 2016; Wang et al., 2019). In this sense, the objectives of the present study were: i) to estimate the genetic variability in tropical inbred maize lines for PUE-related traits under contrasting P conditions, ii) verify possible correlations between the traits evaluated in the greenhouse and field, iii) detect genomic regions associated with PUE-associated traits, and iv) identify potential candidate genes to improve maize PUE.
Materials and methods
Genetic material
A total of 132 tropical maize inbred lines were evaluated, composed of 77 field corn and 55 popcorn lines from the Maize Breeding Program of the State University of Maringá (UEM), Maringá, Brazil. These inbred lines represent part of the genetic variability present in the main hybrids and varieties of field corn and popcorn cultivated in Brazil. Seed samples were obtained from the UEM Germplasm Bank and later multiplied in the 2017-2018 summer season to standardize seed germination. Information on the origin of the maize lines is presented in Supplementary Table 1.
Greenhouse trials
Inbred lines were evaluated under controlled conditions in a greenhouse at the Agronomy Department of the State University of Londrina (UEL) in Londrina, Paraná, Brazil (23°17'34”S, 51°10'24”W, and 550 m altitude) in September 2019. Two independent hydroponic systems were developed for low (2.5 μM) and normal P (250 μM) conditions using Magnavaca nutrient solution (Magnavaca et al., 1987). Each hydroponic system consisted of eight 28-L polyethylene boxes (58 × 40 × 16.5 cm). The hydroponic boxes were interconnected and connected to a 300-L reservoir, that is, the same nutrient solution ran throughout the entire system. The pH of the nutrient solution was maintained at 5.8 ± 0.2 and permanently aerated by a pressurizing water pump (Model Pl400P 40 MCA, Lorenzetti Ltda, Campinas, Brazil).
Seeds were disinfested by immersion in 95% ethanol solution (v/v) for 30 s followed by immersion in 5% H2O2 solution (v/v) for 10 min and six washes with sterile deionized water. The seeds were then placed on filter paper (GermtestTM, 24 × 33 × 0.02 cm) (Cienlab Ltda., Campinas, Brazil), moistened with distilled water, and placed in a growth chamber at a temperature of 27 ± 2°C and 70% relative humidity. Seven days after sowing (at development stage V1), uniform seedlings were selected and transplanted to hydroponic systems after removing the endosperm to eliminate seed reserves. The experiment was carried out using a completely randomized design with three repetitions. After 12 days of growth in nutrient solution (stage V2), the inbred lines root system was digitized in a 600 dpi image using an Epson L3110 scanner (Seiko Epson Corp., California, USA), and the root architecture traits were assessed using the GiaRoots software (Galkovskyi et al., 2012).
Field trials
Inbred lines were evaluated at the School Farm of UEL (23°17'34”S, 51°10'24”W, and 550 m altitude) during the 2018–2019 summer and 2019 fall/winter seasons. This region has a humid subtropical climate and soil classified as Dystroferric Red Latosol. Two distinct areas were selected to conduct the experiments under low and normal P conditions, carried out in the same areas during the two seasons. P (Mehlich 1) contents in the areas ranged from 4.87–5.11 mg dm3 (low P) to 17.32–19.32 mg dm3 (normal P). The soil physicochemical analyses and other traits of the experimental areas are presented in Supplementary Table 2.
The experiment was set in a randomized complete block design with three repetitions. Plots consisted of a 4 m long line, 0.45 m spacing between lines, and 0.20 m between plants. Two levels of phosphate fertilization were used in each environment, totaling four independent experiments. Before planting, normal P environments were fertilized with 120 kg P2O5 ha–1, 40 kg K2O ha–1, and 40 kg N ha–1, while low P environments only received 40 kg K2O ha–1 and 40 kg N ha–1. The topdressing nitrogen (N) fertilization was carried out using 180 kg N ha–1 applied at stage V6, while the other cultural practices were performed according to crop demand. At physiological maturation (stage R6), three uniform and representative plants from each plot were collected for phenotypic evaluations.
Phenotyping under greenhouse and field conditions
The detailed description of the 24 traits evaluated in the greenhouse and field experiments is presented in Table 1. To determine the P content in plant tissues, samples were oven-dried at 60°C for 72 h and milled in Willey-type knife mill MA340 (Piracicaba, São Paulo, Brazil). Then 0.1 g aliquots were digested in nitroperchloric solution (HNO3:HClO4) according to Malavolta et al. (1989). P content was determined by the molybdenum blue spectrophotometric method (Pradhan and Pokhrel, 2013), reading the samples in an Agilent 8453 spectrophotometer (Agilent Technologies, California, USA) at 660 nm.
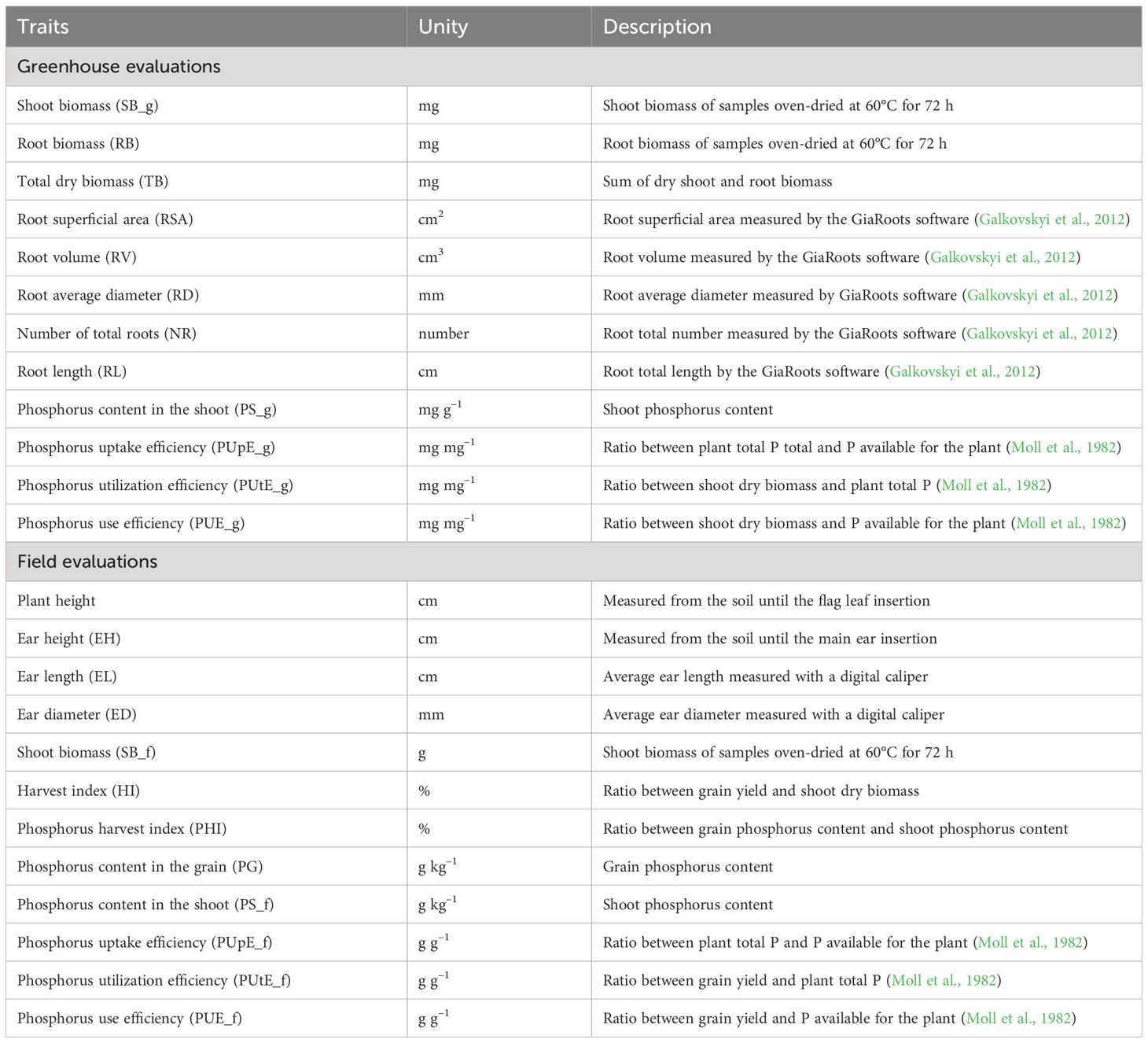
Table 1. Description of the 24 traits evaluated in 132 tropical corn lines under greenhouse and field conditions.
Deviance analysis and genetic parameters
Data were analyzed using the best linear unbiased predictor (BLUP) and restricted maximum likelihood (REML) methods by the software Selegen–REML/BLUP (Resende, 2016) and R version 3.6.0 (https://www.r-project.org) via the 'lme4' package. The deviance analyses (ANADEV) of the traits obtained from the greenhouse and field trials were carried out using the following mathematical models, respectively:
Where µ is the overall mean, Gi is the random effect of the i-th genotype, Pm is the fixed effect of the m-th level of P, GPim is the random effect of the genotype × P level interaction, and ϵim ~ N(0, σ²) is the random effect of the error associated with each experimental unit.
Where µ is the overall mean, Gi is the random effect of the i-th genotype, Bj/k/m is the random effect of the j-th block within the k-th season and within the m-th P level, Sk is the fixed effect of the k-th season, Pm is the fixed effect of the m-th P level, GSik is the random effect of the genotype × season interaction, GPim is the random effect of the genotype × P level interaction, SPkm is the fixed effect of the yield × P level interaction, GSPikm is the random effect of the genotype × yield × P level interaction, and ϵijkn ~ N(0, σ²) is the random effect of the error associated with each experimental unit.
The significance of the ANADEV random effects was verified by the likelihood ratio test (Resende, 2016). The heritability in the broad sense (h2) of the traits evaluated in the greenhouse and field were estimated, respectively, using the following formulas: and , where is the genotypic variance, is the variance of the genotype × season interaction, is the residual variance, r is the number of repetitions in each season, and s is the number of seasons. The selective accuracy (Ac) was obtained as follows: , where PEV is the variance of the prediction error of the genotypic values, and is the genotypic variance.
Correlations and principal component analysis
Correlations between traits and principal component analyses (PCA) were performed using BLUP means. The significance of the estimates was verified using the t-test at a 5% probability level (α = 0.05). The correlation estimates were visualized using the correlation network approach. These analyses were performed by the 'qgraph' and 'ggbiplot' packages of the R software version 3.6.0.
Genotyping-by-sequencing
Genomic DNA was initially isolated from leaf tissues of inbred lines, as established by Coan et al. (2018). DNA samples were then sent to the Genomic Diversity Institute at Cornell University for genotyping-by-sequencing (GBS) according to the protocol described by Elshire et al. (2011). Sequencing was performed in the Illumina HiSeq 2000 sequencer (Illumina Inc., San Diego, USA). The sequences were deposited in the European Variation Archive (EVA) (GCF_902167145.1).
Sequencing data were analyzed using the Tassel 5.0 GBS v2 software (Glaubitz et al., 2014). The sequences obtained were aligned to the reference genome of Zea mays L. version AGPV3 (B73 RefGen v3) obtained from the MaizeGDB database (https://www.maizegdb.org). SNP markers quality control was performed using the VCFtools software version 0.1.15 (Danecek et al., 2011), removing the SNPs through the following criteria: i) non-biallelic, ii) minor allele frequency (MAF) less than 5%, iii) inbreeding coefficient less than 90%, and iv) missing data greater than 90%. Heterozygous SNPs were treated as missing data since the evaluated lines are highly inbred and, thus, these SNPs may come from sequencing errors. Missing data were imputed with the hidden Markov model (HMM) using the Beagle software version 5.0 (Browning et al., 2018). After filtering, a total of 273,775 high-quality SNPs were retained for subsequent analyses (Supplementary Figure 1).
Population structure and kinship matrix
The population genetic structure was inferred using the Bayesian clustering model through the software Structure version 2.3.4 (Pritchard et al., 2000) based on the method described by Evanno et al. (2005). One hundred thousand MCMC (Monte Carlo Markov chain) iterations, a burn-in period of 10,000 iterations, an admixture model, and correlated allelic frequencies were used. Subgroup values (ΔK) between one and ten were tested, with ten independent interactions for each K value. The ideal number of K was determined using the Structure Harvester software version 0.6.92 (Earl, 2012).
PCA was performed using the Tassel software version 5.2.48 (Bradbury et al., 2007). Additionally, the Euclidean distance between the inbred lines was calculated, and the UPGMA (unweighted pair group method using arithmetic averages) hierarchical grouping was performed. These analyses were performed using the R software version 3.6.0 via the packages 'SNPRelate', 'factoextra', and 'ggplot2'. The kinship matrix was calculated based on the centralized identity-by-state (IBS) method (Endelman and Jannink, 2012) using the Tassel software version 5.2.48 (Bradbury et al., 2007).
Linkage disequilibrium analysis
Linkage disequilibrium (LD) analysis was performed using the 'LDcorSV' package of the R software version 3.6.0. LD was estimated on all chromosomes simultaneously and individually. In addition to the conventional measure of r2, corrected r2 was estimated by: i) population structure (r2Q), ii) kinship matrix (r2K), and iii) population structure plus kinship matrix (r2QK) (Mangin et al., 2012). The results of the Bayesian clustering and IBS method were used to correct the population structure and kinship matrix, respectively, as presented above. LD was adjusted using the nonlinear regression method proposed by Hill and Weir (1988) using the nls function of the R software version 3.6.0. The LD decay was defined by the distance at which half of the maximum LD decayed (LD half decay). This estimate indicates the initial slope of the LD decay, and it was considered the most consistent in the comparative study conducted by Vos et al. (2017).
Multi-locus GWAS
For multi-locus GWAS analyses, least square means (lsmeans) estimated from the lsmeans function of PROC GLM in SAS software version 9.0 (SAS Institute, Cary, USA) were used. Multi-locus GWAS analyses were performed using eight datasets: i) 2018-2019 summer season at low P (LP_18), ii) 2018-2019 summer season under normal P (NP_18), iii) 2019 fall/winter season in low P (LP_19), iv) 2019 fall/winter season in high P (NP_19), v) combination of 2018–2019 and 2019 seasons in low P (LP_C), vi) combination of 2018–2019 and 2019 seasons under normal P (NP_C), vii) greenhouse at low P (LP_G), and vii) greenhouse in normal P (NP_G).
Five multi-locus GWAS methods implemented in the ‘mrMLM.GUI’ package (Zhang et al., 2020) of the R software version 3.6.0 were used: i) mrMLM (Wang et al., 2016), ii) FASTmrMLM (Tamba and Zhang, 2018), iii) FASTmrEMMA (Wen et al., 2018), iv) ISIS EM-BLASSO (Tamba et al., 2017), and v) pLARmEB (Zhang et al., 2017). The population structure (Q) and kinship matrix (K) were included in the tested models to minimize the identification of false-positive associations and increase the statistical analysis power. Critical values for significant associations were LOD (logarithm of odds) ≥ 3 (or P = 0.0002) for all methods. To obtain more accurate results, only the QTNs detected by at least three different methods were considered truly significant and, later, used in the search for candidate genes.
Favorable alleles
The QTNs detected had their favorable alleles identified, that is, the favorable alleles that cause positive effects on the traits. Then, a heatmap based on Ward's method and Euclidean distance was performed to group the inbred maize lines into clusters with different amounts of favorable alleles. In addition, box plots were made to verify if the pyramiding of these favorable alleles would result in higher PUE and its components. These analyses were performed using R software version 3.6.0. through the 'pheatmap' and 'ggplot2' packages.
Candidate genes
Candidate genes were selected based on the reference genome of Zea mays L. version AGPV3 (B73 RefGen v3) obtained from the MaizeGDB database (https://www.maizegdb.org). The search radius of candidate genes was established based on the results obtained from the LD half decay. The classical genes or those with known functions in maize were annotated using the MaizeGDB database. Additionally, the molecular functions of all candidate genes were annotated according to the Gene Ontology (GO) database (http://www.geneontology.org/).
Results
Deviance analysis and genetic parameters
The deviance analyses showed a significant effect (P ≤ 0.01) of genotype and genotype–P level interaction for all traits evaluated in the greenhouse trials (Table 2). Low P reduced all characteristics, except for PUtE_g, PUpE_g, and PUE_g. The reductions in these traits ranged from –4.8 (RD) to –65.0% (PS_g), while a high increase was observed for PUtE_g (161.9%), PUpE_g (169.6%), and PUE_g (678.9%) (Supplementary Figure 2). The estimates of h2 ranged from 0.55 (RV) to 0.74 (RD and RSA) at low P, while under normal P conditions, the estimates varied from 0.63 (RV) to 0.86 (RD). Ac values ranged from 0.74 (RV) to 0.86 (RD and RSA) and 0.86 (RV and SB_g) to 0.92 (RD and RSA) under low and normal P conditions, respectively.
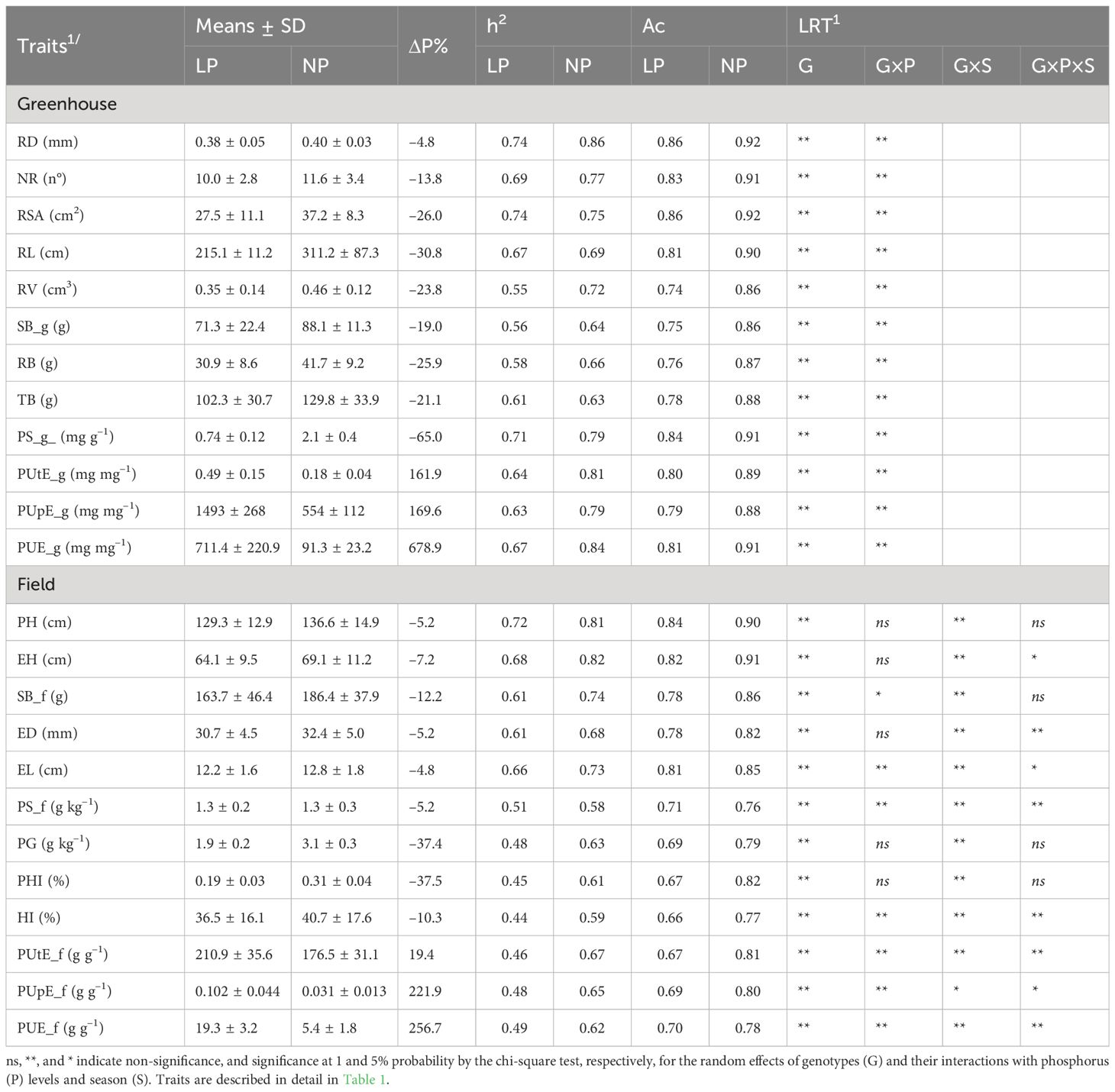
Table 2. Mean, standard deviation (SD), broad-sense heritability (h2), selective accuracy (Ac), and likelihood ratio test (LRT) for 24 traits evaluated under normal (NP) and low phosphorus (LP) conditions obtained in the greenhouse and field.
In the field trials, the deviance analyses presented significant triple interaction (P ≤ 0.05) between genotype–season–P level for EH, ED, EL, PS_f, HI, PUtE_f, and PUE_f. For genotype–P level interaction, significant effects (P ≤ 0.05) were observed for SB_f, EL, PS_f, HI, PUtE_f, PUpE_f, and PUE_f. All traits assessed showed significant effects of genotype (P ≤ 0.01) and genotype-season interaction (P ≤ 0.05). Low P led to reduced values for all evaluated traits, except PUtE_f, PUpE_f, and PUE_f. The reductions in trait values ranged from –4.8 (EL) to –37.5% (PHI), while PUtE_f (19.4%), PUpE_f (221.9%), and PUE_f (256.7%) increased greatly. The estimates of h2 ranged from 0.44 (HI) to 0.72 (PH) under low P and from 0.52 (PS_f) to 0.82 (EH) under normal P conditions. Ac values ranged from 0.66 (HI) to 0.84 (PH) and 0.76 (PS_f) to 0.91 (EH) under low and normal P conditions, respectively.
Correlation and principal component analysis
The correlations among traits in the greenhouse and field are shown in Figure 1. In general, there was a greater correlation between the traits within than between the field and greenhouse trials. However, few differences were observed between low and normal P conditions, mainly due to the magnitudes of the correlation estimates rather than their direction. Strong positive correlations were observed between greenhouse traits under both P conditions, primarily root system-related traits, PUtE_g, and PUE_g. Under field conditions, high and positive correlation estimates were found for PH × EH, PUE_f × HI, PUE_f × PUpE_f, PUE_f × SB_f, PG × PHI, and PUpE_f × SB_f. Conversely, negative correlations were reported for PS_g × PUpE_g in the greenhouse under low and normal P conditions.
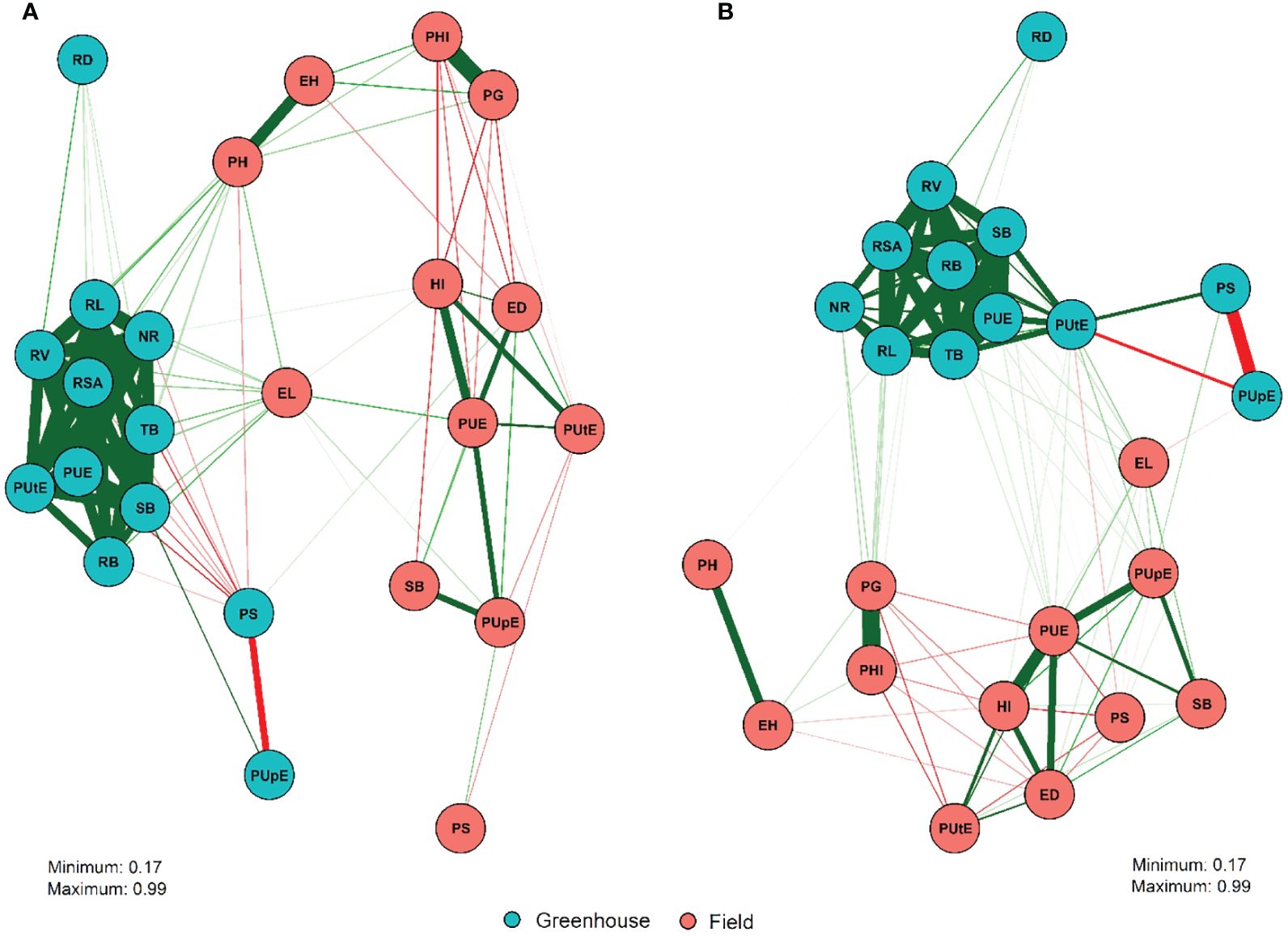
Figure 1. Correlation network among 24 traits evaluated in 132 inbred tropical maize lines under low (A) and normal P (B) conditions obtained in field and greenhouse trials. A detailed description of the characteristics is presented in Table 1. Correlation network between 24 traits evaluated in 132 inbred lines of tropical maize under low (A) and normal P (B) conditions obtained in field and greenhouse trials. Respectfully, the green and red lines represent Pearson estimates positive and negative linear correlations and the thickness of the lines is proportional to the magnitude of the correlation.
PCA of low versus normal P, low P, and normal P conditions are shown in Figure 2. Together, the first two principal components (PC1 and PC2) explained 65.2% (low P), 70.5% (normal P), and 72.9% (low versus normal P) of the total existing variation. There was a clear distinction between low versus normal P, with PUE_g, PUE_f, PUtE_g, PUtE_f, PUpE_g, and PUpE_f vectors associated with low P, while the others were associated with normal P (Figure 2A). The field corn and popcorn lines showed distinct collective behavior under both P conditions. At low P, the field corn lines were associated with ED, SB_f, PUtE_f, PUpE_f, and PUE_f vectors, whereas the popcorn lines were mainly linked to the PH, EH, PG, and PHI vectors (Figure 2B). Under normal P, the field corn lines were associated with the ED, HI, SB_f, PUtE_f, PUpE_f, and PUE_f vectors, while the popcorn lines were primarily linked with the PH, EH, PG, PHI, and PS_f vectors (Figure 2C).
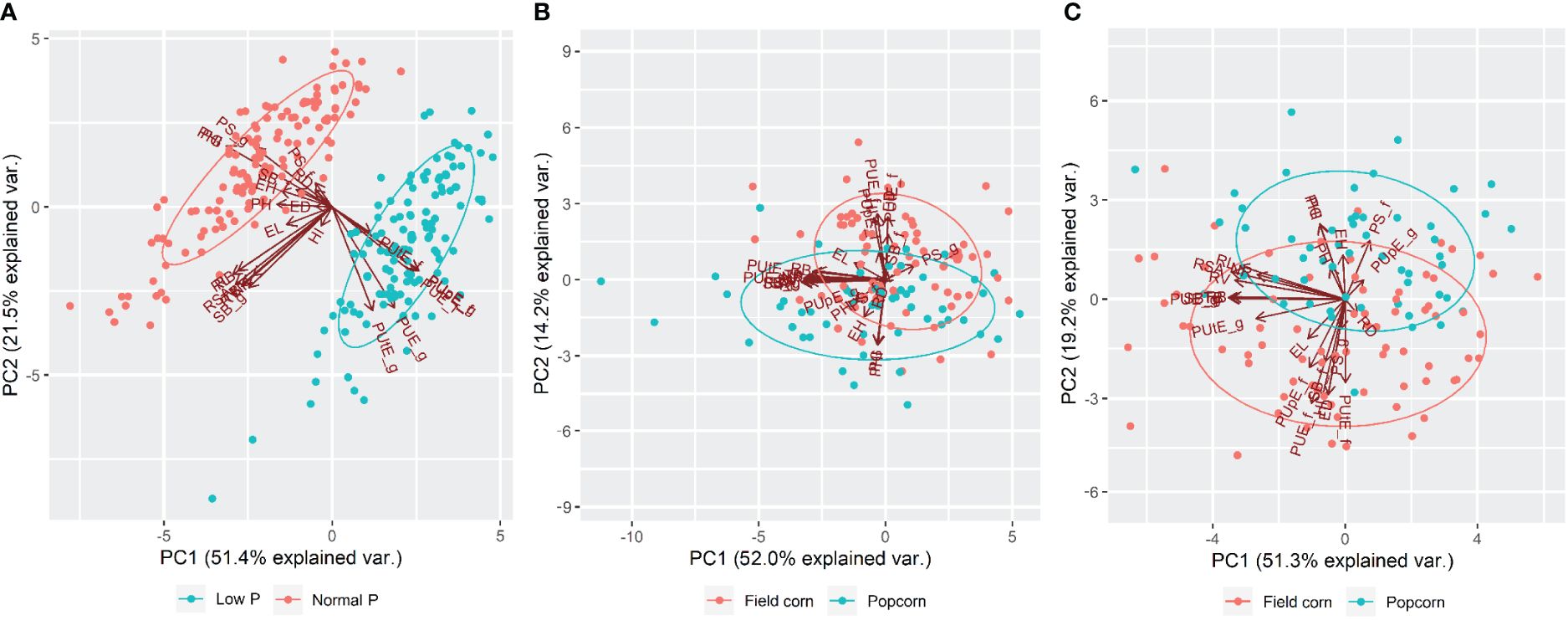
Figure 2. Principal component analysis (PCA) of 132 inbred tropical maize lines evaluated for 24 traits in the greenhouse and field under low versus normal P (A), low P (B), and normal P (C) conditions. A detailed description of the characteristics can be found in Table 1.
Population structure
Based on the PCA, the first two components explained 19.27 and 2.54% of the total existing variation, respectively, and it is possible to observe a distinction between field corn and popcorn lines (Figure 3A). Similar results were found by the UPGMA and Bayesian groupings (Figures 3B, C). Bayesian analysis classified seven popcorn lines as admixture, with an ancestry coefficient lower than 0.6 for each subpopulation.
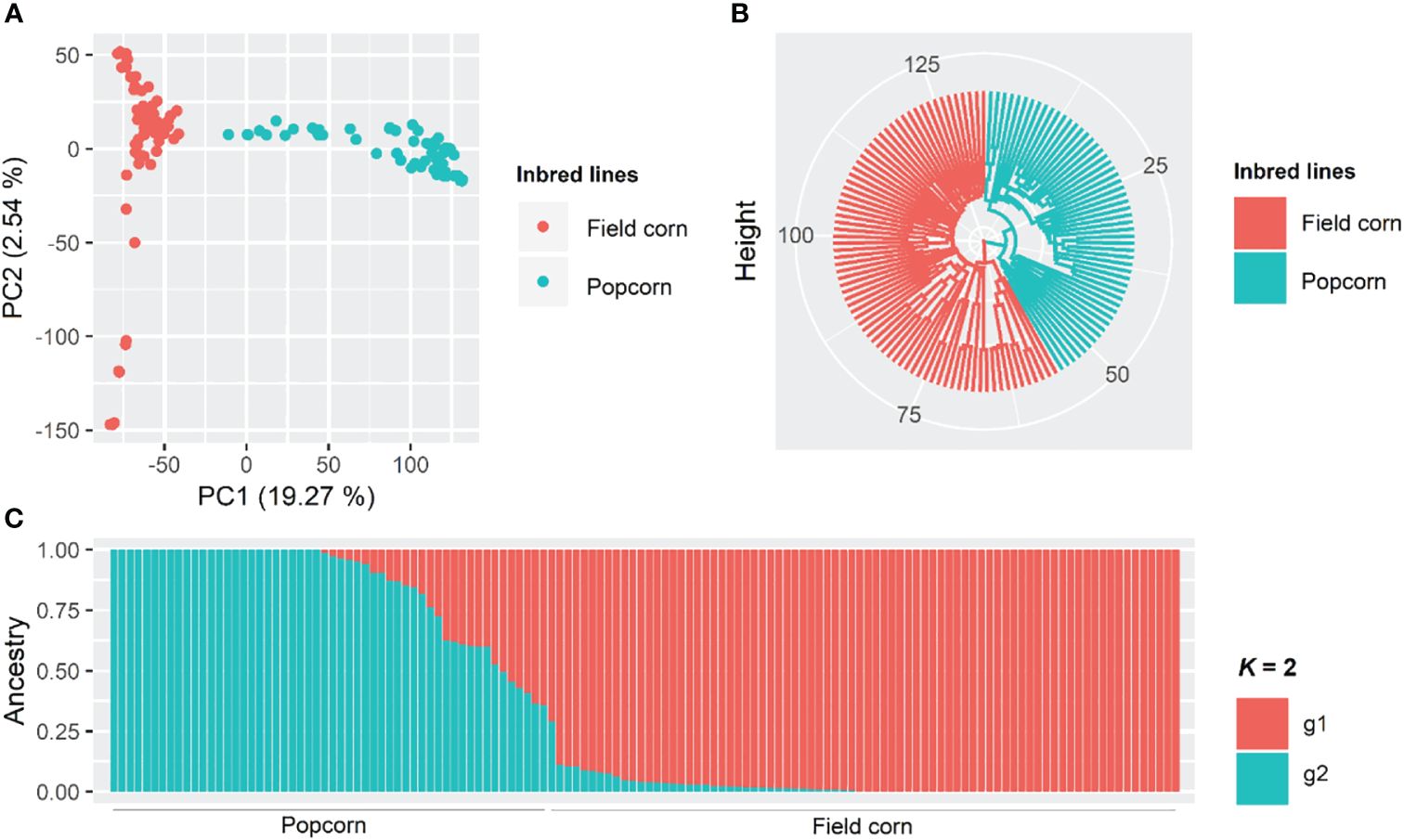
Figure 3. Principal component analysis (A), dendrogram obtained by the UPGMA method (unweighted pair group method using arithmetic averages) through the Euclidean distance (B), and Bayesian clustering considering K = 2 (C) among 132 tropical maize lines using 273,775 SNP (single nucleotide polymorphism) markers.
Linkage disequilibrium analysis
LD showed rapid decay and exhibited variations within each chromosome (Supplementary Figure 3). Considering all chromosomes, the differences between uncorrected r2 (r2 = 0.16; LD half decay = 1.41 kb) and corrected population structure (r2K = 0.15; LD half decay = 1.27 kb) were small. On the other hand, r2 was affected by the corrections of the kinship matrix (r2Q = 0.07; LD half decay = 0.89 kb) and kinship matrix plus population structure (r2KQ = 0.07; LD half decay = 0.89 kb), presenting similar results (Supplementary Figure 4). Regarding individual chromosomes, Chr07 and Chr05 showed the fastest and slowest decay, respectively. From the results obtained from the LD half decay, the search distance for candidate genes was defined as ± 1 kb.
QTNs identified and method performance
The histograms of the lsmeans used to identify the QTNs are shown in Supplementary Figures 5, 6. A total of 5838 QTNs was discovered by the five different multi-locus GWAS methods evaluated (Supplementary Figure 7). The pLARmEB method (n = 1710) identified the highest number of QTNs, followed by the FASTmrMLM (n = 1604), ISIS EM-BLASSO (n = 1224), mrMLM (n = 756), and FASTmrEMMA (n = 544) methods. Among these QTNs, 810 were co-detected by two methods, 255 by three, 44 by four, and seven by five. On the other hand, 3242 QTNs were found by only one of the evaluated methods. Thus, only 255 QTNs were considered highly reliable, as they were co-detected by at least three methods and, consequently, were maintained in the present study (Supplementary Table 3).
The FASTmrMLM method was the most efficient (95.4%), as 292 of the 306 QTNs were detected by this method, followed by pLARmEB (94.1%), ISIS EM-BLASSO (85.0%), FASTmrEMMA (29.1%), and mrMLM (19.9%). The 306 QTNs discovered are distributed in the ten chromosomes (Chr) of maize, ranging from 15 (Chr08) to 60 QTNs (Chr01) (Supplementary Figure 8A). A greater number of QTNs was detected in the field trials (n = 227) compared to greenhouse trials (n = 79) (Supplementary Figure 8B). In the greenhouse, 35 and 44 QTNs were found under low and normal P conditions, respectively (Supplementary Figure 8C), whereas 118 (low P) and 107 (normal P) QTNs were detected in the field trials (Supplementary Figure 8D).
The 79 QTNs identified in the greenhouse were associated with RV (n = 3), PUpE (n = 4), PUE (n = 5), SB (n = 5), NR (n = 6), RD (n = 6), RL (n = 6), PS (n = 7), TB (n = 7), RSA (n = 8), PUtE (n = 9), and RB (n = 13) (Supplementary Table 3). The phenotypic variation explained (PVE) by these QTNs ranged from 0.01 to 16.31%. In turn, the 227 QTNs identified in the field were associated with PUE (n = 13), EL (n = 14), ED (n = 16), PG (n = 18), PH (n = 18), EH (n = 19), HI (n = 19), SB (n = 19), PUtE (n = 20), PUpE (n = 22), PS (n = 24), and PHI (n = 25) (Supplementary Table 3). The PVE by these QTNs varied from 0.01 to 18.62%.
A total of 16 pleiotropic QTNs were detected in the greenhouse (n = 10) and field (n = 6) trials (Table 3). In the greenhouse experiment under low P, pleiotropic QTNs were associated with SB–TB (S2_170934541), PUtE–RL (S4_198262446), and RL–RSA (S5_97898187 and S9_11783675). Furthermore, a pleiotropic QTN (S1_46130565) was detected in three characteristics (RSA, NR, and RL). Under normal P conditions, pleiotropic QTNs associated with PUtE–PS (S1_1159069), RB–TB (S1_151079747), RSA–TB (S3_225847941), and RB–RSA (S5_212662145) were revealed. Moreover, a highly pleiotropic QTN (S10_119011145) was found in four traits simultaneously (PUE, RB, SB, and TB). In the field trials under low P, pleiotropic QTNs were associated with PUE–PUpE (S3_190210784) and HI–PUE (S8_148017105). Under normal P conditions, pleiotropic QTNs were identified in PG–PH (S1_259083391), HI–PUE (S5_10594899), and ED–PUE (S7_7624808).
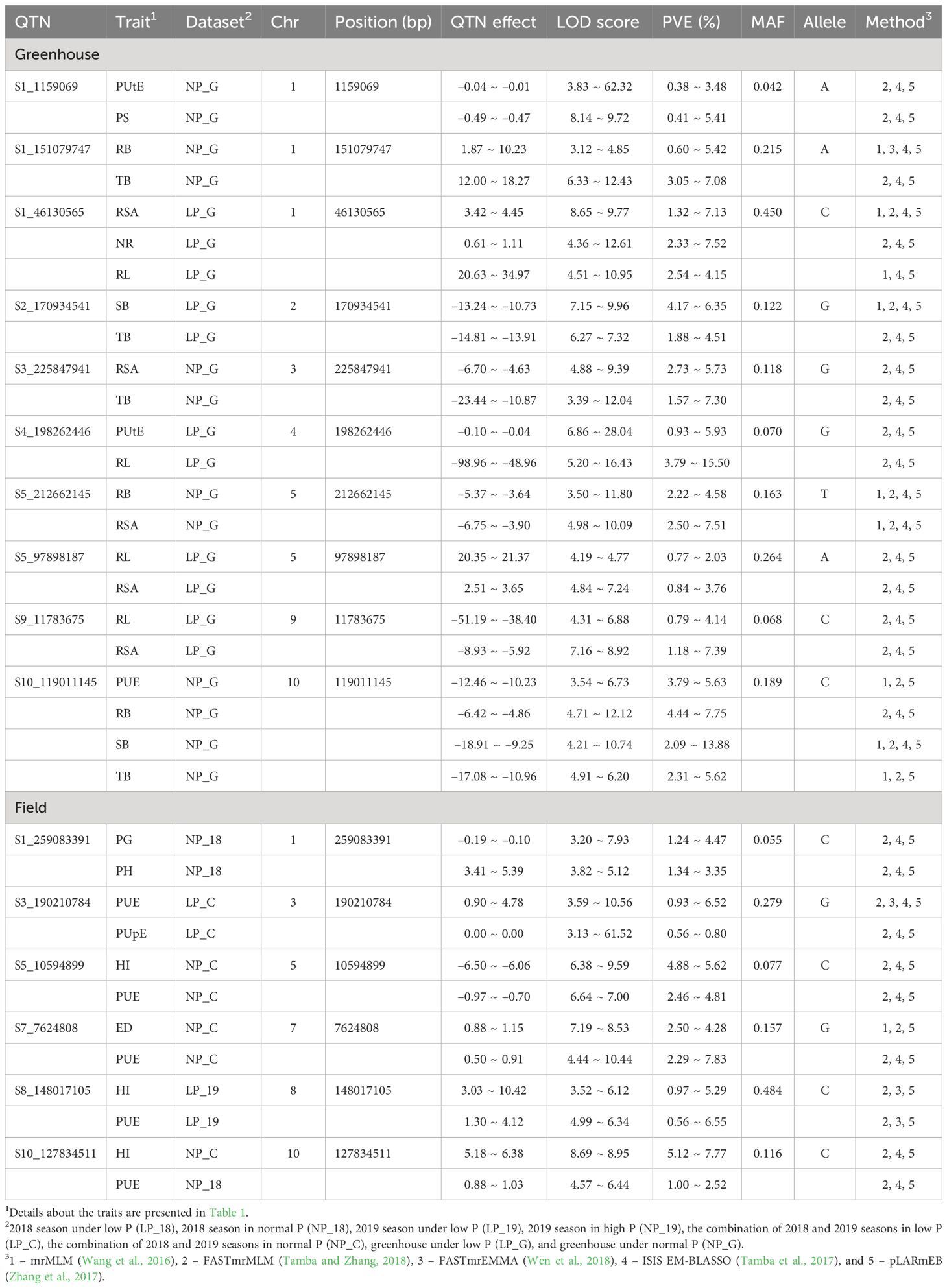
Table 3. Pleiotropic QTNs identified in traits evaluated in greenhouse and field trials under low and normal P conditions.
Favorable alleles
The heatmap grouped the inbred maize lines into three distinct groups regarding favorable allele number (Supplementary Figure 9). Group 1 was composed of 41 lines, characterized by having the smallest number of favorable alleles in the field experiments, mainly under low P conditions. Groups 2 and 3 were formed by 36 and 55 lines, respectively. In general, the lines in group 2 had the highest numbers of favorable alleles in the greenhouse and field trials in both P conditions. On the other hand, group 3 lines had the lowest numbers of favorable alleles, mainly in the greenhouse experiments. In general, there was no distinction between popcorn and field corn lines concerning the accumulation of favorable alleles.
Considering only the favorable alleles related to PUE and its components, a gradual increase in these traits was observed by pyramiding these favorable alleles (Figure 4). Popcorn lines 17-P9-1-6 and 162-P1780 had the highest and lowest number of favorable alleles considering all traits, respectively (Figure 5). The 17-P9-1-6 line, considered P-efficient, presented 58.33% (field in normal P), 57.14% (field in low P), 62.16% (greenhouse in normal P), and 58.27% (greenhouse under low P) of the favorable alleles. On the other hand, line 162P1780, considered P-inefficient, presented only 32.18% (field in normal P), 31.45% (field in low P), 27.02% (greenhouse in normal P), and 24.13% (greenhouse under low P) of the favorable alleles.
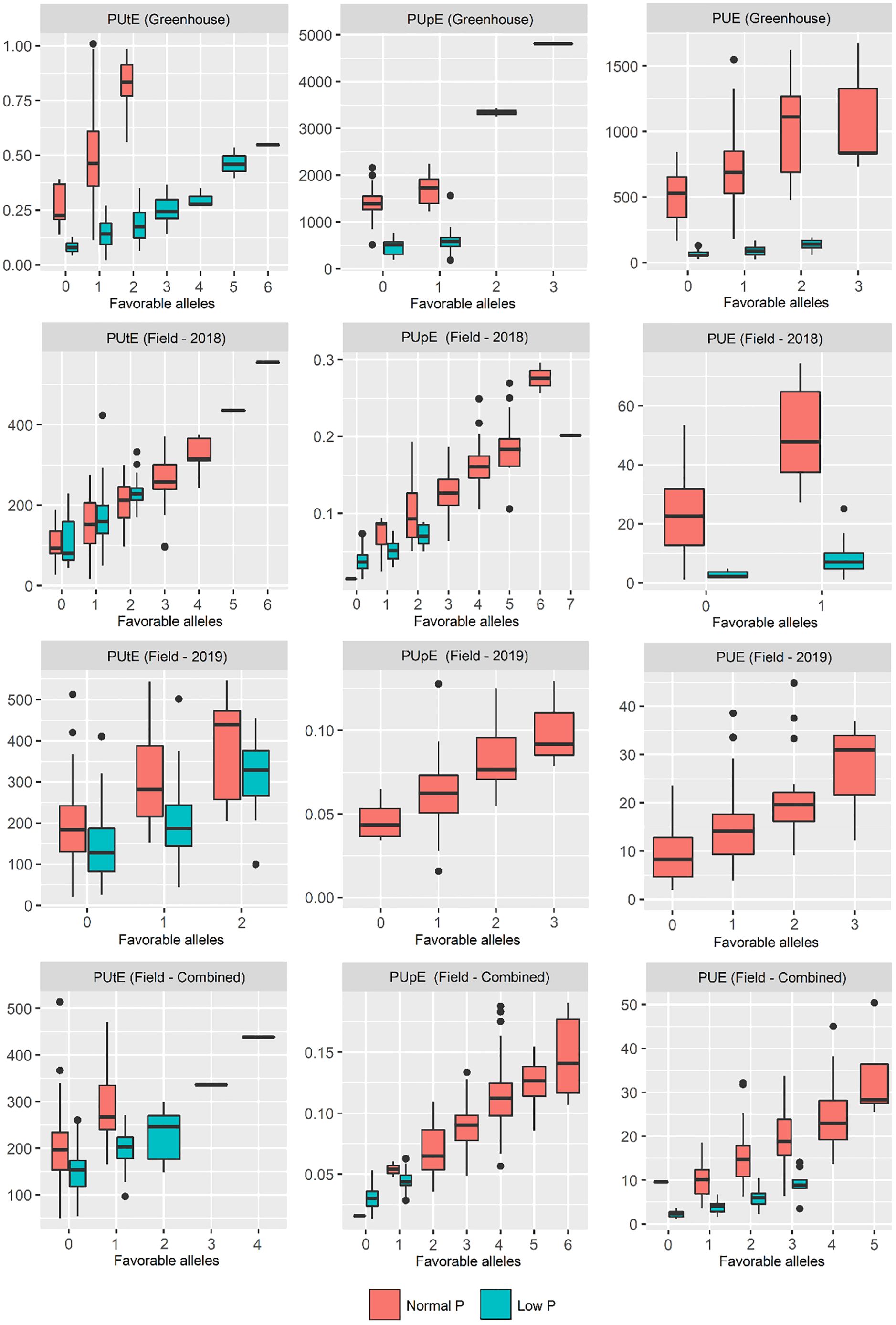
Figure 4. Pyramidization of favorable alleles in inbred maize lines evaluated for traits related to phosphorus utilization efficiency (PUtE), phosphorus uptake efficiency (PUpE), and phosphorus use efficiency (PUE).
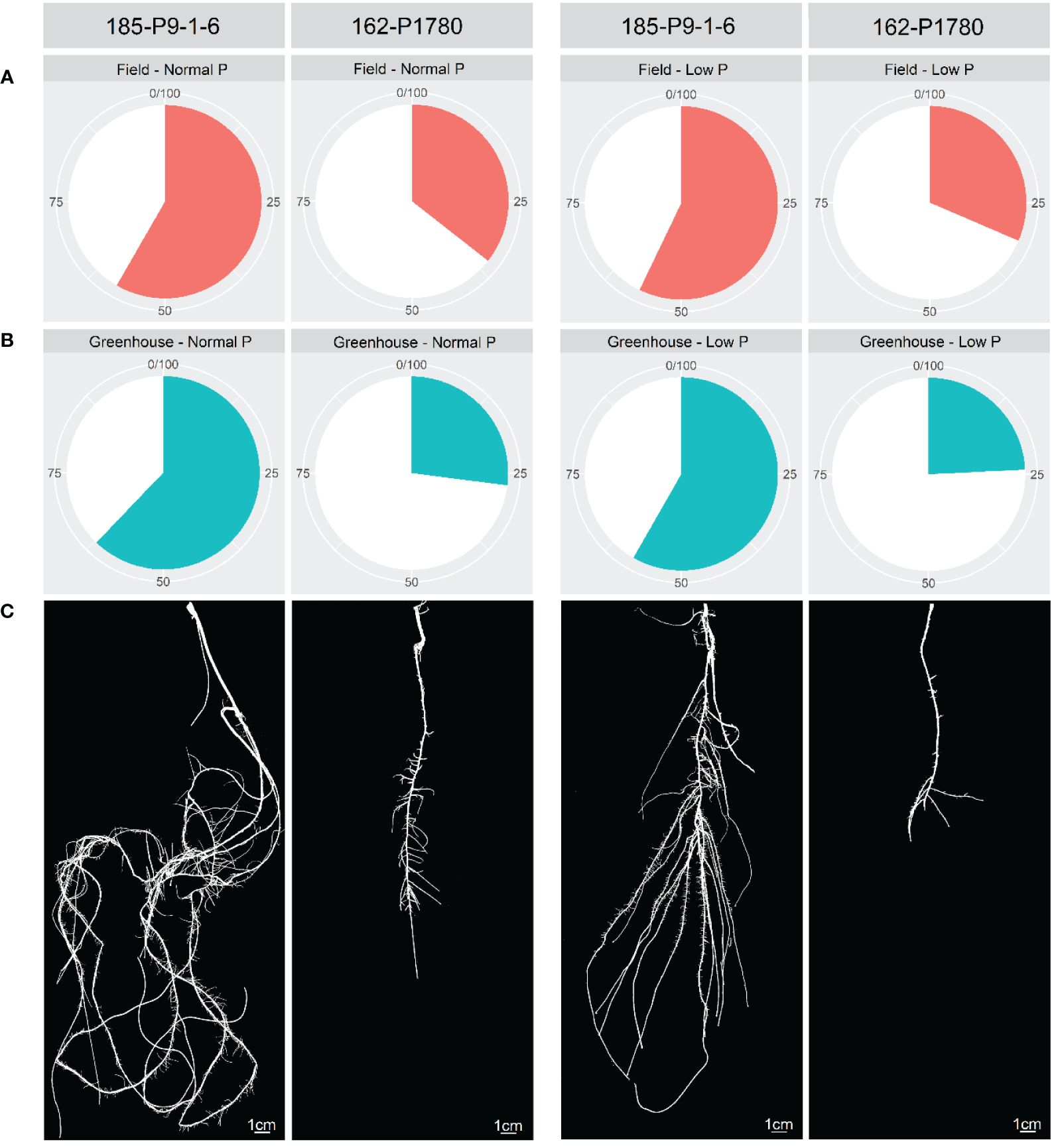
Figure 5. Percentage of favorable alleles of two contrasting maize inbred lines for P-efficiency in the field (A) and greenhouse (B) under low and normal P conditions. The root system of the 185-P9-1-6 and 162-P1788 inbred lines under low and normal P conditions (C).
Candidate genes
A total of 186 potential candidate genes distributed in all maize chromosomes were identified (Supplementary File 1). Among them, 45 have already been annotated as classical or known genes in the maize genome, 21 of which have functions related to transcription factor activity, while the others are mainly associated with transport and transferase activity. According to the GO annotation, the primary molecular functions of the candidate genes included transcription factor, transferase, hydrolase, catalytic binding, ATP binding, DNA binding, and nucleotide-binding activity. In addition, several molecular functions directly related to P molecules were also identified, such as activities of phosphotransferase, phosphatase, pyrophosphatase, calcium-dependent phospholipid binding, all-trans-nonaprenyl-diphosphate synthase, glucose-6-phosphate isomerase, phosphorelay sensor kinase, phosphorylative mechanism, phospholipid binding, and phosphoenolpyruvate carboxylase.
Discussion
Genotype-environment interaction and genetic parameters
Genotype–season–P level interaction was observed in most of the traits evaluated in the field, indicating that the differential behavior of genotypes depends on the combination of P levels and seasons studied. Additionally, there were more significant interactions between genotype–season compared to genotype–P level interactions, suggesting that season strongly influences the evaluated traits. In the greenhouse trials, there was a significant genotype-P level interaction for all characteristics, indicating that the differential behavior of genotypes also depends on the P level in early evaluations. The genotype–P levels interaction in maize has already been reported in several greenhouse and field studies, which confirms that studies related to PUE and its components should be carried out under specific P conditions (Xu et al., 2018; Sahito et al., 2020; Li et al., 2021).
The negative influence of low P on the characteristics evaluated in the greenhouse and field was already expected since P is an essential element for plant development, constituting key cell molecules such as ATP, nucleic acids, and phospholipids, in addition to acting in the central regulation of many metabolisms, including energy transfer, protein activation, and carbon and amino acids metabolic processes (Wang et al., 2021). In contrast, the lines showed higher PUE, PutE, and PUpE under conditions of low P availability. In general, plants present higher PUE under low P since, under high phosphate fertilization, part of this element is lost in erosive processes and/or made unavailable by adsorption and immobilization processes in the soil (Alewell et al., 2020).
Although PUE-related traits are complex and strongly influenced by the environment, the heritability and selective accuracy estimates obtained in the present study fell into moderate to high, indicating favorable conditions for improvement aimed at increasing PUE. Heritability is the central parameter of any breeding program, used to estimate selection response and explain the proportion of phenotypic variation due to genetics (Hallauer et al., 2010). In the present study, heritability estimates were higher under normal P than low P conditions. In general, stress conditions tend to reduce heritability estimates, mainly due to the increased environmental influence on plant phenotype (Vats, 2018). Xu et al. (2018), evaluating 11 PUE-associated traits in maize, also observed reductions in heritability estimates under low P compared to normal P conditions.
Correlation between traits
The existence of correlations among traits evaluated in the greenhouse and field trials would enable the early selection of genotypes with higher PUE. Unfortunately, however, no relevant correlations were observed between the traits evaluated in both trials, indicating that early selection in hydroponic systems may not be efficient in selecting plants with higher PUE under field conditions. Similar results were reported in other studies, suggesting that different genes and morphophysiological mechanisms act at different stages of plant development (Dissanayaka et al., 2018; Wang et al., 2019a). In fact, experiments in hydroponic systems or pots using nutrient solutions may present conditions that are very different from real field conditions since the environmental influence is much greater in the field than under greenhouse-controlled conditions (Araus et al., 2018).
P is relatively immobile in the soil, and its availability is greater at surface soil horizons, decreasing substantially at deeper soil horizons (Menezes-Blackburn et al., 2018). Thus, several studies have reported the importance of the maize root architecture system for higher PUE under field and greenhouse conditions (Jia et al., 2018; Klamer et al., 2019). The present study corroborated these results, showing a high correlation between the root-system-related traits (RB, RSA, RV, NR, and RL) with PUE in both P conditions under a hydroponic system. The indirect selection of PUE through root system traits is highly relevant in breeding programs, representing a faster and money-saving alternative, as it does not require laboratory analysis for P quantification. Further, high-yield phenotyping methods are already well established to characterize the root system of plants (Tracy et al., 2020).
Linkage disequilibrium
Linkage disequilibrium (LD) is the non-random association of alleles at two or more loci and plays a central role in association mapping analyses (Romay et al., 2013). In the present study, we observed a rapid LD decay. In addition, we also report differences in LD decay in relation to chromosomes and correction methods assessed, with LD decay being mainly affected by population structure. Several studies have reported rapid LD decay in maize, ranging primarily from 0.1 to 10 kb (Yan et al., 2009; Romay et al., 2013; Bennetzen et al., 2018; Coan et al., 2018). In addition to the different approaches to estimating LD and LD decay, LD estimates may vary depending on the genomic region and germplasm evaluated (Vos et al., 2017). In maize, tropical and subtropical germplasms have faster LD decay when compared to temperate germplasms, as they have greater genetic diversity and the presence of rare alleles (Yan et al., 2009; Romay et al., 2013).
Multi-locus GWAS methods
Multi-locus GWAS methods have been recently used to investigate the genetic basis of essential traits in several agricultural crops, such as maize (Lu et al., 2021), rice (Cui et al., 2018), bread wheat (Yang et al., 2020), soybean (Zhang et al., 2018), barley (Hu et al., 2018), and upland cotton (Su et al., 2018). In the present study, five different multi-locus GWAS methods were assessed. Among them, the FASTmrMLM and pLARmEB were the most efficient methods, as they detected more than 94% of the reliable QTNs in the present study, that is, QTNs co-detected by three or more methods. Although these methods present a combined two-step approach, the quantitative and qualitative differences in the identified QTNs are due to the different algorithms used in each method (Zhang et al., 2020). Thus, Zhang et al. (2019) suggested using combined results between the different methods of multi-locus GWAS to obtain more accurate results.
QTNs identified
The present study identified a large number of small effects QTNs, confirming the complex and quantitative nature of PUE in maize. Using small-effect QTNs associated with traits of interest represents a smart strategy in genomic selection (GS) approaches since the use of these QTNs alone can replace the need for high-density genotyping through random SNPs, thus reducing genotyping costs (Lan et al., 2020). Furthermore, GS models using only SNPs known to be associated with traits of interest showed greater prediction accuracy, as they had lower background noise (Ali et al., 2020). In addition to their use in GS, these QTNs may be promising for application in breeding programs aimed at pyramiding favorable alleles through marker-assisted selection (MAS) (Boopathi, 2020).
In a QTLome study for low P tolerance in maize under limiting phosphorous conditions, Zhang et al. (2014) identified 23 meta-QTLs, that is, genomic regions more likely to harbor candidate genes responsible for traits related to tolerance to low P and/or PUE. Among the 306 QTNs discovered in the present study, 20 are located within the ranges of 11 meta-QTLs. In another analysis of meta-QTLs, Guo et al. (2018) identified 53 meta-QTLs associated with the maize root architecture system in the presence and absence of abiotic stresses (water deficit, high temperature, and P and N deficiency). Among the 54 QTNs found in the present study to be associated with root traits, 10 are located within the ranges of eight meta-QTLs. Thereby, the location of QTNs in genomic regions known to be associated with traits and characteristics of interest reinforces the significance and relevance of the QTNs pinpointed in the present study.
Candidate genes
Among the 186 candidate genes detected in the present study, 45 have already been annotated as classical or known genes in maize. For instance, the GRMZM2G083841 gene (associated with PUpE in LP_G) codes for a phosphoenolpyruvate carboxylase, catalyzing the carboxylation of phosphoenolpyruvate to produce oxaloacetate and Pi, performing the primary fixation of atmospheric CO2 in C4 plants (Nimmo, 2003). The GRMZM2G140614 gene (associated with PUE in NP_C) encodes a glucose-6-phosphate isomerase, an essential enzyme in the oxidative pathway of pentose phosphate responsible for producing energy-rich cofactors and generation of carbon skeletons required for biosynthetic reactions (Yu et al., 2000). Interestingly, GRMZM2G307119 (associated with RV in NP_G) was initially related to the formation of spikelet meristems (Chuck and Hake, 2005). However, Jansen et al. (2013) revealed that this gene plays a fundamental role in developing lateral roots in maize. In turn, the GRMZM2G177792 gene (associated with PG in LP_C) has a peroxidase activity and has been associated with resistance to biotic stresses (Santiago et al., 2016; Musungu et al., 2020). In addition to these genes, three main mechanisms and pathways were discovered:
1. Transcription regulator activity. Transcription factors regulate gene expression. Previous studies have reported that many of these factors trigger P stress response and/or PUE in maize (Calderon-Vazquez et al., 2008; Xu et al., 2018). Of the 45 classical or known genes identified in the present study, 21 (~46%) are classified as transcription factors. The GRMZM2G317160 gene (associated with PS in LP_19) is a member of the AP2-EREBP family of transcription factors and plays a significant role in abiotic stress response (Du et al., 2014). The GRMZM2G479760 gene (associated with HI and PUE in NP_C) belongs to the basic leucine zipper (bZIP) family. It regulates several phenomena during maize growth and development and participates in responses to abiotic stresses and hormonal signaling (Cao et al., 2019). GRMZM5G808366 (associated with HI in LP_18) belongs to the auxin response factor (ARF) gene family and has an elevated expression level under P stress conditions (Pei et al., 2013). In turn, the GRMZM2G073823 gene (associated with RB in NP_G) is already known as an important gene during maize root growth and development (Jiang et al., 2012; Li et al., 2019b).
2. Transporter activity. Several specific and non-specific nutrient transporters have been reported in response to P deficiency stress (Shabala et al., 2016; Xu et al., 2018; Wang et al., 2021). GRMZM2G161459 (associated with PUtE at NP_18) encodes the transport peptide PTR2 that transports a broad spectrum of dipeptides and is involved in several pathways (Li et al., 2016a). GRMZM2G064467 (associated with PUE in LP_C) is involved in magnesium transmembrane transporter activity and responds to abiotic stresses (Li et al., 2016b). GRMZM2G455557 (associated with PHI in NP_C) encodes a plasma membrane H+-ATPase, creating electrochemical gradients for soil nutrient uptake by roots and is involved in additional solute xylem loading (Falhof et al., 2016). This gene has already been associated with N assimilation in maize (Plett et al., 2016), while other genes related to plasma membrane H+-ATPase have been identified in response to P deficiency (Yuan et al., 2017; Stein et al., 2019).
3. Transferase activity. Transferases are enzymes that transfer functional groups from one molecule to another, catalyzing several reactions involving nutrient absorption, translocation, and storage. They also respond to abiotic stresses. Among the genes identified in this study, GRMZM2G104511 (associated with PUE in LP_18) encodes a protein from the O-fucosyltransferase family, physically interacting with proteins involved in cell division and responses to stress and hormones (Jia et al., 2013). This gene was differentially expressed in maize under water deficit (Zheng et al., 2020) and P conditions (Du et al., 2016). The GRMZM5G851405 gene (associated with PHI in NP_18) encodes a histone acetyltransferase whose function is to catalyze the acetylation of central histones by adding an acetyl group to the lysine residue in the terminal tail of histones. The regulation of histone acetyltransferases has already been associated with the phosphate starvation response in Arabidopsis thaliana (Wang et al., 2019b). As for GRMZM2G033767 (associated with PUtE in NP_G), it codes for a glycerol-3-phosphate acyltransferase, an enzyme that catalyzes an acyl group from an acyl donor to the sn-1 position of glycerol 3-phosphate and has great importance in regulating lipid biosynthesis (Murata and Tasaka, 1997). Finally, the GRMZM2G141810 gene (associated with PS in LP_19) encodes a tryptophan aminotransferase and is importantly related to nutrient storage functions in maize endosperm (Bernardi et al., 2012; Zhan et al., 2018).
Conclusion
Wide genetic variability was observed for PUE, and we confirmed its complex nature. On the other hand, we did not verify relevant correlations between traits evaluated in the greenhouse and field, indicating that early screening may not be efficient in selecting genotypes with higher PUE under field conditions. A total of 306 QTNs were associated with the 24 evaluated traits in the present study using different multi-locus GWAS models. From these QTNs, 186 potential candidates were identified, mainly involved with transcription regulators, transporters, and transference activities. Our study provides new insights into PUE genetic architecture and may serve as a basis for further functional investigation. In addition, the QTNs detected in this study can be used for pyramiding favorable alleles to develop maize varieties with higher PUE and, consequently, less dependent on phosphate fertilization.
Data availability statement
The data presented in the study are deposited in the at European Variation Archive (EVA) repository, accession number PRJEB72805.
Author contributions
DZ: Methodology, Investigation, Formal analysis, Writing – review & editing, Writing – original draft, Conceptualization. LP: Writing – review & editing, Methodology, Investigation. RA: Writing – review & editing, Methodology, Investigation. JD: Writing – review & editing, Methodology, Investigation, Formal analysis. AM: Writing – review & editing, Methodology, Investigation. AK: Writing – review & editing, Methodology, Investigation. VB: Writing – review & editing, Methodology, Investigation. LC: Writing – review & editing, Methodology, Investigation. RU: Writing – review & editing, Investigation, Formal analysis. AN: Writing – review & editing, Methodology, Investigation. VM-C: Writing – review & editing, Methodology, Investigation, Conceptualization. CS: Writing – review & editing, Writing – original draft, Methodology, Investigation, Funding acquisition. LG: Writing – review & editing, Writing – original draft, Validation, Supervision, Project administration, Funding acquisition, Conceptualization.
Funding
The author(s) declare financial support was received for the research, authorship, and/or publication of this article. This work was supported by National Council for Scientific and Technological Development - CNPq and Coordination of Superior Level Staff Improvement - CAPES. DZ, LP, AM, AK, LC, AN, and JD have received research support from Coordination of Superior Level Staff Improvement - CAPES for postgraduate fellowship funding. VB has received research support from CNPq for postdoctoral fellowship funding (152260/2018-8).
Conflict of interest
The authors declare that the research was conducted in the absence of any commercial or financial relationships that could be construed as a potential conflict of interest.
Publisher’s note
All claims expressed in this article are solely those of the authors and do not necessarily represent those of their affiliated organizations, or those of the publisher, the editors and the reviewers. Any product that may be evaluated in this article, or claim that may be made by its manufacturer, is not guaranteed or endorsed by the publisher.
Supplementary material
The Supplementary Material for this article can be found online at: https://www.frontiersin.org/articles/10.3389/fpls.2024.1366173/full#supplementary-material
References
Alewell, C., Ringeval, B., Ballabio, C., Robinson, D. A., Panagos, P., Borrelli, P. (2020). Global phosphorus shortage will be aggravated by soil erosion. Nat. Commun. 11, 4546. doi: 10.1038/s41467-020-18326-7
Ali, M., Zhang, Y., Rasheed, A., Wang, J., Zhang, L. (2020). Genomic prediction for grain yield and yield-related traits in chinese winter wheat. Int. J. Mol. Sci. 21, 1342. doi: 10.3390/ijms21041342
Araus, J. L., Kefauver, S. C., Zaman-Allah, M., Olsen, M. S., Cairns, J. E. (2018). Translating high-throughput phenotyping into genetic gain. Trends Plant Sci. 23, 451–466. doi: 10.1016/j.tplants.2018.02.001
Bennetzen, J., Flint-Garcia, S., Hirsch, C., Tuberosa, R. (Eds.) (2018). The maize genome (New York: Springer International Publishing). doi: 10.1007/978-3-319-97427-9
Bernardi, J., Lanubile, A., Li, Q. B., Kumar, D., Kladnik, A., Cook, S. D., et al. (2012). Impaired auxin biosynthesis in the defective endosperm18 mutant is due to mutational loss of expression in the ZmYuc1 gene encoding endosperm-specific YUCCA1 protein in maize. Plant Physiol. 160, 1318–1328. doi: 10.1104/pp.112.204743
Bernardino, K. C., Pastina, M. M., Menezes, C. B., Souza, S. M., Maciel, L. S., Jr, Carvalho, G., et al. (2019). The genetic architecture of phosphorus efficiency in sorghum involves pleiotropic QTL for root morphology and grain yield under low phosphorus availability in the soil. BMC Plant Biol. 19, 87. doi: 10.1186/s12870-019-1689-y
Bindraban, P. S., Dimkpa, C. O., Pandey, R. (2020). Exploring phosphorus fertilizers and fertilization strategies for improved human and environmental health. Biol. Fertil Soils 56, 299–317. doi: 10.1007/s00374-019-01430-2
Boopathi, N. M. (2020). “Marker-assisted selection (MAS),” in Genetic mapping and marker assisted selection (Springer, Singapore), 343–388. doi: 10.1007/978-981-15-2949-8_9
Bradbury, P. J., Zhang, Z., Kroon, D. E., Casstevens, T. M., Ramdoss, Y., Buckler, E. S. (2007). TASSEL: software for association mapping of complex traits in diverse samples. Bioinformatics 23, 2633–2635. doi: 10.1093/bioinformatics/btm308
Browning, B. L., Zhou, Y., Browning., S. R. (2018). A one-penny imputed genome from next-generation reference panels. Am. J. Hum. Genet. 103, 338–348. doi: 10.1016/j.ajhg.2018.07.015
Byerlee, D. (2020). The globalization of hybrid maize 1921–70. J. Glob Hist 15, 101–122. doi: 10.1017/S1740022819000354
Calderon-Vazquez, C., Ibarra-Laclette, E., Caballero-Perez, J., Herrera-Estrella, L. (2008). Transcript profiling of Zea mays roots reveals gene responses to phosphate deficiency at the plant-and species-specific levels. J. Exp. Bot. 59, 2479–2497. doi: 10.1093/jxb/ern115
Cao, L., Lu, X., Zhang, P., Wang, G., Wei, L., Wang, T. (2019). Systematic analysis of differentially expressed maize ZmbZIP genes between drought and rewatering transcriptome reveals bZIP family members involved in abiotic stress responses. Int. J. Mol. Sci. 20, 4103. doi: 10.3390/ijms20174103
Chuck, G., Hake, S. (2005). Regulation of developmental transitions. Curr. Opin. Plant Biol. 8, 67–70. doi: 10.1016/j.pbi.2004.11.002
Coan, M. M., Senhorinho, H. J., Pinto, R. J., Scapim, C. A., Tessmann, D. J., Williams, W. P., et al. (2018). Genome-wide association study of resistance to ear rot by Fusarium verticillioides in a tropical field maize and popcorn core collection. Crop Sci. 58, 564–578. doi: 10.2135/cropsci2017.05.0322
Cortes, L. T., Zhang, Z., Yu, J. (2021). Status and prospects of genome-wide association studies in plants. Plant Genome 14, e20077. doi: 10.1002/tpg2.20077
Cui, Y., Zhang, F., Zhou, Y. (2018). The application of multi-locus GWAS for the detection of salt-tolerance loci in rice. Front. Plant Sci. 9. doi: 10.3389/fpls.2018.01464
Danecek, P., Auton, A., Abecasis, G., Albers, C. A., Banks, E., DePristo, M. A., et al. (2011). The variant call format and VCFtools. Bioinformatics 27, 2156–2158. doi: 10.1093/bioinformatics/btr330
Dissanayaka, D. M. S. B., Ghahremani, M., Siebers, M., Wasaki, J., Plaxton, W. C. (2021). Recent insights into the metabolic adaptations of phosphorus-deprived plants. J. Exp. Bot. 72, 199–223. doi: 10.1093/jxb/eraa482
Dissanayaka, D. M. S. B., Plaxton, W. C., Lambers, H., Siebers, M., Marambe, B., Wasaki, J. (2018). Molecular mechanisms underpinning phosphorus-use efficiency in rice. Plant Cell Environ. 41, 1483–1496. doi: 10.1111/pce.13191
Du, H., Huang, M., Zhang, Z., Cheng, S. (2014). Genome-wide analysis of the AP2/ERF gene family in maize waterlogging stress response. Euphytica 198, 115–126. doi: 10.1007/s10681-014-1088-2
Du, Q., Wang, K., Xu, C., Zou, C., Xie, C., Xu, Y., et al. (2016). Strand-specific RNA-Seq transcriptome analysis of genotypes with and without low-phosphorus tolerance provides novel insights into phosphorus-use efficiency in maize. BMC Plant Biol. 16, 222. doi: 10.1186/s12870-016-0903-4
Earl, D. A. (2012). STRUCTURE HARVESTER: a website and program for visualizing STRUCTURE output and implementing the Evanno method. Conserv. Genet. Resour 4, 359–361. doi: 10.1007/s12686-011-9548-7
Elshire, R. J., Glaubitz, J. C., Sun, Q., Poland, J. A., Kawamoto, K., Buckler, E. S., et al. (2011). A robust, simple genotyping-by-sequencing (GBS) approach for high diversity species. PloS One 6, e19379. doi: 10.1371/journal.pone.0019379
Endelman, J. B., Jannink, J. L. (2012). Shrinkage estimation of the realized relationship matrix. G3 (Bethesda) 2, 1405–1413. doi: 10.1534/g3.112.004259
Evanno, G., Regnaut, S., Goudet, J. (2005). Detecting the number of clusters of individuals using the software STRUCTURE: a simulation study. Mol. Ecol. 14, 2611–2620. doi: 10.1111/j.1365-294X.2005.02553.x
Falhof, J., Pedersen, J. T., Fuglsang, A. T., Palmgren, M. (2016). Plasma membrane H+-ATPase regulation in the center of plant physiology. Mol. Plant 9, 323–337. doi: 10.1016/j.molp.2015.11.002
Galkovskyi, T., Mileyko, Y., Bucksch, A., Moore, B., Symonova, O., Price, C. A., et al. (2012). GiA Roots: software for the high throughput analysis of plant root system architecture. BMC Plant Biol. 12, 116. doi: 10.1186/1471-2229-12-116
Glaser, B., Lehr, V. I. (2019). Biochar effects on phosphorus availability in agricultural soils: A meta-analysis. Sci. Rep. 9, 1–9. doi: 10.1038/s41598-019-45693-z
Glaubitz, J. C., Casstevens, T. M., Lu, F., Harriman, J., Elshire, R. J., Sun, Q., et al. (2014). TASSEL-GBS: a high capacity genotyping by sequencing analysis pipeline. PloS One 9, e90346. doi: 10.1371/journal.pone.0090346
Gu, R., Chen, F., Long, L., Cai, H., Liu, Z., Yang, J., et al. (2016). Enhancing phosphorus uptake efficieny through QTL-based selection for root system architecture in maize. J. Genet. Genomics 43, 663–672. doi: 10.1016/j.jgg.2016.11.002
Guo, J., Chen, L., Li, Y., Shi, Y., Song, Y., Zhang, D., et al. (2018). Meta-QTL analysis and identification of candidate genes related to root traits in maize. Euphytica 214, 223. doi: 10.1007/s10681-018-2283-3
Hallauer, A. R., Carena, M. J., Miranda Filho, J. D. (2010). Quantitative genetics in maize breeding (vol. 6) (New York: Springer Science & Business Media). doi: 10.1007/978-1-4419-0766-0
Hill, W. G., Weir, B. S. (1988). Variances and covariances of squared linkage disequilibria in finite populations. Theor. Popul Biol. 33, 54–78. doi: 10.1016/0040-5809(88)90004-4
Hu, X., Zuo, J., Wang, J., Liu, L., Sun, G., Li, C., et al. (2018). Multi-locus genome-wide association studies for 14 main agronomic traits in barley. Front. Plant Sci. 9. doi: 10.3389/fpls.2018.01683
Iqbal, S., Akhtar, J., Naz, T., Riaz, U., Hussain, S., Mazhar, Z., et al. (2020). Root morphological adjustments of crops to improve nutrient use efficiency in limited environments. Commun. Soil Sci. Plant Anal. 51, 2452–2465. doi: 10.1080/00103624.2020.1836199
Jansen, L., Hollunder, J., Roberts, I., Forestan, C., Fonteyne, P., Van Quickenborne, C., et al. (2013). Comparative transcriptomics as a tool for the identification of root branching genes in maize. Plant Biotechnol. J. 11, 1092–1102. doi: 10.1111/pbi.12104
Jia, F., Wu, B., Li, H., Huang, J., Zheng, C. (2013). Genome-wide identification and characterisation of F-box family in maize. Mol. Genet. Genom 288, 559–577. doi: 10.1007/s00438-013-0769-1
Jia, X., Liu, P., Lynch, J. P. (2018). Greater lateral root branching density in maize improves phosphorus acquisition from low phosphorus soil. J. Exp. Bot. 69, 4961–4970. doi: 10.1093/jxb/ery252
Jiang, Y., Zeng, B., Zhao, H., Zhang, M., Xie, S., Lai, J. (2012). Genome-wide transcription factor gene prediction and their expressional tissue-specificities in maize. J. Integr. Plant Biol. 54, 616–630. doi: 10.1111/j.1744-7909.2012.01149.x
Klamer, F., Vogel, F., Li, X., Bremer, H., Neumann, G., Neuhäuser, B., et al. (2019). Estimating the importance of maize root hairs in low phosphorus conditions and under drought. Ann. Bot. 124, 961–968. doi: 10.1093/aob/mcz011
Lan, S., Zheng, C., Hauck, K., McCausland, M., Duguid, S. D., Booker, H. M., et al. (2020). Genomic prediction accuracy of seven breeding selection traits improved by QTL identification in flax. Int. J. Mol. Sci. 21, 1577. doi: 10.3390/ijms21051577
Li, D., Chen, Z., Wang, M., Leiser, W. L., Weiß, T. M., Zhao, Z., et al. (2021). Dissecting the phenotypic response of maize to low phosphorus soils by field screening of a large diversity panel. Euphytica 217, 1–12. doi: 10.1007/s10681-020-02727-2
Li, D., Wang, M., Kuang, X., Liu, W. (2019a). Genetic study and molecular breeding for high phosphorus use efficiency in maize. Front. Agric. Sci. Eng. 6, 366–379. doi: 10.15302/J-FASE-2019278
Li, H., Du, H., Huang, K., Chen, X., Liu, T., Gao, S., et al. (2016b). Identification, and functional and expression analyses of the CorA/MRS2/MGT-type magnesium transporter family in maize. Plant Cell Physiol. 57, 1153–1168. doi: 10.1093/pcp/pcw064
Li, X., Zhou, Z., Ding, J., Wu, Y., Zhou, B., Wang, R., et al. (2016a). Combined linkage and association mapping reveals QTL and candidate genes for plant and ear height in maize. Front. Plant Sci. 7. doi: 10.3389/fpls.2016.00833
Li, Y., Liu, X., Chen, R., Tian, J., Fan, Y., Zhou, X. (2019b). Genome-scale mining of root-preferential genes from maize and characterization of their promoter activity. BMC Plant Biol. 19, 1–12. doi: 10.1186/s12870-019-2198-8
Lu, X., Wang, J., Wang, Y., Wen, W., Zhang, Y., Du, J., et al. (2021). Genome-wide association study of maize aboveground dry matter accumulation at seedling stage. Front. Genet. 11. doi: 10.3389/fgene.2020.571236
Magnavaca, R., Gardner, C. O., Clark, R. B. (1987). “Evaluation of inbred maize lines for aluminum tolerance in nutrient solution,” in Genetic aspects of plant mineral nutrition (Springer, Dordrecht), 255–265). doi: 10.1007/978-94-009-3581-5_23
Malavolta, E., Vitti, G. C., de Oliveira, S. A. (1989). Evaluation of the nutritional state of plants: principles and applications. (Piracicaba: Associação Brasileira para Pesquisa de Potássio e Fósforo).
Mangin, B., Siberchicot, A., Nicolas, S., Doligez, A., This, P., Cierco-Ayrolles, C. (2012). Novel measures of linkage disequilibrium that correct the bias due to population structure and relatedness. Heredity 108, 285–291. doi: 10.1038/hdy.2011.73
Meirelles, W. F., Parentoni, S. N., Guimarães, L. J. M., Guimarães, P. E. O., Pacheco, C. A. P., de Oliveira, A. C., et al. (2016). Diallel anlysis of maize lines as to their phosphorus responsiveness and use efficiency. Pesq Agropec Bras. 51, 224–232. doi: 10.1590/S0100-204X2016000300004
Mendes, F. F., Guimarães, L. J. M., Souza, J. C., Guimarães, P. E. O., Magalhaes, J. V., Garcia, A. A. F., et al. (2014). Genetic architecture of phosphorus use efficiency in tropical maize cultivated in a low-P soil. Crop Sci. 54, 1530–1538. doi: 10.2135/cropsci2013.11.0755
Menezes-Blackburn, D., Giles, C., Darch, T., George, T. S., Blackwell, M., Stutter, M., et al. (2018). Opportunities for mobilizing recalcitrant phosphorus from agricultural soils: a review. Plant Soil 427, 5–16. doi: 10.1007/s11104-017-3362-2
Moll, R. H., Kamprath, E. J., Jackson, W. A. (1982). Analysis and interpretation of factors which contribute to efficiency of nitrogen utilization. Agron. J. 74, 562–564. doi: 10.2134/agronj1982.00021962007400030037x
Murata, N., Tasaka, Y. (1997). Glycerol-3-phosphate acyltransferase in plants. Biochim. Biophys. Acta Lipids Lipid Metab. 1348, 10–16. doi: 10.1016/S0005-2760(97)00115-X
Musungu, B., Bhatnagar, D., Quiniou, S., Brown, R. L., Payne, G. A., O’Brian, G., et al. (2020). Use of Dual RNA-seq for Systems Biology Analysis of Zea mays and Aspergillus flavus interaction. Front. Microbiol. 11. doi: 10.3389/fmicb.2020.00853
Nimmo, H. G. (2003). Control of the phosphorylation of phosphoenolpyruvate carboxylase in higher plants. Arch. Biochem. Biophys. 414, 189–196. doi: 10.1016/S0003-9861(03)00115-2
Parentoni, S. N., Souza Junior, C. L., Alves, V. M. C., Gama, E. E. G., Coelho, A. M., et al. (2010). Inheritance and breeding strategies for phosphorus efficiency in tropical maize (Zea mays L.). Maydica 55, 1–15.
Pavinato, P. S., Cherubin, M. R., Soltangheisi, A., Rocha, G. C., Chadwick, D. R., Jones, D. L. (2020). Revealing soil legacy phosphorus to promote sustainable agriculture in Brazil. Sci. Rep. 10, 1–11. doi: 10.1038/s41598-020-72302-1
Pei, L., Jin, Z., Li, K., Yin, H., Wang, J., Yang, A. (2013). Identification and comparative analysis of low phosphate tolerance-associated microRNAs in two maize genotypes. Plant Physiol. Biochem. 70, 221–234. doi: 10.1016/j.plaphy.2013.05.043
Plett, D., Holtham, L., Baumann, U., Kalashyan, E., Francis, K., Enju, A., et al. (2016). Nitrogen assimilation system in maize is regulated by developmental and tissue-specific mechanisms. Plant Mol. Biol. 92, 293–312. doi: 10.1007/s11103-016-0512-5
Pradhan, S., Pokhrel, M. R. (2013). Spectrophotometric determination of phosphate in sugarcane juice, fertilizer, detergent and water samples by molybdenum blue method. Sci. World 11, 58–62.
Pritchard, J. K., Stephens, M., Donnelly, P. (2000). Inference of population structure using multilocus genotype data. Genetics 155, 945–959. doi: 10.1093/genetics/155.2.945
Resende, M. D. V. D. (2016). Software Selegen-REML/BLUP: a useful tool for plant breeding. Crop Breed Appl. Biotechnol. 16, 330–339. doi: 10.1590/1984-70332016v16n4a49
Rodrigues, M., Withers, P. J. A., Soltangheisi, A., Vargas, V., Holzschuh, M., Pavinato, P. S. (2021). Tillage systems and cover crops affecting soil phosphorus bioavailability in Brazilian Cerrado Oxisols. Soil Tillage Res. 205, 104770. doi: 10.1016/j.still.2020.104770
Romay, M. C., Millard, M. J., Glaubitz, J. C., Peiffer, J. A., Swarts, K. L., Casstevens, T. M., et al. (2013). Comprehensive genotyping of the USA national maize inbred seed bank. Genome Biol. 14, 1–18. doi: 10.1186/gb-2013-14-6-r55
Sahito, J. H., Zheng, F., Tang, H., He, X., Luo, B., Zhang, X., et al. (2020). Identification, association, and expression analysis of ZmNAC134 gene response to phosphorus deficiency tolerance traits in maize at seedling stage. Euphytica 216, 100. doi: 10.1007/s10681-020-02634-6
Santiago, R., Malvar, R. A., Barros-Rios, J., Samayoa, L. F., Butrón, A. (2016). Hydroxycinnamate synthesis and association with Mediterranean corn borer resistance. J. Agric. Food Chem. 64, 539–551. doi: 10.1021/acs.jafc.5b04862
Seleiman, M. F., Almutairi, K. F., Alotaibi, M., Shami, A., Alhammad, B. A., Battaglia, M. L. (2021). Nano-fertilization as an emerging fertilization technique: why can modern agriculture benefit from its use? Plants 10, 2. doi: 10.3390/plants10010002
Shabala, S., Bose, J., Fuglsang, A. T., Pottosin, I. (2016). On a quest for stress tolerance genes: membrane transporters in sensing and adapting to hostile soils. J. Exp. Bot. 67, 1015–1031. doi: 10.1093/jxb/erv465
Stein, S., Faust, F., Jung, S., Schubert, S. (2019). Expression of plasma membrane H+-ATPase in cluster roots of white lupin under phosphorus deficiency. J. Plant Nutr. Soil Sci. 182, 867–870. doi: 10.1002/jpln.201900124
Su, J., Ma, Q., Li, M., Hao, F., Wang, C. (2018). Multi-locus genome-wide association studies of fiber-quality related traits in Chinese early-maturity upland cotton. Front. Plant Sci. 9. doi: 10.3389/fpls.2018.01169
Tamba, C. L., Ni, Y. L., Zhang, Y. M. (2017). Iterative sure independence screening EM-Bayesian LASSO algorithm for multi-locus genome-wide association studies. PloS Comput. Biol. 13, e1005357. doi: 10.1371/journal.pcbi.1005357
Tamba, C. L., Zhang, Y. M. (2018). A fast mrMLM algorithm for multi-locus genome-wide association studies. bioRxiv, 341784. doi: 10.1101/341784
Tracy, S. R., Nagel, K. A., Postma, J. A., Fassbender, H., Wasson, A., Watt, M. (2020). Crop improvement from phenotyping roots: highlights reveal expanding opportunities. Trends Plant Sci. 25, 105–118. doi: 10.1016/j.tplants.2019.10.015
Vats, S. (Ed.) (2018). Biotic and abiotic stress tolerance in plants (Singapore: Springer). doi: 10.1007/978-981-10-9029-5
Vos, P. G., Paulo, M. J., Voorrips, R. E., Visser, R. G., van Eck, H. J., van Eeuwijk, F. A. (2017). Evaluation of LD decay and various LD-decay estimators in simulated and SNP-array data of tetraploid potato. Theor. Appl. Genet. 130, 123–135. doi: 10.1007/s00122-016-2798-8
Wang, Q., Yuan, Y., Liao, Z., Jiang, Y., Wang, Q., Yuan, Y., Liao, Z., Jiang, Y., Wang, Q., Zhang, T., et al. (2019). Genome-wide association study of 13 traits in maize seedlings under low phosphorus stress. Plant Genome 12, 190039. doi: 10.3835/plantgenome2019.06.0039
Wang, S. B., Feng, J. Y., Ren, W. L., Huang, B., Zhou, L., Wen, Y. J., et al. (2016). Improving power and accuracy of genome-wide association studies via a multi-locus mixed linear model methodology. Sci. Rep. 6, 1–10. doi: 10.1038/srep19444
Wang, T., Xing, J., Liu, Z., Zheng, M., Yao, Y., Hu, Z., et al. (2019b). Histone acetyltransferase GCN5-mediated regulation of long non-coding RNA At4 contributes to phosphate starvation response in Arabidopsis. J. Exp. Bot. 70, 6337–6348. doi: 10.1093/jxb/erz359
Wang, W., Ding, G. D., White, P. J., Wang, X. H., Jin, K. M., Xu, F. S., et al. (2019a). Mapping and cloning of quantitative trait loci for phosphorus efficiency in crops: opportunities and challenges. Plant Soil 439, 91–112. doi: 10.1007/s11104-018-3706-6
Wang, Y., Lambers, H. (2020). Root-released organic anions in response to low phosphorus availability: recent progress, challenges and future perspectives. Plant Soil 447, 135–156. doi: 10.1007/s11104-019-03972-8
Wang, Y., Chen, Y. F., Wu, W. H. (2021). Potassium and phosphorus transport and signaling in plants. J. Integr. Plant Biol. 63, 34–52. doi: 10.1111/jipb.13053
Wen, Y. J., Zhang, H., Ni, Y. L., Huang, B., Zhang, J., Feng, J. Y., et al. (2018). Methodological implementation of mixed linear models in multi-locus genome-wide association studies. Brief Bioinform. 19, 700–712. doi: 10.1093/bib/bbw145
Withers, P. J., Rodrigues, M., Soltangheisi, A., De Carvalho, T. S., Guilherme, L. R., Benites, V. D. M., et al. (2018). Transitions to sustainable management of phosphorus in Brazilian agriculture. Sci. Rep. 8, 1–13. doi: 10.1038/s41598-018-20887-z
Xu, C., Zhang, H., Sun, J., Guo, Z., Zou, C., Li, W. X., et al. (2018). Genome-wide association study dissects yield components associated with low-phosphorus stress tolerance in maize. Theor. Appl. Genet. 131, 1699–1714. doi: 10.1007/s00122-018-3108-4
Yan, J., Shah, T., Warburton, M. L., Buckler, E. S., McMullen, M. D., Crouch, J. (2009). Genetic characterization and linkage disequilibrium estimation of a global maize collection using SNP markers. PloS One 4, e8451. doi: 10.1371/journal.pone.0008451
Yang, Y., Chai, Y., Zhang, X., Lu, S., Zhao, Z., Wei, D., et al. (2020). Multi-locus GWAS of quality traits in bread wheat: mining more candidate genes and possible regulatory network. Front. Plant Sci. 11. doi: 10.3389/fpls.2020.01091
Yu, T. S., Lue, W. L., Wang, S. M., Chen, J. (2000). Mutation of Arabidopsis plastid phosphoglucose isomerase affects leaf starch synthesis and floral initiation. Plant Physiol. 123, 319–326. doi: 10.1104/pp.123.1.319
Yuan, W., Zhang, D., Song, T., Xu, F., Lin, S., Xu, W., et al. (2017). Arabidopsis plasma membrane H+-ATPase genes AHA2 and AHA7 have distinct and overlapping roles in the modulation of root tip H+ efflux in response to low-phosphorus stress. J. Exp. Bot. 68, 1731–1741. doi: 10.1093/jxb/erx040
Zhan, J., Li, G., Ryu, C. H., Ma, C., Zhang, S., Lloyd, A., et al. (2018). Opaque-2 regulates a complex gene network associated with cell differentiation and storage functions of maize endosperm. Plant Cell 30, 2425–2446. doi: 10.1105/tpc.18.00392
Zhang, H., Uddin, M. S., Zou, C., Xie, C., Xu, Y., Li, W. X. (2014). Meta-analysis and candidate gene mining of low-phosphorus tolerance in maize. J. Integr. Plant Biol. 56, 262–270. doi: 10.1111/jipb.12168
Zhang, J., Feng, J. Y., Ni, Y. L., Wen, Y. J., Niu, Y., Tamba, C. L., et al. (2017). pLARmEB: integration of least angle regression with empirical Bayes for multilocus genome-wide association studies. Heredity 118, 517–524. doi: 10.1038/hdy.2017.8
Zhang, K., Liu, S., Li, W., Liu, S., Li, X., Fang, Y., et al. (2018). Identification of QTNs controlling seed protein content in soybean using multi-locus genome-wide association studies. Front. Plant Sci. 9. doi: 10.3389/fpls.2018.01690
Zhang, Y. M., Jia, Z., Dunwell, J. M. (2019). The applications of new multi-locus GWAS methodologies in the genetic dissection of complex traits. Front. Plant Sci. 10. doi: 10.3389/fpls.2019.00100
Zhang, Y. W., Tamba, C. L., Wen, Y. J., Li, P., Ren, W. L., Ni, Y. L., et al. (2020). mrMLM v4. 0: An R platform for multi-locus genome-wide association studies. Genomics Proteomics Bioinf. 18, 481–487. doi: 10.1016/j.gpb.2020.06.006
Zheng, H., Yang, Z., Wang, W., Guo, S., Li, Z., Liu, K., et al. (2020). Transcriptome analysis of maize inbred lines differing in drought tolerance provides novel insights into the molecular mechanisms of drought responses in roots. Plant Physiol. Biochem. 149, 11–26. doi: 10.1016/j.plaphy.2020.01.027
Keywords: Zea mays L., GWAS, PUE, root architecture system, associative mapping
Citation: Zeffa DM, Júnior LP, de Assis R, Delfini J, Marcos AW, Koltun A, Baba VY, Constantino LV, Uhdre RS, Nogueira AF, Moda-Cirino V, Scapim CA and Gonçalves LSA (2024) Multi-locus genome-wide association study for phosphorus use efficiency in a tropical maize germplasm. Front. Plant Sci. 15:1366173. doi: 10.3389/fpls.2024.1366173
Received: 05 January 2024; Accepted: 10 July 2024;
Published: 23 August 2024.
Edited by:
Sushil Satish Chhapekar, University of Missouri, United StatesReviewed by:
Parameswaran C., Indian Council of Agricultural Research (ICAR), IndiaWricha Tyagi, Central Agricultural University, India
Copyright © 2024 Zeffa, Júnior, de Assis, Delfini, Marcos, Koltun, Baba, Constantino, Uhdre, Nogueira, Moda-Cirino, Scapim and Gonçalves. This is an open-access article distributed under the terms of the Creative Commons Attribution License (CC BY). The use, distribution or reproduction in other forums is permitted, provided the original author(s) and the copyright owner(s) are credited and that the original publication in this journal is cited, in accordance with accepted academic practice. No use, distribution or reproduction is permitted which does not comply with these terms.
*Correspondence: Leandro Simões Azeredo Gonçalves, bGVhbmRyb3NhZ0B1ZWwuYnI=