- 1Department of Smart Agriculture Systems, Chungnam National University, Daejeon, Republic of Korea
- 2Department of Biosystems Machinery Engineering, Chungnam National University, Daejeon, Republic of Korea
Pierce’s disease (PD) is a serious threat to grape production in Europe. This disease is caused by Xylella fastidiosa and is mediated by insect vectors, suggesting its high potential for spread and necessity for early monitoring. In this study, hence, potential distribution of Pierce’s disease varied with climate change and was spatially evaluated in Europe using ensemble species distribution modeling. Two models of X. fastidiosa and three major insect vectors (Philaenus spumarius, Neophilaenus campestris, and Cicadella viridis) were developed using CLIMEX and MaxEnt. The consensus areas of the disease and insect vectors, along with host distribution, were evaluated using ensemble mapping to identify high-risk areas for the disease. Our predictions showed that the Mediterranean region would be the most vulnerable to Pierce’s disease, and the high-risk area would increase three-fold due to climate change under the influence of N. campestris distribution. This study demonstrated a methodology for species distribution modeling specific to diseases and vectors while providing results that could be used for monitoring Pierce’s disease by simultaneously considering the disease agent, vectors, and host distribution.
1 Introduction
Pierce’s disease, caused by Xylella fastidiosa, damages various economically important agricultural crops, including grapes, almonds, citrus fruits, coffee, and peaches (Almeida et al., 2005; European Food Safety Authority, 2020). X. fastidiosa was first reported in the USA (Pierce, 1892) and has spread to Europe and Asia (Leu and Su, 1993; Montero-Astua et al., 2008; Amanifar et al., 2014; Denancé et al., 2017), causing Pierce’s disease with symptoms of leaf chlorosis, wilting, and diebacks in infected plants (Almeida et al., 2005). X. fastidiosa has been of particular significance in Europe since its initial discovery in olive trees in southern Italy (Saponari et al., 2013). This disease has severely damaged agricultural crops in European countries, including France, Spain, Portugal, and Germany (EFSA Panel on Plant Health, 2015; Olmo et al., 2017). X. fastidiosa is currently listed on the EPPO A2 list of pests recommended for regulation as a quarantine pest, demonstrating the need for monitoring to suppress damage and disease severity (EPPO, 2019).
X. fastidiosa is transmitted to other hosts through insect vectors that feed on the xylem tissue of plants (Almeida, 2016) and establish persistent and non-circulative infections within the foregut of insects (Purcell and Finlay, 1979; Almeida et al., 2005). These vectors of Pierce’s disease are found in many parts of the world and have spread the disease. In Europe, Philaenus spumarius is a major insect vector widely found in various habitats, including agricultural fields, grasslands, and woodland edges (Cornara et al., 2017). This pest causes significant damage to olive trees in Italy (Cornara et al., 2017). Species from the Aphrophoridae family, including Neophilaenus campestris and Philaenus italosignus, and species from the Cicadellidae family, including Cicadella viridis, are known vectors of X. fastidiosa in Europe (Trkulja et al., 2022). These vectors acquire the bacterium when they feed on infected plants and can spread Pierce’s disease by feeding on host plants. Moreover, because the flight ability of these vectors increases the risk of the spread of X. fastidiosa it is important to control insect vectors to prevent the damage caused by this disease, requiring a method that effectively confines potential areas exposed to insect vector distribution (Lago et al., 2021).
Species Distribution Model (SDM) evaluates the potential distribution and occurrence probability of a species as a function of the estimated relationships among species biology, occurrence areas, and environmental characteristics (Elith and Leathwick, 2009) and has been further applied for the spatial prediction of disease and surveillance of invasive species (Peterson and Vieglais, 2001; Peterson et al., 2003). Owing to its advantages in screening areas exposed to the target species in advance, it has been applied to develop the fundamentals necessary for monitoring and controlling diseases and pests (Bosso et al., 2017; Jung et al., 2019; Byeon et al., 2021; Lee et al., 2022; Song et al., 2022; Yoon et al., 2023). SDM algorithms can generally be classified into mechanistic and correlative models (Kearney et al., 2010; Li and Wang, 2013). Each algorithm differs in the required data, variable format, operational method, and process of obtaining predicted results, meaning that suitable algorithms vary according to the available information, target species, and research purpose. Recently, an ensemble model that uses two or more models has been used to complement the uncertainty of individual models and improve their reliability (Araújo and New, 2007; Kumar et al., 2015; Narouei-Khandan et al., 2020). Hence, its application to evaluate the potential distribution of a species is increasing, which has led to the development of ensemble models for studies with worldwide concerns (Araújo and New, 2007; Mainali et al., 2015). The use of multiple environmental variables, not just climatic factors, improves predictive performance (Matyukhina et al., 2014; Bradie and Leung, 2017; Lee et al., 2021).
Few studies have investigated the characteristics of Pierce’s disease in terms of symptoms, ecology, and vectors (Davis et al., 1978; Anas et al., 2008; Chatterjee et al., 2008; Raffini et al., 2020). However, SDM studies assessing the risk of the disease are relatively limited with two notable studies by using the MaxEnt model (Bosso et al., 2016a), and the CLIMEX model (Hoddle, 2004). These studies showed the notable application of SDM to the disease, but both are simple models only utilizing the disease records and climatic data, suggesting a need for considering additional environmental conditions with a recently advanced modeling technique. In this study, we evaluated the potential risk areas for Pierce’s disease in Europe using a novel ensemble SDM, integrating different algorithms used for the disease and vectors with host distribution due to climate change. CLIMEX, a mechanistic model, was used to predict climatically suitable regions for X. fastidiosa, while MaxEnt, a correlative presence-only model, was used to evaluate potential areas of occurrence for major insect vectors (Raffini et al., 2020). The ensemble model was then spatially constructed by projecting the consensus areas of the disease and insect vectors in addition to the host distribution so that the final outcome could identify high-risk areas vulnerable to Pierce’s disease.
2 Materials and methods
2.1 Acquisition and processing of occurrence data
The occurrence coordinates of X. fastidiosa were obtained from the disease distribution by using Global Biodiversity Information Facility (GBIF, 2022a), Center for Agriculture and Bioscience International (CABI, www.cabi.org), and previous studies (Bosso et al., 2016b; Castillo et al., 2019; Safady et al., 2019). A total of 49 distribution coordinates in Europe were confirmed after cross-checking the above multiple sources, which should minimize occurrence uncertainty.
For insect vectors of Pierce’s disease, P. spumarius, N. campestris, and C. viridis were selected because they are the widely distributed predominant vectors of X. fastidiosa in Europe (Janse and Obradovic, 2010; Elbeaino et al., 2014; Morente et al., 2018). To obtain specific occurrence coordinates, we used GBIF (GBIF, 2022b; GBIF, 2022c; GBIF, 2022d) and CABI, and the occurrence data were carefully determined by cross-checking the two databases. Then, spatial filtering was applied to the occurrence data to minimize the sampling bias by balancing the sampling density (Kramer-Schadt et al., 2013). The spatial filtering buffer was determined based on flying ability, setting 5 and 3 km for P. spumarius and N. campestris, respectively, whereas C. viridis was spatially filtered with a default buffer radius of 10 km (Lago et al., 2021). The spatial rarefying function in the SDM toolbox developed for ArcGIS (version 10.8.1, ESRI, USA) was employed for spatial filtering (Brown, 2014). Finally, 4772, 234, and 993 European occurrence points for P. spumarius, N. campestris, and C. viridis, respectively, were confirmed on the map (Figure 1).
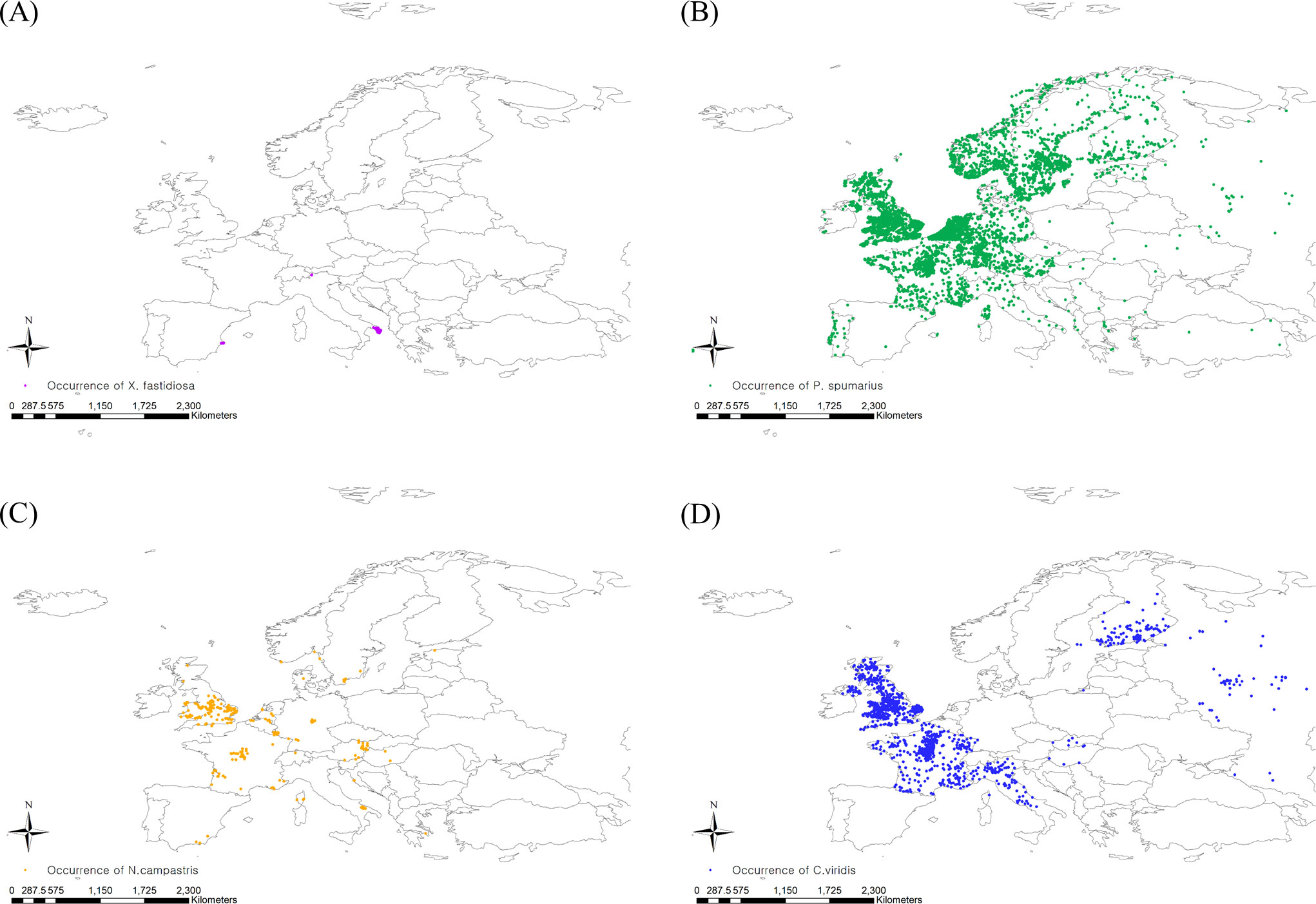
Figure 1 Occurrence coordinates of (A) Xylella fastidiosa, (B) Philaenus spumarius, (C) Neophilaenus campestris and (D) Cicadella viridis.
2.2 Acquisition and processing of meteorological data
Meteorological data from 1990–2018, including maximum temperature, minimum temperature, precipitation, and elevation, were obtained with a 2.5-minute resolution from WorldClim (https://www.worldclim.org) (Fick and Hijmans, 2017). The meteorological data were then converted into 19 bioclimatic variables to be used as MaxEnt model variables in ASCII format using R software (R Core Team, 2021) (Hijmans et al., 2017). The obtained meteorological data were extracted for the cells assigned to Europe and converted into two separate files, recording locations (loc file) and meteorology (met file), which was the required format for the CLIMEX model (Kriticos et al., 2015).
For future prediction, a 2.5-minute resolution of the Shared Socioeconomic Pathways (SSP) 585 climate change scenario for 2041–2060, generated by the MIROC6 model, was obtained (Fick and Hijmans, 2017). For the MaxEnt model, the scenario was obtained in the form of a bioclimatic variable identically defined to the current bioclimatic variables. In contrast, the monthly average minimum temperature, maximum temperature, and precipitation of SSP 585 were obtained for the CLIMEX model. Then, the same data processing was applied to obtain a format applicable to the CLIMEX model, as was done for the current meteorological data. Consequently, we obtained current and future meteorological data of the same type and time for use with different SDM tools.
2.3 MaxEnt modeling for insect vectors
MaxEnt is a modeling algorithm that we used for the three insects (Raffini et al., 2020). This model evaluates the possibility of occurrence by training environmental variables in the occurrence areas of a species (Phillips et al., 2004; Phillips et al., 2006; Elith et al., 2011). Because the spatial autocorrelation of bioclimatic variables can lead to model overfitting, it is necessary to select model variables that are not correlated. In this study, a variable showing a correlation coefficient > 0.8 for a biologically driven variable was removed (Kumar et al., 2014; Ancillotto et al., 2019; Yoon and Lee, 2021). The selected MaxEnt model variables for insect vectors were 10, 12, and 10 bioclimatic variables for P. spumarius, N. campestris, and C. viridis, respectively (Table 1).
Because it is required to determine the model features and regularization multiplier (RM), we used ENMeval in the R package, which compares all possible combinations of model settings to find the RM and the best model features of linear (L), quadratic (Q), product (P), threshold (T), and hinge (H) based on the Akaike information criterion (AIC) (Muscarella et al., 2014). The optimal model features for P. spumarius and C. viridis were RM of 0.5, and LQHPT features, while RM of 0.5 and LQ features were optimal for N. campestris evaluation. The model was then operated with 10,000 random backgrounds using 10-fold cross-validation, and the output was recorded in logistic format and projected onto a map using ArcGIS.
Two widely used metrics were employed to evaluate the reliability of the developed model: the area under the receiver operator curve (AUC) and true skill statistics (TSS) (Fielding and Bell, 1997; Allouche et al., 2006; Merow et al., 2013). In general, AUC < 0.7 is considered a poor performance, 0.7 ≤ AUC < 0.8 is moderate, and AUC ≥ 0.8 is good to excellent performance (Merckx et al., 2011; Peterson et al., 2011). True skill statistics, a more practical metric than the AUC, were calculated using a threshold value that maximized the sum of sensitivity and specificity (Liu et al., 2005). In general, it was considered that a value of TSS < 0.2 indicated a poor performance, 0.2 ≤ TSS < 0.4 was an acceptable performance, 0.4 ≤ TSS <0.6 was a moderate performance, and TSS ≥ 0.6 suggested a good performance (Landis and Koch, 1977; Tobeña et al., 2016).
2.4 CLIMEX modeling for Pierce’s disease
CLIMEX (version 4.0; Hearne Software, Melbourne, Australia) predicts the potential distribution of a species by evaluating the biologically suitable areas in a local climate (Kriticos et al., 2015). CLIMEX uses parameters representing the biological responses of a species to climate to evaluate the possibility of pest invasion based on climatic suitability (Byeon et al., 2018). The outcome is Ecoclimatic Index (EI), a quantitative representation of the climatic suitability of a species in a specific area. The EI value, which comprehensively estimates species growth and inhibition under given climatic conditions, was scaled from 0 to 100 (Kriticos et al., 2015). A species cannot be theoretically established at zero EI, whereas an EI >30 suggests an optimal climate for species inhabitation (Kriticos et al., 2015). In this study, we employed a previously developed CLIMEX model for Pierce’s disease (Hoddle, 2004) (Table 2).
Because of the characteristics of the CLIMEX model, which determines the parameter sets showing the best fit to the actual distribution data, there is no standard method for evaluating the performance of the CLIMEX model. Therefore, we estimated its accuracy by counting the actual occurrence records included in the simulation, as in previous CLIMEX studies (McConnachie et al., 2011; Saavedra et al., 2015).
2.5 Distribution of host of X. fastidiosa
Pierce’s disease affects more than 300 plant species, including grapes, citrus fruits, coffee, olives, almonds, blueberries, and other herbaceous plants (Stancanelli et al., 2015). To consider the distribution of host plants, we obtained a geodatabase of land cover maps in Europe using the Copernicus Land Monitoring Service (CLC, https://land.copernicus.eu/) (Büttner, 2014). Among the 44 classified areas in the land cover, areas planted with vineyards, fruit trees, berry plantations, and olive groves that were particularly damaged by Pierce’s disease, were extracted and projected onto the map (Figure 2).
2.6 Ensemble mapping of the potential distributions of Pierce’s disease and insect vector
The current and future potential distributions of insect vectors and the climatic suitability for Pierce’s disease predicted using MaxEnt and CLIMEX, respectively, were overlaid using ArcGIS (Byeon et al., 2021; Lee et al., 2021). The prediction of insect vectors was converted into binary maps by establishing a common threshold value (the 10th percentile training presence logistic threshold in MaxEnt) to classify presence or absence. The binary map of each insect vector was then superimposed on a scale of cells (the minimum projection unit under a given resolution) to find the consensus areas for all three insect vectors in Europe. The CLIMEX result of Pierce’s disease was also converted into a binary map divided into suitable regions with EI ≥1 and unsuitable regions with EI<1. The two binary maps were overlapped to define consensus areas showing the potential distributions of both the disease and insect vectors. The regions that were potentially the most vulnerable to Pierce’s disease were identified by overlapping the host distribution map with the disease vector map.
3 Results
3.1 Evaluating potential distribution of Pierce’s disease using CLIMEX
The existing CLIMEX model includes all occurrence coordinates of X. fastidiosa in Europe within the prediction region, suggesting that the model is reliable (Hoddle, 2004). When applying a threshold level of EI > 1, X. fastidiosa was predicted to be distributed in the southern regions of Europe under the current climate, with an estimated area of 1,948,597 km2 (Figure 3A). However, it is predicted to increase to 3,137,960 km2, reaching Hungary, England, Belgium, and Germany, in future based on climate change.
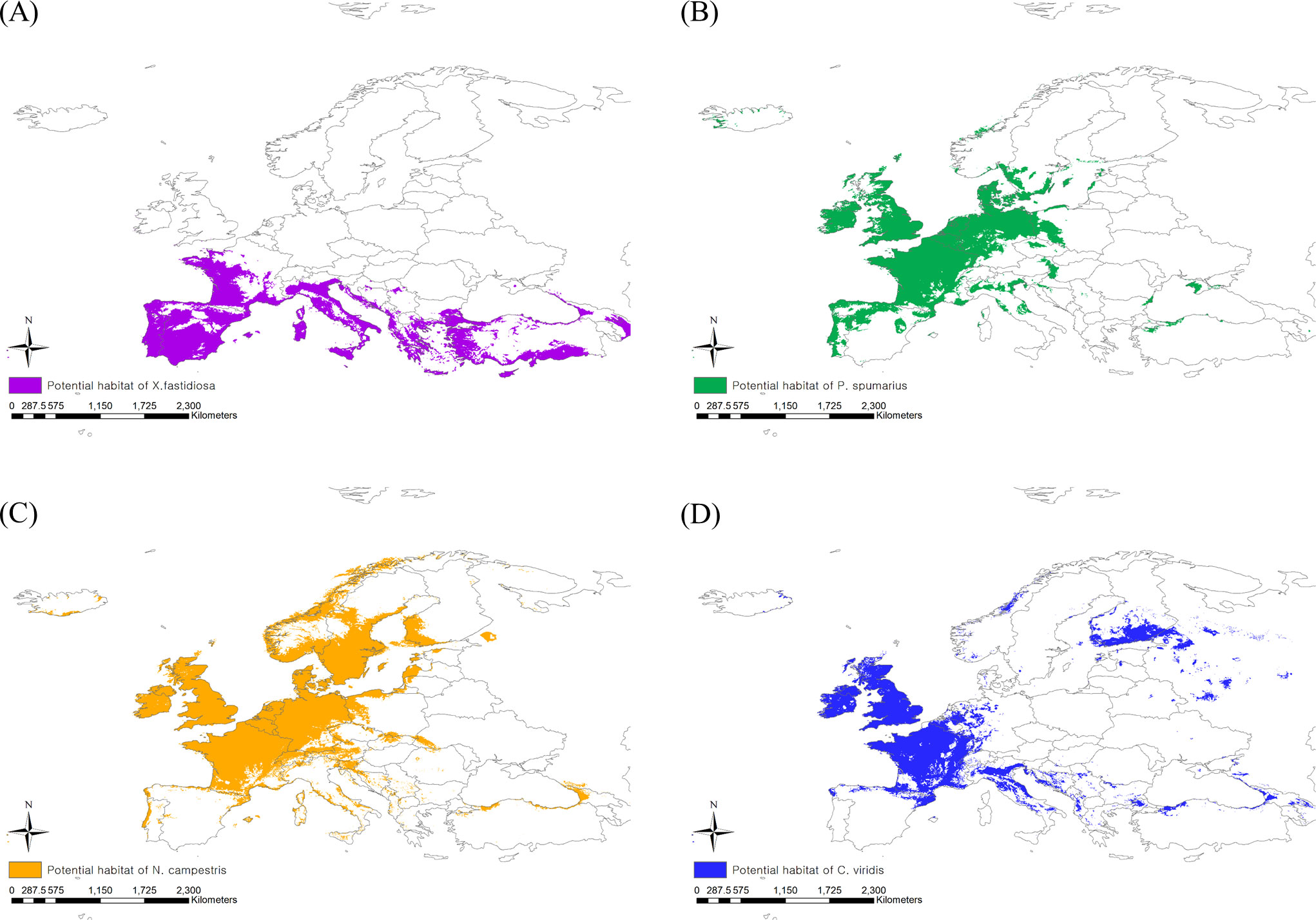
Figure 3 Potential risk area under the current climate of (A) Xylella fastidiosa, (B) Philaenus spumarius, (C) Neophilaenus campestris and (D) Cicadella viridis.
3.2 Evaluating the potential distribution of insect vectors of Pierce’s disease using MaxEnt
The developed MaxEnt models for insect vectors showed AUC values of 79, 0.94, and 0.90, and TSS values of 0.84, 0.89, and 0.91 for P. spumarius, N. campestris, and C. viridis, respectively, suggesting the model performance was sufficient.
When applying the 10th percentile training presence logistic threshold under the current climate, the potential distribution areas of P. spumarius were estimated to be 2,333,408 km2 including France, Germany, Belgium, the Netherlands, the United Kingdom, some neighboring countries, and the northern area of Turkey (Figure 3B). The largest potential distribution areas were observed for N. campestris at 3,460,361 km2, reaching northern Europe (Denmark, Sweden, Norway, and Finland) (Figure 3C). The potential distribution area of C. viridis was estimated to be 1,854,718 km2, covering France, the United Kingdom, Finland, and eastern Russia (Figure 3D). With climate change, the potential distribution areas of the three insect vectors tended to shift southward, but their sizes decreased drastically (Figures 4A–D). The potential distribution area of P. spumarius was observed only in small spots in Italy, Croatia, Albania, Greece, and Georgia, totaling 26,487 km2, which is a 98% decrease from the area under the current climate. N. campestris is expected to move southward to Spain, Italy, Greece, and Turkey. However, the area decreased to 1,895,461 km2, equivalent to 55% of the current area. The potential distribution area of C. viridis shrank to 14,358 km2, which is approximately 99% less than the potential area under the current climate and showed small spots in Italy, Greece, and Georgia.
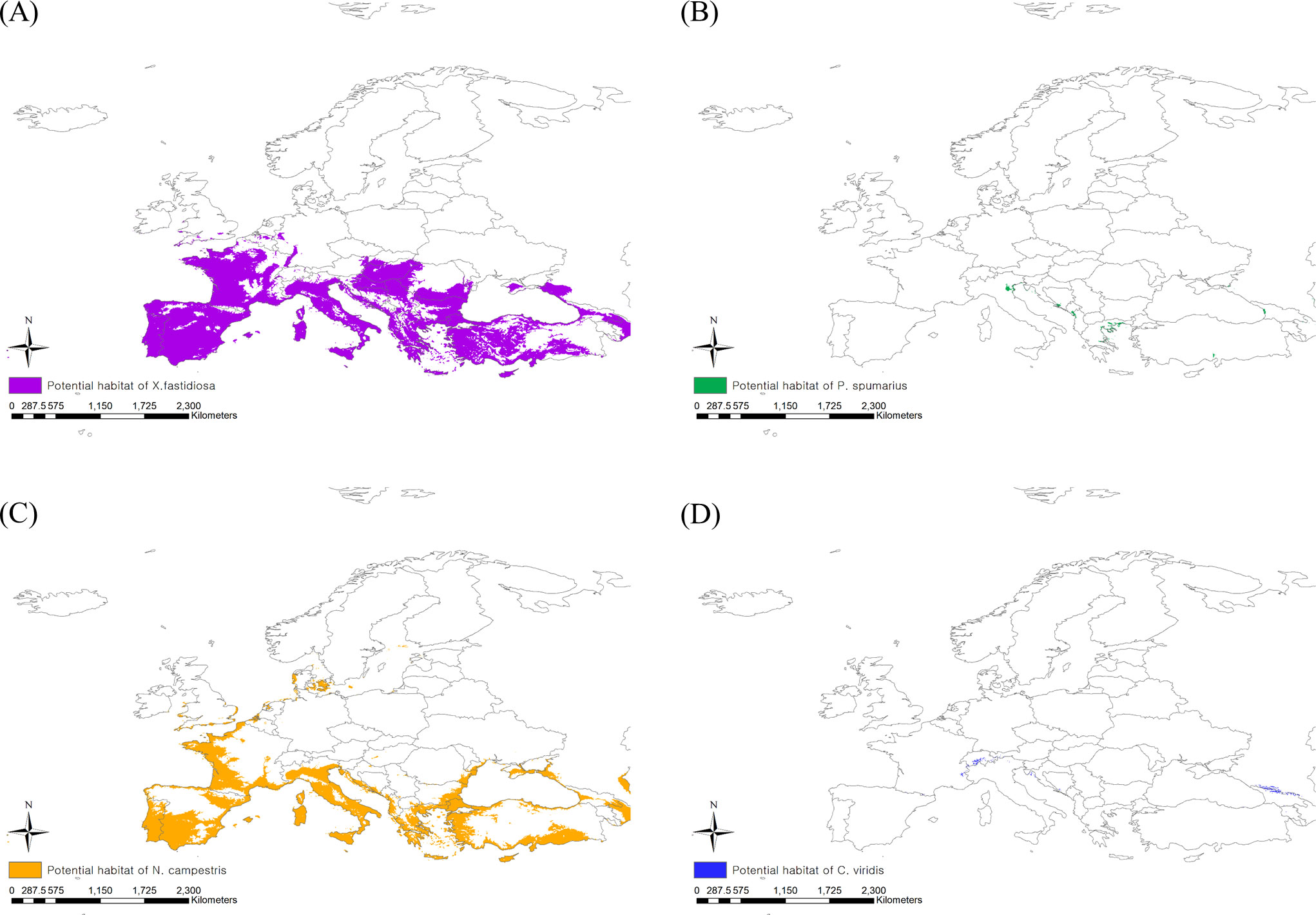
Figure 4 Potential risk area under the future climate (2041–2060) of (A) Xylella fastidiosa, (B) Philaenus spumarius, (C) Neophilaenus campestris and (D) Cicadella viridis.
Bioclimatic variables related to climatic variation contributed to the model performance, suggesting that these pests are sensitive to climatic conditions (Table 1). Temperature seasonality showed the highest contribution (65.6%) to the model for P. spumarius, followed by precipitation in the warmest quarter (9%) and annual mean temperature (7.4%). For N. campestris, the minimum temperature of the coldest month showed the highest contribution (36.3%), followed by isothermality (17.4%) and temperature seasonality (17.2%). Isothermality contributed the most (39.3%) to the model of C. viridis, followed by annual temperature range (16.8%) and precipitation of the warmest quarter (10.7%).
3.3 Evaluating the potential risk areas of Pierce’s disease damage by using ensemble mapping
High-risk areas where Pierce’s disease could be mediated by insect vectors were derived by extracting the consensus areas for Pierce’s disease and insect vectors under current and future climates (Figure 5). The present high-risk areas, which is estimated to be 849,062 km2, were in southern Europe, such as Spain and Italy, as well as in eastern France. Based on climate change, risky areas could expand to 1,731,618 km2, covering most regions of southern Europe, such as Portugal, Spain, Italy, Greece, and Turkey. This is because the consensus areas increased as the potential area for Pierce’s disease moved southward, although the potential distribution area of the vectors decreased.
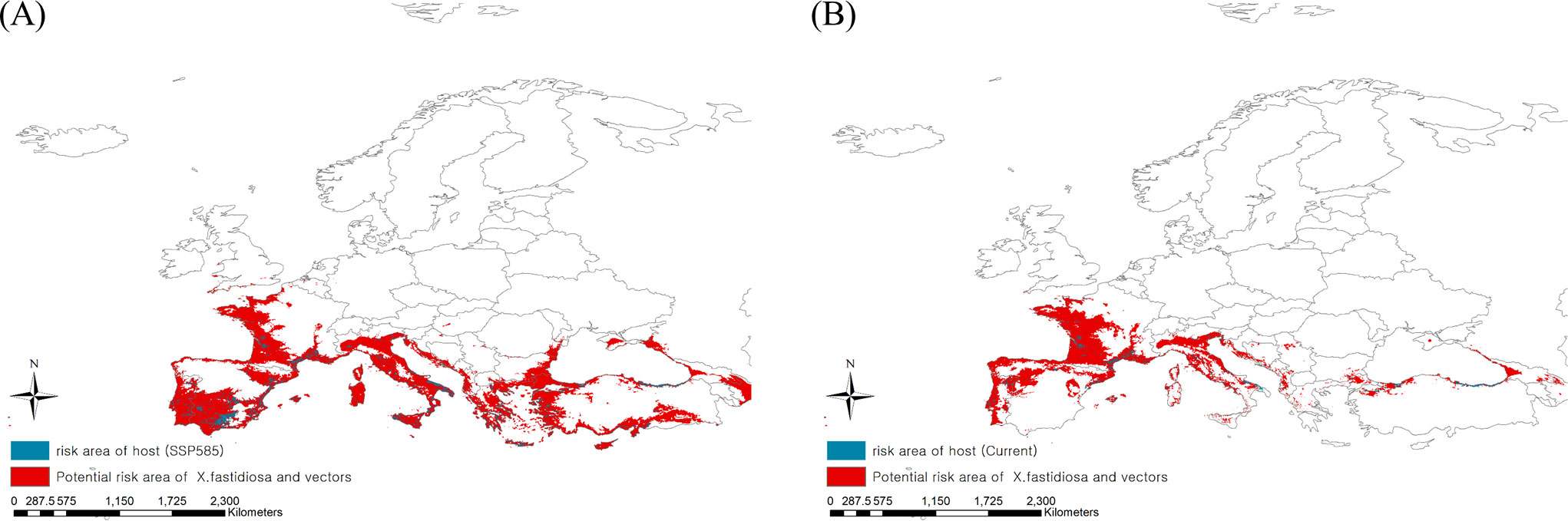
Figure 5 Ensemble mapping to illustrate high risk areas of Pierce’s disease mediated by insect vectors under the (A) current and (B) future climate (2041–2060).
Three maps projecting the disease, insect vectors, and hosts were constructed to identify the most threatened host area (Figure 5). Because of the southward expansion of potential areas of Pierce’s disease, the host distribution region was estimated to be affected more than three-fold due to climate change: 121,637 km2 in the future from 36,082 km2 under the current climate of 149,286 km2 of host distribution.
4 Discussion
This study used dual-species distribution modeling of mechanistic and correlative algorithms for an ensemble spatial analysis of the potential distribution of Pierce’s disease. Ensemble models have recently been used to build reliable models by combining the characteristics of different algorithms in cases with many uncertainties, such as in species distribution modeling (Stohlgren et al., 2010). The decision on which type of algorithm to apply to each species should be based on the characteristics of the target species, the amount of available data, and the purpose of the prediction (Yoon et al., 2023). Previously, it was shown that SDM was applicable for evaluating risk areas exposed to X. fastidiosa distribution according to climate change (Bosso et al., 2016a). However, the model was developed using the limited coordinates and outdated climatic variables. Because the correlative SDM highly depends on occurrence coordinates, the limited number of X. fastidiosa occurrence records might increase uncertainty of a modeling result. In addition, the correlative SDM is trained by values assigned to variables at occurrence coordinates, meaning the variables that can reflect the current conditions can increase the model reliability. Thus, it is necessary to compensate these drawbacks by employing additional conditions and updated variable information. This attempted to decrease model uncertainty with the ensemble modeling of the disease and insect vectors as the disease spread is constrained by habitats of them, and to increase model reliability with the updated climatic variables recorded by 2018. This study focused on identifying areas exposed to the risk of Pierce’s disease mediated by insect vectors. Thus, we aimed to construct a model that incorporates the habitats of actual vectors with biologically possible regions of disease occurrence, along with the availability of occurrence data. The climate is an important factor affecting the outbreak of Pierce’s disease, as the viability of Pierce’s disease varies with weather conditions, in addition to the breed or age of the infected host (Feil and Purcell, 2001). Tropical, subtropical, and Mediterranean climates favor the survival and development of Pierce’s disease, implying that the probability of the disease occurring is high under favorable climatic conditions through vector mediation (Purcell, 1997). For this reason, the CLIMEX model might be more suitable because it constructs a physiological niche by analyzing the suitability within a given climate based on the biological characteristics of a species, compared with a machine learning-based model that finds a realized niche that is environmentally similar to the occurrence area (Kriticos et al., 2015). In contrast, the MaxEnt model was used for insect vectors because a relatively sufficient number of occurrence coordinates was available, which is the most important requirement in developing a reliable machine learning-based model (Phillips et al., 2004; Elith et al., 2011). Moreover, vectors are affected by other factors such as host and topology; thus, a model incorporating different variables other than climate would be suitable. We believe that ensemble models, which combine different models depending on data availability and the main targets of prediction, can increase the reliability of predictions, as opposed to simply applying the same algorithm to two or more species or applying different algorithms to one species.
The climate is a dominant factor affecting the distribution of insect species (Björkman and Niemelä, 2015). In our analysis, some areas shared the potential distribution of all three insect vectors, indicating a common climatic factor that confined their habitats. Isothermality (Bio3) contributed significantly to the MaxEnt model of the three insect vectors, which quantified the ratio of the annual maximum and minimum temperature differences to the monthly average daily temperature difference (O’donnell and Ignizio, 2012). All insect vectors were highly likely to occur at approximately 30% or higher, suggesting that small seasonal differences favored their occurrence of the insect vectors. The three species used in this study are mainly distributed in the southern and western regions of Europe, where the isothermality is high enough to aid the spread of X. fastidiosa. The annual temperature range in Europe is between approximately 15 and 25°C, and this range is included within the maximum and minimum temperatures (5–28°C) observed in the actual outbreak area of Pierce’s disease. This indicates that favorable conditions for the disease and vectors are consistent (Yoon and Lee, 2023). Interestingly, N. campestris was the only one of three vectors that could potentially mediate Pierce’s disease under climate change, while the habitats of two other species in Europe were predicted to decrease significantly. This may be due to the differences in the dominant factors that contributed the most to the model. In the model of N. campestris, the minimum temperature of the coldest month (Bio6) showed the highest contribution. It was found that the probability of occurrence was high in areas where Bio6 was 0 or more, and the occurrence area appeared to move to the Mediterranean coast where Bio6 was above 0 due to climate change. N. campestris overwinters as eggs and develops into larvae in spring; therefore, warm temperatures due to global warming may lead to the early hatching and development of the pest (Elbeaino et al., 2014). This is consistent with a previous SDM study which showed that the lowest temperature in the coldest month was a main explanatory variable for X. fastidiosa distribution (Raffini et al., 2020). This suggests that winter climate not only serves as an important model variable for predicting the spread of diseases mediated by insect vectors but also indicates its significance as a factor in forecasting disease outbreaks necessary for implementing control measures. In contrast, temperature seasonality (Bio4), a major variable of P. saltuarius, decreased with climate change, leading to an unsuitable environment for pest occurrence, whereas C. viridis was expected to decrease because of a decrease in isothermality (Bio3) due to climate change.
Among the hosts affected by Pierce’s disease, vineyards and olive groves play a significant role in Europe, a major wine-producing country (Bisson et al., 2002). The optimal temperature for growing grapes is 25–32°C, and they are largely cultivated near the Mediterranean coast (Hochberg et al., 2015; Gutiérrez-Gamboa et al., 2021). Temperatures below 12°C and above 34°C limited the growth and survival of X. fastidiosa, which is similar to the optimal temperature for growing grapes (Feil and Purcell, 2001). Growth Index (GI) is an indicator of population growth potential during the favorable season in the CLIMEX model, showing high values in the spring and fall seasons by avoiding hot and dry environments that can delay symptoms (Feil and Purcell, 2001). Although there are differences depending on the vector biology, adult emergence occurs between April and June or between July and October (Bodino et al., 2019). This is similar to the peak GI period, worsening the spread of Pierce’s disease; consequently, it is necessary to pay particular attention during this period. Pierce’s disease eventually occurs in an environment where both the host and vector are available. Unfortunately, the favorable climate and time for growing hosts are consistent with the requirements for disease and vector development. From this point of view, countries along the Mediterranean coast and western France are at high risk of Pierce’s disease occurrence regardless of climate change, whereas southern Spain is projected to become the most at-risk region for the disease due to climate change. Consequently, intensive pest and disease control before disease outbreaks outbreak is necessary for these countries (Janse and Obradovic, 2010; Raffini et al., 2020; Morelli et al., 2021).
5 Conclusion
This study applied different algorithms for disease and insect vectors and predicted the potential occurrence areas of Pierce’s disease that vary with climate change using an ensemble modeling approach by spatially relating the model results with the host distribution. The main occurrence areas in Europe were predicted to be near the Mediterranean coast. However, it could expand southward, mediated by insect vectors due to climate change, causing severe damage due to consistency between areas of disease occurrence and host cultivation. Therefore, it is possible to effectively identify high-risk areas for the potential occurrence of the disease in advance and to implement intensive monitoring and control to suppress the spread of the disease and minimize the potential damage that may increase due to climate change. From the perspective of SDM, this study is significant because it predicts Pierce’s disease and it methodologically proposes an ensemble model by integrating individual models of disease and vectors with the host as a model variable. Although this model does not consider changes in host distribution owing to climate change, integrating a model that considers host changes can further enhance its reliability.
Data availability statement
The raw data supporting the conclusions of this article will be made available by the authors, without undue reservation.
Author contributions
SY: conceptualization, methodology, software, validation, formal analysis, investigation, data curation, writing –original draft, and visualization. W-HL: conceptualization, methodology, resources, writing - review & editing, supervision, and funding acquisition. All authors contributed to the article and approved the submitted version.
Funding
This work was supported by the Basic Science Research Program through the National Research Foundation of Korea (NRF) funded by the Ministry of Science, ICT and Future Planning (NRF-2021R1I1A3050757).
Conflict of interest
The authors declare that the research was conducted in the absence of any commercial or financial relationships that could be construed as a potential conflict of interest.
Publisher’s note
All claims expressed in this article are solely those of the authors and do not necessarily represent those of their affiliated organizations, or those of the publisher, the editors and the reviewers. Any product that may be evaluated in this article, or claim that may be made by its manufacturer, is not guaranteed or endorsed by the publisher.
References
Allouche, O., Tsoar, A., Kadmon, R. (2006). Assessing the accuracy of species distribution models: prevalence, kappa and the true skill statistic (TSS). J. Appl. Ecol. 43 (6), 1223–1232. doi: 10.1111/j.1365-2664.2006.01214.x
Almeida, R. P. (2016). “Xylella fastidiosa vector transmission biology,” in Vector-mediated transmission of plant pathogens (Minnesota, USA: American Phytopathological Society Press), 165–174.
Almeida, R. P., Blua, M. J., Lopes, J. R., Purcell, A. H. (2005). Vector transmission of Xylella fastidiosa: applying fundamental knowledge to generate disease management strategies. Ann. Entomol. Soc Am. 98 (6), 775–786. doi: 10.1603/0013-8746(2005)098[0775:VTOXFA]2.0.CO;2
Amanifar, N., Taghavi, M., Izadpanah, K., Babaei, G. (2014). Isolation and pathogenicity of Xylella fastidiosa from grapevine and almond in Iran. Phytopathol. Mediterranea 53 (2), 318–327.
Anas, O., Harrison, U. J., Brannen, P. M., Sutton, T. B. (2008). The effect of warming winter temperatures on the severity of pierce's disease in the Appalachian mountains and piedmont of the southeastern united states. Plant Health Progress 9 (1), 13. doi: 10.1094/PHP-2008-0718-01-RS
Ancillotto, L., Mori, E., Bosso, L., Agnelli, P., Russo, D. (2019). The Balkan long-eared bat (Plecotus kolombatovici) occurs in Italy-first confirmed record and potential distribution. Mamm. Biol. 96, 61–67. doi: 10.1016/j.mambio.2019.03.014
Araújo, M. B., New, M. (2007). Ensemble forecasting of species distributions. Trends Ecol. Evol. 22 (1), 42–47. doi: 10.1016/j.tree.2006.09.010
Bisson, L. F., Waterhouse, A. L., Ebeler, S. E., Walker, M. A., Lapsley, J. T. (2002). The present and future of the international wine industry. Nature 418 (6898), 696–699. doi: 10.1038/nature01018
Bodino, N., Cavalieri, V., Dongiovanni, C., Plazio, E., Saladini, M. A., Volani, S., et al. (2019). Phenology, seasonal abundance and stage-structure of spittlebug (Hemiptera: aphrophoridae) populations in olive groves in Italy. Sci. Rep. 9 (1), 17725. doi: 10.1038/s41598-019-54279-8
Bosso, L., Di Febbraro, M., Cristinzio, G., Zoina, A., Russo, D. (2016a). Shedding light on the effects of climate change on the potential distribution of Xylella fastidiosa in the Mediterranean basin. Biol. Invasions 18, 1759–1768. doi: 10.1007/s10530-016-1118-1
Bosso, L., Luchi, N., Maresi, G., Cristinzio, G., Smeraldo, S., Russo, D. (2017). Predicting current and future disease outbreaks of diplodia sapinea shoot blight in Italy: species distribution models as a tool for forest management planning. For. Ecol. Manage. 400, 655–664. doi: 10.1016/j.foreco.2017.06.044
Bosso, L., Russo, D., Di Febbraro, M., Cristinzio, G., Zoina, A. (2016b). Potential distribution of Xylella fastidiosa in Italy: a maximum entropy model. Phytopathol. Mediterranea. 55 (1), 62–72.
Bradie, J., Leung, B. (2017). A quantitative synthesis of the importance of variables used in MaxEnt species distribution models. J. Biogeogr. 44 (6), 1344–1361. doi: 10.1111/jbi.12894
Brown, J. L. (2014). SDM toolbox: a python-based GIS toolkit for landscape genetic, biogeographic and species distribution model analyses. Methods Ecol. Evol. 5, 694–700. doi: 10.1111/2041-210X.12200
Büttner, G. (2014). CORINE land cover and land cover change products. In: Manakos, I., Braun, M. (eds) Land Use and Land Cover Mapping in Europe. Remote Sensing and Digital Image Processing, vol 18. Springer, Dordrecht. doi: 10.1007/978-94-007-7969-3_5
Byeon, D., Jung, S., Lee., W. H. (2018). Review of CLIMEX and MaxEnt for studying species distribution in south Korea. J. Asia-Pac Biodivers. 11 (3), 325–333. doi: 10.1016/j.japb.2018.06.002
Byeon, D. H., Kim, S. H., Jung, J. M., Jung, S., Kim, K. H., Lee, W. H. (2021). Climate-based ensemble modelling to evaluate the global distribution of Anoplophora glabripennis (Motschulsky). Agr For. Entomol. 23 (4), 569–583. doi: 10.1111/afe.12462
Castillo, A. I., Tuan, S. J., Retchless, A. C., Hu, F. T., Chang, H. Y., Almeida, R. P. (2019). Draft whole-genome sequences of Xylella fastidiosa subsp. fastidiosa strains TPD3 and TPD4, isolated from grapevines in hou-li, Taiwan. Microbiol. resource announcements 8 (47), e00835–e00819. doi: 10.1128/MRA.00835-19
Chatterjee, S., Almeida, R. P. P., Lindow, S. (2008). Living in two worlds: the plant and insect lifestyles of Xylella fastidiosa. Annu. Rev. Phytopathol. 46, 243–271. doi: 10.1146/annurev.phyto.45.062806.094342
Cornara, D., Saponari, M., Zeilinger, A. R., de Stradis, A., Boscia, D., Loconsole, G., et al. (2017). Spittlebugs as vectors of xylella fastidiosa in olive orchards in Italy. J. Pest Sci. 90, 521–530. doi: 10.1007/s10340-016-0793-0
Davis, M. J., Purcell, A. H., Thomson, S. V. (1978). Pierce's disease of grapevines: isolation of the causal bacterium. Sci. 199 (4324), 75–77. doi: 10.1126/science.199.4324.75
Denancé, N., Legendre, B., Briand, M., Olivier, V., de Boisseson, C., Poliakoff, F., et al. (2017). Several subspecies and sequence types are associated with the emergence of Xylella fastidiosa in natural settings in France. Plant Pathol. 66 (7), 1054–1064. doi: 10.1111/ppa.12695
European Food Safety Authority (2020). Update of the Xylella spp. host plant database−systematic literature search up to 30 June 2019. EFSA J. 18, 6114. doi: 10.2903/j.efsa.2020.6114
EFSA Panel on Plant Health (PLH) (2015). Scientific opinion on the risks to plant health posed by Xylella fastidiosa in the EU territory, with the identification and evaluation of risk reduction options. EFSA J. 13, 3989. doi: 10.2903/j.efsa.2015.3989
Elbeaino, T., Yaseen, T., Valentini, F., Moussa, I. E. B., Mazzoni, V., D'onghia, A. M. (2014). Identification of three potential insect vectors of Xylella fastidiosa in southern Italy. Phytopathol. Mediterr. 53 (2), 328–332.
Elith, J., Leathwick, J. R. (2009). Species distribution models: ecological explanation and prediction across space and time. Annu. Rev. ecology evolution systematics. 40, 677–697. doi: 10.1146/annurev.ecolsys.110308.120159
Elith, J., Phillips, S. J., Hastie, T., Dudík, M., Chee, Y. E., Yates, C. J. (2011). A statistical explanation of MaxEnt for ecologists. Divers. Distrib. 17 (1), 43–57. doi: 10.1111/j.1472-4642.2010.00725.x
EPPO. (European and Mediterranean Plant Protection Organization) (2019). PM 7/24 (4) Xylella fastidiosa. EPPO Bull. 49, 175–227.
Feil, H., Purcell, A. H. (2001). Temperature-dependent growth and survival of Xylella fastidiosa in vitro and in potted grapevines. Plant Disease 85 (12), 1230–1234. doi: 10.1094/PDIS.2001.85.12.1230
Fick, S. E., Hijmans, R. J. (2017). WorldClim 2: new 1km spatial resolution climate surfaces for global land areas. Int. J. Climatology 37 (12), 4302–4315. doi: 10.1002/joc.5086
Fielding, A. H., Bell, J. F. (1997). A review of methods for the assessment of prediction errors in conservation presence/absence models. Environ. Conserv. 24, 38–49. doi: 10.1017/S0376892997000088
GBIF (2022a). Available at: GBIF.org (Accessed 8 February 2022).
GBIF (2022b). Available at: GBIF.org (Accessed 24 March 2022).
GBIF (2022c). Available at: GBIF.org (Accessed 30 May 2022).
GBIF (2022d). Available at: GBIF.org (Accessed 8 February 2022).
Gutiérrez-Gamboa, G., Zheng, W., Martinez de Toda, F. (2021). Strategies in vineyard establishment to face global warming in viticulture: a mini review. J. Sci. Food Agriculture 101 (4), 1261–1269. doi: 10.1002/jsfa.10813
Hijmans, R. J., Phillips, S., Leathwick, J., Elith, J., Hijmans, M. R. J. (2017). Package ‘dismo’. Circles 9 (1), 1–68.
Hochberg, U., Batushansky, A., Degu, A., Rachmilevitch, S., Fait, A. (2015). Metabolic and physiological responses of shiraz and cabernet sauvignon (Vitis vinifera l.) to near optimal temperatures of 25 and 35 c. Int. J. Mol. Sci. 16 (10), 24276–24294. doi: 10.3390/ijms161024276
Hoddle, M. S. (2004). The potential adventive geographic range of glassy-winged sharpshooter, Homalodisca coagulata and the grape pathogen Xylella fastidiosa: implications for California and other grape growing regions of the world. Crop Protection 23 (8), 691–699. doi: 10.1016/j.cropro.2003.11.017
Janse, J. D., Obradovic, A. (2010). Xylella fastidiosa: its biology, diagnosis, control and risks. J. Plant Pathol. 92 (S1), 35‒48.
Jung, J. M., Byeon, D. H., Jung, S., Lee, W. H. (2019). Effect of climate change on the potential distribution of the common cutworm (Spodoptera litura) in south Korea. Entomol. Res. 49 (12), 519–528. doi: 10.1111/1748-5967.12398
Kearney, M. R., Wintle, B. A., Porter, W. P. (2010). Correlative and mechanistic models of species distribution provide congruent forecasts under climate change. Conserv. letters 3 (3), 203–213. doi: 10.1111/j.1755-263X.2010.00097.x
Kramer-Schadt, S., Niedballa, J., Pilgrim, J. D., Schröder, B., Lindenborn, J., Reinfelder, V., et al. (2013). The importance of correcting for sampling bias in MaxEnt species distribution models. Divers. Distrib. 19 (11), 1366–1379. doi: 10.1111/ddi.12096
Kriticos, D. J., Maywald, G. F., Yonow, T., Zurcher, E. J., Herrmann, N. I., Sutherst, R. W. (2015). CLIMEX version 4: exploring the effects of climate on plants, animals and diseases (Canberra: CSIRO), 184.
Kumar, S., Graham, J., West, A. M., Evangelista, P. H. (2014). Using district-level occurrences in MaxEnt for predicting the invasion potential of an exotic insect pest in India. Computers and Electronics in Agriculture 103, 55–62. doi: 10.1016/j.compag.2014.02.007
Kumar, S., Neven, L. G., Zhu, H., Zhang, R. (2015). Assessing the global risk of establishment of Cydia pomonella (Lepidoptera: tortricidae) using CLIMEX and MaxEnt niche models. J. Econ. Entomol. 108, 1708–1719. doi: 10.1093/jee/tov166
Lago, C., Garzo, E., Moreno, A., Barrios, L., Martí-Campoy, A., Rodríguez-Ballester, F., et al. (2021). Flight performance and the factors affecting the flight behaviour of Philaenus spumarius the main vector of Xylella fastidiosa in Europe. Sci. Rep. 11 (1), 1–14. doi: 10.1038/s41598-021-96904-5
Landis, J. R., Koch, G. G. (1977). The measurement of observer agreement for categorical data. Biometrics 33, 159–174. doi: 10.2307/2529310
Lee, D. S., Choi, W. I., Nam, Y., Park, Y. S. (2021). Predicting potential occurrence of pine wilt disease based on environmental factors in south Korea using machine learning algorithms. Ecol. informatics 64, 101378. doi: 10.1016/j.ecoinf.2021.101378
Lee, W. H., Jung, J. M., Lee, H. S., Lee, J. H., Jung, S. (2021). Evaluating the invasion risk of longhorn crazy ants (Paratrechina longicornis) in south Korea using spatial distribution model. J. Asia-Pacific Entomology 24 (2), 279–287. doi: 10.1016/j.aspen.2021.01.007
Lee, W. H., Song, J. W., Yoon, S. H., Jung, J. M. (2022). Spatial evaluation of machine learning-based species distribution models for prediction of invasive ant species distribution. Appl. Sci. 12 (20), 10260. doi: 10.3390/app122010260
Leu, L. S., Su, C. C. (1993). Isolation, cultivation, and pathogenicity of Xylella fastidiosa, the causal bacterium of pear leaf scorch disease in Taiwan. Plant Disease 77 (6), 642–646. doi: 10.1094/PD-77-0642
Li, X., Wang, Y. (2013). Applying various algorithms for species distribution modelling. Integrative Zoology 8 (2), 124–135. doi: 10.1111/1749-4877.12000
Liu, C., Berry, P. M., Dawson, T. P., Pearson, R. G. (2005). Selecting thresholds of occurrence in the prediction of species’ distributions. Ecography 28, 385–393. doi: 10.1111/1749-4877.12000
Mainali, K. P., Warren, D. L., Dhileepan, K., McConnachie, A., Strathie, L., Hassan, G., et al. (2015). Projecting future expansion of invasive species: comparing and improving methodologies for species distribution modeling. Global Change Biol. 21 (12), 4464–4480. doi: 10.1111/gcb.13038
Matyukhina, D. S., Miquelle, D. G., Murzin, A. A., Pikunov, D. G., Fomenko, P. V., Aramilev, V. V., et al. (2014). Assessing the influence of environmental parameters on amur tiger distribution in the Russian far East using a MaxEnt modeling approach. Achievements Life Sci. 8 (2), 95–100. doi: 10.1016/j.als.2015.01.002
McConnachie, A. J., Strathie, L. W., Mersie, W., Gebrehiwot, L., Zewdie, K., Abdurehim, A., et al. (2011). Current and potential geographical distribution of the invasive plant parthenium hysterophorus (Asteraceae) in eastern and southern Africa. Weed Res. 51 (1), 71–84. doi: 10.1111/j.1365-3180.2010.00820.x
Merckx, B., Steyaert, M., Vanreusel, A., Vincx, M., Vanaverbeke, J. (2011). Null models reveal preferential sampling,spatial autocorrelation and overfitting in habitat suitability modelling. Ecol. Modelling 222, 588–597. doi: 10.1016/j.ecolmodel.2010.11.016
Merow, C., Smith, M. J., Silander, Jr J.A. (2013). A practical guide to MaxEnt for modeling species' distributions: what it does, and why inputs and settings matter. Ecography 36 (10), 1058–1069. doi: 10.1111/j.1600-0587.2013.07872.x
Montero-Astúa, M., Saborío-R, G., Chacón-Díaz, C., Garita, L., Villalobos, W., Moreira, L., et al. (2008). First report of Xylella fastidiosa in avocado in Costa Rica. Plant Dis. 92 (1), 175–175. doi: 10.1094/PDIS-92-1-0175C
Morelli, M., García-Madero, J. M., Jos, Á., Saldarelli, P., Dongiovanni, C., Kovacova, M., et al. (2021). Xylella fastidiosa in olive: a review of control attempts and current management. Microorganisms 9 (8), 1771. doi: 10.3390/microorganisms9081771
Morente, M., Cornara, D., Moreno, A., Fereres, A. (2018). Continuous indoor rearing of philaenus spumarius, the main European vector of xylella fastidiosa. J. Appl. entomology 142 (9), 901–904. doi: 10.1111/jen.12553
Muscarella, R., Galante, P. J., Soley-Guardia, M., Boria, R. A., Kass, J. M., Urearte, M., et al. (2014). ENMeval: an r package for conducting spatially independent evaluations and estimating optimal model complexity for maxent ecological niche models. Methods Ecol. Evolution 6, 119–120. doi: 10.1111/2041-210X.12261
Narouei-Khandan, H. A., Worner, S. P., Viljanen, S. L. H., van Bruggen, A. H. C., Jones, E. E. (2020). Projecting the suitability of global and local habitats for myrtle rust (Austropuccinia psidii) using model consensus. Plant Pathology 69 (1), 17–27. doi: 10.1111/ppa.13111
O’donnell, M. S., Ignizio, D. A. (2012). Bioclimatic predictors for supporting ecological applications in the conterminous united states. US geological survey Data series 691 (10), 4–9.
Olmo, D., Nieto, A., Adrover, F., Urbano, A., Beidas, O., Juan, A., et al. (2017). First detection of xylella fastidiosa infecting cherry (Prunus avium) and polygala myrtifolia plants, in mallorca island, Spain. Plant Dis. 101, 1820. doi: 10.1094/PDIS-04-17-0590-PDN
Peterson, A. T., Soberón, J., Pearson, R. G., Anderson, R. P., Martínez-Meyer, E., Nakamura, M., et al. (2011). Ecological niches and geographic distributions (MPB-49) (Princeton University Press). doi: 10.1515/9781400840670
Peterson, A. T., Vieglais, D. A. (2001). Predicting species invasions using ecological niche modeling: new approaches from bioinformatics attack a pressing problem: a new approach to ecological niche modeling, based on new tools drawn from biodiversity informatics, is applied to the challenge of predicting potential species' invasions. BioScience 51 (5), 363–371. doi: 10.1641/0006-3568(2001)051[0363:PSIUEN]2.0.CO;2
Peterson, A. T., Vieglais, D. A., Andreasen, J. K. (2003). Migratory birds modeled as critical transport agents for West Nile virus in north America. Vector-Borne Zoonotic Diseases 3 (1), 27–37. doi: 10.1089/153036603765627433
Phillips, S. J., Anderson, R. P., Schapire, R. E. (2006). Maximum entropy modeling of species geographic distributions. Ecol. Modelling 190, 231–259. doi: 10.1016/j.ecolmodel.2005.03.026
Phillips, S. J., Dudík, M., Schapire, R. E. (2004). A maximum entropy approach to species distribution modeling. Proc. twenty-first Int. Conf. Mach. Learn., 83. doi: 10.1145/1015330.1015412
Pierce, N. B. (1892). The California vine disease: a preliminary report of investigations (No. 2) (US Government Printing Office).
Purcell, A. H. (1997). Xylella fastidiosa, a regional problem or global threat? J. Plant Pathol. 79, 99–105.
Purcell, A. H., Finlay, A. H. (1979). Evidence for noncirculative transmission of pierce’s disease bacterium by sharpshooter leafhoppers. Phytopathology 69 (4), 393–395. doi: 10.1094/Phyto-69-393
Raffini, F., Bertorelle, C., Biello, R., D’Urso, G., Russo, D., Bosso, L. (2020). From nucleotides to satellite imagery: approaches to identify and manage the invasive pathogen xylella fastidiosa and its insect vectors in Europe. Sustainability 12 (11), 4508. doi: 10.3390/su12114508
R Core Team (2021). R: a language and environment for statistical computing (Vienna, Austria: R Foundation for Statistical Computing). Available at: https://www.R-project.org/.
Saavedra, M. C., Avila, G. A., Withers, T. M., Holwell, G. I. (2015). The potential global distribution of the bronze bug thaumastocoris peregrinus carpintero and dellapé (H emiptera: thaumastocoridae). Agric. For. Entomology 17 (4), 375–388. doi: 10.1111/afe.12117
Safady, N. G., Lopes, J. R., Francisco, C. S., Coletta-Filho, H. D. (2019). Distribution and genetic diversity of Xylella fastidiosa subsp. pauca associated with olive quick syndrome symptoms in southeastern Brazil. Phytopathology 109 (2), 257–264. doi: 10.1094/PHYTO-07-18-0273-FI
Saponari, M., Boscia, D., Nigro, F., Martelli, G. P. (2013). Identification of DNA sequences related to xylella fastidiosa in oleander, almond and olive trees exhibiting leaf scorch symptoms in apulia (Southern Italy) J. Plant Pathol. 95, 659–668.
Song, J. W., Jung, J. M., Nam, Y., Jung, J. K., Jung, S., Lee, W. H. (2022). Spatial ensemble modeling for predicting the potential distribution of Lymantria dispar asiatica (Lepidoptera: erebidae: lymantriinae) in south Korea. Environ. Monit. Assessment 194 (12), 889. doi: 10.1007/s10661-022-10609-4
Stancanelli, G., Almeida, R., Bosco, D., Caffier, D., Czwienczek, E., Gregoire, J. C., et al. (2015). Assessing the risk posed to plant health by Xylella fastidiosa in the European union. CIHEAM international centre for advanced Mediterranean agronomic studies. Watch Letter 33, 1–8.
Stohlgren, T. J., Ma, P., Kumar, S., Rocca, M., Morisette, J. T., Jarnevich, C. S., et al. (2010). Ensemble habitat mapping of invasive plant species. Risk Analysis: Int. J. 30 (2), 224–235. doi: 10.1111/j.1539-6924.2009.01343.x
Tobeña, M., Prieto, R., Machete, M., Silva, M. A. (2016). Modeling the potential distribution and richness of cetaceans in the Azores from fisheries observer program data. Front. Mar. Sci. 3. doi: 10.3389/fmars.2016.00202
Trkulja, V., Tomić, A., Iličić, R., Nožinić, M., Milovanović, T. P. (2022). Xylella fastidiosa in Europe: from the introduction to the current status. Plant Pathol. J. 38 (6), 551–571. doi: 10.5423/PPJ.RW.09.2022.0127
Yoon, S., Jung, J. M., Hwang, J., Park, Y., Lee, W. H. (2023). Ensemble evaluation of the spatial distribution of pine wilt disease mediated by insect vectors in south Korea. For. Ecol. Management 529, 120677. doi: 10.1016/j.foreco.2022.120677
Yoon, S., Lee, W. H. (2021). Methodological analysis of bioclimatic variable selection in species distribution modeling with application to agricultural pests (Metcalfa pruinosa and Spodoptera litura). Comput. Electron. Agriculture 190, 106430. doi: 10.1016/j.compag.2021.106430
Keywords: CLIMEX, ensemble modeling, insect vectors, MaxEnt, Xylella fastidiosa
Citation: Yoon S and Lee W-H (2023) Assessing potential European areas of Pierce’s disease mediated by insect vectors by using spatial ensemble model. Front. Plant Sci. 14:1209694. doi: 10.3389/fpls.2023.1209694
Received: 21 April 2023; Accepted: 29 May 2023;
Published: 16 June 2023.
Edited by:
Liangxiu Han, Manchester Metropolitan University, United KingdomReviewed by:
Luciano Bosso, University of Naples Federico II, ItalyPaulo Adriano Zaini, University of California, Davis, United States
Copyright © 2023 Yoon and Lee. This is an open-access article distributed under the terms of the Creative Commons Attribution License (CC BY). The use, distribution or reproduction in other forums is permitted, provided the original author(s) and the copyright owner(s) are credited and that the original publication in this journal is cited, in accordance with accepted academic practice. No use, distribution or reproduction is permitted which does not comply with these terms.
*Correspondence: Wang-Hee Lee, d2FuZ2hlZUBjbnUuYWMua3I=