- 1Integrative Science Center of Germplasm Creation in Western China (Chongqing) Science City and Southwest University, College of Agronomy and Biotechnology, Southwest University, Chongqing, China
- 2Academy of Agricultural Sciences, Southwest University, Chongqing, China
- 3Engineering Research Center of South Upland Agriculture, Ministry of Education, Chongqing, China
Crop production is the primary goal of agricultural activities, which is always taken into consideration. However, global agricultural systems are coming under increasing pressure from the rising food demand of the rapidly growing world population and changing climate. To address these issues, improving high-yield and climate-resilient related-traits in crop breeding is an effective strategy. In recent years, advances in omics techniques, including genomics, transcriptomics, proteomics, and metabolomics, paved the way for accelerating plant/crop breeding to cope with the changing climate and enhance food production. Optimized omics and phenotypic plasticity platform integration, exploited by evolving machine learning algorithms will aid in the development of biological interpretations for complex crop traits. The precise and progressive assembly of desire alleles using precise genome editing approaches and enhanced breeding strategies would enable future crops to excel in combating the changing climates. Furthermore, plant breeding and genetic engineering ensures an exclusive approach to developing nutrient sufficient and climate-resilient crops, the productivity of which can sustainably and adequately meet the world’s food, nutrition, and energy needs. This review provides an overview of how the integration of omics approaches could be exploited to select crop varieties with desired traits.
1. Introduction
Since the origin of agriculture, food security has been one of the utmost precedence contemplations. In turn, plant breeding is one of the oldest agricultural activities that developed along with human civilization and a foremost method to meet the upsurged food demand. Humans have been cultivating and selecting crops that would serve their taste, nutritional values, high yield, and resistance to biotic and abiotic environments (Dossa et al., 2017). After the discovery of Mendel’s laws (1866) plant breeding enter a new era (Mendal, 1866), afterward pedigree breeding was developed based on the hybridization principle. The discovery of DNA structure (1863-1865) revolutionized plant breeding in the molecular era (Watson and Crick, 1953); thereupon, new breeding techniques were introduced such as marker-assisted selection (MAS) and the genetically modified (GM) approach (Shen et al., 2022). These discoveries shifted plant breeding from utter phenotype-selection to a combination of genotype and phenotype selection. Based on technical innovations plant breeding has been divided into four prominent categories; ensuant selection by farmers, statistical and experimental approach to improve selection, the convergence of genetic and genomic data, and the currently progressing era of optimal and precise design breeding (Shen et al., 2022).
Presently, it is a big challenge to feed the exponentially growing world population in changing climate for agriculture, particularly due to the diminution of fertile land with the incessant conversion of fertile land to semi-arid and nutrient deficient areas alongside salinity and waterlogging. The crop production is already under risk due to climate change, mainly staple food crops such as rice, maize, and wheat (Farooq et al., 2022; Syed et al., 2022). Crop productivity will be significantly impacted in the next decades by climate factors such as extreme temperature stresses, nutrient toxicity, or deficiency, changes in precipitation intensity and frequency, and other climate change-driven problems including salinity, waterlogging, drought, and land degradation (Teshome et al., 2020; Mahmood et al., 2021; Raza et al., 2021a). In addition to the detrimental effects of abiotic stress on plants, climate change has also exacerbated the impact of biotic constraints (insect and fungus), which significantly reduce the crop yield (Vaughan et al., 2018). These environmental constraints are the primary cause of the declining food productivity, which directly impacts economies worldwide. The basis for the sustained production of new varieties to address current and upcoming issues is the genetic diversity of crop plants.
Hence, an expeditious method of introducing elite climate-smart cultivars with desired traits (stress tolerance, yield and nutrition) is requisite. Plant breeding has always been pivotal for developing agriculture to produce sufficient food for a growing population. Its efficiency is tremendously improved by the technological innovations through OMICS approaches (genomics, transcriptomics, proteomics, metabolomics, and phenomics) and ensued greater food supply to meet the ever-increasing demand (Muthamilarasan et al., 2019; Yang et al., 2021). Multi-omics studies of plants have played a crucial role in interpreting metabolic pathways and their molecular regulators that control key traits and the growth processes of multiple plant species (Razzaq et al., 2019). Recent advances in next-generation sequencing (NGS) technologies have managed high throughput and swift data generation for OMICS experiments (Großkinsky et al., 2017; Schmidt et al., 2020), which has improved the accuracy, sensitivity, and detection throughput (Qi et al., 2019).
In addition, the integration of multi-omics data could interpret gene functions and networks better, under different biotic and abiotic stresses (Figure 1) (Yang et al., 2021). Using comprehensive multi-omics techniques, researchers have identified essential key factors in senescence, stress response, and harvest index of many economically important crops such as rice, wheat, soybeans, rapeseed, and maize (McLoughlin et al., 2018; Peng et al., 2020; Uchida et al., 2020; Ma et al., 2021a; Raza et al., 2021b). In the present review, we represent how the multi-omics revolution has upgraded plant breeding efficiency to enhance nutritional values, crop yield, and resistance against biotic and abiotic stresses of wild species for sustainable food security. In the future, the integration of multi-omics strategies will play an immense role in improving genetic development and crop breeding.
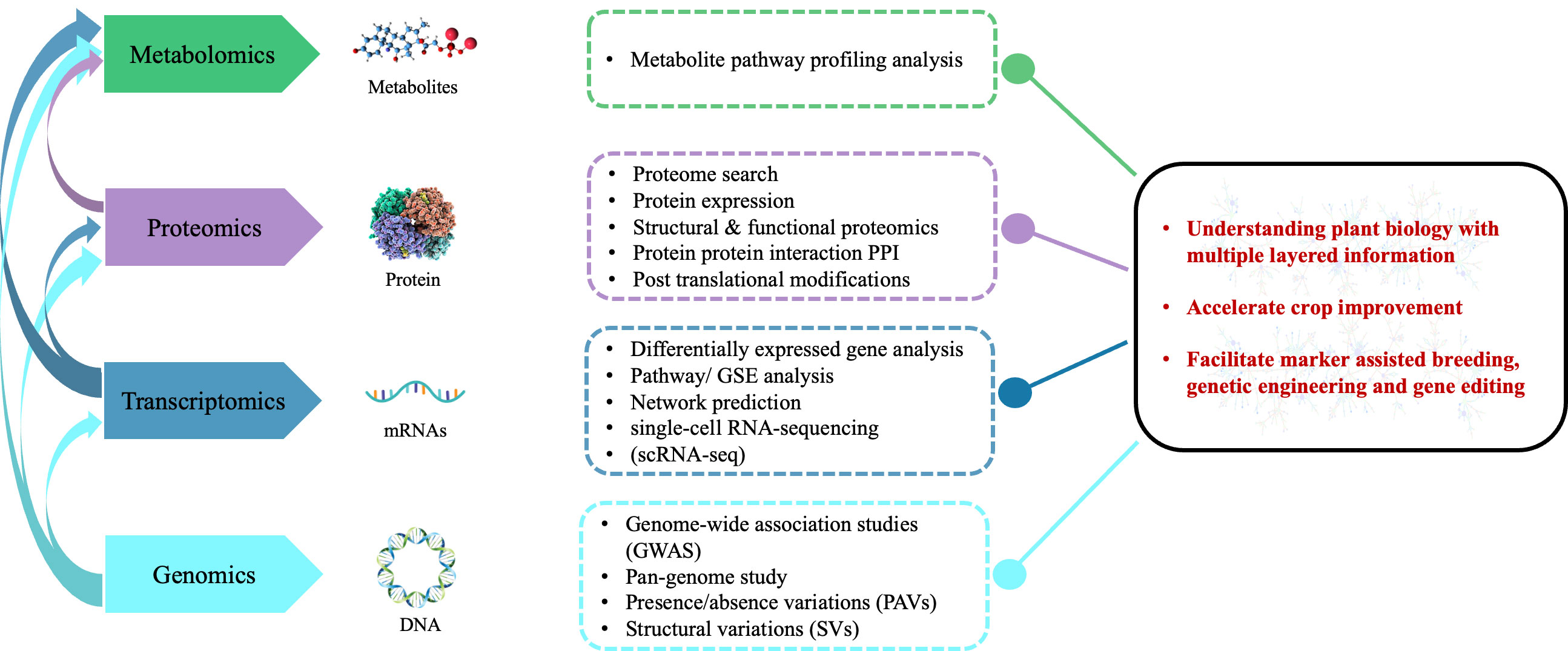
Figure 1 The prospects, limitations, and outlook for multi-omics based agricultural development in the future. Different multi-omics data layers, their interactions, the kinds of omics features found in each layer, and the methods used to evaluate omics data in various layers.
2. Multi-omics techniques accelerate the genetic dissection of complex traits
2.1. Genomics and pan-genomics
The relationship of genotype with phenotype is a prerequisite for accurate breeding design. Therefore, it is essential to genetically dissect the agronomic traits and identify the corresponding phenotypic variations. Numerous revolutions in DNA sequencing technology over the last 40 years have significantly improved sequencing throughput and quality continued to reduce costs, and significantly facilitated genome advancement and functional research (Shendure et al., 2017).
NGS is a deep sequencing (DP) or massively parallel sequencing (MPS) that permit plant genomes decoding. The first plant genome of Arabidopsis was constructed 22 years ago (Kaul et al., 2000; Theologis et al., 2000). Less than 300 whole genome assemblies at the chromosomal level (representing about 900 species) have been sequenced so far out of the estimated ~0.5 million species in the green plant clade (Kress et al., 2022), that include rice (Goff et al., 2002), maize (Schnable et al., 2009), tomato (The Tomato Genome Consortium, 2012), wheat (Ling et al., 2013) and rapeseed (Chalhoub et al., 2014). Benefitting from available high-quality reference genomes, a fairly large collection of genetic resources or populations of different crops are genotyped at the whole genome level. For example, it is estimated that whole-genome resequencing has so far been done for more than 6,000 soybeans accessions (Zhang et al., 2022b) and at least 10,000 rice accessions (Wing et al., 2018), which provide abundant genetic variation resources for genomic breeding of crops.
So far, advances in high-throughput sequencing technology have made the reference genome available in more than 800 plants (Shen et al., 2022), and most of which are de novo assembled by third-generation sequencing (TGS). The pan-genome was proposed first in 2005 and rapidly developed in recent twenty years due to the advent of Pacific Biosciences (PacBio) and Oxford Nanopore Sequencing (ONT) platforms. Compared to short insertions/deletions (indels) and SNPs, the structural difference (such as presence/absence variations (PAVs) and structural variations (SVs)) identified by pan-genome analysis play a vital role in the dissection of complex traits. The structural differences that have been determined in the pan-genome era requires a reassessment of the bases of phenotypes. The SVs have already been associated with environmental changes (Sutton et al., 2007; Cook et al., 2012) flowering time (Nitcher et al., 2013; Würschum et al., 2015), stress tolerance (Gabur et al., 2019), and plant domestication traits such as plant architecture (Tan et al., 2008; Zhou et al., 2009) and dehiscence (Lin et al., 2012).
It has been shown that SVs are associated with a wide range of biotic and abiotic stress tolerance in a various crop species. In maize, resistance to the sugarcane mosaic virus was induced at the Scmv1 locus by a complex SV comprising several transposable elements (TEs) that changed the expression level of atypical h-type thioredoxin (Liu et al., 2017). In potato, resistance to late blight in was acquired by two genes R1 and ELR that were introgressed from wild potato although missing in cultivated potato (Du et al., 2015). Furthermore, the graph-based pan-genome will revitalize the re-sequenced data in intercepting novel genetic variations, especially for large SVs (Shen et al., 2022). In PAVs of varied sizes and different lines of sorghum, the common deletion of a sulfotransferase gene conferred tolerance to the parasitic weed Striga by minimizing its germination stimulant effect (Gobena et al., 2017). Moreover, in abiotic stress resistance, variation in HvCBF4 and HvCBF2 copy number at the FR-H2 locus was linked to frost tolerance in barley (Francia et al., 2016). Salt sensitivity in soybean was found to be caused by an insertion of a Ty1/copia retrotransposon in the GmGHX1 cation H+ exchanger gene (Qi et al., 2014). The Pup1 locus in rice has been associated with the presence of a receptor-like cytoplasmic kinase gene that confers tolerance to phosphorus deficiency (Gamuyao et al., 2012). These studies suggested that SVs, despite their narrow focus, are likely to significantly influence crop improvement in changing climate.
Recently, the pan-genomes of cucumber, soybean, and rice were analyzed using graph-based approaches, revealing numerous novel large SVs and considerably easing the identification of relevant genetic variations for certain complex traits (Table 1) (Liu et al., 2020; Qin et al., 2021; Li et al., 2022a). In-depth, the rice pangenome research has revealed that SVs and gene copy number variations (gCNVs) supported environmental response and artificial selection during the process of evolution and domestication (Qin et al., 2021). A prior study on 25 inbred lines of maize identified similarities between PAVs and heterotic group membership (Darracq et al., 2018), showing that SVs can be useful for characterizing heterotic groups and selecting parental lines for the development of hybrid crops. In general, the comprehensive genome composition heterogeneity outlined by genomes and pan-genomes facilitates the establishment of novel methodologies for plant scientists to investigate functional alleles for phenotypic variations and for breeders to increase genetic resources to enhance crop germ plasm in changing climates (Della Coletta et al., 2021; James et al., 2021).
2.2. Epigenomics
During the last few decades, epigenomics has been developed into a frontier omics technique that can be used to explain the changes in the regulation of gene activities and expression under the epigenetic modifications of DNA sequences (Saeed et al., 2022). These epigenetic changes, such as DNA methylation, histone modifications, and chromatin accessibility, may assist us in determining how crops adapt to environmental changes without altering their DNA sequences.
Several techniques that can be used to investigate DNA methylation in plants, including whole genome bisulfite sequencing (WGBS), methyl-sensitive amplification polymorphism (MSAP), and methylated DNA immunoprecipitation (MEDIP) (Sun et al., 2022). The MSAP and WGBS techniques are frequently used to investigate the methylation status involved in regulating gene expression in plants under environmental stresses. Epigenetic research has revealed that drought-tolerant plants exhibited a more stable methylome, with differentially methylated regions (DMRs) linking genes primarily involved in the programmed cell death and stress response in mulberry (Ackah et al., 2022), mungbean (Zhao et al., 2022) and rice (Wang et al., 2016). Likewise, the differentially methylated epiloci (DME) identified 12 stress-related genes in rice genotypes under high temperature treatment (Li et al., 2022b). The methylome content of alfalfa plants increased during salinity stress, and 5-AzaC treatment (DNA methylation inhibitor) reduced the salt tolerance (Al-Lawati et al., 2016). Similarly, hypermethylation is one defense mechanism for plants that protects them from potential injury from heavy metal compounds and enables them to survive in harsh environments (Raza et al., 2022a; Sun et al., 2022). Numerous DNA-methylation studies have been conducted associating with seed development, plant organ differentiation, fruit ripening, and response to environmental stresses AND the functional role in gene regulation (Zhang et al., 2018). These studies provided evidence that DNA methylation may have regulatory roles in determining plant stress tolerance under a range of stress conditions.
In relation to plant growth and stress response mechanisms, histone changes and chromatin remodeling are important epigenetic processes that control gene expression by altering the chromatin status and activating transcription regulators. Most of techniques for detecting epigenome-level histone modifications rely on immunoprecipitation such as chromatin immunoprecipitation sequencing (ChIP-seq) and its modified form ChIP-exo, which has more specific binding sites. Other histone profiling techniques that are available and can be used effectively include ChIPmentation (ChIP-Tn5 transposase tagmentation), CUT&Tag (cleavage-under targets & tagmentation), and DamID (E. coli deoxyadenosinemethylase + protein of interest) (Mehrmohamadi et al., 2021). However, ChIP-seq is a commonly used technique in plants to identify the interaction between DNA-methylation sites and histone proteins (Chen et al., 2018). The dynamic variation in H3K4me3 and H3K27me3 in castor beans under salt stress was closely correlated with the transcript abundance of RSM1(RADIALIS-LIKE SANT/MYB 1), which has previously been identified as a positive regulator of salt resistance (Han et al., 2020). Following the post-harvest desiccation phase, the ABA-biosynthesis genes are activated in tea plants, resulting in ABA accumulation due to increased histone acetylation and decreased H3K9me2 (Gu et al., 2021). According to a recent study on rice, the dynamic nature of histone H3K27me3 and H3K27ac modifications regulates gene expression that are responsive to cold (Dasgupta et al., 2022). In addition to triggering broad changes in the histone methylome, stress signals specifically affect the methylation of genes responsive to stress (Xiao et al., 2022). These results demonstrate a correlation between stress-induced histone modifications and genome-wide transcription remodeling.
Chromatin accessibility profiling has been carried out in numerous plant species using DNase-seq (Dnase I hypersensitive sites sequencing), Mnase-seq (micrococcal-nuclease digestion sequencing), FAIRE-seq (formaldehyde-assisted isolation of regulatory element sequencing) and ATAC-seq (assay for transposase-accessible chromatin-sequencing) has found a wealth of novel information about the regulatory dynamics in plant genomes. Studies on chromatin accessibility have made it possible to build transcriptional networks that respond to environmental changes in rice (Wilkins et al., 2016). To illustrate the effect of abiotic stresses on A. thaliana two different techniques, FAIRE-seq and Dnase-seq were used to capture the open chromatin states (Raxwal et al., 2020). Moreover, chromatin accessibility analysis in maize cold tolerant lines during stress has revealed the re-allocation of resources from growth to defense (Jonczyk et al., 2020). To investigate the relationship between chromatin characteristics and gene expression in grapevine, chromatin accessibility was determined using ATAC-seq, Hi-C, and ChIP-seq (Schwope et al., 2021). Epigenetic changes are also involved in organ development and cell differentiation across the species (Maher et al., 2018). These studies contribute to our understanding of how plants respond to environmental cues by changing their gene expression, and how chromatin-based regulation of gene expression is probably essential for these responses.
2.3. Transcriptomics
Transcriptomics is the study of “transcriptome” which refers to the entire collection of RNA transcripts generated by an organism’s genome in a cell or tissue (Zhou et al., 2022a). Transcriptomics encompasses both mRNAs and ncRNAs in the cells, and it has recently been used extensively in crop breeding to investigate gene expression under different conditions (Schiessl et al., 2020). Traditional profiling approaches, which include differential display-PCR (DD-PCR), cDNAs-AFLP, and SSH were initially used to assess transcriptome dynamics, but these methods had poor resolution (Nataraja et al., 2017). The emergence of advanced throughput sequencing has allowed plant scientists to conduct extensive transcriptomics research (Mir et al., 2019). Recently, RNA-seq employing NGS methods has made it possible to characterize the transcriptome more precisely than with microarray.
The differential expression of mRNAs in rapeseed during development stages was determined to find the candidate genes controlling seed size (Niu et al., 2020). Signal peptides have been linked to the regulation of many plants’ biological processes, including immune response and development, according to the rice transcriptome study (Wang et al., 2020a). A comparative transcriptomic analysis of two extreme Chinese cabbage genotypes revealed that ion homeostasis is a significant biological pathway associated with plant’s instant adaptation to salt tolerance (Li et al., 2021a). It was also discovered that the transcription factors MYB, bZIP, and WRKY serve as regulators in the salt-responsive signaling pathway of maize roots (Zhang et al., 2021a). Similarly, genome-wide transcriptome profiling showed that the apple’s WRKY gene family responded to various biotic stresses (Zhang et al., 2021b). Transcriptome studies also include various kinds of non-coding RNAs. These non-coding RNAs come in many forms, including micro RNAs (miRNAs), circular RNAs (circRNAs), long non-coding RNAs (lncRNAs), ribosomal RNAs (rRNAs), and short interfering RNAs (siRNA), and they thought to be a promising target for crop improvement (Waititu et al., 2020; Zhou et al., 2022a). These non-coding RNAs perform various functions, such as miRNAs involved in slicing and post-transcriptional modification of target mRNAs. While lncRNAs serve as important regulators in several vital biological processes and circRNAs functioned as miRNA sponges, transcriptional and splicing regulators, and moderators of primary gene expression in plants (Lai et al., 2018; Waititu et al., 2020). Whole-transcriptome sequencing in plants was used to create the global landscape of these mRNAs in plants, such as maize (Liu et al., 2022a) Chinese cabbage (Shi et al., 2022) citrus (Fu et al., 2019).
Since it is generally recognized that different cell types play different biological functions in plant growth and development, it has become critically important in recent years to examine the transcriptome responses of plants at cellular level. In molecular biology, single-cell RNA-sequencing (scRNA-seq) is a high-resolution method is growing in popularity for studying plant functional genomes and transcriptional activity at the single-cell level. This method enabled researchers to examine heterogeneity in plants within different cell types (Yaschenko et al., 2022). The polyploidization events in plants were poorly understood by traditional transcriptome studies. However, the advent of scRNA-seq enabled to study of female gametes cells (Arabidopsis) without cross-contamination, advancing plant hybridization, polyploid genetics, and reproductive biology (Song et al., 2020). While scRNA-seq technologies have been extensively used in animal science, their application in plant sciences has just recently been understood. Hence, there have been fewer studies on the high-throughput single-cell transcriptome in plants than in animals until now. Thereafter, this knowledge could be put to better use in breeding initiatives with innovative and targeted goals to assist, for instance, crop production and quality, plant climate change adaptations, and plant tolerance to biotic and abiotic stressors.
2.4. Proteomics
Proteomics is a technique for analyzing all the proteins expressed within an organism, and it splits into four major types: sequence, structure, function, and expression proteomics (Aizat and Hassan, 2018). Different approaches are used to analyze these types, for intence, sequence proteomics analyzed by HPLC (high-performance liquid chromatography) (Hao et al., 2018) and structural proteomics by nuclear magnetic resonance (NMR), electron microscopy, crystallization, and X-ray diffraction of protein crystals (Woolfson, 2018; Opdensteinen et al., 2022). However, functional proteomics is examined through various methods as yeast one (Y1H) or two hybrids (Y2H) and protein micro-array profiling (Yang et al., 2020; Mao et al., 2020).
The development of protein extraction and separation techniques at the sample and genome-wide level has led to a rapid breakthrough in plant proteome research. Traditional proteomics methods rely on chromatography-based approaches. However, enzyme-linked immunosorbent assays (ELISA) could be used to study specific proteins. Subsequently, some advanced gel-based techniques were developed for protein quantification such as SDS-PAGE, 2-DE (two-dimensional gel-electrophoresis), and 2D-DIGE (two-dimensional differential gel-electrophoresis) (Yang et al., 2020).
In addition, protein microarrays and chips have been developed to analyze protein expression in small amounts of protein samples efficiently. Likewise, more hi-tech methods for quantitative proteomic analysis have been developed, including isotope-coded affinity tag (ICAT) labeling, stable isotope labeling with amino acids in cell culture (SILAC), and isobaric tag for relative and absolute quantification (iTRAQ) (Yang et al., 2020). The three-dimensional structure of proteins can be determined using a high-throughput technique called NMR spectroscopy, which may help to explain how proteins work biologically (Xiang et al., 2018). Despite the recent development of single-cell sequencing technologies to study the mechanism of cellular activity, single-cell proteome quantification still lags single-cell transcriptome achievements (Kashima et al., 2020; Vistain and Tay, 2021). However, Mass spectrometry (MS) has been evolving recently to measure the single-cell protein level (Vistain and Tay, 2021).
All the proteomic technological achievements could help scientists study the environmental effects of protein modifications in terms of crop resilience (Zhang et al., 2022a). Numerous studies have used proteomic analysis of the genetic complements of proteins to measure proteins and identify changes in protein expression as they relate to crop response to abiotic stimuli (Ueda and Seki, 2020; Zhang et al., 2022a). So, it might be possible to identify protein-regulatory mechanisms and understand how a particular protein contributes to stress tolerance by examining the proteome differences in crop responses to abiotic stimuli (Yu et al., 2018). This can be applied to crop breeding to increase agricultural outputs in the future even in challenging environmental conditions (Schulze et al., 2021).
2.5. Metabolomics
Metabolomics is one of the most current omics technologies for probing metabolites and elucidating crop resilience. According to the plant study trials, the inclusion of untargeted metabolome detection sped up the development of integrated metabolomics (Kumar et al., 2021). Primary metabolites are required for the synthesis of lipids, sugars, and amino acids in plants however, secondary metabolites comprised of flavonoids, atropine, carotenoids, phytic acid, as well as ROS, coenzymes, and antioxidants (Razzaq et al., 2019). Since metabolites are typically the main product in complex metabolic cascades, they can link the phenotype with the genome, transcriptome, and proteome (Misra et al., 2018).
The plant kingdom contains at least 391000 unique metabolomes (Nakabayashi and Saito, 2020); therefore, the development of analytical techniques is required to determine as many specialized metabolomes as possible. Analyses that are either targeted or untargeted can be used to assess the relative and absolute levels of metabolites. The analytical methods used for metabolomics primarily comprised liquid chromatography-mass spectrometry (LC-MS), gas chromatography-mass spectrometry (GS-MS), capillary electrophoresis-mass spectrometry (CE-MS), HPLC (high-performance liquid chromatography), NMR, and direct flow injection (DFI) (Razzaq et al., 2019; Raza, 2022).
NGS technologies have emerged as effective tools for examining gene regulation and the molecular dynamics of plant cellular responses to biotic and abiotic stresses (Abdelrahman et al., 2018). However, it is now possible to infer an early metabolic network from an organism’s genomic sequence by integrating metabolomics and NGS (Pan et al., 2020). The integrated information obtained from the NGS and metabolites profiling could help us to improve the crops in changing climatic conditions (Scossa et al., 2021). In the presence of numerous biotic stresses, the crucial function of metabolites in cereal crops, such as maize, barley, and rice has been recognized (Yang et al., 2021). The integrated transcriptome and metabolomic analysis of sesame emphasized the significance of amino acid metabolism, especially the saccharopine pathway, ABA metabolism, and signaling pathway for drought resistance (You et al., 2019). The identification of several candidate genes and metabolites associated with isoflavone synthesis and the tricarboxylic acid cycle further supports the significance of these metabolic pathways in the response of soybean to drought (Wang et al., 2022). Similarly, the role of metabolites (phenolics and phenylpropanoids) has been identified in maize under biotic and abiotic stresses (Block et al., 2020). In tomato, Trichoderma-induced biotic stress resulted in various metabolome alterations, which revealed that treated plants accumulated more isoprenoids (Coppola et al., 2019). In addition to stress resistance, metabolomics research in combination with other omics platforms is important for crop nutritional values that can provide adequate insights about the quality-related genes to develop future crops (Pott et al., 2021). These examples demonstrate how omics research can define complex molecular relationships. Therefore, metabolome studies provide an integrated depiction of numerous activities extending from the genome to metabolome as well as phenotypic traits when combined with other omics data including genomics, transcriptomics, and proteomics.
3. Multi-omics data integration for crop improvement
Crop improvement, including high yield and tolerance to biotic and abiotic stresses, is a long-term and time-consuming process in current plant breeding. The development of omics techniques and the rapid accumulation of omics data provide possible solution and foundational information. Approaches to integrating multi-omics information are being leveraged in plants for a better understanding of molecular mechanisms underlying complex traits and acceleration of the crop improvement (Figure 3). Here, we discuss in detail how to apply multi-omics strategies or tools to dissect the genetic basis/architecture of complex traits and predict key agronomic phenotypes in crops.
3.1. Genetic dissection of complex traits using multi-omics data
To dissect the genetic architecture of complex traits, candidate gene mining is an essential step. In the early days, gene mapping is usually difficult due to the lack of a high-quality crop genome. Luckily, the number of crop genome and pan-genome have increased dramatically in the past 20 years, bridging the genotype–phenotype gap easy. The most widely used strategies to identify genes responsible for complex traits are linkage mapping studies in family-based populations, association mapping in natural plant population, joint linkage-association mapping using both bi-parental and natural populations, and genomic selection between diversification population produced by plant domestication. In linkage analysis, genetic maps are usually constructed based on segregating mapping populations, such as doubled haploid (DH) lines, F2 populations, recombinant inbred lines (RILs), and backcross inbred lines, is to be used for quantitative trait locus (QTL) mapping. As an alternative to conventional linkage analysis, bulk segregation analysis (BSA) (Gao et al., 2022) coupled with next-generation sequencing (NGS) can be used in the genetic mapping of simple qualitative traits controlled by major genes when two sample pooling with extreme phenotypes exists.
For association analysis, we usually exploit genetic variation of the whole-genomic level in natural populations, to use linkage disequilibrium (LD) to map genes that cause a specific phenotype. In addition, a gene-based association approach, transcriptome-wide association studies (TWAS) can be used to investigate gene-trait associations using genetically regulated gene expression (Wainberg et al., 2019). The genes selected during domestication and artificial selection play an important role in modern crop breeding. Genome-wide selective sweep analysis is usually used to identify the major genes that enhance environmental adaptation (Lu et al., 2019; Li et al., 2021b), involved in morphotype changes (Kang et al., 2021), and selection for key agronomic traits (Hu et al., 2022).
However, gene identified as part of functional genomic studies, is just the beginning of the genetic dissection of complex traits. Its major disadvantages are: (1) The number of genes identified by QTL mapping is far beyond experimental expected. (2) It’s difficult to directly infer the causal relation between genes and final phenotypic traits due to the lack of evidence of middle omics. To address it, the integrating genomics with other omics data sets will reduce further candidate genes and benefit system analysis of gene function. Molecular phenotype is defined as a collection of molecular characteristics (i.e., mRNA transcripts, proteins, and metabolic compounds) in central dogma (Pramanik et al., 2020), which can be used for deep phenotyping. These phenotypic data permit a better understanding of end-to-end mechanistic from genes to final traits in crops (Figure 2). Recent advances in omics techniques allow for producing many molecular phenotypes at a larger-scale population level, making the association implementation of multi-omics molecular phenotypes easy (Table 2).
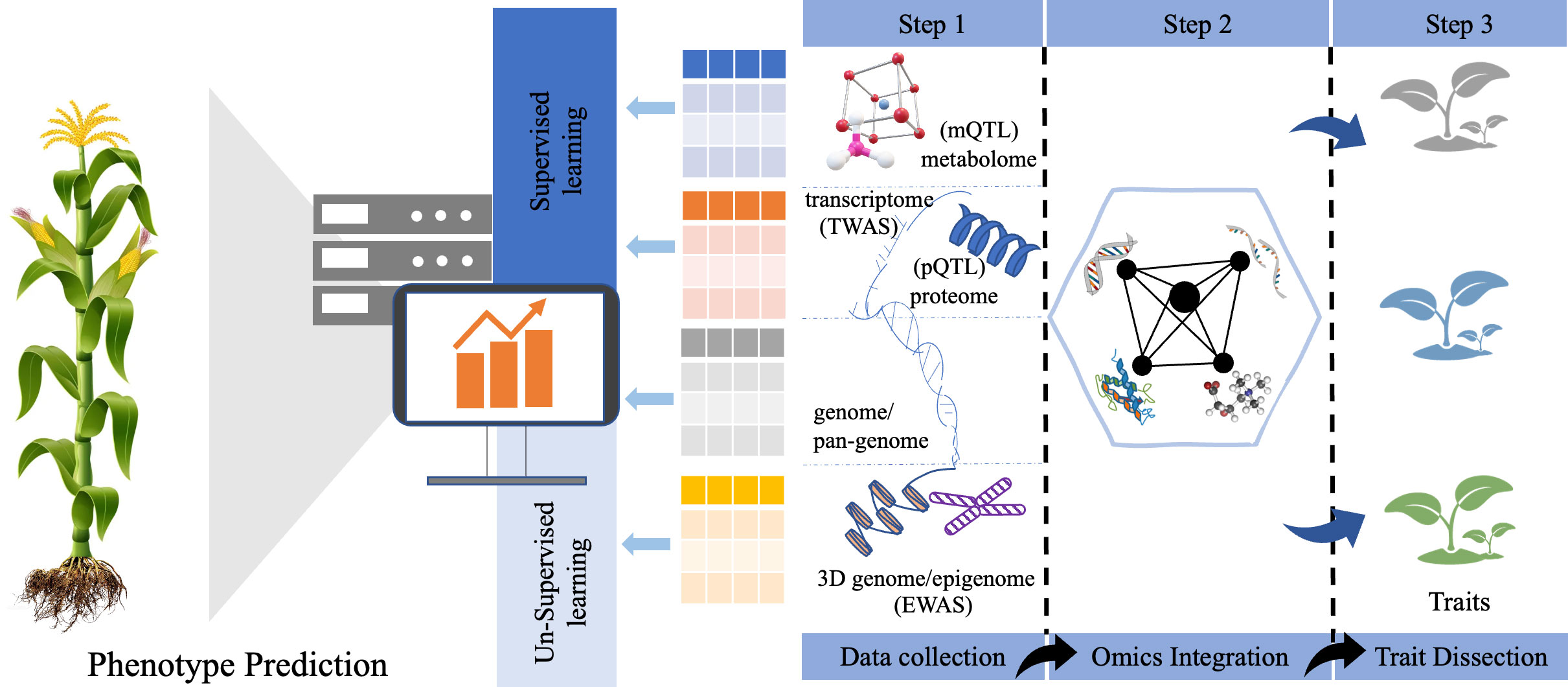
Figure 2 Strategies of multi-omics data integration between different layers of omics data to dissect the complex agronomic traits and phenotype prediction by using machine learning methods.
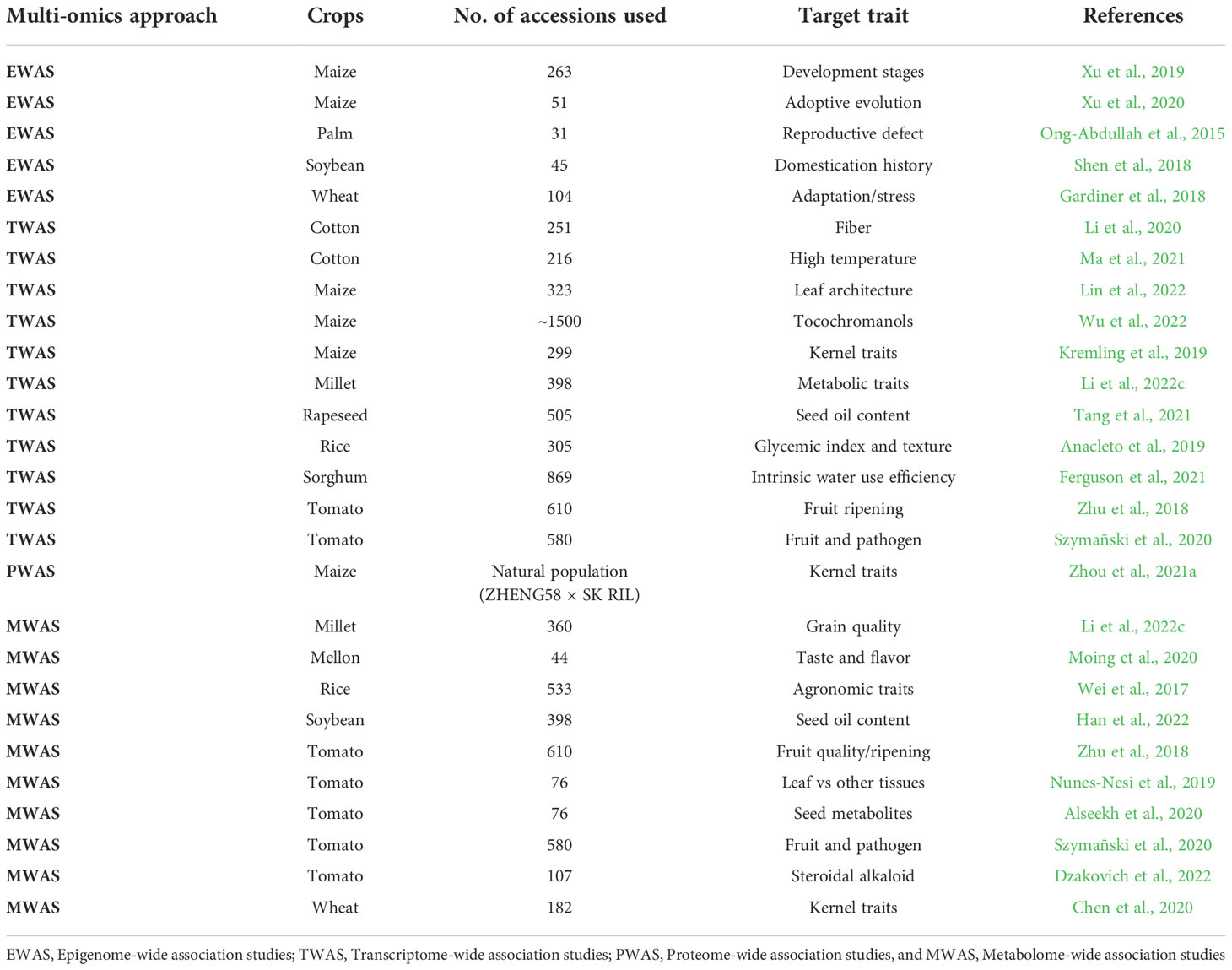
Table 2 Recent GWAS studies combined with RNA, protein, and metabolites to find the target traits in breeding programs.
3.2. GWAS + epigenome-wide association studies (epi-GWAS or EWAS)
Epigenome-wide association studies (EWAS) is a powerful method for identifying traits-associated epigenetic variation, specifically variation in DNA methylation, which has been used in human studies (Rakyan et al., 2011; Hawe et al., 2022). The integration of EWAS (i.e., methylation quantitative trait loci (meQTL)) with GWAS can help us to illuminate functional mechanisms underlying genetic variant-trait associations (Huan et al., 2019). However, there are still few EWAS applications based on the large-scale population level although the studies on GWAS have been widely used in plants, which present novel opportunities but also create new challenges for future crop improvement.
3.3. GWAS + transcriptome-wide association studies
GWAS and transcriptome-wide association studies (TWAS) are renowned techniques for locating genomic regions or genes for which DNA sequence or gene expression variations are associated with quantitative variability in a trait of interest (Ferguson et al., 2021). TWAS is a useful tool to complement GWAS since it explores the relationships between variations in transcript abundance and phenotypic variance. The most recent use of Fisher’s combination test to integrate GWAS and TWAS offered proof-of-concept by showing how it improved the efficiency with which identified associated genes could be “re-discovered” for well-known maize kernel traits (Kremling et al., 2019).
GWAS-implicated genes were further refined using expression QTL (eQTL) analysis through TWAS as a fine-mapping technique to find candidate genes. To identify the key genes affecting both the glycemic index and cooking properties of rice, a similar approach was used to determine the amylose content and ultimate stickiness (Anacleto et al., 2019). Cotton eQTL mapping was employed in a prior study to connect the regulatory variations to gene expression in fiber formation (Li et al., 2020). TWAS creates a direct relationship between gene expression and phenotype utilizing eQTL data, as contrasted to the functional study of leading SNPs or homology-based identification of relevant genes for GWAS locations (Zhu et al., 2018). These studies provided evidence that examining the intermediary transcriptome between variome and phenome can help us better understand the regulatory functions of genetic variations driving phenotypic change.
3.4. GWAS + proteome-wide association studies
Protein QTL (pQTL) analysis, which has produced proteome networks for clinical applications, has been used extensively in epidemiological research (Suhre et al., 2017), but it is still rarely used in plant-based GWAS studies. Moreover, it is important to comprehend the functional contexts of gene expression variation through modern crop breeding (Jiang et al., 2019). Recently, pQTL mapping of maize inbred lines was studied using an integrated multi-omics approach to identify the candidate genes associated with kernel size (Zhou et al., 2021a). These findings revealed that genes involved in signal transduction, amino acid metabolism, and an unidentified mechanism may control maize kernel size. Protein abundance variation in crops gains a new level of functional context owing to an integrated multi-omics approach combining pQTL analysis. The use of protein-based GWAS studies in crop breeding is still limited and needs to be utilized to discover the hidden candidates to improve the breeding programs.
3.5. GWAS + metabolome-wide association studies
Metabolite-based genome-wide association studies (mGWASs) or metabolite QTL (mQTL) made it possible to concurrently screen a large number of accessions for possible associations between their genomes and various metabolites and can provide insights on the genetic basis of complex traits and metabolic diversity (Peng et al., 2017; Fang and Luo, 2019; Li et al., 2022c). To discover new associations between genes and metabolites, mGWASs has been effectively used on several model plants and agronomic crops (Zhu et al., 2018; Zeng et al., 2020; Li et al., 2022c). The mGWAS analysis discovered notable variations in the core genetic architecture and the natural variability of the metabolites between different subgroups of foxtail millet (Li et al., 2022c). The degree of natural variability in metabolism and its genetic and biochemical regulation in tomatoes have been extensively elucidated by the integration of metabolomics, linkage mapping investigations, and mGWAS (Zhu et al., 2018). The metabolic breeding history of the tomato was recently discovered by an integrated analysis of eQTL and mQTL (Zhu et al., 2018). Understanding the mechanisms driving the evolution of metabolism is made possible by the mGWAS investigations. Despite the great progress that has been made in the mGWAS research, comprehensive understandings of metabolic control are still rare.
In addition, integration of eQTL, pQTL, and/or mQTL can be used to predict the quality trait to facilitate breeding as in the phenotypic prediction, multi-omics data modeling can explain that how a final phenotype is controlled by the differential expression of mRNA, protein, and metabolite in the central dogma. Similarly, Xu et al. (2016) found that the predictability of hybrid rice yield can be further increased by using these omics data. An earlier investigation utilizing potato tubers has demonstrated how a particular trait is related to gene expression, protein profiles, and metabolite data using the random forest regression method (Acharjee et al., 2016). These omics platforms can help us to develop strategies for integrating these omics data sets to forecast phenotypic features. This results in networks with relatively small sets of interlinked omics variables that are better able to predict the desired trait.
3.6. Exploiting multi-omics data for phenotypes prediction
Integrating data from multiple sources to create a model that can be used to predict complex traits and improve predictive accuracy is imperative. To date, an increasing number of statistical models including linear and nonlinear models, have been developed and widely used in phenotypes prediction. Genomic Best Linear Unbiased Prediction (GBLUP) is a linear model, which has been used extensively for genotype to phenotype prediction. To identify optimal prediction models, Wang et al. (2019) and Xu et al. (2016) have demonstrated that GBLUP generally yielded better results than other prediction methods using multi-omics data for selecting hybrid rice. Recently, Hu et al. (2021a) built a two-kernel linear model for multi-omics prediction of oat agronomic and seed nutritional traits in multi-environment trials and distantly related populations, show greater prediction accuracy than GBLUP. Similarly, as an extension of linear models, Linear mixed models (LMMs) have great potential multi-omics data prediction analysis (Weissbrod et al., 2016). However, a large amount of noise presented in high-dimensional omics data will limit predictive power of LMM. To filter out the noise, several novel methods were produced by extending the standard LMM and combining them with other prediction models (e.g., Bayesian sparse linear mixed model (BSLMM) (Zhou et al., 2013) and penalized linear mixed model with generalized method of moments estimator (MpLMMGMM) model (Wang and Wen, 2022)) have been proposed for modeling multi-omics data.
In nonlinear methods, machine learning (ML) is a new programming paradigm that can learn statistical rules from large-scale complex data, providing a scalable and interpretable framework for multi-omics integration and is usually applied to phenotypes prediction of crops in practice. The basic ML model can be divided into two major types supervised learning and unsupervised learning (Wang et al., 2020b). In supervised learning, classification (e.g., quality traits) and regression (e.g., quantitative traits) are the two major tasks to be predicted. Through the integration of multi-omics data using random forest, Acharjee et al. (2016) perform prediction of four quality traits in potato, including tuber flesh color, differential scanning calorimetry (DSC) onset, tuber shape and enzymatic discoloration. Deep learning (DL) is a specific subfield of machine learning which extensively used in the life science and health field for high-dimensional multi-source data integration and phenotype prediction (Chaudhary et al., 2018). Although DL has been rarely reported in multi-omics integration studies of the crop, it still has great potential and advantages for crop breeding in the future. Unsupervised learning is mostly used to seek the representations in data, such as clustering, association, and dimensionality reduction (DR). Among these, DR plays an important role in high dimensional biology due to reducing the number of random variables to consider. For instance, the algorithms of DR applied in maize contribute to the development of multi-omics data association studies (Liu et al., 2022b).
ML has revolutionized plant research to analyze and interpret large phenotypic data sets, as it is now possible to measure and correlate genotypic to phenotypic data at different levels. In addition, ML also made it possible to access large amounts of high-throughput data and solve problems in pertinent domains by using freely available software, and algorithms such as DL in prediction of protein structures (Senior et al., 2020). However, there are still many challenges such as large data sets, different species, genotypes, phenotypes, and variable environments, and the heterogeneous and fragmented nature of the data (van Dijk et al., 2021). Contrary to ML modules, statistical approaches were used to predict genotype to phenotype in traditional approaches. These approaches have been very effective since they produce good estimation values (p-value), easy to interpret and not complicated to use as compared with ML modules. However, similar issues are also addressed in advanced ML research, and solutions are being discovered in the plant research field (Azodi et al., 2020) to expand the multi-omics research in variable environments.
4. Challenges and future perspective
4.1. Environmental challenges
The pressure on agricultural systems worldwide is rising owing to the world’s exponentially increasing population, which is expected to hit 10 billion in the next 30 years (Scossa et al., 2021). The rising global temperature, which is expected to increase by 1.1 to 5.4°C by the end of this century, is also a challenge for agriculture (Tollefson, 2020). These frightening projections indicate that crops would experience heat stress and a rise in the frequency of droughts during their growing seasons (Rustgi et al., 2021; Raza et al., 2022b). Furthermore, climatic changes will probably increase the intensity of both single and combination abiotic stresses, including drought (Shahzad et al., 2021), cold, heat, salinity, and submergence (Anwar et al., 2021). Abiotic stresses are expected to worsen with the predicted climate-change scenarios by increasing the prevalence and severity of insects, pests, and pathogens as well as weed species proliferation and beneficial soil bacteria, as well as endangering essential plant pollinators (Zenda et al., 2021). The world’s most resource-constrained and populous regions would endure malnutrition and food insecurity due to the lower food yield brought on by these changes (Molotoks et al., 2021). Even though resistant and high-yielding varieties have evolved into intensive farming methods, it is crucial to consider the probability that crop yields, land use, and food demand will increase in the future (Voss-Fels et al., 2019).
Plant breeders are putting forth innovative strategies to deal with the growing need for food grains in the context of environmental problems such as rising global temperatures, irregular rainfall patterns, and concurrent changes in pest and disease attacks (Figure 3). Resource depletion (land degradation, nutrient insufficiency, water availability) makes it even more important to enhance agriculture sustainably and reduce its CO2 emission (Mir et al., 2022). Increased temperatures and higher CO2 levels, which are connected to the impoverished nutrient density of several staple crops, exacerbate the serious health issues encountered by billions of malnourished people in low-income regions (Macdiarmid and Whybrow, 2019). Plant breeders have developed better cultivars of various crop plants to achieve these goals, generally by utilizing traditional plant breeding techniques involving genetic crossing and selection for the desired features. However, this approach chiefly focused on the crop’s major gene pool (Kaiser et al., 2020). Recent developments have made it possible for molecular plant breeding to now uses integrated multi-omics approaches, allowing plant breeders to insert desired genetic variations in the crop genome from a larger gene pool with better accuracy and speed. Hence, molecular plant breeding techniques are being aggressively added to the traditional crop improvement methods to efficiently get the desired results (Mir et al., 2022).
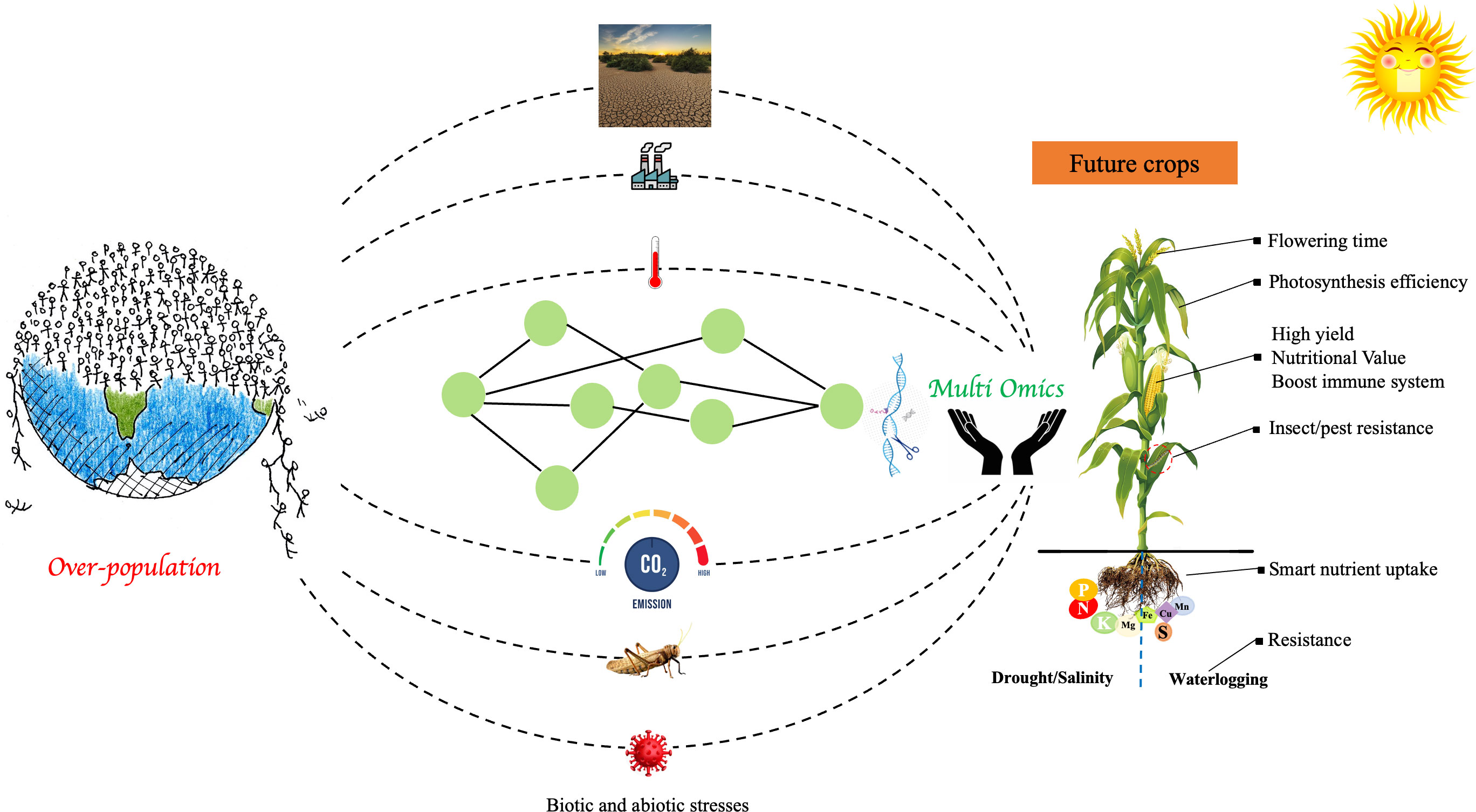
Figure 3 Schematic representation of the importance of integrated omics techniques in accelerating the development of future crops to feed the exponentially growing population. The development of climate-resilient and nutrient-rich crops is facilitated by integrated multi-omics approaches paired with genetics approaches, improved plant breeding, gene editing, and computational modeling techniques in a systems biology approach.
4.2. Challenges in multi-omics data integration
4.2.1. Data archiving and sharing
There is an increasing need for integrated, rigorous omics research, like many other scientific domains. Data archiving is crucial for the repeatability of both individual and integrated omics datasets, as well as for adhering to Findability, Accessibility, Interoperability, and Reusability (FAIR) principles (Krassowski et al., 2020). The demand for open sharing of scripts and codes for these analyses (MATLAB, Java, R, and Python, etc.) via websites like GitHub (https://www.github.com), where developers can share code, review code, manage projects, and develop software alongside other developers, is a potential solution. High-throughput molecular abundance data, primarily gene expression data, are archived and publicly distributed via the Gene Expression Omnibus (GEO) collection at the National Center for Biotechnology Information (NCBI) (Rangwala et al., 2021). There are a few integrated omics databases (Table 1) available that can help researchers to better comprehend the flow of genetic information (RNA, protein, metabolite and phenotype) of which a trait is influenced.
4.2.2. Data interpretation
The biggest challenge for omics datasets is the understanding of that large datasets. Biomarker discovery is one of the main goals of multi-dimensional omics approaches; regardless of the omics layer from which the key molecules are derived, the specificity and sensitivity of molecular biomarkers are crucial for the application of findings to breeding research and their practical application. Complex multi-layered network interpretation and curation are arduous, computationally, and time-consuming and demand in-depth knowledge of the biological system under study. Studies using an integrated omics approach frequently choose significant biological pathways and processes for hypothesis testing that are not physiologically relevant without applying a biological understanding of the system. Being familiar with understudied biological systems is crucial since it takes time and is often challenging to integrate the verification of datasets and networks (genes, proteins, and metabolites) for key biological pathways or mechanisms.
4.3. Future crops thanks to integrated multi-omics
4.3.1. Crops with stress resistance
Several potential solutions to these issues have been put forth, including the development of climate-resilient crops, increasing the efficiency with which natural resources are used, diversifying agricultural systems, and linking agricultural intensification to the preservation of natural ecosystems (Peng et al., 2020). The most efficient approach to adapt our agricultural system to handle climate change is to design crops with desired agronomic traits that are climate resistant (Table 3) (Kumari et al., 2020) to that specific environment and demand. Omics approaches now enable developing elite climate-smart cultivars with desired traits like high yield, abiotic and biotic stress tolerance, and nutritive quality (Naeem et al., 2022; Reimer et al., 2022).
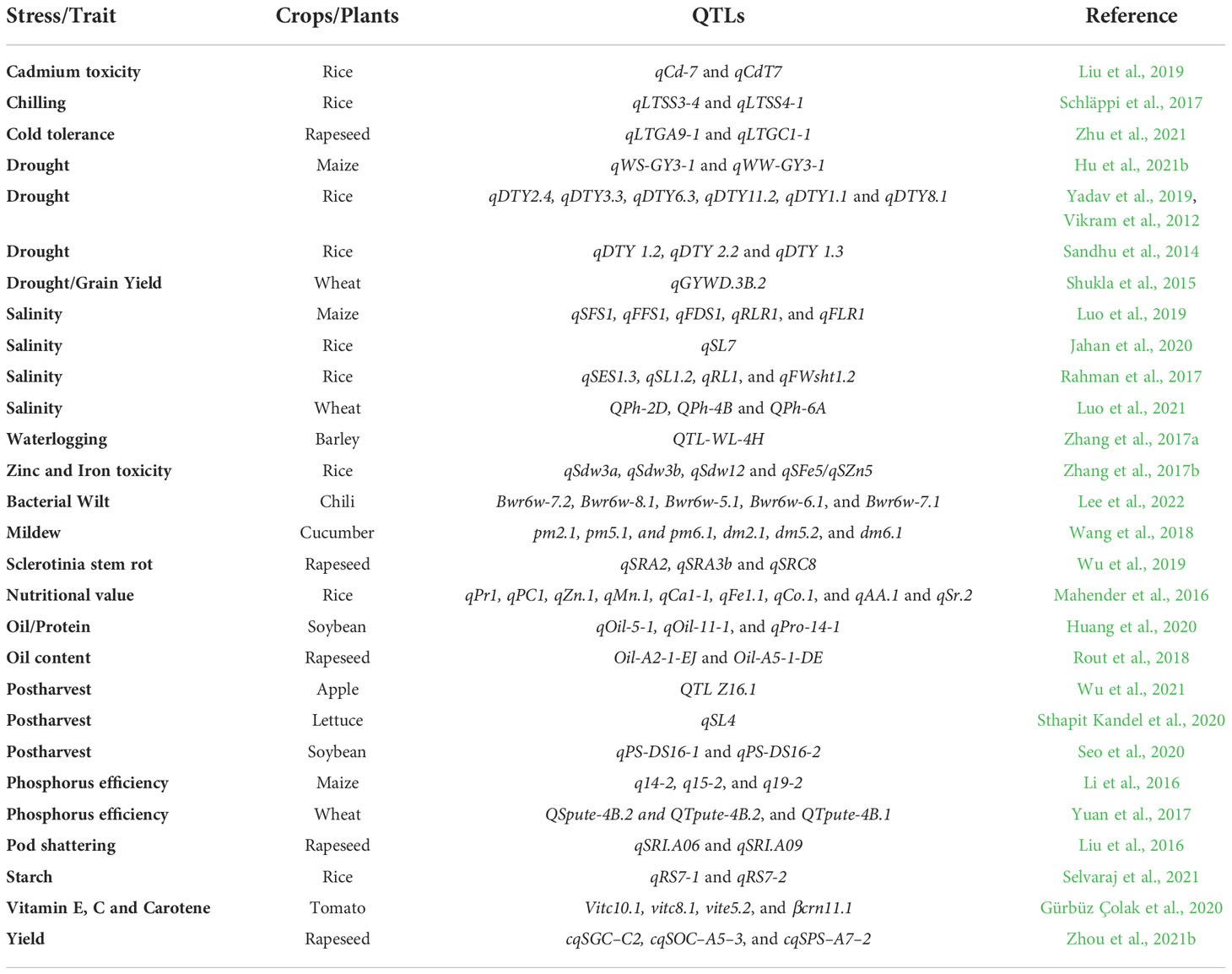
Table 3 List of stress (abiotic and biotic), nutrition, quality, and post-harvest related QTLs for future crop improvements.
To accelerate genetic advancement and reduce the effects of climate change on crop yield, advanced integrative breeding platforms are required (Bevan et al., 2017). A single reference genome cannot provide the full range of genetic variation needed for crop breeding. Therefore pan-genome research could help us to understand the genome composition of the population, whether cultivated, landrace, or wild progenitors. The PAVs in various species have been identified by a variety of biotic stress-responsive genes (Cook et al., 2012). The pan-genome of B. napus was examined to characterize disease-resistant genes, and 106 potential QTLs associated with blackleg resistance were found recently (Dolatabadian et al., 2020).
The developmental processes, epigenetic markers like histone modifications and DNA methylation influence how plants respond to environmental signals. The patterns of DNA methylation and subsequent differential expression of genes related to stress have all been shown to be influenced by a variety of biotic (Correa et al., 2020) and abiotic stress conditions such as water stress, nutrient deficiency, temperature stress, as well as in vitro stresses (such as tissue culture) (Scossa et al., 2021). If epigenetic processes also control plant phenotypic variation, then at least in part, hence epialleles should be considered when developing crop enhancement strategies.
On the other hand, meiosis has the potential to transfer stress-induced DNA methylation states, leading to some types of transgenerational memory (Dar et al., 2022), even though paramutation-like activities might complicate inheritance patterns (Minow et al., 2022). The availability of several important natural or induced epigenetic variations for agriculture, such as rice, maize, and soybean (Lloyd and Lister, 2022), demonstrates how screening epialleles can significantly increase the genetic variation that breeders can exploit to improve agricultural practices.
Moreover, integrated transcriptome and metabolome studies revealed that canola seedlings could survive the impacts of alkaline-salt stress by controlling the metabolism of organic acids and amino acids in roots (Wang et al., 2021). Another study has demonstrated that ZmUGT genes may control SA (salicylic acid) homeostasis at transcriptional and metabolic levels, contributing to maize pathogen defense response (Ge et al., 2021). Integrated transcriptome and proteomic datasets to identify pathways driving S. subterranea resistance in potato roots were examined, and this multi-omics approach discovered an increase in glutathione metabolism at the RNA and protein levels in the resistant cultivars (Balotf et al., 2022). Therefore, integrated omics techniques enable detailed comprehension of complicated physiological and molecular mechanisms underlying several crucial traits of agronomic value (Singh et al., 2020), integrating large molecular datasets and the developing predictive models for those key traits (Scossa et al., 2021). Developing climate-smart cultivars require a comprehensive, critical, and effective approach (Pazhamala et al., 2021). When it comes to generating climate-ready crops, these multi-omics-generated data will need to be combined with contemporary plant breeding and gene editing techniques (Gogolev et al., 2021). These high throughput sequencing technologies and integrated multi-omics platforms will help the breeders to develop climate-smart elite cultivars with desired characteristics to fight challenging climatic conditions.
4.3.2. Crops with better nutrition
Since crops are the main source of vital nutrients like vitamins, iron, zinc, folate, fiber, limiting access to and consumption of plant-based diets can have detrimental effects on one’s health. For example, it can increase the risk of non-communicable diseases (NCD) and lead to increased nutritional deficiencies that may be challenging to ameliorate through food substitution (Scheelbeek et al., 2018). In the context of such climate change, enhancing crop nutritional quality through breeding, agronomic management, or transgenic techniques becomes essential. A promising, affordable, and sustainable method for ensuring that millions of people have healthy diets is the genetic biofortification of crops through breeding (Zenda et al., 2021).
Identification of the key genes, metabolic pathways, and QTLs that assist in understanding the genetic architecture of plant nutrient uptake is crucial for the successful improvement of crop nutritional quality. These nutritional qualities have been targeted using a variety of omics approaches, the results of which have improved GAB initiatives (Roorkiwal et al., 2021). Potential QTLs have been found in the major cereals related to nutrition such as rice (Park et al., 2019; Calayugan et al., 2020), wheat (Krishnappa et al., 2017; Ruan et al., 2020), and maize (Yang et al., 2016).
In recent work, GWAS was used to conduct comparative genomics to identify the genomic regions controlling the nutritional content of grains. In a recent GWAS study, 190 genotypes of Eleusine coracana were used to discover the genetic regions affecting grain nutritional content (Fe, Zn, Mg, Ca, Na, K, and proteins) (Puranik et al., 2020). Enhancing nutrient bioavailability requires a thorough understanding of the mechanisms behind crop nutrient absorption, transport, and assimilation into seeds because numerous genes and complex metabolic pathways are involved. Using omics techniques, it is feasible to comprehend the genes and metabolic pathways involved in nutrient uptake or biosynthesis, absorption, transport, assimilation, and storage (Zenda et al., 2021). For instance, a metabolic technique has been carried out to target carotenoid biosynthesis pathways and maximize the level of b-carotene in crops (rice, maize, and potato) considering that beta carotene and carotenoids are the primary precursors to vitamin A (Qutub et al., 2021; Sharma et al., 2021).
Since aflatoxin contamination has serious effects on human and animal health (Ojiewo et al., 2020), thus transcriptomics and metabolomic investigations have been performed to elucidate the aflatoxin biosynthesis in peanuts to develop the resistant varieties. The high oleic acid level is a pivotal quality attribute determining peanut flavor, stability, shelf life, and nutritional value. Therefore, high oleic acid and low linoleic acid in peanut cultivars have been developed using a various genetic technique, including QTL analysis, molecular markers, and gene editing (Amoah et al., 2020; Ojiewo et al., 2020). Therefore, these genomic advances (genomics, transcriptomics, proteomics, metabolomics, and gene editing) have made it possible to incorporate multiple agronomic traits into a single cultivar. Future developments in integrated genomic approaches are anticipated to make it easier to detect important genetic variations, identify key genes driving priority traits (high yield, stress resistance, and high-level micronutrients and proteins), and introduce those priority traits into elite cultivars, boosting economic and the nutritional value of those cultivars.
5. Conclusion and perspective
Recent developments in sequencing and phenotyping technologies have made it possible to extract distinctive genetic variations from the diverse spectrum of germplasm for use in plant breeding. To demonstrate how various omics techniques have anchored agricultural research and development projects, we have described several pertinent instances in this context. The identification of candidate genes, proteins, and metabolites encompassing numerous quantitative and quality traits of agronomic significance in major crops have been made possible primarily using these omics’ techniques, especially genomics, transcriptomics, proteomics, and metabolomics to study how plants respond to different biotic and abiotic stresses. Additionally, machine learning contributed to integrating the GWAS to produce a full set of data on genetic variation to predict the phenotypic parameters influencing the improvement of quality, quantity, or stress tolerance. Moreover, GWAS at the epigenome, transcriptome, protein, and metabolic levels could help the scientists to identify the detrimental and beneficial alleles for crop breeding. Through molecular breeding, or genetic engineering techniques, several reported candidate genes and metabolic pathways have been implemented in breeding programs. Integrated omics platforms are the latest avenue with significant potential in crop breeding to bridge the gap between environmental challenges and food security. Researchers will be able to implement innovative methods in forward/reverse genetics and breeding programs using an integrative omics platform that provides access to entire bioinformatics data. In conclusion, omics-based breeding research and cutting-edge technologies significantly impact crop yield, nutritional value enhancement, and stress tolerance to feed the globe. Multidisciplinary partnerships between plant scientists, computational biologists, breeding corporates, and farmers should be established to share knowledge and build a community to discover novel and ground-breaking insights in crop breeding using multi-omics techniques.
Author contributions
CQ, KL: Conceptualization, Methodology. UM, XL, YF: Investigation, Writing - original draft. WC, YN and JL: Investigation. UM, CQ, KL: Visualization, Writing - reviewing and editing. All authors contributed to the article and approved the submitted version.
Funding
This work was supported by the National Natural Science Foundation of China [32272111, 32250410284, 31830067], the Talent Project of Chongqing Natural Science Foundation [cstc2021ycjh-bgzxm0033], and Germplasm Creation Special Program of Southwest University.
Conflict of interest
The authors declare that the research was conducted in the absence of any commercial or financial relationships that could be construed as a potential conflict of interest.
Publisher’s note
All claims expressed in this article are solely those of the authors and do not necessarily represent those of their affiliated organizations, or those of the publisher, the editors and the reviewers. Any product that may be evaluated in this article, or claim that may be made by its manufacturer, is not guaranteed or endorsed by the publisher.
References
Abdelrahman, M., El-Sayed, M. A., Hashem, A., Abd-Allah, E. F., Alqarawi, A. A., Burritt, D. J., et al. (2018). Metabolomics and transcriptomics in legumes under phosphate deficiency in relation to nitrogen fixation by root nodules. Front. Plant Sci. 9, 922. doi: 10.3389/fpls.2018.00922
Acharjee, A., Kloosterman, B., Visser, R. G., Maliepaard, C. (2016). Integration of multi-omics data for prediction of phenotypic traits using random forest. BMC Bioinform. 17, 363–373. doi: 10.1186/s12859-016-1043-4
Ackah, M., Guo, L., Li, S., Jin, X., Asakiya, C., Aboagye, E. T., et al. (2022). DNA Methylation changes and its associated genes in mulberry (Morus alba l.) Yu711 response to drought stress using MethylRAD sequencing. Plants 11, 190. doi: 10.3390/plants11020190
Aizat, W. M., Hassan, M. (2018). ““Proteomics in systems biology,”,” in Omics applications for systems biology. advances in experimental medicine and biology. Eds. Aizat, W., Goh, H. H., Baharum, S. (Cham: Springer), 31–49. doi: 10.1007/978-3-319-98758-3_3
Al-Lawati, A., Al-Bahry, S., Victor, R., Al-Lawati, A. H., Yaish, M. W. (2016). Salt stress alters DNA methylation levels in alfalfa (Medicago spp). Genet. Mol. Res. 15, 15018299. doi: 10.4238/gmr.15018299
Alseekh, S., Ofner, I., Liu, Z. Y., Osorio, S., Vallarino, J., Last, R. L., et al. (2020). Quantitative trait loci analysis of seed-specialized metabolites reveals seed-specific flavonols and differential regulation of glycoalkaloid content in tomato. Plant J. 103, 2007–2024. doi: 10.1111/tpj.14879
Amoah, R. A., Akromah, R., Asibuo, J. Y., Wireko-Kena, A., Asare, K. B., Lamptey, M., et al. (2020). Mode of inheritance and combining ability of oleic acid content in groundnut (Arachis hypogaea l.). Ecol. Genet. Genom. 17, 100064. doi: 10.1016/j.egg.2020.100064
Anacleto, R., Badoni, S., Parween, S., Butardo, V. M., Misra, G., Cuevas, R. P., et al. (2019). Integrating a genome wide association study with a large scale transcriptome analysis to predict genetic regions influencing the glycaemic index and texture in rice. Plant Biotechnol. J. 17, 1261–1275. doi: 10.1111/pbi.13051
Anwar, K., Joshi, R., Dhankher, O. P., Singla-Pareek, S. L., Pareek, A. (2021). Elucidating the response of crop plants towards individual, combined and sequentially occurring abiotic stresses. Int. J. Mol. Sci. 22, 6119. doi: 10.3390/ijms221161
Azodi, C. B., Tang, J., Shiu, S.-H. (2020). Opening the black box: Interpretable machine learning for geneticists. Trends Genet. 36, 442–455. doi: 10.1016/j.tig.2020.03.005
Balotf, S., Wilson, R., Nichols, D. S., Tegg, R. S., Wilson, C. R. (2022). Multi-omics reveals mechanisms of resistance to potato root infection by Spongospora subterranea. Sci. Rep. 12, 10804. doi: 10.1038/s41598-022-14606-y
Bayer, P. E., Petereit, J., Durant, É., Monat, C., Rouard, M., Hu, H., et al. (2022). Wheat panache: A pangenome graph database representing presence–absence variation across sixteen bread wheat genomes. Plant Genome 15, e20221. doi: 10.1002/tpg2.20221
Bevan, M. W., Uauy, C., Wulff, B. B., Zhou, J., Krasileva, K., Clark, M. D. (2017). Genomic innovation for crop improvement. Nature 543, 346–354. doi: 10.1038/nature22011
Block, A. K., Hunter, C. T., Sattler, S. E., Rering, C., McDonald, S., Basset, G. J. (2020). Fighting on two fronts: Elevated insect resistance in flooded maize. Plant Cell Environ. 43, 223–234. doi: 10.1111/pce.13642
Calayugan, M. I. C., Formantes, A. K., Amparado, A., Descalsota-Empleo, G. I., Nha, C. T., Inabangan-Asilo, et al. (2020). Genetic analysis of agronomic traits and grain iron and zinc concentrations in a doubled haploid population of rice (Oryza sativa l.). Sci. Rep. 10, 2283. doi: 10.1038/s41598-020-59184-z
Chalhoub, B., Denoeud, F., Liu, S., Parkin, I. A., Tang, H., Wang, X., et al. (2014). Plant genetics. early allopolyploid evolution in the post-neolithic brassica napus oilseed genome. Science 345, 950–953. doi: 10.1126/science.1253435
Chaudhary, K., Poirion, O. B., Lu, L., Garmire, L. X. (2018). Deep learningbased multi-omics integration robustly predicts survival in liver cancer. Clin. Cancer Res. 24, 1248–1259. doi: 10.1158/1078-0432.CCR-17-0853
Chen, X., Bhadauria, V., Ma, B. (2018). ChIP-seq: a powerful tool for studying protein–DNA interactions in plants. Curr. Issues Mol. Biol. 27, 171–180. doi: 10.21775/cimb.027.171
Chen, J., Hu, X., Shi, T., Yin, H., Sun, D., Hao, Y., et al. (2020). Metabolite-based genome-wide association study enables dissection of the flavonoid decoration pathway of wheat kernels. Plant Biotechnol. J. 18, 1722–1735. doi: 10.1111/pbi.13335
Cook, D. E., Lee, T. G., Guo, X., Melito, S., Wang, K., Bayless, A. M., et al. (2012). Copy number variation of multiple genes at Rhg1 mediates nematode resistance in soybean. Science 338, 1206–1209. doi: 10.1126/science.1228746
Coppola, M., Diretto, G., Digilio, M. C., Woo, S. L., Giuliano, G., Molisso, D., et al. (2019). Transcriptome and metabolome reprogramming in tomato plants by Trichoderma harzianum strain T22 primes and enhances defense responses against aphids. Front. Physiol. 10. doi: 10.3389/fphys.2019.00745
Correa, R. L., Sanz-Carbonell, A., Kogej, Z., Muller, S. Y., Ambros, S., Lopez-Gomollon, S., et al. (2020). Viral fitness determines the magnitude of transcriptomic and epigenomic reprograming of defense responses in plants. Mol. Biol. Evol. 37, 1866–1881. doi: 10.1093/molbev/msaa091
Dar, F. A., Mushtaq, N. U., Saleem, S., Rehman, R. U., Dar, T. U. H., Hakeem, K. R. (2022). Role of epigenetics in modulating phenotypic plasticity against abiotic stresses in plants. Int. J. Genomics 2022, 1092894. doi: 10.1155/2022/1092894
Darracq, A., Vitte, C., Nicolas, S., Duarte, J., Pichon, J.-P., Mary-Huard, T., et al. (2018). Sequence analysis of European maize inbred line F2 provides new insights into molecular and chromosomal characteristics of presence/absence variants. BMC Genom. 19, 1–20. doi: 10.1186/s12864-018-4490-7
Dasgupta, P., Prasad, P., Bag, S. K., Chaudhuri, S. (2022). Dynamicity of histone H3K27ac and H3K27me3 modifications regulate the cold-responsive gene expression in Oryza sativa l. ssp. indica. Genomics 114, 110433. doi: 10.1016/j.ygeno.2022.110433
Della Coletta, R., Qiu, Y., Ou, S., Hufford, M. B., Hirsch, C. N. (2021). How the pan-genome is changing crop genomics and improvement. Genome Biol. 22, 3. doi: 10.1186/s13059-020-02224-8
Dolatabadian, A., Bayer, P. E., Tirnaz, S., Hurgobin, B., Edwards, D., Batley, J. (2020). Characterization of disease resistance genes in the brassica napus pangenome reveals significant structural variation. Plant Biotechnol. J. 18, 969–982. doi: 10.1111/pbi.13262
Dossa, K., Diouf, D., Wang, L., Wei, X., Zhang, Y., Niang, M., et al. (2017). The emerging oilseed crop sesamum indicum enters the “Omics” Era. Front. Plant Sci. 8. doi: 10.3389/fpls.2017.01154
Droc, G., Lariviere, D., Guignon, V., Yahiaoui, N., This, D., Garsmeur, O., et al. (2013). The banana genome hub. Database 2013, bat035. doi: 10.1093/database/bat035
Du, J., Verzaux, E., Chaparro-Garcia, A., Bijsterbosch, G., Keizer, L. C. P., Zhou, J., et al. (2015). Elicitin recognition confers enhanced resistance to phytophthora infestans in potato. Nat. Plants 1, 15034. doi: 10.1038/NPLANTS.2015.34
Dzakovich, M. P., Francis, D. M., Cooperstone, J. L. (2022). Steroidal alkaloid biosynthesis is coordinately regulated and differs among tomatoes in the red-fruited clade. Plant Genome 15, e20192. doi: 10.1002/tpg2.20192
Fang, C., Luo, J. (2019). Metabolic GWAS-based dissection of genetic bases underlying the diversity of plant metabolism. Plant J. 97, 91–100. doi: 10.1111/tpj.14097
Farooq, M. S., Uzair, M., Raza, A., Habib, M., Xu, Y., Yousuf, M., et al. (2022). Uncovering the research gaps to alleviate the negative impacts of climate change on food security: a review. Front. Plant Sci. 13. doi: 10.3389/fpls.2022
Ferguson, J. N., Fernandes, S. B., Monier, B., Miller, N. D., Allen, D., Dmitrieva, A., et al. (2021). Machine learning-enabled phenotyping for GWAS and TWAS of WUE traits in 869 field-grown sorghum accessions. Plant Physiol. 187, 1481–1500. doi: 10.1093/plphys/kiab346
Francia, E., Morcia, C., Pasquariello, M., Mazzamurro, V., Milc, J. A., Rizza, F., et al. (2016). Copy number variation at the HvCBF4- HvCBF2 genomic segment is a major component of frost resistance in barley. Plant Mol. Biol. 92, 161–175. doi: 10.1007/s11103-016-0505-4
Fu, X. Z., Zhang, X. Y., Qiu, J. Y., Zhou, X., Yuan, M., He, Y. Z., et al. (2019). Whole-transcriptome RNA sequencing reveals the global molecular responses and ceRNA regulatory network of mRNAs, lncRNAs, miRNAs and circRNAs in response to copper toxicity in ziyang xiangcheng (Citrus junos sieb. ex Tanaka). BMC Plant Biol. 19, 509. doi: 10.1186/s12870-019-2087-1
Gabur, I., Chawla, H. S., Snowdon, R. J., Parkin, I. A. P. (2019). Connecting genome structural variation with complex traits in crop plants. Theor. Appl. Genet. 132, 733–750. doi: 10.1007/s00122-018-3233-0
Gamuyao, R., Chin, J. H., Pariasca-Tanaka, J., Pesaresi, P., Catausan, S., Dalid, C., et al. (2012). The protein kinase Pstol1 from traditional rice confers tolerance of phosphorus deficiency. Nature 488, 535–539. doi: 10.1038/nature11346
Gao, Y., Du, L., Ma, Q., Yuan, Y., Liu, J., Song, H., et al. (2022). Conjunctive analyses of bulk segregant analysis sequencing and bulk segregant RNA sequencing to identify candidate genes controlling spikelet sterility of foxtail millet. Front. Plant Sci. 13. doi: 10.3389/fpls.2022.842336
Gardiner, L. J., Joynson, R., Omony, J., Rusholme-Pilcher, R., Olohan, L., Lang, D., et al. (2018). Hidden variation in polyploid wheat drives local adaptation. Genome Res. 28, 1319–1332. doi: 10.1101/gr.233551.117
Ge, C., Wang, Y. G., Lu, S., Zhao, X. Y., Hou, B. K., Balint-Kurti, P. J., et al. (2021). Multi-omics analyses reveal the regulatory network and the function of ZmUGTs in maize defense response. Front. Plant Sci. 12. doi: 10.3389/fpls.2021.738261
Gobena, D., Shimels, M., Rich, P. J., Ruyter-Spira, C., Bouwmeester, H., Kanuganti, S., et al. (2017). Mutation in sorghum LOW GERMINATION STIMULANT 1 alters strigolactones and causes striga resistance. Proc. Natl. Acad. Sci. U.S.A. 114, 4471–4476. doi: 10.1073/pnas.1618965114
Goff, S. A., Ricke, D., Lan, T. H., Presting, G., Wang, R., Dunn, M., et al. (2002). A draft sequence of the rice genome (Oryza sativa l. ssp. japonica). Science 296, 92–100. doi: 10.1126/science.1068275
Gogolev, Y. V., Ahmar, S., Akpinar, B. A., Budak, H., Kiryushkin, A. S., Gorshkov, V. Y., et al. (2021). OMICs, epigenetics, and genome editing techniques for food and nutritional security. Plants 10, 1423. doi: 10.3390/plants10071423
Großkinsky, D. K., Syaifullah, S. J., Roitsch, T. (2017). Integration of multiomics techniques and physiological phenotyping within a holistic phenomics approach to study senescence in model and crop plants. J. Exp. Bot. 66825–844. doi: 10.1093/jxb/erx333
Gürbüz Çolak, N., Eken, N. T., Ülger, M., Frary, A., Doğanlar, S. (2020). Mapping of quantitative trait loci for antioxidant molecules in tomato fruit: Carotenoids, vitamins c and e, glutathione and phenolic acids. Plant Sci. 292, 110393. doi: 10.1016/j.plantsci.2019.110393
Gu, D., Yang, J., Wu, S., Liao, Y., Zeng, L., Yang, Z. (2021). Epigenetic regulation of the phytohormone abscisic acid accumulation under dehydration stress during postharvest processing of tea (Camellia sinensis). J. Agric. Food Chem. 69, 1039–1048. doi: 10.1021/acs.jafc.0c07220
Han, B., Xu, W., Ahmed, N., Yu, A., Wang, Z., Liu, A. (2020). Changes and associations of genomic transcription and histone methylation with salt stress in castor bean. Plant Cell Physiol. 61, 1120–1133. doi: 10.1093/pcp/pcaa037
Han, X., Zhang, Y. W., Liu, J. Y., Zuo, J. F., Zhang, Z. C., Guo, L., et al. (2022). 4D genetic networks reveal the genetic basis of metabolites and seed oil-related traits in 398 soybean RILs. Biotechnol. Biofuels Bioprod 15, 92. doi: 10.1186/s13068-022-02191-1
Hao, P. Y., Feng, Y. L., Zhou, Y. S., Song, X. M., Li, H. L., Ma, Y., et al. (2018). Schaftoside interacts with NlCDK1 protein: a mechanism of rice resistance to brown planthopper, Nilaparvata lugens. Front. Plant Sci. 9. doi: 10.3389/fpls.2018.00710
Hawe, J. S., Wilson, R., Schmid, K. T., Zhou, L., Lakshmanan, L. N., Lehne, B. C., et al. (2022). Genetic variation influencing DNA methylation provides insights into molecular mechanisms regulating genomic function. Nat. Genet. 54, 18–29. doi: 10.1038/s41588-021-00969-x
Huang, J., Ma, Q., Cai, Z., Xia, Q., Li, S., Jia, J., et al. (2020). Identification and mapping of stable QTLs for seed oil and protein content in soybean [Glycine max (L.) merr.]. J. Agric. Food Chem. 68, 6448–6460. doi: 10.1021/acs.jafc.0c01271
Huan, T., Joehanes, R., Song, C., Peng, F., Guo, Y., Mendelson, M., et al. (2019). Genome-wide identification of DNA methylation QTLs in whole blood highlights pathways for cardiovascular disease. Nat. Commun. 10, 4267. doi: 10.1038/s41467-019-12228-z
Hu, H., Campbell, M. T., Yeats, T. H., Zheng, X., Runcie, D. E., Covarrubias-Pazaran, G., et al. (2021a). Multi-omics prediction of oat agronomic and seed nutritional traits across environments and in distantly related populations. Theor. Appl. Genet. 134, 4043–4054. doi: 10.1007/s00122-021-03946-4
Hu, J., Chen, B., Zhao, J., Zhang, F., Xie, T., Xu, K., et al. (2022). Genomic selection and genetic architecture of agronomic traits during modern rapeseed breeding. Nat. Genet. 54, 694–704. doi: 10.1038/s41588-022-01055-6
Hufford, M., Seetharam, A., Woodhouse, M., Chougule, K., Ou, S., Liu, J., et al. (2021). De novo assembly, annotation, and comparative analysis of 26 diverse maize genomes. Science 373, 655–662. doi: 10.1126/science.abg5289
Hu, X., Wang, G., Du, X., Zhang, H., Xu, Z., Wang, J., et al. (2021b). QTL analysis across multiple environments reveals promising chromosome regions associated with yield-related traits in maize under drought conditions. Crop J. 9, 759–766. doi: 10.1016/j.cj.2020.10.004
Jahan, N., Zhang, Y., Lv, Y., Song, M., Zhao, C., Hu, H., et al. (2020). QTL analysis for rice salinity tolerance and fine mapping of a candidate locus qSL7 for shoot length under salt stress. Plant Growth Reg. 90, 307–319. doi: 10.1007/s10725-019-00566-3
James, D., Rennya, P. R., Mallavarapu, M. D., Panigrahi, R. C., Patel, H. K. (2021). Integrating pan-omics data in a systems approach for crop improvement: opportunities and challenges. Omics Technol. Sustain. Agric. Global Food Secur. Vol II), 215–246. doi: 10.1007/978-981-16-2956-3_8
Jiang, L. G., Li, B., Liu, S. X., Wang, H. W., Li, C. P., Song, S. H., et al. (2019). Characterization of proteome variation during modern maize breeding. Mol. Cell Proteomics 18, 263–276. doi: 10.1074/mcp.RA118.001021
Jonczyk, M., Sobkowiak, A., Trzcinska-Danielewicz, J., Sowinski, P. (2020). Chromatin-level differences elucidate potential determinants of contrasting levels of cold sensitivity in maize lines. Plant Mol. Biol. Rep. 39, 335–350. doi: 10.1007/s11105-020-01254-7
Kaiser, N., Douches, D., Dhingra, A., Glenn, K. C., Herzig, P. R., Stowe, E. C., et al. (2020). The role of conventional plant breeding in ensuring safe levels of naturally occurring toxins in food crops. Trends Food Sci. Technol. 100, 51–66. doi: 10.1016/j.tifs.2020.03.042
Kang, L., Qian, L., Zheng, M., Chen, L., Chen, H., Yang, L., et al. (2021). Genomic insights into the origin, domestication and diversification of Brassica juncea. Nat. Genet. 53, 1392–1402. doi: 10.1038/s41588-021-00922-y
Kashima, Y., Sakamoto, Y., Kaneko, K., Seki, M., Suzuki, Y., Suzuki, A. (2020). Single-cell sequencing techniques from individual to multiomics analyses. Exp. Mol. Med. 52, 1419–1427. doi: 10.1038/s12276-020-00499-2
Kaul, S., Koo, H. L., Jenkins, J., Rizzo, M., Rooney, T., Tallon, L. J., et al. (2000). Analysis of the genome sequence of the flowering plant Arabidopsis thaliana. Nature 408, 796–815. doi: 10.1038/35048692
Krassowski, M., Das, V., Sahu, S. K., Misra, B. B. (2020). State of the field in multi-omics research: From computational needs to data mining and sharing. Front. Genet. 11. doi: 10.3389/fgene.2020.610798
Kremling, K. A., Diepenbrock, C. H., Gore, M. A., Buckler, E. S., Bandillo, N. B, (2019). Transcriptome-wide association supplements genome-wide association in Zea mays. Genes Genomes Genet. 9, 3023–3033. doi: 10.1534/g3.119.400549
Kress, W. J., Soltis, D. E., Kersey, P. J., Wegrzyn, J. L., Leebens-Mack, J. H., Gostel, M. R., et al. (2022). Green plant genomes: What we know in an era of rapidly expanding opportunities. Proc. Natl. Acad. Sci. 119, e2115640118. doi: 10.1073/pnas.2115640118
Krishnappa, G., Singh, A. M., Chaudhary, S., Ahlawat, A. K., Singh, S. K., Shukla, R. B., et al. (2017). Molecular mapping of the grain iron and zinc concentration, protein content and thousand kernel weight in wheat (Triticum aestivum l.). PloS One 12, e0174972. doi: 10.1371/journal.pone.0174972
Kumari, P., Rastogi, A., Yadav, S. (2020). Effects of heat stress and molecular mitigation approaches in orphan legume, chickpea. Mol. Biol. Rep. 47, 4659–4670. doi: 10.1007/s11033-020-05358-x
Kumar, R., Sharma, V., Suresh, S., Ramrao, D. P., Veershetty, A., Kumar, S., et al. (2021). Understanding omics driven plant improvement and de novo crop domestication: some examples. Front. Genet. 12. doi: 10.3389/fgene.2021.637141
Lai, X., Bazin, J., Webb, S., Crespi, M., Zubieta, C., Conn, S. J. (2018). ““CircRNAs in plants,”,” in Circular RNAs. advances in experimental medicine and biology, vol. 1087 . Ed. Xiao, J. (Singapore: Springer), 370.
Lee, S., Chakma, N., Joung, S., Lee, J. M., Lee, J. (2022). QTL mapping for resistance to bacterial wilt caused by two isolates of Ralstonia solanacearum in chili pepper (Capsicum annuum l.). Plants 11, 1551. doi: 10.3390/plants11121551
Li, B., Cai, H., Liu, K., An, B., Wang, R., Yang, F., et al. (2022b). DNA Methylation alterations and their association with high temperature tolerance in rice anthesis. J. Plant Growth Regul., 1–15. doi: 10.1007/s00344-022-10586-5
Li, Y., Cao, K., Li, N., Zhu, G., Fang, W., Chen, C., et al. (2021b). Genomic analyses provide insights into peach local adaptation and responses to climate change. Genome Res. 31, 592–606. doi: 10.1101/gr.261032.120
Li, X., Gao, J., Song, J., Guo, K., Hou, S., Wang, X., et al. (2022c). Multi-omics analyses of 398 foxtail millet accessions reveal genomic regions associated with domestication, metabolite traits, and anti-inflammatory effects. Mol. Plant 15, 1367–1383. doi: 10.1016/j.molp.2022.07.003
Ling, H. Q., Zhao, S., Liu, D., Wang, J., Sun, H., Zhang, C., et al. (2013). Draft genome of the wheat a-genome progenitor Triticum urartu. Nature 496, 87–90. doi: 10.1038/nature11997
Lin, Z., Li, X., Shannon, L. M., Yeh, C. T., Wang, M. L., Bai, G., et al. (2012). Parallel domestication of the Shattering1 genes in cereals. Nat. Genet. 44, 720–724. doi: 10.1038/ng.2281
Lin, M., Qiao, P., Matschi, S., Vasquez, M., Ramstein, G. P., Bourgault, R., et al. (2022). Integrating GWAS and TWAS to elucidate the genetic architecture of maize leaf cuticular conductance. Plant Physiol. 189, 2144–2158. doi: 10.1093/plphys/kiac198
Liu, Y., Du, H., Li, P., Shen, Y., Peng, H., Liu, S., et al. (2020). Pan-genome of wild and cultivated soybeans. Cell 182, 162–176. doi: 10.1016/j.cell.2020.05.023
Liu, Q., Liu, H., Gong, Y., Tao, Y., Jiang, L., Zuo, W., et al. (2017). An atypical thioredoxin imparts early resistance to sugarcane mosaic virus in maize. Mol. Plant 10, 483–497. doi: 10.1016/j.molp.2017.02.002
Liu, W., Pan, X., Li, Y., Duan, Y., Min, J., Liu, S., et al. (2019). Identification of QTLs and validation of qCd-2 associated with grain cadmium concentrations in rice. Rice Sci. 26, 42–49. doi: 10.1016/j.rsci.2018.12.003
Liu, H., Wang, X., Liu, S., Huang, Y., Guo, Y.-X., Xie, W.-Z., et al. (2022c). Citrus pan-genome to breeding database (CPBD): A comprehensive genome database for citrus breeding. Mol. Plant. 15 (10), 1503–1505. doi: 10.1016/J.MOLP.2022.08.006
Liu, J., Wang, J., Wang, H., Wang, W., Zhou, R., Mei, D., et al. (2016). Multigenic control of pod shattering resistance in Chinese rapeseed germplasm revealed by genome-wide association and linkage analyses. Front. Plant Sci. 7. doi: 10.3389/fpls.2016.01058
Liu, S., Xu, F., Xu, Y., Wang, Q., Yan, J., Wang, J., et al. (2022b). MODAS: exploring maize germplasm with multi-omics data association studies. Sci. Bull. 67, 903–906. doi: 10.1016/j.scib.2022.01.021
Liu, P., Zhang, Y., Zou, C., Yang, C., Pan, G., Ma, L., et al. (2022a). Integrated analysis of long non-coding RNAs and mRNAs reveals the regulatory network of maize seedling root responding to salt stress. BMC Genomics 23, 50. doi: 10.1186/s12864-021-08286-7
Li, H., Wang, S., Chai, S., Yang, Z., Zhang, Q., Xin, H., et al. (2022a). Graph-based pan-genome reveals structural and sequence variations related to agronomic traits and domestication in cucumber. Nat. Commun. 3, 682. doi: 10.1038/s41467-022-28362-0
Li, Z., Wang, P., You, C., Yu, J., Zhang, X., Yan, F., et al. (2020). Combined GWAS and eQTL analysis uncovers a genetic regulatory network orchestrating the initiation of secondary cell wall development in cotton. New Phytol. 226, 1738–1752. doi: 10.1111/nph.16468
Li, H., Yang, Y., Zhang, H., Chu, S., Zhang, X., Yin, D., et al. (2016). A genetic relationship between phosphorus efficiency and photosynthetic traits in soybean as revealed by QTL analysis using a high-density genetic map. Front. Plant Sci. 7. doi: 10.3389/fpls.2016.00924
Li, N., Zhang, Z., Chen, Z., Cao, B., Xu, K. (2021a). Comparative transcriptome analysis of two contrasting Chinese cabbage (Brassica rapa l.) genotypes reveals that ion homeostasis is a crucial biological pathway involved in the rapid adaptive response to salt stress. Front. Plant Sci. 12. doi: 10.3389/fpls.2021.683891
Lloyd, J. P., Lister, R. (2022). Epigenome plasticity in plants. Nat. Rev. Genet. 23, 55–68. doi: 10.1038/s41576-021-00407-y
Luo, M. J., Zhang, Y. X., Chen, K., Kong, M. S., Song, W., Lu, B. S., et al. (2019). Mapping of quantitative trait loci for seedling salt tolerance in maize. Mol. Breed. 39, 64–76. doi: 10.1007/s11032-019-0974-7
Luo, Q., Zheng, Q., Hu, P., Yang, G., Li, H., Li, B., et al. (2021). Mapping QTL for agronomic traits under two levels of salt stress in a new constructed RIL wheat population. Theor. Appl. Genet. 134, 171–189. doi: 10.1007/s00122-020-03689-8
Lu, K., Wei, L., Li, X., Wang, Y., Wu, J., Liu, M., et al. (2019). Whole-genome resequencing reveals brassica napus origin and genetic loci involved in its improvement. Nat. Commun. 10, 1154. doi: 10.1038/s41467-019-09134-9
Macdiarmid, J. I., Whybrow, S. (2019). Nutrition from a climate change perspective. Proc. Nutr. Soc 78, 380–387. doi: 10.1017/S0029665118002896
Mahender, A., Anandan, A., Pradhan, S. K., Pandit, E. (2016). Rice grain nutritional traits and their enhancement using relevant genes and QTLs through advanced approaches. Springerplus 5, 2086. doi: 10.1186/s40064-016-3744-6
Maher, K. A., Bajic, M., Kajala, K., Reynoso, M., Pauluzzi, G., West, D. A., et al. (2018). Profiling of accessible chromatin regions across multiple plant species and cell types reveals common gene regulatory principles and new control modules. Plant Cell 30, 15–36. doi: 10.1105/tpc.17.00581
Mahmood, U., Hussain, S., Hussain, S., Ali, B., Ashraf, U., Zamir, S., et al. (2021). Morpho-physio-biochemical and molecular responses of maize hybrids to salinity and waterlogging during stress and recovery phase. Plants 10, 1345. doi: 10.3390/plants10071345
Mao, C., He, J., Liu, L., Deng, Q., Yao, X., Liu, C., et al. (2020). OsNAC2 integrates auxin and cytokinin pathways to modulate rice root development. Plant Biotechnol. J. 18, 429–442. doi: 10.1111/pbi.13209
Ma, S., Wang, M., Wu, J., Guo, W., Chen, Y., Li, G., et al. (2021). WheatOmics: A platform combining multiple omics data to accelerate functional genomics studies in wheat. Mol. Plant 14, 1965–1968. doi: 10.1016/j.molp.2021.10.006
McLoughlin, F., Augustine, R. C., Marshall, R. S., Li, F., Kirkpatrick, L. D., Otegui, M. S., et al. (2018). Maize multi-omics reveal roles for autophagic recycling in proteome remodelling and lipid turnover. Nat. Plants 4, 1056–1070. doi: 10.1038/s41477-018-0299-2
Mehrmohamadi, M., Sepehri, M. H., Nazer, N., Norouzi, M. R. (2021). A comparative overview of epigenomic profiling methods. Front. Cel Dev. Biol. 9. doi: 10.3389/fcell.2021.714687
Minow, M. A., Lukens, L., Rossi, V., Colasanti, J. (2022). Patterns of stability and change in the maize genome: a case study of small RNA transcriptomes in two recombinant inbred lines and their progenitors. Genome 65, 7–18. doi: 10.1139/gen-2021-0040
Mir, R. R., Reynolds, M., Pinto, F., Khan, M. A., Bhat, M. A. (2019). High-throughput phenotyping for crop improvement in the genomics era. Plant Sci. 282, 60–72. doi: 10.1016/j.plantsci.2019.01.007
Mir, R. R., Rustgi, S., Zhang, Y. M., Xu, C. (2022). Multi-faceted approaches for breeding nutrient-dense, disease-resistant, and climate-resilient crop varieties for food and nutritional security. Heredity 128, 387–390. doi: 10.1038/s41437-022-00542-0
Misra, B. B., Langefeld, C. D., Michael, O., Cox, L. A. (2018). Integrated omics: tools, advances, and future approaches. J. Mol. Endocrinol. 62, 21–45. doi: 10.1530/JME-18-0055
Moing, A., Allwood, J. W., Aharoni, A., Baker, J., Beale, M. H., Ben-Dor, S., et al. (2020). Comparative metabolomics and molecular phylogenetics of melon (Cucumis melo, cucurbitaceae) biodiversity. Metabolites 10, 121. doi: 10.3390/metabo10030121
Molotoks, A., Smith, P., Dawson, T. P. (2021). Impacts of land use, population, and climate change on global food security. Food Energy Secur. 10, e261. doi: 10.1002/fes3.261
Muthamilarasan, M., Singh, N. K., Prasad, M. (2019). Multi-omics approaches for strategic improvement of stress tolerance in underutilized crop species: a climate change perspective. Adv. Genet. 103, 1–38. doi: 10.1016/bs.adgen.2019.01.001
Naeem, M., Ali, Z., Khan, A., Chaudhary, H. J., Ashraf, J., Baloch, F. S. (2022). Omics: a tool for resilient rice genetic improvement strategies. Mol. Biol. Rep. 49, 5075–5088. doi: 10.1007/s11033-022-07189-4
Nakabayashi, R., Saito, K. (2020). Higher dimensional metabolomics using stable isotope labeling for identifying the missing specialized metabolism in plants. Curr. Opin. Plant Biol. 55, 84–92. doi: 10.1016/j.pbi.2020.02.009
Nataraja, K. N., Madhura, B. G., Parvathi, S. M. (2017). ““Omics: modern tools for precise understanding of drought adaptation in plants,”,” in Plant OMICS and crop breeding. Eds. Zargar, S. M., Rai, V. (Palm Bay, FL: Apple Academic Press), 289–320.
Nitcher, R., Distelfeld, A., Tan, C., Yan, L., Dubcovsky, J. (2013). Increased copy number at the HvFT1 locus is associated with accelerated flowering time in barley. Mol. Genet. Genomics 288, 261–275. doi: 10.1007/s00438-013-0746-8
Niu, Y., Wu, L., Li, Y., Huang, H., Qian, M., Sun, W., et al. (2020). Deciphering the transcriptional regulatory networks that control size, color, and oil content in Brassica rapa seeds. Biotechnol. Biofuels. 13, 90. doi: 10.1186/s13068-020-01728-6
Nunes-Nesi, A., Alseekh, S., de Oliveira Silva, F. M., Omranian, N., Lichtenstein, G., Minezhad, M., et al. (2019). Identification and characterization of metabolite quantitative trait loci in tomato leaves and comparison with those reported for fruits and seeds. Metabolomics 15, 46. doi: 10.1007/s11306-019-1503-8
Ojiewo, C. O., Janila, P., Bhatnagar-Mathur, P., Pandey, M. K., Desmae, H., Okori, P., et al. (2020). Advances in crop improvement and delivery research for nutritional quality and health benefits of groundnut (Arachis hypogaea l.). Front. Plant Sci. 11. doi: 10.3389/fpls.2020.00029
Ong-Abdullah, M., Ordway, J. M., Jiang, N., Ooi, S., Kok, S.-Y., Sarpan, N., et al. (2015). Loss of karma transposon methylation underlies the mantled somaclonal variant of oil palm. Nature 525, 533–537. doi: 10.1038/nature15365
Opdensteinen, P., Sperl, L. E., Mohamadi, M., Kündgen-Redding, N., Hagn, F., Buyel, J. F. (2022). The transient expression of recombinant proteins in plant cell packs facilitates stable isotope labeling for NMR spectroscopy. Plant Biotechnol. J. 20, 1928–1939. doi: 10.1111/pbi.13873
Pan, J., Li, Z., Dai, S., Ding, H., Wang, Q., Li, X., et al. (2020). Integrative analyses of transcriptomics and metabolomics upon seed germination of foxtail millet in response to salinity. Sci. Rep. 10, 13660. doi: 10.1038/s41598-020-70520-1
Park, S. G., Park, H. S., Baek, M. K., Jeong, J. M., Cho, Y. C., Lee, G. M., et al. (2019). Improving the glossiness of cooked rice, an important component of visual rice grain quality. Rice 12, 87. doi: 10.1186/s12284-019-0348-0
Pazhamala, L. T., Kudapa, H., Weckwerth, W., Millar, A. H., Varshney, R. K. (2021). Systems biology for crop improvement. Plant Genome 14, e20098. doi: 10.1002/tpg2.20098
Peng, M., Shahzad, R., Gul, A., Subthain, H., Shen, S., Lei, L., et al. (2017). Differentially evolved glucosyltransferases determine natural variation of rice flavone accumulation and UV-tolerance. Nat. Commun. 8, 1975. doi: 10.1038/s41467-017-02168-x
Peng, H., Wang, K., Chen, Z., Cao, Y., Gao, Q., Li, Y., et al. (2020). MBKbase for rice: an integrated omics knowledgebase for molecular breeding in rice. Nucleic Acids Res. 48, 1085–1092. doi: 10.1093/nar/gkz921
Pott, D. M., Durán-Soria, S., Osorio, S., Vallarino, J. G. (2021). Combining metabolomic and transcriptomic approaches to assess and improve crop quality traits. CABI Agric. Biosci. 2, 1–20. doi: 10.1186/s43170-020-00021-8
Pramanik, D., Shelake, R. M., Kim, M. J., Kim, J. Y. (2020). CRISPR mediated engineering across the central dogma in plant biology for basic research and crop improvement. Mol. Plant. 14 (1), 127–150. doi: 10.1016/j.molp.2020.11.002
Puranik, S., Sahu, P. P., Beynon, S., Srivastava, R. K., Sehgal, D., Ojulong, H., et al. (2020). Genome-wide association mapping and comparative genomics identifies genomic regions governing grain nutritional traits in finger millet (Eleusine coracana l. gaertn.). Plants People Planet 2, 649–662. doi: 10.1002/ppp3.10120
Qi, C., Jiang, H., Xiong, J., Yuan, B., Feng, Y. (2019). On-line trapping/capillary hydrophilic-interaction liquid chromatography/mass spectrometry for sensitive determination of RNA modifications from human blood. Chin. Chem. Lett. 30, 553–557. doi: 10.1016/j.cclet.2018.11.029
Qi, X., Li, M. W., Xie, M., Liu, X., Ni, M., Shao, G., et al. (2014). Identification of a novel salt tolerance gene in wild soybean by whole-genome sequencing. Nat. Commun. 5, 4340. doi: 10.1038/ncomms5340
Qin, P., Lu, H., Du, H., Wang, H., Chen, W., Chen, Z., et al. (2021). Pan-genome analysis of 33 genetically diverse rice accessions reveals hidden genomic variations. Cell 184, 3542–3558. doi: 10.1016/j.cell.2021.04.046
Qutub, M., Chandran, S., Rathinavel, K., Sampathrajan, V., Rajasekaran, R., Manickam, S., et al. (2021). Improvement of a yairipok ChujakMaize landrace from north Eastern Himalayan region for b-carotene content through molecular marker-assisted backcross breeding. Genes 12, 762. doi: 10.3390/genes12050762
Rahman, M. A., Bimpong, I. K., Bizimana, J., Pascual, E. D., Arceta, M., Swamy, B., et al. (2017). Mapping QTLs using a novel source of salinity tolerance from hasawi and their interaction with environments in rice. Rice 10, 1–17. doi: 10.1186/s12284-017-0186-x
Rakyan, V. K., Down, T. A., Balding, D. J., Beck, S. (2011). Epigenome-wide association studies for common human diseases. Nat. Rev. Genet. 12, 529–541. doi: 10.1038/nrg3000
Rangwala, S. H., Kuznetsov, A., Ananiev, V., Asztalos, A., Borodin, E., Evgeniev, V., et al. (2021). Accessing NCBI data using the NCBI sequence viewer and genome data viewer (GDV). Genome Res. 31, 159–169. doi: 10.1101/gr.266932.120
Raxwal, V. K., Ghosh, S., Singh, S., Katiyar-Agarwal, S., Goel, S., Jagannath, A., et al. (2020). Abiotic stress-mediated modulation of the chromatin landscape in Arabidopsis thaliana. J. Exp. Bot. 71, 5280–5293. doi: 10.1093/jxb/eraa286
Raza, A. (2022). Metabolomics: a systems biology approach for enhancing heat stress tolerance in plants. Plant Cell Rep. 41(3), 741–763. doi: 10.1007/s00299-020-02635-8
Raza, A., Charagh, S., García-Caparrós, P., Rahman, M. A., Ogwugwa, V. H., Saeed, F., et al. (2022b). Melatonin-mediated temperature stress tolerance in plants. GM Crops Food. 13, 196–217. doi: 10.1080/21645698.2022.2106111
Raza, A., Razzaq, A., Mehmood, S. S., Hussain, M. A., Wei, S., He, H., et al. (2021b). Omics: the way forward to enhance abiotic stress tolerance in Brassica napus l. GM Crops Food 12, 251–281. doi: 10.1080/21645698.2020.1859898
Raza, A., Tabassum, J., Kudapa, H., Varshney, R. K. (2021a). Can omics deliver temperature resilient ready-to-grow crops? Crit. Rev. Biotechnol. 7, 1–24. doi: 10.1080/07388551.2021.1898332
Raza, A., Tabassum, J., Zahid, Z., Charagh, S., Bashir, S., Barmukh, R., et al. (2022a). Advances in “Omics”. approaches for improving toxic Metals/Metalloids tolerance in plants. Front. Plant Sci. 12. doi: 10.3389/fpls.2021.794373
Razzaq, A., Sadia, B., Raza, A., Hameed, M. K., Saleem, F. (2019). Metabolomics: a way forward for crop improvement. Metabolites 9, 303. doi: 10.3390/metabo9120303
Reimer, J. J., Shaaban, B., Drummen, N., Sanjeev Ambady, S., Genzel, F., Poschet, G., et al. (2022). Capsicum leaves under stress: Using multi-omics analysis to detect abiotic stress network of secondary metabolism in two species. Antioxidants 11, 671. doi: 10.3390/antiox11040671
Roorkiwal, M., Pandey, S., Thavarajah, D., Hemalatha, R., Varshney, R. K. (2021). Molecular mechanisms and biochemical pathways for micronutrient acquisition and storage in legumes to support biofortification for nutritional security. Fronts. Plant Sci. 12. doi: 10.3389/fpls.2021.682842
Rout, K., Yadav, B. G., Yadava, S. K., Mukhopadhyay, A., Gupta, V., Pental, D., et al. (2018). QTL landscape for oil content in brassica juncea: analysis in multiple bi-parental populations in high and "0" erucic background. Front. Plant Sci. 9. doi: 10.3389/fpls.2018.01448
Ruan, Y., Yu, B., Knox, R. E., Singh, A. K., DePauw, R., Cuthbert, R., et al. (2020). High density mapping of quantitative trait loci conferring gluten strength in Canadian durum wheat. Front. Plant Sci. 11. doi: 10.3389/fpls.2020.00170
Rustgi, S., Kakati, J. P., Jones, Z. T., Zoong Lwe, Z. S., Narayanan, S. (2021). Heat tolerance as a function of membrane lipid remodeling in the major US oilseed crops (soybean and peanut). J. Plant Biochem. Biotechnol. 30, 652–667. doi: 10.1007/s13562-021-00729-2
Saeed, F., Chaudhry, U. K., Bakhsh, A., Raza, A., Saeed, Y., Bohra, A., et al. (2022). Moving beyond DNA sequence to improve plant stress responses. Front. Genet. 13. doi: 10.3389/fgene.2022.874648
Sandhu, N., Singh, A., Dixit, S., Cruz, M. T. S., Maturan, P. C., Jain, R. K., et al. (2014). Identification and mapping of stable QTL with main and epistasis effect on rice grain yield under upland drought stress. BMC Genet. 15, 63. doi: 10.1186/1471-2156-15-63
Scheelbeek, P. F., Bird, F. A., Tuomisto, H. L., Green, R., Harris, F. B., Joy, E. J., et al. (2018). Effect of environmental changes on vegetable and legume yields and nutritional quality. Proc. Natl. Acad. Sci. 115, 6804–6809. doi: 10.1073/pnas.1800442115
Schiessl, S. V., Quezada-Martinez, D., Orantes-Bonilla, M., Snowdon, R. J. (2020). Transcriptomics reveal high regulatory diversity of drought tolerance strategies in a biennial oil crop. Plant Sci. 297, 110515. doi: 10.1016/j.plantsci
Schläppi, M. R., Jackson, A. K., Eizenga, G. C., Wang, A., Chu, C., Shi, Y., et al. (2017). Assessment of five chilling tolerance traits and GWAS mapping in rice using the USDA mini-core collection. Front. Plant Sci. 8. doi: 10.3389/fpls.2017.00957
Schmidt, J., Blessing, F., Fimpler, L., Wenzel, F. (2020). Nanopore sequencing in a clinical routine laboratory: challenges and opportunities. Clin. Lab. 66, 1097. doi: 10.7754/Clin.Lab.2019.191114
Schnable, P. S., Ware, D., Fulton, R. S., Stein, J. C., Wei, F., Pasternak, S., et al. (2009). The B73 maize genome: complexity, diversity, and dynamics. Science 326, 1112–1115. doi: 10.1126/science.1178534
Schulze, W. X., Altenbuchinger, M., He, M., Kränzlein, M., Zörb, C. (2021). Proteome profiling of repeated drought stress reveals genotype-specific responses and memory effects in maize. Plant Physiol. Biochem. 159, 67–79. doi: 10.1016/j.plaphy.2020.12.009
Schwope, R., Magris, G., Miculan, M., Paparelli, E., Celii, M., Tocci, A., et al. (2021). Open chromatin in grapevine marks candidate CREs and with other chromatin features correlates with gene expression. Plant J. 107, 1631–1647. doi: 10.1111/tpj.15404
Scossa, F., Alseekh, S., Fernie, A. R. (2021). Integrating multi-omics data for crop improvement. J. Plant Physiol. 257, 153352. doi: 10.1016/j.jplph.2020.153352
Selvaraj, R., Singh, A. K., Singh, V. K., Abbai, R., Habde, S. V., Singh, U. M., et al. (2021). Superior haplotypes towards development of low glycemic index rice with preferred grain and cooking quality. Sci. Rep. 11, 10082. doi: 10.1038/s41598-021-87964-8
Senior, A. W., Evans, R., Jumper, J., Kirkpatrick, J., Sifre, L., Green, T., et al. (2020). Improved protein structure prediction using potentials from deep learning. Nature 577, 706–710. doi: 10.1038/s41586-019-1923-7
Seo, J. H., Kang, B. K., Dhungana, S. K., Oh, J. H., Choi, M. S., Park, J. H., et al. (2020). QTL mapping and candidate gene analysis for pod shattering tolerance in soybean (Glycine max). Plants 9, 1163. doi: 10.3390/plants9091163
Shahzad, A., Qian, M., Sun, B., Mahmood, U., Li, S., Fan, Y., et al. (2021). Genome-wide association study identifies novel loci and candidate genes for drought stress tolerance in rapeseed. Oil Crop Sci. 6, 12–22. doi: 10.1016/j.ocsci.2021.01.002
Sharma, V., Gupta, P., Priscilla, K., SharanKumar, H. B., Veershetty, A., Ramrao, D. P., et al. (2021). Metabolomics intervention towards better understanding of plant traits. Cells 10, 346. doi: 10.3390/cells10020346
Shendure, J., Balasubramanian, S., Church, G. M., Gilbert, W., Rogers, J., Schloss, J. A., et al. (2017). DNA Sequencing at 40: past, present and future. Nature 550, 345–353. doi: 10.1038/nature24286
Shen, Y., Zhang, J., Liu, Y., Liu, S., Liu, Z., Duan, Z., et al. (2018). DNA Methylation footprints during soybean domestication and improvement. Genome Biol. 19, 128. doi: 10.1186/s13059-018-1516-z
Shen, Y., Zhou, G., Liang, C., Tian, Z. (2022). Omics-based interdisciplinarity is accelerating plant breeding. Curr. Opin. Plant Biol. 66, 102167. doi: 10.1016/j.pbi.2021.102167
Shi, F., Pang, Z., Liu, C., Zhou, L., Tan, C., Ren, J., et al. (2022). Whole-transcriptome analysis and construction of an anther development-related ceRNA network in Chinese cabbage (Brassica campestris l. ssp. pekinensis). Sci. Rep. 12, 2667. doi: 10.1038/s41598-022-06556-2
Shukla, S., Singh, K., Patil, R. V., Kadam, S., Bharti, S., Prasad, P., et al. (2015). Genomic regions associated with grain yield under drought stress in wheat (Triticum aestivum l.). Euphytica 203, 449–467. doi: 10.1007/s10681-014-1314-y
Singh, N., Rai, V., Singh, N. K. (2020). Multi-omics strategies and prospects to enhance seed quality and nutritional traits in pigeonpea. Nucleus 63, 249–256. doi: 10.1007/s13237-020-00341-0
Song, Q., Ando, A., Jiang, N., Ikeda, Y., Chen, Z. J. (2020). Single-cell RNA-seq analysis reveals ploidy-dependent and cell-specific transcriptome changes in arabidopsis female gametophytes. Genome Biol. 21, 178. doi: 10.1186/s13059-020-02094-0
Song, J. M., Liu, D. X., Xie, W. Z., Yang, Z., Guo, L., Liu, K., et al. (2021). BnPIR: Brassica napus pan-genome information resource for 1689 accessions. Plant Biotechnol. J. 19, 412–414. doi: 10.1111/pbi.13491
Sthapit Kandel, J., Peng, H., Hayes, R. J., Mou, B., Simko, I. (2020). Genome-wide association mapping reveals loci for shelf life and developmental rate of lettuce. Theor. Appl. Genet. 133, 1947–1966. doi: 10.1007/s00122-020-03568-2
Suhre, K., Arnold, M., Bhagwat, A. M., Cotton, R. J., Engelke, R., Raffler, J., et al. (2017). Connecting genetic risk to disease end points through the human blood plasma proteome. Nat. Commun. 8, 14357. doi: 10.1038/ncomms14357
Sun, C., Hu, Z., Zheng, T., Lu, K., Zhao, Y., Wang, W., et al. (2017). RPAN: rice pan-genome browser for approximately 3000 rice genomes. Nucleic Acids Res. 45, 597–605. doi: 10.1093/nar/gkw958
Sun, M., Yang, Z., Liu, L., Duan, L. (2022). DNA Methylation in plant responses and adaption to abiotic stresses. Int. J. Mol. Sci. 23, 6910. doi: 10.3390/ijms23136910
Sutton, T., Baumann, U., Hayes, J., Collins, N. C., Shi, B. J., Schnurbusch, T., et al. (2007). Boron-toxicity tolerance in barley arising from efflux transporter amplification. Science 318, 1446–1449. doi: 10.1126/science.1146853
Syed, A., Raza, T., Bhatti, T. T., Eash, N. S. (2022). Climate impacts on the agricultural sector of Pakistan: risks and solutions. Environ. Challenges 6, 100433. doi: 10.1016/J.ENVC.2021.100433
Szymañski, J., Bocobza, S., Panda, S., Sonawane, P., Cárdenas, P. D., Lashbrooke, J., et al. (2020). Analysis of wild tomato introgression lines elucidates the genetic basis of transcriptome and metabolome variation underlying fruit traits and pathogen response. Nat. Genet. 52, 1111–1121. doi: 10.1038/s41588-020-0690-6
Tang, S., Zhao, H., Lu, S., Yu, L., Zhang, G., Zhang, Y., et al. (2021). Genome- and transcriptome-wide association studies provide insights into the genetic basis of natural variation of seed oil content in Brassica napus. Mol. Plant 14, 470–487. doi: 10.1016/j.molp.2020.12.003
Tan, L., Li, X., Liu, F., Sun, X., Li, C., Zhu, Z., et al. (2008). Control of a key transition from prostrate to erect growth in rice domestication. Nat. Genet. 40, 1360–1364. doi: 10.1038/ng.197
Teshome, D. T., Zharare, G. E., Naidoo, S. (2020). The threat of the combined effect of biotic and abiotic stress factors in forestry under a changing climate. Front. Plant Sci. 11. doi: 10.3389/fpls.2020.601009
Theologis, A., Ecker, J. R., Palm, C. J., Federspiel, N. A., Kaul, S., White, O., et al. (2000). Sequence and analysis of chromosome 1 of the plant Arabidopsis thaliana. Nature 408, 816–820. doi: 10.1038/35048500
The Tomato Genome Consortium (2012). The tomato genome sequence provides insights into fleshy fruit evolution. Nature 485, 635–641. doi: 10.1038/nature11119
Tollefson, J. (2020). How hot will earth get by 2100? Nature 580, 443–446. doi: 10.1038/d41586-020-01125-x
Torkamaneh, D., Lemay, M. A., Belzile, F. (2021). The pan-genome of the cultivated soybean (PanSoy) reveals an extraordinarily conserved gene content. Plant Biotechnol. J. 19, 1852–1862. doi: 10.1111/pbi.13600
Uchida, K., Sawada, Y., Ochiai, K., Sato, M., Inaba, J., Hirai, M. Y. (2020). Identification of a unique type of isoflavone O-methyltransferase, GmIOMT1, based on multi-omics analysis of soybean under biotic stress. Plant Cell Physiol. 61, 1974–1985. doi: 10.1093/pcp/pcaa112
Ueda, M., Seki, M. (2020). Histone modifications form epigenetic regulatory networks to regulate abiotic stress response. Plant Physiol. 182, 15–26. doi: 10.1104/pp.19.00988
Valentin, G., Abdel, T., Gaetan, D., Jean-Francois, D., Matthieu, C., Mathieu, R. (2021). GreenPhylDB v5: a comparative pangenomic database for plant genomes. Nucleic Acids Res. 49, D1464–D1471. doi: 10.1093/nar/gkaa1068
van Dijk, A. D. J., Kootstra, G., Kruijer, W., de Ridder, D. (2021). Machine learning in plant science and plant breeding. Iscience 24, 101890. doi: 10.1016/j.isci.2020.101890
Vaughan, M. M., Block, A., Christensen, S. A., Allen, L. H., Schmelz, E. A. (2018). The effects of climate change associated abiotic stresses on maize phytochemical defenses. Phytochem. Rev. 17, 37–49. doi: 10.1007/s11101-017-9508-2
Vikram, P., Swamy, B. P. M., Dixit, S., Ahmed, H., Cruz, M. T., Singh, A. K., et al. (2012). Bulk segregant analysis: “an effective approach for mapping consistent-effect drought grain yield QTLs in rice”. Field Crops Res. 134, 185–192. doi: 10.1016/j.fcr.2012.05.012
Vistain, L. F., Tay, S. (2021). Single-cell proteomics. Trends Biochem. Sci. 46, 661–672. doi: 10.1016/j.tibs.2021.01.013
Voss-Fels, K. P., Stahl, A., Hickey, L. T. (2019). Q&A: modern crop breeding for future food security. BMC Biol. 17, 18. doi: 10.1186/s12915-019-0638-4
Wainberg, M., Sinnott-Armstrong, N., Mancuso, N., Barbeira, A. N., Knowles, D. A., Golan, D., et al. (2019). Opportunities and challenges for transcriptome-wide association studies. Nat. Genet. 51, 592–599. doi: 10.1038/s41588-019-0385-z
Waititu, J. K., Zhang, C., Liu, J., Wang, H. (2020). Plant non-coding RNAs: origin, biogenesis, mode of action and their roles in abiotic stress. Int. J. Mol. Sci. 9, 8401. doi: 10.3390/ijms21218401
Wang, H., Cimen, E., Singh, N., Buckler, E. (2020b). Deep learning for plant genomics and crop improvement. Curr. Opin. Plant Biol. 54, 34–41. doi: 10.1016/j.pbi.2019.12.010
Wang, W., Qin, Q., Sun, F., Wang, Y., Xu, D., Li, Z., et al. (2016). Genome-wide differences in DNA methylation changes in two contrasting rice genotypes in response to drought conditions. Front. Plant Sci. 7. doi: 10.3389/fpls.2016
Wang, X. Y., Song, S., Wang, X., Liu, J., Dong, S. K. (2022). Transcriptomic and metabolomic analysis of seedling-stage soybean responses to PEG-simulated drought stress. Int. J. Mol. Sci. 23, 6869. doi: 10.3390/ijms23126869
Wang, S., Wei, J., Li, R., Qu, H., Chater, J. M., Ma, R., et al. (2019). Identification of optimal prediction models using multi-omic data for selecting hybrid rice. Heredity 123, 395–406. doi: 10.1038/s41437-019-0210-6
Wang, X., Wen, Y. (2022). A penalized linear mixed model with generalized method of moments for prediction analysis on high-dimensional multi-omics data. Brief. Bioinform. 23, bbac193. doi: 10.1093/bib/bbac193
Wang, P., Yao, S., Kosami, K. I., Guo, T., Li, J., Zhang, Y., et al. (2020a). Identification of endogenous small peptides involved in rice immunity through transcriptomics-and proteomics-based screening. Plant Biotechnol. J. 18, 415–428. doi: 10.1111/pbi.13208
Wang, Y., VandenLangenberg, K., Wen, C., Wehner, T. C., Weng, Y. (2018). QTL mapping of downy and powdery mildew resistances in PI 197088 cucumber with genotyping-by-sequencing in RIL population. Theor Appl Genet. 131 (3), 597–611. doi: 10.1007/s00122-017-3022-1.
Wang, W., Pang, J., Zhang, F., Sun, L., Yang, L., Zhao, Y., et al. (2021). Integrated transcriptomics and metabolomics analysis to characterize alkali stress responses in canola (Brassica napus L.). Plant Physiol. Biochem. 166, 605–620. doi: 10.1016/j.plaphy.2021.06.021
Watson, J. D., Crick, F. H. (1953). Molecular structure of nucleic acids: a structure for deoxyribose nucleic acid. Nature 171, 737–738. doi: 10.1038/171737a0
Weissbrod, O., Geiger, D., Rosset, S. (2016). Multikernel linear mixed models for complex phenotype prediction. Genome Res. 26, 969–979. doi: 10.1101/gr.201996.115
Wei, J. L., Wang, A. G., Li, R. D., Qu, H., Jia, Z. Y. (2017). Metabolome-wide association studies for agronomic traits of rice. Heredity 120, 342–355. doi: 10.1038/s41437-017-0032-3
Wilkins, O., Hafemeister, C., Plessis, A., Holloway-Phillips, M. M., Pham, G. M., Nicotra, A. B., et al. (2016). EGRINs (Environmental gene regulatory influence networks) in rice that function in the response to water deficit, high temperature, and agricultural environments. Plant Cell 28, 2365–2384. doi: 10.1105/tpc.16.00158
Wing, R. A., Purugganan, M. D., Zhang, Q. (2018). The rice genome revolution: from an ancient grain to green super rice. Nat. Rev. Genet. 19, 505–517. doi: 10.1038/s41576-018-0024-z
Woolfson, M. (2018). The development of structural x-ray crystallography. Phys. Scr. 93, 1–32. doi: 10.1088/1402-4896/aa9c30
Wu, J., Chen, P., Zhao, Q., Cai, G., Hu, Y., Xiang, Y., et al. (2019). Co-Location of QTL for Sclerotinia stem rot resistance and flowering time in Brassica napus. Crop J. 7, 227–237. doi: 10.1016/j.cj.2018.12.007
Wu, D., Li, X., Tanaka, R., Wood, J. C., Tibbs-Cortes, L. E., Magallanes-Lundback, M., et al. (2022). Combining GWAS and TWAS to identify candidate causal genes for tocochromanol levels in maize grain. Genetics 221, iyac091. doi: 10.1093/genetics/iyac091
Würschum, T., Boeven, P. H., Langer, S. M., Longin, C. F. H., Leiser, W. L. (2015). Multiply to conquer: copy number variations at ppd-B1 and vrn-A1 facilitate global adaptation in wheat. BMC Genet. 16, 96. doi: 10.1186/s12863-015-0258-0
Wu, B., Shen, F., Chen, C. J., Liu, L., Wang, X., Zheng, W. Y., et al. (2021). Natural variations in a pectin acetylesterase gene, MdPAE10, contribute to prolonged apple fruit shelf life. Plant Genome 14, e20084. doi: 10.1002/tpg2.20084
Xiang, H., Okamura, H., Kezuka, Y., Katoh, E. (2018). Physical and thermodynamic characterization of the rice gibberellin receptor/gibberellin/DELLA protein complex. Sci. Rep. 8, 17719. doi: 10.1038/s41598-018-35765-x
Xiao, M., Wang, J., Xu, F. (2022). Methylation hallmarks on the histone tail as a linker of osmotic stress and gene transcription. Front. Plant Sci. 13. doi: 10.3389/fpls.2022.967607
Xu, J., Chen, G., Hermanson, P. J., Xu, Q., Sun, C., Chen, W., et al. (2019). Population-level analysis reveals the widespread occurrence and phenotypic consequence of DNA methylation variation not tagged by genetic variation in maize. Genome Biol. 20, 243. doi: 10.1186/s13059-019-1859-0
Xu, G., Lyu, J., Li, Q., Liu, H., Wang, D., Zhang, M., et al. (2020). Evolutionary and functional genomics of DNA methylation in maize domestication and improvement. Nat. Commun. 11, 5539. doi: 10.1038/s41467-020-19333-4
Xu, S., Xu, Y., Gong, L., Zhang, Q. (2016). Metabolomic prediction of yield in hybrid rice. Plant J. 88, 219–227. doi: 10.1111/tpj.13242
Yadav, S., Sandhu, N., Singh, V. K., Catolos, M., Kumar, A. (2019). Genotyping-by-sequencing based QTL mapping for rice grain yield under reproductive stage drought stress tolerance. Sci. Rep. 9, 14326. doi: 10.1038/s41598-019-50880-z
Yang, J., Liu, S., Ji, L., Tang, X., Zhu, Y., Xie, G. (2020). Identification of novel OsCML16 target proteins and differential expression analysis under abiotic stresses in rice. J. Plant Physiol. 249, 153165. doi: 10.1016/j.jplph.2020.153165
Yang, Z., Li, X., Zhang, N., Zhang, Y. N., Jiang, H. W., Gao, J., et al. (2016). Detection of quantitative trait loci for kernel oil and protein concentration in a B73 and Zheng58 maize cross. Genet. Mol. Res. 15, 10. doi: 10.4238/gmr.15038951
Yang, Y., Saand, M. A., Huang, L., Abdelaal, W. B., Zhang, J., Wu, Y., et al. (2021). Applications of multi-omics technologies for crop improvement. Front. Plant Sci. 12. doi: 10.3389/fpls.2021.563953
Yaschenko, A. E., Fenech, M., Mazzoni-Putman, S., Alonso, J. M., Stepanova, A. N. (2022). Deciphering the molecular basis of tissue-specific gene expression in plants: Can synthetic biology help? Curr. Opin. Plant Biol. 68, 102241. doi: 10.1016/j.pbi.2022.102241
You, J., Zhang, Y., Liu, A., Li, D., Wang, X., Dossa, K., et al. (2019). Transcriptomic and metabolomic profiling of drought-tolerant and susceptible sesame genotypes in response to drought stress. BMC Plant Biol. 19, 267. doi: 10.1186/s12870-019-1880-1
Yuan, Y., Gao, M., Zhang, M., Zheng, H., Zhou, X., Guo, Y., et al. (2017). QTL mapping for phosphorus efficiency and morphological traits at seedling and maturity stages in wheat. Front. Plant Sci. 8. doi: 10.3389/fpls.2017.00614
Yu, Z., Wang, X., Zhang, L. (2018). Structural and functional dynamics of dehydrins: a plant protector protein under abiotic stress. Int. J. Mol. Sci. 19, 16–18. doi: 10.3390/ijms19113420
Zenda, T., Liu, S., Dong, A., Li, J., Wang, Y., Liu, X., et al. (2021). Omics-facilitated crop improvement for climate resilience and superior nutritive value. Front. Plant Sci. 12. doi: 10.3389/fpls.2021.774994
Zeng, X., Yuan, H., Dong, X., Peng, M., Jing, X., Xu, Q., et al. (2020). Genome-wide dissection of coselected UV-b responsive pathways in the UV-b adaptation of qingke. Mol. Plant 13, 112–127. doi: 10.1016/j.molp.2019.10.009
Zhang, X. C., Fan, Y., Shabala, S., Koutoulis, A., Shabala, L., Johnson, P., et al. (2017a). A new major-effect QTL for waterlogging tolerance in wild barley (H. spontaneum). Theor. Appl. Genet. 130, 1559–1568. doi: 10.1007/s00122-017-2910-8
Zhang, J., Chen, K., Pang, Y., Naveed, S. A., Zhao, X., Wang, X., et al. (2017b). QTL mapping and candidate gene analysis of ferrous iron and zinc toxicity tolerance at seedling stage in rice by genome-wide association study. BMC genomics 18(1), 828. doi: 10.1186/s12864-017-4221-5
Zhang, H., Lang, Z., Zhu, J. K. (2018). Dynamics and function of DNA methylation in plants. Nat. Rev. Mol. Cell Biol. 19, 489–506. doi: 10.1038/s41580-018-0016-z
Zhang, X., Liu, P., Qing, C., Yang, C., Shen, Y., Ma, L. (2021a). Comparative transcriptome analyses of maize seedling root responses to salt stress. PeerJ 9, e10765. doi: 10.7717/peerj.10765
Zhang, M., Liu, S., Wang, Z., Yuan, Y., Zhang, Z., Liang, Q., et al. (2022b). Progress in soybean functional genomics over the past decade. Plant Biotechnol. J. 20, 256–282. doi: 10.1111/pbi.13682
Zhang, H., Yang, L., Zhou, R., Zhang, Y., Wang, Z., Pang, H., et al. (2021b). Genome wide transcriptomic analysis of WRKY gene family response to biotic stresses in Malus × domestica. Am. J. Plant Sci. 12, 858–885. doi: 10.4236/ajps.2021.125058
Zhang, H., Zhu, J., Gong, Z., Zhu, J. K. (2022a). Abiotic stress responses in plants. Nat. Rev. Genet. 23, 104–119. doi: 10.1038/s41576-021-00413-0
Zhao, P., Ma, B., Cai, C., Xu, J. (2022). Transcriptome and methylome changes in two contrasting mungbean genotypes in response to drought stress. BMC Genomics 23, 80. doi: 10.1186/s12864-022-08315-z
Zhou, X., Carbonetto, P., Stephens, M. (2013). Polygenic modeling with bayesian sparse linear mixed models. PloS Genet. 9, e1003264. doi: 10.1371/journal.pgen.1003264
Zhou, X., Dai, L., Wang, P., Liu, Y., Xie, Z., Zhang, H., et al. (2021b). Mining favorable alleles for five agronomic traits from the elite rapeseed cultivar zhongshuang 11 by QTL mapping and integration. Crop J. 9, 1449–1459. doi: 10.1016/j.cj.2020.12.008
Zhou, Q., Fu, Z., Liu, H., Wang, J., Guo, Z., Zhang, X., et al. (2021a). Mining novel kernel size-related genes by pQTL mapping and multi-omics integrative analysis in developing maize kernels. Plant Biotechnol. J. 19, 1489–1491. doi: 10.1111/pbi.13634
Zhou, R., Jiang, F., Niu, L., Song, X., Yu, L., Yang, Y., et al. (2022a). Increase crop resilience to heat stress using omic strategies. Front. Plant Sci. 13. doi: 10.3389/fpls.2022.891861
Zhou, Y., Zhang, Z., Bao, Z., Li, H., Lyu, Y., Zan, Y., et al. (2022b). Graph pangenome captures missing heritability and empowers tomato breeding. Nature 606, 527–534. doi: 10.1038/s41586-022-04808-9
Zhou, Y., Zhu, J., Li, Z., Yi, C., Liu, J., Zhang, H., et al. (2009). Deletion in a quantitative trait gene qPE9-1 associated with panicle erectness improves plant architecture during rice domestication. Genetics 183, 315–324. doi: 10.1534/genetics.109.102681
Zhu, G., Wang, S., Huang, Z., Zhang, S., Liao, Q., Zhang, C., et al. (2018). Rewiring of the fruit metabolome in tomato breeding. Cell 172, 249–261. doi: 10.1016/j.cell.2017.12.019
Keywords: multi-omics, breeding, genomics, proteomics, transcriptomics, metabolomics
Citation: Mahmood U, Li X, Fan Y, Chang W, Niu Y, Li J, Qu C and Lu K (2022) Multi-omics revolution to promote plant breeding efficiency. Front. Plant Sci. 13:1062952. doi: 10.3389/fpls.2022.1062952
Received: 06 October 2022; Accepted: 24 November 2022;
Published: 08 December 2022.
Edited by:
Tangchun Zheng, Beijing Forestry University, ChinaReviewed by:
Ali Raza, Fujian Agriculture and Forestry University, ChinaShibo Wang, University of California, Riverside, United States
Copyright © 2022 Mahmood, Li, Fan, Chang, Niu, Li, Qu and Lu. This is an open-access article distributed under the terms of the Creative Commons Attribution License (CC BY). The use, distribution or reproduction in other forums is permitted, provided the original author(s) and the copyright owner(s) are credited and that the original publication in this journal is cited, in accordance with accepted academic practice. No use, distribution or reproduction is permitted which does not comply with these terms.
*Correspondence: Cunmin Qu, drqucunmin@swu.edu.cn; Kun Lu, drlukun@swu.edu.cn
†ORCID: Cunmin Qu, orcid.org/0000-0003-1370-8633
Kun Lu, orcid.org/0000-0003-1370-8633