- 1College of Electronic Science and Technology, National University of Defense, Changsha, China
- 2Key Laboratory of Satellite Navigation Technology, Changsha, China
- 3Beijing Satellite Navigation Center, Beijing, China
- 4Transcom (Shanghai) Technology Co., Ltd., Shanghai, China
Satellite navigation interference monitoring is an important means to effectively evaluate interference and ensure the normal operation of global navigation satellite system (GNSS). Once interference is detected, this monitoring can identify the type of it, perform direction-finding and localization, evaluate its impact on GNSS, and guide the implementation of effective countermeasures. With the continuous progress of interference technology, the power required to cause the same jamming effect to the navigation system is getting smaller and smaller. Traditional radio monitoring system has been unable to meet the needs of the current satellite navigation monitoring in terms of sensitivity and accuracy. It is of great significance to develop and improve the dedicated satellite navigation monitoring system. This paper introduces the basic concept of satellite navigation interference monitoring and the composition of the system, analyzes the key technologies and finally gives an outlook on the development trends in this field.
1 Introduction
The Global Navigation Satellite System (GNSS) is a generic term for satellite navigation systems that provide all-weather, continuous, global coverage for positioning, navigation, and timing services. Currently, the world has seen the formation of four major global satellite navigation systems: the United States’ Global Positioning System (GPS) [1–3], the European Union’s Galileo Satellite Navigation System (Galileo) [1–3], Russia’s Global Navigation Satellite System (GLONASS) [1, 3], and China’s BeiDou Navigation Satellite System (BDS) [3, 4]. In addition, India and Japan have respectively constructed the Indian Regional Navigation Satellite System (IRNSS) [1, 3, 5] and the Quasi-Zenith Satellite System (QZSS) [1, 3, 6].
Satellite navigation systems have become fundamental spatiotemporal reference infrastructures. The development of navigation technology profoundly impacts various sectors of society including economy, surveying, power, transportation, and military affairs. An increasing number of infrastructures have developed a strong reliance on satellite navigation systems. Should these systems suffer a breakdown, it could lead to severe consequences, thereby making the enhancement of GNSS system stability increasingly critical.
However, the GNSS system is inherently vulnerable [7] and is highly susceptible to various types of interference [8]. Interference can be broadly categorized into natural and anthropogenic types. Anthropogenic interference can be further subdivided into unintentional and intentional categories. Given the finite nature of the electromagnetic spectrum, GNSS systems are particularly susceptible to out-of-band interference from harmonics and inter-modulation products, as well as in-band interference from co-channel operations [9]. A notable example occurred at a U.S. port where GPS receivers were disrupted for several hours each evening following the workday, eventually traced back to unintentional interference from active TV antennas atop nearby residents’ homes after months of investigation [3].Satellite navigation signals, originating from satellites orbiting approximately 20,000 km above the Earth’s surface, arrive at ground level with powers as low as −130 dBm which is over a billion times weaker than typical broadcast television signals. Civilian signal formats used in navigation systems are publicly known, with information modulated onto fixed frequencies, rendering GNSS highly vulnerable to intentional malicious interference. Table 1 gives a brief description of some of the interference events that have occurred globally over the past period.
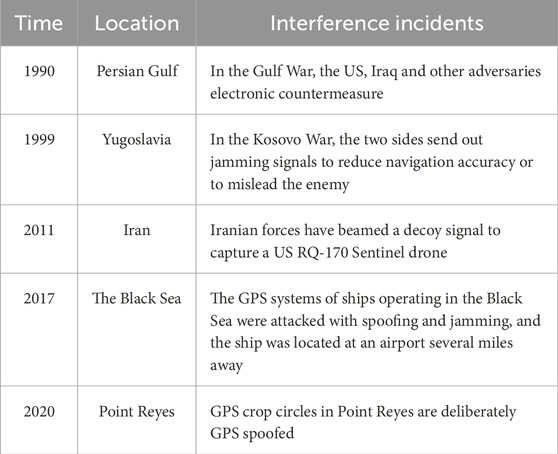
Table 1. Some malicious interference incidents [10].
From the past to the present, interference and anti-interference in satellite navigation systems have been focal points of electronic warfare worldwide, particularly evident in military confrontations. Therefore, the necessity of anti-interference and interference monitoring is self-evident. This review primarily addresses malicious jamming interference. As long as the emitted interference targets the GNSS frequency bands or covers the entire system frequency spectrum with sufficient power, it can achieve significant disruptive effects, posing the greatest threat to user-end navigation receivers. The principle of interference is that as the interference power increases, the equivalent carrier-to-noise ratio (C/N0) at the receiver output degrades, leading to prolonged acquisition times or even loss of lock during signal capture, and reduced pseudo-range accuracy during tracking [11]. This results in a decline in the reliability of the GNSS system, manifesting as significant positioning errors.
Anti-interference refers to the adoption of various technologies and measures [12–17] to reduce or eliminate the impact of interference on system performance. Interference monitoring, on the other hand, involves the detection, identification, and analysis of interference signals in the electromagnetic environment. Specifically, it encompasses the localization of interference sources, the identification of interference signal types and characteristics, and the assessment of their impact. Anti-interference and interference monitoring are complementary; interference monitoring provides detailed information about the interference [18], which is the foundation for implementing anti-interference techniques. Only through effective interference monitoring can the sources and characteristics of interference be accurately identified, enabling targeted anti-interference measures to be taken. For instance, if the interference is identified as frequency sweeping or continuous wave interference, Infinite Impulse Response (IIR) adaptive notch filters can be directly employed to suppress it [19]. When interference is detected in the transform domain, adaptive filters can be directly designed in that domain to suppress the interference, after which the signal can be transformed back to the time domain to accurately recover the original GNSS signal [20–23].
In response to the challenges currently faced in interference monitoring, this paper reviews the development process of GNSS interference monitoring, focusing on the recent advancements in key monitoring technologies. It summarizes and analyzes the existing issues and identifies future research directions in this field.
2 Interference monitoring system
2.1 General situation
The development of GPS by the United States in the 1970s was primarily for military purposes, with early satellite interference monitoring relying on military electronic equipment to ensure the precision of weapon strikes. The Gulf War and the Kosovo War in the 1990s highlighted the importance of satellite navigation interference monitoring in electronic warfare environments, prompting researchers to design specialized equipment for this purpose. As GPS expanded into civilian use and became more globalized, fixed ground-based satellite navigation interference monitoring systems began to emerge. The development of digital signal processing (DSP) technology and software-defined radio (SDR) enabled these systems to identify various types of interference in complex signal environments. In the 21st century, as satellite navigation systems diversified into a quadripartite structure, there arose a need for compatibility in interference monitoring systems. Monitoring platforms have evolved from fixed ground-based systems towards mobile air- and space-based systems, with nations working to establish comprehensive, three-dimensional maritime, land, air, and space interference monitoring systems. Table 2 provides an analysis and comparison of the three types of interference monitoring platforms.
2.2 The development status
2.2.1 General interference monitoring System
Before the advent of satellite navigation systems, many non-navigation systems experienced disruptions due to radio interference that prevented them from operating normally. This was particularly evident in civil aviation systems, where radio interference posed a significant threat to air traffic systems, causing flight delays and even endangering personal safety. To effectively address incidents of electromagnetic interference, the U.S. Federal Aviation Administration (FAA) began constructing a nationwide radio interference monitoring system at the end of the 20th century. This system consists of multiple airborne, mobile, portable, and fixed interference monitoring systems, ensuring the takeoff and landing of flights at key airports and minimizing the impact of radio interference [24]. The primary hardware for this system is manufactured by Cubic Corporation in the United States and has been adopted by many countries, playing a significant role in civil aviation radio interference monitoring. The main modules included in the FAA’s interference monitoring system are shown in Figure 1.
After years of development, in addition to the United States and other countries have also been mature radio monitoring technology. For instance, most provinces can now achieve comprehensive monitoring of critical areas such as airports within their jurisdictions. A large number of fixed monitoring stations, mobile monitoring stations, and portable interference detection devices have been established, forming a relatively complete radio interference monitoring network [25]. Figure 2 [26] shows some of the interference monitoring equipment in our country.
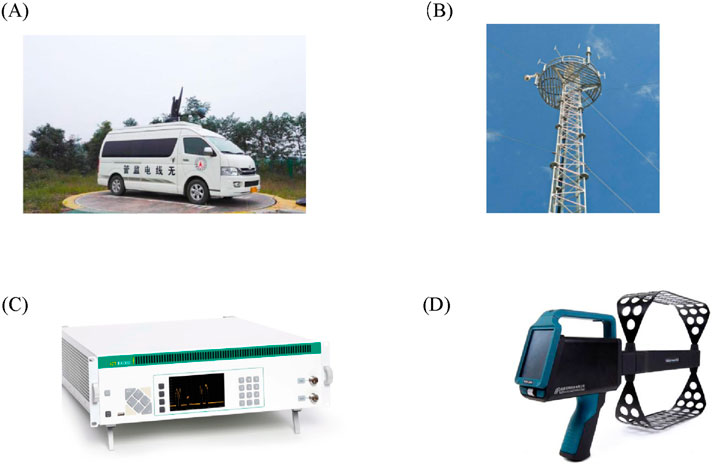
Figure 2. Interference monitoring equipment. (A) Radio monitoring vehicle. (B) Radio monitoring direction finding system. (C) Radio monitoring direction finding receiver. (D) Radio monitoring direction finding system.
2.2.2 Dedicated GNSS interference monitoring system
General-purpose radio interference monitoring networks monitor the electromagnetic environment across the entire frequency spectrum. In contrast, the frequency bands used by GNSS systems are fixed, and the navigation signals reaching the ground are extremely weak, often buried below the noise floor. With the evolution of interference technology, the variety of interference methods has increased, and different interference techniques can produce varying effects [27]. Consequently, the power required to achieve the same level of interference on GNSS systems is decreasing. Traditional general-purpose radio interference monitoring networks are no longer sufficient in terms of sensitivity, accuracy, and speed to meet the monitoring requirements of GNSS systems. Recognizing the challenges faced by GNSS interference monitoring, countries led by the United States began constructing dedicated monitoring networks from the last century.
In 1994, the U.S. National Geodetic Survey began establishing a national network of continuously operating reference stations (CORS) for GPS, which later expanded globally. These CORS receive GPS signals and provide high-precision positioning data for applications such as geodesy and meteorological observations.any GPS CORS are equipped with anti-interference devices and can also assess the quality of received GPS signals. They utilize various techniques, including radio monitoring and noise level measurements, to monitor and locate interference near the base stations.
In 1997, the U.S. Congress directed a project involving Spawar and Falon companies, which developed and demonstrated a prototype system called “LOCO GPSI.” The demonstration results showed that the system was effective and practical, with the ability to locate interference sources. The entire system utilized a short baseline interferometry approach, determining the source of interference through triangulation methods [28].
The JLOC system (Joint Landaster Oriented Coordinate System) is a system commissioned by the Joint Space Operations Center under the U.S. Department of Defense and developed by NAVSYS Corporation. The system was established in 2002 and primarily provides precise geographical location information and time standards. It can monitor abnormal changes in satellite navigation signals and quickly locate the position of interference sources, conducting comprehensive performance testing and evaluation of satellite navigation systems to ensure their normal operation.
In 2009, CHRONO Technologies in the UK developed a handheld interference monitoring device capable of monitoring GPS signals and interference signals in the L1 frequency band [29]. In addition, the U.S. FAA established a GPS interference source monitoring and localization experimental system, which includes a large number of interference sources and localization systems, employing almost all interference source localization technologies [30].
Currently, the American company HawkEye 360 is building the world’s first commercial radio frequency (RF) signal mapping system based on a low-orbit satellite constellation, with plans to launch a total of 60 small satellites into low-earth orbit by 2025. Its products include RFGeo, RFIQ, and SEAker. RFGeo is used to detect and locate RF signals on the Earth’s surface, with Figure 3 [31] showing a precisely mapped image of Earth’s RF signals. RFIQ is dedicated to space-based radio frequency spectrum data collection, providing visualization of the spectrum data gathered by the HawkEye 360 RF sensor satellite constellation, as depicted in Figure 4 [31]. SEAker leverages sophisticated algorithms to integrate automatic identification systems with HawkEye 360 sensors, enhancing maritime awareness capabilities. The entire system primarily focuses on spectrum mapping and signal source localization, offering comprehensive and timely interference detection and early warning services [32].
2.3 Summary
Currently, the development of interference monitoring systems faces numerous challenges: 1) Technical challenges. The electromagnetic environment is becoming increasingly complex, and the accuracy, sensitivity, and speed of traditional radio interference monitoring systems are no longer sufficient for satellite navigation system interference monitoring. Efforts to overcome key technical bottlenecks within the system and promote technological innovation and advancement are essential trends. 2) Construction challenges. There are few dedicated satellite navigation interference monitoring networks, and the coverage areas of these networks are limited. Developing new systems and equipment for GNSS interference monitoring, increasing the number of monitoring stations, and expanding coverage areas are crucial for ensuring reliable GNSS services globally. 3) International Cooperation challenges. Satellite navigation monitoring involves the interests of multiple countries. Strengthening international cooperation and exchange, encouraging active participation in international organizations and activities, and promoting the establishment of multilateral cooperation mechanisms to jointly maintain space security and stability remain challenging.
3 Key technologies for interference monitoring
Interference monitoring refers to the process of detecting, identifying, direction-finding, locating, and assessing interference in the electromagnetic environment. Its purpose is to promptly discover and identify interference sources, evaluate the nature and intensity of the interference, and assess its impact on communication and navigation systems, thereby enabling the implementation of appropriate measures to mitigate or eliminate these interference. Figure 5 illustrates the complete interference monitoring process. Initially, specialized equipment and techniques are used to continuously collect signal data from the electromagnetic environment to detect the presence of interference. Subsequently, the detected interference is analyzed to identify its type and characteristics. Next, the impact of the interference on the system is evaluated, and direction-finding is performed to locate the position of the interference source. A monitoring report is then generated and submitted to relevant departments to assist them in making decisions regarding interference suppression [33].
Considering the importance of interference monitoring technology in interference monitoring systems, this section will detail three key technologies for suppression interference monitoring: interference detection technology, interference identification technology, and interference direction finding technology. Simulations will be used to verify the implementation of some of the algorithms involved, and the issues associated with each technology will be pointed out. Based on recent technological trends, the section will also summarize the research directions that warrant further investigation.
3.1 Interference detection technology
3.1.1 Time-domain detection algorithms
The time-domain energy detection algorithm is suitable for detecting high-power blanket interference. It does not require prior information about the signal; it only needs to compare the energy of the signal with a preset energy threshold to determine the presence or absence of interference, regardless of the type of interference. However, it cannot determine the specific frequency points of the interference. The time-domain energy detection method is simple to implement and uses the binary hypothesis testing theory from the field of mathematical statistics.
The signal received by a satellite navigation receiver can be modeled as Equation 1 [34]:
In the model,
The energy of the received signal can be expressed as Equation 2 [35]:
In the equation,
The hypothesis testing problem can be simply expressed as Equation 3:
In the equation,
The energy detection algorithm is significantly affected by noise uncertainty and has a low probability of detecting interference in low SNR conditions. In practical environments, noise is time-varying, and to improve detection probability, multi-node cooperative detection algorithms have been developed. Reference [29] proposes an adaptive multi-threshold energy detection method under time-varying conditions by estimating the noise range. This method offers better detection performance compared to single-threshold energy detection algorithms. Reference [10] suggests using a hard decision strategy for dual-threshold energy detection at individual nodes. The credibility weights are assigned based on the quality of the channel environment at each node, and the final decision is made at the fusion center. This approach achieves good detection performance even under low interference-to-noise ratio (INR) conditions. Wu Jin [36] derived the expression for the error probability in energy detection algorithms and determined the optimal threshold value to minimize this probability, thereby improving detection performance to some extent. Wang Jing [37] adopted a segmented detection method for interference detection in Beidou civilian signals. This method is highly efficient and effective because it focuses on the noise within each sub-band during detection, thereby reducing the influence of noise from other bands and effectively improving the INR during detection.
3.1.2 Frequency-domain detection algorithms
The principle of frequency-domain interference detection is similar, although it may be slightly more complex in terms of computation compared to the time-domain energy detection method. However, it can not only detect the presence of interference but also determine the specific frequency points of the interference [38].
Frequency-domain interference detection often uses the Consecutiveean Excision (CME) algorithm proposed by P. Henttu and S. Aromaa [39]. This algorithm assumes that the initial signal samples do not contain interference signals, and thus the signal spectrum envelope follows a Rayleigh distribution with an expected value as Equation 4:
In the equation,
Based on Equation 5, we can get Equation 6:
Ath is the detection threshold for interference frequency points under the false alarm probability Pfa (the probability of erroneously detecting interference frequency points when no interference signal exists) which is defined as Equation 7.
The threshold factor is defined as Equation 8:
The CME (Consecutiveean Excision) algorithm sets the size of the false alarm probability in advance and obtains the corresponding threshold factor. In each iteration, it calculates the spectral power of the signal set for frequency points that do not contain interference, multiplies this by the threshold factor to update the detection threshold, and then compares the spectral envelope at each frequency point with the detection threshold to classify them into frequency points with and without interference.
Subsequently, P. Henttu et al. [40] proposed the forward sequential mean excision algorithm, also known as the forward consecutive mean excision (FCME) method, to address the problem of impulse interference detection in radio systems. The algorithm first reorders the signal spectra in ascending order according to their energy values, selects a portion of the spectra to form a set of interference-free signals, calculates their energy, and sets a threshold value. If the energy value of the next frequency line is less than the threshold value, this spectrum line is added to the initial signal set to form a new signal set, and its energy is recalculated and a new threshold value is set. Otherwise, the algorithm ends, and the process continues iteratively. Yang Chao et al. [41] proposed an improved CME interference detection algorithm, which sorts the spectrum lines in descending order and considers the mean of the latter half of the spectrum lines as the mean in the absence of interference. This mean is used to initialize the detection threshold, reducing the number of iterations and accelerating the convergence rate of the CME algorithm without decreasing the probability of interference detection. Setting a single threshold has certain issues, such as the possibility of an interference signal with a certain bandwidth having energy below the threshold at a particular frequency point, leading to the misidentification of a single interference signal as two separate ones. Vartiainen et al. [42] addressed this issue by proposing a dual-threshold-based interference frequency point localization algorithm. The main principle of the algorithm is to set high and low detection thresholds. First, the adjacent frequency points of signal samples exceeding the low threshold are clustered, and then the maximum value of the signal spectra in each cluster is compared with the high threshold. If it is greater than the high threshold value, the frequency points belonging to that cluster are determined to correspond to the same interference signal. Otherwise, they are not. Based on this, the algorithm can estimate the bandwidth of the interference.
In frequency-domain interference detection algorithms, traditional Fourier transforms are used. Essentially, these transforms convert one-dimensional time functions into one-dimensional frequency functions, which is a relatively simple transformation method. Its disadvantages are quite apparent: it can only analyze the time-domain characteristics and frequency-domain characteristics of signals independently as a whole, and it cannot analyze the frequency characteristics of signals at specific moments or the time characteristics of signals at specific frequencies. Therefore, it is only suitable for analyzing stationary signals.
3.1.3 Time-frequency detection algorithms
Indeed, when GNSS signals mixed with interference are received by the receiver, they become non-stationary signals. Therefore, time-frequency analysis methods are more suitable for interference detection and analysis. The purpose of time-frequency analysis is to transform one-dimensional time signal functions into two-dimensional joint distribution functions of time and frequency, which can reflect the time-varying characteristics of non-stationary signals [43]. Linear time-frequency analysis is typified by the Short-Time Fourier Transform (STFT), proposed by Dennis Gabor in 1946. The STFT is obtained by multiplying the signal by a sliding time window and then performing a Fourier transform. Due to its linearity and low complexity, the STFT has been used in the development of interference mitigation algorithms, such as those developed by Daniele Borio et al. [44], to estimate the instantaneous frequency of interference. Wang Pai et al. [45] have combined the time-frequency characteristics and statistical properties of received GNSS signals to propose an interference detection algorithm based on the STFT, improving the detection performance of broadband and narrowband interference in low signal-to-noise ratio environments. However, the STFT also has limitations. Because of the windowing process, it is constrained by the Heisenberg uncertainty principle, meaning that the time resolution and frequency resolution cannot be simultaneously optimized.
Comparing different time-frequency analysis methods, quadratic time-frequency analysis based on the Fourier transform of the instantaneous autocorrelation function provides almost the best resolution [8]. Among these, the most commonly used is the Wigner-Ville distribution (WVD), introduced to signal processing in 1948. The WVD can achieve the lower bound of the Heisenberg uncertainty principle and can address some of the issues present in the STFT. However, when analyzing signals with multiple components, the WVD produces cross-term interference, causing the signal energy to spread over areas of the time-frequency plane where there should be no energy, making it difficult to accurately capture the signal features. Choosing appropriate time-frequency analysis methods, such as adaptive kernel time-frequency distributions or linear time-frequency distributions, can suppress cross-terms, but this leads to a degradation in the clustering property of the signal’s time-frequency distribution and increases computational complexity [46]. To address these issues, Sun Kewen et al. [47] analyzed the principles and problems of STFT and WVD, proposing a new time-frequency analysis method based on a reassigned spectrogram for detecting frequency-sweeping interference. This method strikes a good balance between suppressing cross-terms and maintaining time-frequency resolution. Later, he [48] proposed using the Fractional Fourier Transform (FRFT) for detecting satellite navigation interference. The FRFT has excellent detection capabilities for linear frequency modulation (LFM) interference. Xu Huifa [49] and colleagues similarly proposed a new method based on the FRFT to solve the detection and estimation problems of strong and weak LFM signals, improving detection efficiency.
For the common frequency-sweeping interference in satellite navigation systems, many scholars in Professor Sun Kewen’s team have conducted extensive and in-depth research on its detection [22, 50–52]. Their main work involves combining various time-frequency transformation methods to leverage the strengths of each method while compensating for their respective weaknesses. Chen Yuanyuan [50] used the Radon-Wigner transform to detect frequency-sweeping interference and estimate interference parameters. By combining the smoothed pseudo Wigner-Ville distribution based on time-frequency reassignment with the Radon transform, she validated the effectiveness of combining the Radon transform with time-frequency analysis methods for interference detection. Zhao Huizi [51] combined reassignment techniques and wavelet transforms to effectively address issues related to energy concentration, cross-terms, and resolution, thereby improving the accuracy of interference detection. Sun Kewen [52] combined the Hough transform with the Wigner-Ville distribution (WVD) to eliminate cross-term interference and enhance detection sensitivity. The detection performance remains excellent even at an INR of −10 dB.
The FrFT uses a set of orthogonal chirp signals as basis functions. By selecting an appropriate order, the FrFT transforms the chirp signal into the transform domain, where the energy of the chirp signal becomes concentrated, forming a peak. This allows for accurate estimation of interference parameters. Zhang Jun [22] improved the traditional method for determining the optimal order of the FrFT by proposing a combination of the bisection method and discrete polynomial algorithms. This approach reduces the computational complexity of searching for the optimal order while improving search accuracy, enabling the detection of multi-component chirp signals. Zheng Yifei [23] combined the FrFT with traditional time-frequency methods such as the short-time Fourier transform (STFT) and WVD. Compared to these traditional methods, the energy concentration of frequency-sweeping interference is enhanced in the transform domain. At an INR of −8 dB, the accuracy of parameter estimation for frequency-sweeping interference is improved by two orders of magnitude.
In interference detection, time-domain energy detection, frequency-domain energy detection, and time-frequency domain detection primarily utilize the energy distribution characteristics of interference signals in the time domain, frequency domain, and time-frequency domain, respectively. Table 3 summarizes the advantages and disadvantages of these three detection algorithms.
3.1.4 Full blind detection algorithms
In practical interference detection scenarios, the problem is often non-cooperative, making it difficult to obtain sufficient prior information. Therefore, researching fully blind interference detection algorithms holds greater practical significance.
Blind interference detection algorithms based on random matrix theory have been proposed. Two typical algorithms are the Covarianceatrix-based All-Blind Detection (CAV) [53] and the Eigenvalue-based All-Blind Detection (BDA) [54]. The CAV algorithm constructs a test statistic as the ratio of the sum of the absolute values of all elements in the covariance matrix of the received signal to the sum of the absolute values of the diagonal elements. The BDA algorithm constructs a test statistic as the ratio of the maximum eigenvalue to the minimum eigenvalue. These algorithms have detection thresholds that are independent of noise information, thus completely overcoming the limitation of energy detection algorithms being sensitive to noise uncertainty. They also exhibit good detection performance even at low SNR. Based on these foundations, many researchers have conducted more in-depth studies on all-blind detection algorithms [55–61]. Their work includes developing new covariance-based decision statistics to address the computational complexity of decision metrics and thresholds, or combining these algorithms with cooperative sensing to further improve detection performance and optimize network overhead.GNSS interference detection can benefit from spectrum sensing techniques. Wu Jin [36] has introduced all-blind detection algorithms into the interference detection of the Beidou system and proposed a weighted fusion detection (WFD) algorithm, which enhances detection performance.
In recent years, with the rise of artificial intelligence, machine learning has been increasingly applied in various fields due to its excellent classification performance. In the context of all-blind detection algorithms, interference detection is essentially a binary classification problem, which aligns well with machine learning algorithms. Based on this, many scholars have introduced machine learning into full-blind detection. Reference [62] combines the traditional K-Nearest Neighbors (KNN) algorithm to achieve the detection task. Yao Di [63] combines the Support Vectorachine (SVM) to perform binary classification tasks for spectrum sensing. These algorithms effectively address the issue of low detection probability under low signal-to-noise ratio (SNR) conditions and offer high detection efficiency. However, they require manual construction of feature vectors, which can significantly impact the classification results. Shi Haodong [64] uses a Convolutional Neural Network (CNN) to achieve collaborative spectrum sensing. Lu Huachao [65] directly inputs the normalized covariance matrices of the combined I and Q signals from each node into the neural network, allowing the network to automatically extract useful features for detection. This approach yields good detection performance.
3.1.5 Summary
Time-domain energy detection algorithms fall into the category of semi-blind detection algorithms, as they require prior information related to the noise. Due to this requirement, their detection performance is poor at low INR and they are highly susceptible to noise uncertainty. However, they are easy to implement and do not require sophisticated detection equipment. Improvements through multi-node cooperative energy detection can somewhat alleviate these issues, but the enhancement is limited and increases the overhead of the detection network.
Full-blind detection algorithms do not require any prior information about the received signals and are independent of the noise environment. They maintain good detection performance even at low SNR. However, these algorithms are based on covariance matrix decomposition, which involves significant computational complexity. As a result, they may not meet the real-time interference detection requirements in practical applications.
The limitations of the aforementioned methods are evident; they can only detect the presence of interference but provide no information about the interference parameters, making interference suppression challenging. Time-frequency detection methods and frequency-domain detection methods, on the other hand, can not only detect the presence of interference but also estimate the interference parameters, thereby facilitating interference suppression. Time-frequency detection methods are particularly suitable for detecting non-stationary interference. Combining various time-frequency transformation methods can reduce parameter estimation errors. However, these methods are computationally complex and the interpretation of the transformation results is challenging.
3.2 Interference identification technology
Blanket interference can be classified in various ways. Based on the time-domain characteristics of the interference, it can be divided into pulse interference and continuous wave interference. According to the stationarity of the interference, it can be categorized into stationary interference and non-stationary interference. Furthermore, it can be classified into broadband interference and narrowband interference according to the spectral width. Narrowband interference has a bandwidth narrower than the GNSS signal, while broadband interference has a bandwidth wider than the GNSS signal [66]. Taking the GPS L1 frequency band with a spread spectrum code of CA as an example, common interferences include matched spectrum interference [67], Gaussian noise interference, linear frequency modulation (LFM) interference [68], pulse interference [69], continuous wave interference [70], etc.
3.2.1 Traditional pattern recognition methods
Radio communication has a relatively long history, with initial reliance on manual methods for identifying modulation schemes of radio signals. However, these manual identifications were significantly influenced by subjective factors and were both time-consuming and labor-intensive. As communication technology advanced, the advantages of automatic modulation recognition became increasingly apparent, eventually evolving into the mainstream approach for identification. Traditional modulation recognition methods can be broadly categorized into two types: decision-theoretic methods and statistical pattern recognition methods [71]. While decision-theoretic methods require substantial computational power and extensive prior information, the relevant parameters associated with the signals to be identified are often unknown. As a result, statistical pattern recognition methods have gained wider acceptance and are more commonly applied.
The pattern identification method includes three modules: signal preprocessing, signal feature parameter extraction, and signal identification classifier design, as shown in Figure 6. Firstly, the received signal is preprocessed, including noise removal, data normalization, unknown parameter estimation, etc. Then, the signal set to be classified is analyzed in different signal domains such as time domain and frequency domain, and the features that can clearly distinguish the signal types in the set are extracted to form feature vectors. This module mainly relies on machine learning methods [72] and pattern identification theory [73], and finally the signal samples to be tested are input into the classifier to realize signal identification.
A good feature parameter should easily highlight the differences between signals, significantly reducing the burden on subsequent classifiers and facilitating the identification of different signals. Azzouz, EE, and Nandi, Ak [74–76] published several papers between 1995 and 1998 on extracting time-domain feature parameters for analog and digital signals. Their work included various typical algorithms for extracting signal instantaneous features. Later scholars built upon this foundation to conduct more research on automatic modulation identification. A good identification classifier should achieve high signal identification rates. Commonly used identification classifiers are based on machine learning and include: decision tree (DT) classifier [77], support vector machine (SVM) classifier [78] and neural network (NN) classifier.
Some scholars in the field of satellite navigation interference identification have drawn on the method of automatic signal modulation identification to identify typical interference types in satellite navigation systems. Huang Ting [30] analyzed the characteristics of pulse interference and continuous wave interference, and provided the results of typical suppression interference characteristic analysis, which provided ideas for selecting appropriate characteristic parameters. Lei Liang [79] did similar work, and Li Jian et al. [80] extracted pulse width estimates, bandwidth ratios, and frequency modulation slopes to conduct identification simulation experiments on six typical interferences. When the signal to noise ratio (SNR) is 3dB, the identification rate reaches 90%. Zhu Pengcheng [10] analyzed the typical interference of GPS and Beidou systems from the time domain, frequency domain, time-frequency domain, and high-order cumulants, extracted feature values composed of high-order cumulants, normalized spectral bandwidth, and other parameters, and used decision tree classifiers for identification. The simulation results show that the identification effect is very good when the INR is large. Some of the selected features are greatly affected by noise, and when selecting a decision tree classifier, the classification threshold is generally not changed once selected, which is not adaptive. Therefore, when the INR is small, the identification effect is not ideal.
Ye Rui [81] also did interference identification work, but he used the KNN (K Nearest Neighbors) algorithm based on the traditional decision tree to calculate the distance between the test samples and the training sample eigenvalues for classification, eliminating the subjective factors brought about by manually setting thresholds, and the identification rate has been improved to a certain extent. However, when the number of samples is large, the calculation of this method is very large. Combining DTs and SVMs and directly bringing test sample data into the maximum classification interval function trained by the support vector machine can solve this problem. The amount of computation and identification effect are the best among these three methods.
The use of neural network classifiers is becoming more and more common. Lu Dongsheng et al. [82] analyzed six types of interference, extracted 13 characteristic parameters to obtain feature vectors, and constructed a CNN (Convolutional Neural Network) + LSTM (Long Short Termemory) double-layer network model for training. Compared with the LSTM network in two scenarios of strong signal interference and interference with similar power, the accuracy, mean square error, and truthfulness are all better.
Based on the research of previous scholars, the characteristics of three commonly used identification classifiers are summarized in Table 4.
In statistical pattern identification methods, the selection of feature parameters and classifiers lacks a theoretical basis. Generally, for a specific identification task to be completed, the selection can only be made based on existing experience and through multiple trials and errors. This leads to the method being exceptionally sensitive to the selection of feature parameters, where choosing different feature parameters may ultimately result in different identification effects. This lack of flexibility results in poor identification rates for interference signals.
3.2.2 Interference recognition method based on deep learning
In 2006, the concept of deep learning was officially proposed [83]. Deep learning networks are composed of multiple layers of neurons, each layer serving different functions and purposes. Common types of layers include convolutional layers, pooling layers, and fully connected layers. Convolutional layers are used to extract local information features from the input data. Pooling layers are used to down-sample the input feature maps, retaining the most important features while reducing the computational load. Fully connected layers learn high-level abstract features from the input data and are typically used as the output layer to perform classification tasks. During the training process of a deep learning network, forward propagation and back-propagation algorithms are utilized. Non-linear activation functions are used in each layer to introduce non-linearity, enabling the network to learn complex patterns and features. The trained model ultimately achieves excellent performance in various tasks. Since then, Deep Neural Networks (DNNs) have been increasingly used by scholars as end-to-end systems for identification tasks. These networks can receive raw data, automatically learn from it, and optimize themselves to ultimately complete the identification task [84], thereby avoiding the complex feature parameter extraction issues present in traditional pattern identification methods.
In the field of recognition, converting one-dimensional interference data into two-dimensional image data and combining it with deep learning for classification has become a mainstream approach in recent years. Li Xiangjun et al. [85] proposed an interference type identification method relying on the SqueezeNet CNN model and the smoothed WVT, aiming to address the problem of low interference identification rates. Iman Ebrahimiehr et al. [86] used the WVT and spectrogram to perform time-frequency analysis on different types of chirp signals, utilizing the analysis results to create an image dataset, part of which was used for training the model and part for identification testing. Chen Xin et al. [87] proposed an interference fingerprint spectrum (FPS) consisting of time-frequency and time-power characteristics of signals, and selected the GoogLeNet DNN architecture as the training model to design the FPS-DNN interference classifier. This classifier significantly improves the identification rate under low interference power conditions and can be extended to solve more complex interference classification problems. Reference [88] uses the power spectral density (PSD) of the received signal as the input feature for the network. Compared to algorithms such as Random Forest and SVM, this approach improves recognition accuracy.
To improve recognition performance, new deep neural network models have been continuously proposed [89]. established two CNN networks that can share parameters, adding the Kullback-Leibler (KL) divergence and Euclidean distance of extracted features as new loss functions. This enables the network to learn the relationships between interference signal categories, enhancing generalization capability and recognition performance at low interference-to-noise ratios (INRs) without increasing network complexity [90]. constructed images from one-dimensional signals and used residual networks to extract multi-semantic features, followed by multi-semantic feature fusion. This approach helps the deep learning network extract more distinctive signal features, thereby improving interference recognition performance [91]. used spectrograms as the training dataset and introduced multi-head attention modules and residual convolutional modules to address the different effects of varying window lengths on Short-Time Fourier Transform (STFT) results. This resulted in improved recognition performance [92]. proposed a new method based on graph models, introducing graph signals and graph neural networks to identify the modulation categories of unknown interference signals. Their method enhances channel information interaction and extracts both local and global features, significantly improving recognition performance.
Deep learning-based recognition methods have several advantages. They can automatically learn features from data, reducing the dependence on expert knowledge. As the amount of training data increases, the performance of the model often improves significantly, demonstrating good generalization capabilities and the ability to identify interference signals in different environments and conditions. However, deep neural network models are often very complex, requiring long training times and consuming substantial network resources during recognition tasks, which can sometimes lead to resource wastage. To address these issues [93]: focused on the challenges of GNSS interference recognition in low-resource environments, emphasizing preprocessing. They proposed a method that combines traditional statistical signal processing with machine learning, effectively reducing model complexity and resource consumption [94]. Introduced a time-frequency component-aware convolutional neural network (TFC-CNN) that can determine the positions of time-frequency components in time-frequency images and perform convolution operations at these positions. During network training, an adaptive forward propagation algorithm is used to dynamically decide the depth of forward propagation based on the samples, improving the computational efficiency of interference classification and reducing resource wastage [95]. Proposed a neural network classification method that combines federated learning and transfer learning. Federated learning is used to distribute data, enhancing resource efficiency and privacy protection, while transfer learning accelerates the model learning process. Compared to traditional CNN models, this method improves classification accuracy by 8%.
3.2.3 Summary
Traditional pattern recognition methods have advantages in computational efficiency and interpretability, but they have limitations in feature engineering and data adaptability. In contrast, deep learning-based recognition methods excel in automatic feature extraction, generalization capabilities, and handling complex data, but they face challenges in computational resource requirements and interpretability.
Deep learning-based recognition adopts an end-to-end learning approach, where raw data is fed into the network model to directly obtain classification results. However, there is a wide variety of deep learning network models, and no theoretical method has been provided to guide the selection of models based on specific recognition problems. Additionally, there is no quantitative explanation for why the output results of a model are good or bad. Instead, people rely on their experience to try different network models iteratively to achieve better results. Improving the interpretability of models to make the decision-making process more transparent, understandable, and trustworthy is an area worthy of further research in deep learning models.
Currently, most classifiers use supervised learning, which can only recognize a few specific types of interference. When new types of interference appear, the overall recognition performance may deteriorate. This is a drawback of feature learning with labeled data. Research on feature learning from unlabeled data and techniques for automatically adding classification labels to unlabeled data [79] is necessary.
3.3 Interference direction finding technology
Accurately determining the direction of interference sources in satellite navigation systems can help people quickly locate them. Although the construction of satellite navigation systems started relatively late and has had a shorter development time compared to the advancement of radio technology, there are numerous methods for radio direction finding. The principles of satellite navigation interference direction finding and ordinary radio direction finding techniques are consistent, with the difference lying in the specific application scenarios. To address the issue of interference direction finding in satellite navigation systems, inspiration can be drawn from radio direction finding techniques.
3.3.1 Traditional direction finding algorithms
The main interference direction finding methods include amplitude comparison direction finding, phase comparison direction finding, and spatial spectrum estimation. The specific algorithms are shown in Figure 7. Among them, the first two are traditional direction finding methods, which are based on the amplitude information or phase information of the interference received by the antenna, respectively. These are the direction finding methods adopted by scalar direction finding systems. The latter benefits from the development of spatial spectrum estimation technology, which is based on both the phase and amplitude information of the interference received by the antenna. It is the direction finding method adopted by vector direction finding systems [96].
Interference direction finding can be performed using either a single antenna or an antenna array. Figure 8 showcases three commonly utilized antenna array models: linear arrays, circular arrays, and planar arrays.
Amplitude comparison direction finding relies on differences in signal amplitude, making it susceptible to noise and resulting in suboptimal direction finding outcomes. Phase comparison direction finding, also known as interferometer direction finding, relies on phase variations that contain more precise directional information. Due to its high accuracy and speed, it is widely used, including in phase interferometer methods and correlative interferometer methods. Phase interferometer-based direction finding utilizes the phase difference of interference signals received by antenna elements on a baseline. Taking single-baseline interferometer direction finding as an example, its schematic diagram is shown in Figure 9.
Assuming there are two antennas with a baseline length of
When the signals received by the two receivers from the antennas are fed as inputs to the phase discriminator, the output of the phase discriminator is the phase difference
After undergoing an angle transformation, we can obtain Equation 12:
Upon observing Equations 10, 11, if we want to ensure that for any angle
Otherwise, there will be an integer multiple difference between the two, which is known as the phase ambiguity problem in phase-based direction finding methods. To obtain the correct angle of arrival (AOA) of the signal, it is necessary to solve for the ambiguity number. There are already many methods to resolve this ambiguity, such as the long-short baseline method, the Chinese Remainder Theorem method [97], and so on.
It is evident that the direction finding performance is influenced by the baseline length. A longer baseline results in higher direction finding accuracy but also introduces the issue of phase ambiguity. On the other hand, a shorter baseline eliminates the phase ambiguity problem, but it can lead to mutual coupling between antenna elements, which reduces the direction finding accuracy and limits the signal bandwidth.
The correlative interferometer direction finding method can overcome the phase ambiguity problem. Its principle involves selecting several antenna pairs to obtain the phase differences of known incoming wave signals from all directions and frequencies as the original phase samples. For an unknown incoming wave signal to be measured, only the phase difference measured by the antenna pair is required, and this is then correlated and interpolated with the phase samples. The angle corresponding to the maximum correlation value is determined as the angle of arrival [98].
3.3.2 Direction finding algorithms based on spatial spectrum estimation
Although traditional direction finding technologies are mature, they are constrained by factors such as array size, beamwidth, and the direction finding environment. In practical applications, their direction finding accuracy and spatial resolution sometimes fail to meet requirements, especially in multipath environments where direction finding performance is poor. Spatial spectrum estimation techniques, developed in the 1960s and 1970s based on minimum variance spectral estimation, can address some of the issues with traditional direction finding methods to a certain extent.
Spatial spectrum estimation can be categorized into subspace fitting algorithms and subspace decomposition algorithms. The former category is typically represented by the maximum likelihood (ML) method, which constructs a logarithmic likelihood function based on the signal model and solves for unknown parameters to obtain the direction of arrival (DOA) of signals. This method remains effective for coherent signal direction finding and performs well even at low SNR, but its processing efficiency needs to be improved [99]. The latter category is predominantly represented by the MUSIC (Multiple Signal Classification) algorithm [100], which works by performing an eigen decomposition on the array output signals. The resulting noise eigenvectors and signal eigenvectors span the noise subspace and signal subspace, respectively. The spatial spectrum is estimated by utilizing the orthogonality between these subspaces, and the DOA is estimated by searching for spectral peaks. The MUSIC algorithm offers relatively lower complexity and computational requirements while achieving high direction finding accuracy. However, the actual electromagnetic environment is much more complex than the theoretical assumptions, leading to suboptimal direction finding results in some electromagnetic conditions. For example, under the influence of multipath effects, the presence of coherent signals can cause the array manifold matrix to become rank-deficient, resulting in poor direction finding performance. In such cases, decorrelation algorithms such as spatial smoothing algorithms are first applied to restore the array manifold matrix to a full-rank state [101]. The ESPRIT (Estimating Signal Parameter via Rotational Invariance Techniques) algorithm, proposed by Roy and Kailath [102], does not require spectral peak searching and has a lower computational burden but may exhibit reduced measurement accuracy compared to MUSIC.
We select an 8-element linear array and set up 3 incoming wave signals with different DOA. These signals have similar powers, and the INR is set to 10 dB for all of them. Among these signals, two are coherent. We conduct simulations using the MUSIC algorithm directly and after applying spatial smoothing to the signals, respectively. The purpose of these simulations is to verify the correctness of the analysis on the direction finding performance of the MUSIC algorithm. The simulation results are presented in Figure 10.
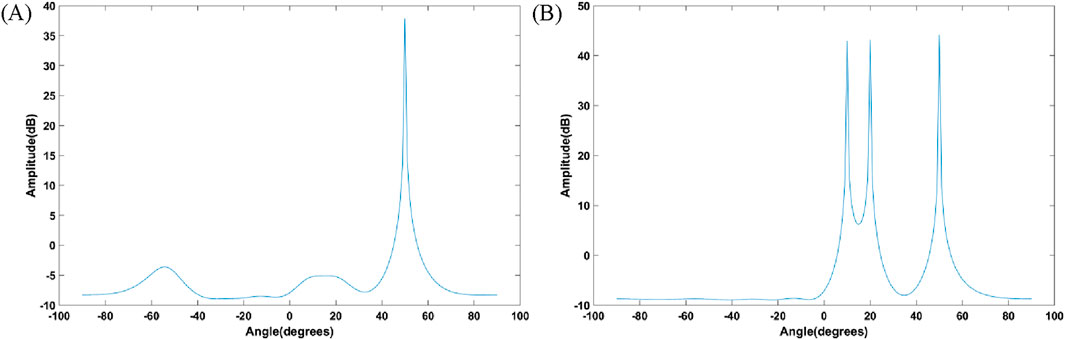
Figure 10. Simulation of Direction Finding by MUSIC Algorithm. (A) Direct MUSIC Direction Finding. (B) MUSIC Direction Finding after Spatial Smoothing.
Table 5 summarizes the characteristics of the aforementioned radio direction-finding techniques, which are currently being applied in interference direction-finding for satellite navigation systems. Interferometer-based direction finding methods offer fast speed and high accuracy, and some researchers have specifically designed GNSS interference direction finding antennas to address issues such as phase ambiguity and reduce mutual coupling effects between array elements [103]. A significant number of satellite navigation interference direction finding and localization equipment employ correlative interferometer direction finding methods to achieve precise direction finding of interference signals [104]. Scholars from Beijing Jiaotong University have conducted simulations under ideal conditions, using a four-element rectangular array and MUSIC and its improved algorithms to estimate the DOA of typical incoherent narrowband interference, coherent narrowband interference, and broadband interference signals in BDS. Their results show good direction finding performance [29, 104, 105].
In practical engineering applications, however, direction finding of interference signals must take into account the impact of adverse factors such as mutual coupling between array elements and boundary effects, which can lead to amplitude and phase errors in the array elements that affect the accuracy of spatial spectrum estimation-based direction finding. To achieve direction finding results comparable to those under ideal conditions, active calibration methods can be employed, where the gain patterns of the antenna array elements are calibrated using specialized equipment to estimate the amplitude-phase error matrix and mutual impedance matrix, which are then used to correct the obtained spatial spectrum [105]. Alternatively, an error cost function can be constructed to estimate the amplitude-phase error matrix, which is then incorporated into a DOA error estimation model to achieve real-time correction [106]. With the popularity of neural network models, methods have gradually emerged that use CNN to perform phase correction on direction-finding channels [107].
As the electromagnetic environment becomes increasingly complex, it is essential to select the appropriate direction finding algorithm for different scenarios. To fully leverage the advantages of various direction finding methods and improve the results, a trend is emerging towards combining multiple direction finding techniques for interference direction finding. For instance, the maximum signal method employs high-gain directional antennas, offering high sensitivity but relatively low accuracy. In contrast, the correlative interferometer uses omnidirectional antennas, providing low sensitivity but high accuracy. By combining these two methods, it is possible to simultaneously achieve high accuracy and sensitivity in direction finding [108]. Additionally, the correlative interferometer boasts fast direction finding speeds, while the MUSIC algorithm excels in accuracy. By first using the correlative interferometer to quickly determine the direction of the interference signal, the search range of the MUSIC spatial spectrum can be narrowed, significantly reducing the computational load. The combination of these two techniques enables fast and high-precision direction finding [109, 110].
3.3.3 Direction finding algorithms based on timeodulated array
Although traditional direction-finding techniques have demonstrated good performance in relatively simple scenarios, they are increasingly showing limitations as the electromagnetic environment becomes more complex. For example, traditional methods struggle to address direction-finding issues in complex situations such as weak signal strength, wide bandwidth, high frequency bands, and the simultaneous presence of multiple interference sources. As a result, their application scope in modern complex electromagnetic environments is limited. Consequently, researchers have begun exploring new direction-finding techniques to overcome these challenges. In recent years, direction-finding methods based on Time modulated Arrays (TMAs) have emerged, offering new approaches to solving these problems.
A TMA is a novel type of antenna array that introduces switches at the RF front-end of a traditional antenna array to periodically time-modulate the incoming wave signals received by each antenna element. The modulated signals are then processed through a single channel by the signal processing module, extracting harmonic components that contain DOA information. By analyzing the relationships between these harmonic components, DOA estimation can be achieved. Because it introduces the time variable into the antenna array, TMA is also referred to as a four-dimensional antenna array [111]. He Chong [112] used a binary TMA to calculate the fundamental and first harmonic components of the TMA output signals. The incident angle of the incoming wave was estimated using the ratio of the harmonic component to the fundamental component. Chen Jingfeng [113] proposed a direction-finding technique based on multi-harmonic analysis, fully utilizing the angle information contained in each harmonic component. The generalized least squares estimation method was used to estimate the direction of the incoming wave signal, and selecting an appropriate number of harmonics significantly improved direction-finding performance even at low SNR. Compared to traditional algorithms that rely on multiple channels to complete direction-finding tasks, TMA uses a single channel, avoiding direction-finding errors caused by inconsistencies between channels [114]. By integrating techniques such as multi-beam arrays and spectrum feature analysis, TMA can achieve precise DOA estimation while reducing system complexity and cost [115–117]. To address direction-finding for broadband signals and potential phase ambiguity issues during the direction-finding process [118], proposed applying different periodic time modulation to different array elements. This approach independently maps the amplitude information of each antenna unit to different harmonic frequencies, avoiding phase ambiguity caused by carrier frequency variations over a wide band, thus extending the direction-finding bandwidth [119]. used channelization to divide broadband signals into multiple sub-bands, converting the broadband direction-finding problem into multiple narrowband direction-finding problems. The DOA of the broadband signal was then estimated through weighted integration [120]. introduced virtual baseline technology into TMA, further enhancing its direction-finding capabilities.
3.4 Summary
After years of development, traditional direction-finding methods have been extensively studied and applied in various fields such as communications, radar, and navigation. They have a solid theoretical foundation and technical accumulation, and for most conventional direction-finding tasks, their accuracy and stability meet the requirements. However, their performance in complex electromagnetic environments is not ideal. Direction-finding algorithms based on TMA offer several advantages through time modulation and spatial synthesis, including high angular resolution, strong interference resistance, low hardware complexity, and robustness. These features make TMA-based methods particularly suitable for direction-finding in complex electromagnetic environments.
Currently, TMA-based direction-finding methods, as a new technology, are still not fully mature and are primarily focused on theoretical research. Translating these methods into practical applications requires overcoming many technical barriers, such as high-precision clock sources, complex control circuits, and high-performance digital signal processing units. With advancements in technology and cost reductions, TMA-based methods are expected to see widespread application in complex electromagnetic environments, representing an important direction for the development of direction-finding technology. Meanwhile, traditional direction-finding methods will continue to play an irreplaceable role in mature fields.
4 Future development trends in interference monitoring
Currently, the transmission of information is generally achieved through the propagation of electromagnetic waves, where various useful signals overlap with useless interference and noise present in the space, posing significant challenges for interference monitoring. Through the analysis of key technologies for satellite navigation system interference monitoring, it can be anticipated that future interference monitoring will face even more severe challenges, with a focus on the development of interference monitoring technologies that offer high precision, high sensitivity, and high real-time performance.
4.1 Highly sensitive real-time interference detection
As interference technology advances, the power required to generate the same interference effect on GNSS receivers is decreasing. Moreover, when multiple interferences with significantly different power levels coexist, the lower-power interference can be overwhelmed by the higher-power interference, leading to a high probability of missed detections during interference monitoring and posing potential assessment risks. Detection equipment monitors interference within its vicinity. Enhancing the sensitivity of interference detection equipment can effectively detect low-power interferences, expand the range of interference detection, reduce the number of devices required for full-area monitoring, and lower the cost of the monitoring system.
Furthermore, if interference detection equipment can quickly identify interference, people can promptly take interference suppression measures to reduce its harmful effects. This requires the detection equipment to adopt low-complexity detection algorithms while ensuring sensitivity. This requires the detection equipment to ensure sensitivity while also maintaining real-time performance.
4.2 Intelligent automatic interference identification
GNSS receivers are sometimes subjected to more than one type of interference simultaneously, and the impacts of different types of interference on them are generally different. Separating and identifying these mixed interferences individually allows for an analysis of the effects of each on the terminal equipment, facilitating more informed decision-making. To achieve better interference effects, new types of interference continue to emerge. For previously identified interference types, rapid identification of their types should be possible upon re-interference, which can be achieved by establishing an interference library. For new types of interference that have never been identified before, the monitoring system’s identification should also possess a certain degree of generalization ability, correctly identifying the new type of interference and adding it to the interference library.
With technological advancements, satellite navigation interference monitoring can integrate artificial intelligence, machine learning, and other technologies. Through self-learning and evolution, intelligent algorithms can continuously adapt to changing interference characteristics, achieving automatic identification and classification of interference signals with high accuracy. Furthermore, they can even predict the occurrence of interference. This deep integration of technologies can save significant human resources and greatly enhance efficiency and accuracy.
4.3 High-precision interference direction finding under complex conditions
Typically, the interference monitoring equipment and the interfered terminals are not located at the same geographical position, making the direction of interference arbitrary for the monitoring equipment. In complex terrain conditions such as “urban canyons,” mountainous regions, jungles, or in the presence of moving obstructions or drastic meteorological changes, the interference signals are prone to various physical phenomena during propagation, including reflection and refraction, which can lead to multipath effects. These effects can reduce the accuracy of direction finding, cause ambiguity in direction finding, and result in unstable direction finding outcomes. The precision of interference direction finding directly impacts the results of interference source localization. If the interference source is located far from the monitoring equipment, even a slight deviation in the direction finding angle can result in a significant discrepancy between the localized position of the interference source and its actual location.
The premise of direction finding for multipath signals is to extract the direct interference from the detected interference signals. Improvements and optimizations to multipath resolution algorithms can be made in terms of real-time performance, implementation difficulty, and complexity. By integrating direction finding with generative AI (Artificial Intelligence) technologies, an adaptable direction finding model can be constructed that automatically adapts to complex and dynamic propagation environments, enhancing the intelligence level of the direction finding system. Furthermore, the direction finding system can introduce multi-modality and perform data fusion to address multipath interference issues.
4.4 Comprehensive and large-scale interference monitoring
Currently, the development of the GNSS interference monitoring network in China is still incomplete, and the capability for comprehensive interference monitoring across the entire region remains inadequate. The evolution of the monitoring network should target intelligence and automation, fully leveraging artificial intelligence, big data, and cloud computing technologies to enable real-time analysis of vast amounts of monitoring data.
Joint monitoring is a necessary means for interference monitoring. On one hand, emphasizing multinational joint monitoring on a global scale and strategically deploying interference monitoring stations worldwide can ensure comprehensive coverage. On the other hand, it is crucial to develop and integrate various interference monitoring platforms, including ground-based, air-based, space-based, and sea-based systems, to effectively tackle complex monitoring environments.
5 Conclusion
Interference monitoring serves as an indispensable cornerstone for maintaining the robust operation of various systems, playing a crucial role in ensuring their performance. Focusing on the domain of GNSS interference monitoring, the current system faces unprecedented challenges in multiple aspects, including technological iteration, infrastructure construction, and international collaboration. To gain a profound understanding of the essence of these challenges and explore effective strategies to address them, this paper systematically traces the developmental history of interference monitoring systems since their inception. It provides a comprehensive and in-depth analysis of the intrinsic mechanisms and unique characteristics of several core interference monitoring technologies. Building on this foundation, the paper reviews the breakthrough advancements in these key technologies over recent years. It delves deeply into the enhanced understanding of technical principles and broadly explores the continuous expansion and innovation in application domains. Through detailed examination and analysis, we gain insights into the significant potential of these technologies in improving monitoring accuracy, enhancing system robustness, and driving technological innovation. Through the review and analysis presented in this paper, we aim to provide scholars in the relevant fields with a comprehensive and in-depth report on interference monitoring technologies. We hope to inspire their innovative thinking and research enthusiasm, contributing valuable wisdom and strength to the continuous advancement and widespread application of interference monitoring technologies.
Author contributions
YH: Writing–original draft, Writing–review and editing. BL: Writing–review and editing. JC: Writing–review and editing. ZW: Writing–review and editing. WX: Writing–review and editing. ZL: Writing–review and editing.
Funding
The author(s) declare that financial support was received for the research, authorship, and/or publication of this article. National Natural Science Foundation of China under Grant U20A20193 and Independent scientific research project of key military scientific research laboratory of satellite navigation technology under Grant WXDHS2023110.
Conflict of interest
Author ZW was employed by Transcom (Shanghai) Technology Co., Ltd.
The remaining authors declare that the research was conducted in the absence of any commercial or financial relationships that could be construed as a potential conflict of interest
Publisher’s note
All claims expressed in this article are solely those of the authors and do not necessarily represent those of their affiliated organizations, or those of the publisher, the editors and the reviewers. Any product that may be evaluated in this article, or claim that may be made by its manufacturer, is not guaranteed or endorsed by the publisher.
References
1. Hegarty CJ, Chatre E. Evolution of the global navigation satellite system (GNSS). Proc IEEE (2008) 96(12):1902–17. doi:10.1109/jproc.2008.2006090
2. Yalvac S. Investigating the historical development of accuracy and precision of Galileo by means of relative GNSS analysis technique. Earth Sci Inform (2021) 14(1):193–200. doi:10.1007/s12145-020-00560-8
3. Bonnor N. A brief history of global navigation satellite systems. The J Navigation (2012) 65(1):1–14. doi:10.1017/s0373463311000506
4. Xie J, Kang CB. Engineering innovation and the development of the BDS-3 navigation constellation. Engineering (2021) 7(5):558–63. doi:10.1016/j.eng.2021.04.002
5. Santra A, ahato S, andal S, Dan S, Verma P, Banerjee P, et al. Augmentation of GNSS utility by IRNSS/NavIC constellation over the Indian region. Adv Space Res (2019) 63(9):2995–3008. doi:10.1016/j.asr.2018.04.020
6. Li XP, Pan L, Yu WK. Assessment and analysis of the Four-Satellite QZSS precise point positioning and the integrated data processing with GPS. IEEE Access (2021) 9:116376–94. doi:10.1109/access.2021.3106050
7. Yan K. Research on GNSS vulnerability simulation and assessment platform technology. Shanghai: Shanghai Jiaotong University (2013) p. 1–6.
8. Li XJ, Lu ZK, Yuan MZ, Liu WX, Wang FX, Yu Y, et al. Tradeoff of code estimation error rate and terminal gain in SCER attack. IEEE Trans Instrum Meas (2024). doi:10.1109/TIM.2024.3406807
9. Ioannides RT, Pany T, Gibbons G. Known vulnerabilities of Global Navigation Satellite Systems, status, and potential mitigation techniques. Proc IEEE (2016) 104(6):1174–94. doi:10.1109/jproc.2016.2535898
10. ROIIT. Top 10 GPS spoofing events in history (2024) Available from: https://threat.te-chnology/top-10-gps-spoofing-events-in-history/ (Accessed March 15, 2024).
11. Zhu PC. Research on GNSS interference detection and identification technologies. Chongqing: Chongqing University (2018) p. 41–57.
12. Wu RB, Wang WY, Lu D, Wang L, Jia Q. Adaptive interference suppression technology of GNSS. Beijing: First Edition (2015).
13. Lv DK, Zhang YJ. A space-time-frequency domain combined anti-jamming technique for GPS. Electron Des Eng (2019) 27(01):94–8. doi:10.14022/j.cnki.dzsjgc.2019.01.019
14. Song J, Lu ZK, Liu Z, Xiao ZB, Dang C, Wang ZY, et al. Review on the time domain interference suppression of navigation receiver. Syst Eng Electronics (2023) 45(04):1164–76. doi:10.12305/j.issn.1001-506X.2023.04.25
15. Zhang Z, Deng K, Zhang Y, Liu YL, Zhu LD. Research on radio frequeney domain interference cancellation technology for non-cooperative signals. Radio Commun Technology (2024) 1–10.
16. Li G. Analysis ofultidimensional domain anti-interference technology in satellite communication. Electron Technology (2024) 53(05):248–9. doi:10.3969/j.issn.1000-0755.2024.05.111
17. Zheng BW, Liu H, Zhai GW, Meng MZ, Li HP. Research on airspace anti-jamming technology based on variable step size LMS algorithm. J Telemetry,Tracking Command (2024) 45(04):75–80. doi:10.12347/j.ycyk.20240228001
18. Qiao J, Lu ZK, Lin BJ, Song J, Xiao ZB, Wang Z A survey of GNSS interference monitoring technologies. Front Phys (2023) 11. doi:10.3389/fphy.2023.1133316
19. Borio D, O’Driscoll C, Fortuny J. GNSS jammers: effects and countermeasures. In: 6th ESA workshop on satellite navigation technologies (navitec 2012) and European workshop on GNSS signalsand signal processing. Noordwijk, Netherlands: IEEE (2012) p. 1–7.
20. Zhao HZ. Research on interference detection technology based ontime-frequency analysis in GNSS receiver. Anhui: Hefei University of Technology (2017) p. 26–40.
21. Wang P. Detection anditigation of chirp-style jammer for global navigation satellite systems receivers. Beijing: Beijing Institute of Technology (2018) p. 80–90.
22. Zhang J. Research on fractional time-frequency analysis based anti-interference techniques for GNSS receivers. Anhui: Hefei Univ Technology (2020) 28-30:44–5. doi:10.27101/d.cnki.ghfgu.2020.001935
23. Zheng YF. Satellite navigation interference detectionethod based on fractional time-frequency transform. Anhui: Hefei University of Technology (2022) p. 36–7. doi:10.27101/d.cnki.ghfgu.2022.000842
24. Chen G. American civil aviation radio interference monitoring system. China Radio 2004 (4):62–3. doi:10.3969/j.issn.1672-7797.2004.04.022
25. Fan J, Li HY, Fei W, Li JC. The construction of an integrated platform to achieve a new leap in radio monitoring. China Radio (2016) (4) 26–9. doi:10.3969/j.issn.1672-7797.2016.04.010
26. Picture of disturbance monitoring direction finding machine. (2024). Available from: https://cn.bing.com/images/search?q=%E5%B9%B2%E6%89%B0%E7%9B%91%E7%9B%91%E6%B5%8B%E6%B5%8B%E5%90%91%E6%9C%BA%E5%9B%BE%E7%89%87&form=IQFRML&first=1. [Accessed, March 15, 2024].
27. Wang SL, Luo JS, Wei P, Hu L, Lai PH, Wang H, et al. Cognitive communication anti-jamming. Beijing: First Edition (2023).
28. Simonsen K, Suycott M, Crumplar R, Wohlfiel J. LOCO GPSI: preserve the GPS advantage for defense and security. IEEE Aerospace Electron Systemsagazine (2004) 19(12):3–7. doi:10.1109/maes.2004.1374060
29. Guo XQ. Study on interference detection and identification technology of Beidou satellite navigation. Beijing: Beijing Jiaotong University (2018).
30. Huang T The key technology research on blanketing jamming monitoring of satellite navigation system, 2-5. Hunan: Hunan University (2014) p. 15–9.
31. HawkEye 360. (2024). Available from: https://www.he360.com/our-products/. [Accessed, March 15, 2024].
32. Hao CY. Overview of Hawkeye 360space-based radio monitoring system. China Radio (2023)(1) 52–4, 62. doi:10.3969/j.issn.1672-7797.2023.01.041
33. Fan GW, Chao L, Liu L. Overview of GNSS interference monitoring techniques. In: Proc. Of the 13th national annual conference on remote sensing, telemetry and remote control (2012) p. 617–20.
34. ao WL, Wang WM, Sheen J, Chen P. The UKF-based RNN predictor for GPS narrowband interference suppression. In: 2012 Australian communications theory workshop (AusCTW). New Zealand: IEEE (2012) p. 7–12.
35. Van Dererwe JR, Franco DC, Jdidi D, Feigl T, Rugamer A, Felber W. Low-cost COTS GNSS interference detection and classification platform: initial results//2022 Internation-al Conference on Localization and GNSS (ICL-GNSS). IEEE (2022) p. 1–8.
36. Wu J, Liao YP. An improved algorithm for energy detection andfull blind detection of eigenvalue. Appl Sci Technology (2023) 50(04):66–70. doi:10.11991/yykj.202211008
37. Wang J. Research on Beidou civil signal interference detection technology. Tianjin: Civil Aviation University of China (2019) p. 36–9.
38. Fan GW, Deng JN, Wang ZH, Cao L. Study of GNSS interference detection technology based on energy detection. Radio Eng (2013) 43(3):33–5. 39. doi:10.3969/j.issn.1003-3106.2013.03.010
39. Henttu P, Aromaa S. Consecutive mean excision algorithm. IEEE Seventh Int Symp Spread Spectr Tech Appl (2002) 2:450–4. doi:10.1155/2010/459623
40. Saamisaari H, Henttu P. Impulse detection and rejection methods for radio systems. In: IEEEilitary communications conference, 2. Boston,A, USA (2003) p. 1126–31.
41. Yang C, Xu K, Yang HL. Study on detection for interference signals in satellite communication. Command Control and Simulation (2016) 38(06):125–8. doi:10.3969/j.issn.1673-3819.2016.06.026
42. Vartiainen J, Lehtomaki JJ, Saarnisaari H Double-Threshold based narrowband signal extraction//2005 IEEE 61st Vehicular Technology Conference, 2. Stockholm, Sweden: IEEE (2005) p. 1288–92.
43. Liu W. Satellite navigation receiver interference detection technology research. Anhui: Hefei University of Technology (2014).
44. Borio D, Camoriano L, Savasta S, Presti L. Time-Frequency excision for GNSS applications. IEEE Syst J (2008) 2(1):27–37. doi:10.1109/jsyst.2007.914914
45. Wang P, Cetin E, Dempster AG, Wang YQ, Wu SL. GNSS interference detection using statistical analysis in the Time-Frequency domain. IEEE Trans Aerospace Electron Syst (2018) 54(1):416–28. doi:10.1109/taes.2017.2760658
46. Zhou HX, Dai QH, Li YD, Lu XG. Non-existence of time-frequency distributions without cross-term interference and with WVD clustering. Sci China (Series E) (2001) (4) 348–54. doi:10.3969/j.issn.1674-7259.2001.04.008
47. Sun KW, Jin T, Yang D. A new reassigned spectrogram method in interference detection for GNSS Receivers. Sensors (2015) 15(9):22167–91. doi:10.3390/s150922167
48. Sun KW, Zhang J. A GNSS interference detection method based on fractional order Time-Frequency analysis. In: Proc. Of the 10th China satellite navigation academic conference (2019) p. 1–5.
49. Xu HF, Liu F, Zhang X. Detection and parameter estimation of strong and weak LFM signals in the Fractional Fourier domain. Joural Signal Process. (2011) 27(7):1063–8. doi:10.3969/j.issn.1003-0530.2011.07.016
50. Chen YY. Satellite navigation interference detection technology based on transform domain analysis. Anhui: Hefei University of Technology (2022).
51. Zhao HZ, Sun KW. GNSS receiver interference detection based on rearranged wavelet transform. Technology Innovation Appl (2017) 11:13–5.
52. Sun KW, Zhang L. A novel interference detection based on wigner-hough transform for GNSS receivers//proc. Of the 10th China satellite navigation academic conference (2019) p. 1–7.
53. Zeng YH, Liang YC. Spectrum-sensing algorithms for cognitive radio based on statistical covariances. IEEE Trans Vehicular Technology (2009) 58(4):1804–15. doi:10.1109/TVT.2008.2005267
54. Zeng YH, Liang YC. Eigenvalue-based spectrum sensing algorithms for cognitive radio. IEEE Trans Commun (2009) 57(6):1784–93. doi:10.1109/TCOMM.2009.06.070402
55. Liu HH, Deng XH, Chen W. Blind sensing algorithm based on eigenvalue in multiple antennas cognitive radio. Appl Res Comput (2015) 32(01):191–3. doi:10.3969/j.issn.1001-3695.2015.01.043
56. Lei KJ, Yang X, Xiang CQ, Wang XM, Tian K, Tan ZW. A total blindulti-antenna spectrum sensing algorithm based on difference of extreme eigenvalues. J Hunan Univ (Natural Sciences) (2023) 50(12):76–85. doi:10.16339/j.cnki.hdxbzkb.2023300
57. Li YX, Lei J, Zhong SY, Huang CM, Huang C. Covariance blind detectionethod based on eigenvector in cognitive radio network. Telecommunications Sci (2015) 31(11):104–8.
58. Jiao CH, Wang KR, Feng H. Cooperative blind sensing based on autocorrelation matrix in cognitive radios. J Circuits Syst (2011) 16(01):12–9. doi:10.3969/j.issn.1007-0249.2011.01.003
59. Liu HH. Multi-user cooperative spectrum sensing in cognitive radio. Hubei: Wuhan University of Technology (2014).
60. Li PJ. Research of cooperative spectrum sensing technology based on adaptive double thresholds in cognitive radio network. Chongqing: Chongqing University of Posts and Telecommunications (2013).
61. Wu J. Research on interference detection and recognition technology in BDS. Heilongjiang. Harbin Engineering University (2023).
62. Qu YT. Research on intelligence spectrum sensing algorithm based on signal energy distribution. Jiangsu: Nanjing University of Posts and Telecommunications (2021).
63. Yao D, Liu HJ, Liu J, Xie ZC. Support vector machine based spectrum sensing algorithm in cognitive radio. Electron Des Eng (2018) 26(21):1–5. doi:10.14022/j.cnki.dzsjgc.2018.21.001
64. Shi HD, Jiang B, Bao JR, Liu C. Cooperative spectrum sensing with Covarianceatrix decomposition and CNN. J Hangzhou Dianzi Univ (Natural Sciences) (2024) 44(01):1–5. doi:10.13954/j.cnki.hdu.2024.01.001
65. Lu HC, Zhao ZJ, Shang JN, Dai SG. Cooperative spectrum sensing algorithm using convolutional neural networks and covariance. J Signal Process (2019) 35(10):1700–7. doi:10.16798/j.issn.1003-0530.2019.10.010
66. Lorraine KJS, Ramarakula M. A comprehensive survey on GNSS interferences and the application of neural networks for anti-interference. IETE J Res (2023) 69(7):4286–305. doi:10.1080/03772063.2021.1953407
67. Li J, Huang YB, Nie J, Wang FX. Parameter selection analysis of matched spectrum interference. In: 2015 4th international conference on computer science and network technology (ICCSNT). Harbin, China: IEEE (2015) p. 1340–4.
68. Qin W, Dovis F. Situational awareness of chirp interference threats to GNSS based on supervised machine learning. IEEE Trans Aerospace Electron Syst (2022) 58(3):1707–20. doi:10.1109/TAES.2021.3135014
69. Ma PC, Tang XM, Lou SQ, Liu KX, Ou G. Code tracking performance analysis of GNSS receivers with blanking model under periodic pulse interference. Radio Eng (2021) 30(1):184–95. doi:10.13164/re.2021.0184
70. Borio D. GNSS Acquisition in the presence of continuous wave interference. IEEE Trans Aerospace Electron Syst (2010) 46(1):47–60. doi:10.1109/taes.2010.5417147
71. Zeng CZ, Jia X, Zhu WG. Odulation classification of communication signals. Commun Technology (2015) 48(3):252–7. doi:10.3969/j.issn.1002-0802.2015.03.002
72. ahadevkar SV, Khemani B, Patil S, Kotecha K, Vora D, Abraham A. A review on machine learning styles in computer vision-techniques and future directions. IEEE Access (2022) 10:107293–329. doi:10.1109/ACCESS.2022.3209825
73. Zhang XY, Liu CL, Suen CY. Towards robust pattern recognition: a review. Proc IEEE (2020) 108(6):894–922. doi:10.1109/jproc.2020.2989782
74. Nandi AK, Azzouz EE. Algorithms for automatic modulation recognition of communication signals. IEEE Trans Commun (1998) 46(4):431–6. doi:10.1109/26.664294
75. Nandi AK, Azzouz EE. Odulation recognition using artificial neural networks. Signal Process. (1997) 56(2):165–75. doi:10.1016/S0165-1684(96)00165-X
76. Azzouz EE, Nandi AK. Automatic modulation recognition-I. J Franklin Inst (1997) 334B(2):241–73. doi:10.1016/s0016-0032(96)00069-5
77. Safavian SR, Landgrebe D. A survey of decision tree classifier methodology. IEEE Trans Systems,an, Cybernetics (1991) 21(3):660–74. doi:10.1109/21.97458
78. Cortes C, Vapnik V. Support-vector networks. achine Learn (1995) 20(3):273–97. doi:10.1007/bf00994018
79. Lei L. GNSS satellite navigation system interference monitoring technology research. Lanzhou: Lanzhou Jiaotong University (2017) p. 30–5.
80. Li J, Wang FX, Li BY, Li CH. An automatic modulation type interference recognition method. In: Proc. Of the 5th China satellite navigation academic conference (2014) p. 5.
81. Ye R. Research on satellite navigation interference feature recognition technology. In: Sichuan. University of Electronic Science and Technology of China (2019) p. 49–59.
82. Lu DS, Huang L, Long H. A civil aviation GPS interference recognition method based on deep learning. Commun Technology (2022) 55(3):330–8.
83. Hinton GE, Salakhutdnov RR. Reducing the dimensionality of data with neural networks. Science (New York, N.Y.) (2006) 313(5786):504–7. doi:10.1126/science.1127647
84. Lecun Y, Bengio Y, Hinton G. Deep learning. Nature (2015) 521(7553):436–44. doi:10.1038/nature14539
85. Li XJ, Fu D, ou WH, Liu WX, Wang FX. An identification method of navigation signal interference type based on SqueezeNet model. In: 2020 IEEE 9th joint international information technology and artificial intelligence conference (ITAIC). Chongqing, China: IEEE (2020) p. 875–80.
86. ehr IE, Dovis F. Detection and classification of GNSS jammers using convolutional neural networks. In: 2022 international conference on localizatio and GNSS (ICL-GNSS). Tampere. Finland: IEEE (2022).
87. Chen X, He D, Yan XY, Yu WX, Truong TK. GNSS interference type recognition with fingerprint spectrum DNN method. IEEE Trans Aerospace Electron Syst (2022) 58(5):4745–60. doi:10.1109/taes.2022.3167985
88. Yu J, Alhassoun M, Buehrer RM. Interference classification using deep neural networks. In: 2020 IEEE 92nd vehicular technology conference (VTC2020-Fall). IEEE (2020) p. 1–6.
89. Wang P, Cheng YF, Dong BH, Xu H. Convolutional neural network-based interference recognition. In: 2020 IEEE 20th international conference on communication technology (ICCT). IEEE (2020) p. 1296–300.
90. Ge HF. Detection and identification of SatelliteInterference signals. Zhejiang: Hangzhou Dianzi University (2022) p. 35–41.
91. Zhang T. Research on jamming detection and recognition techniques of satellite navigation system. Shandong: Shandong University (2023) p. 48–53.
92. Zhang QC, Ji HB, Li L, Zhu ZG. A graph-based ABS-assisted TBS sleep scheme. IEEE Wireless Commun Lett (2024) 13(9):1. doi:10.1109/lwc.2024.3481467
93. Van Dererwe JR, Franco DC, Feigl T, Rugamer A. A deep neural network approach for classification of GNSS interference and jammer. IEEE Trans Aerospace Electron Syst (2024) 60(3):1–18. doi:10.1109/taes.2024.3462662
94. Wang PY, Cheng YF, Shang GY, Wang J, Li S. Time-frequency component-aware convolutional neural network for wireless interference classification. IEEE Wireless Commun Lett (2022) 11(12):2487–91. doi:10.1109/lwc.2022.3204756
95. Jiang M, Ye ZQ, Xiao Y, Gou XG. Federated transfer learning aided interference classification in GNSS signals. 2024 Ieee/cic Int Conf Commun China (Iccc) IEEE (2024) 1988–93. doi:10.1109/ICCC62479.2024.10681801
96. Xu ZJ, Han JY. Overview of radio direction finding system. China Radioanagement (2002) (3) 29–35. doi:10.3969/j.issn.1672-7797.2002.03.013
97. Zhou YQ, Chen Z, Huangfu K, Sun ZK. Algorithm of solving multi-baseline interfer-ometer phase difference ambiguity in noisy circumstance. J Electronics and Inf Technology (2005) 27(2):259–61.
98. Li P. Research on direction finding system of two-channel correlation interferometer. Xian: Xi’an University of Electronic Science and Technology (2009).
100. Schmidt R. Ultiple emitter location and signal parameter estimation. IEEE Trans Antennas Propagation (1986) 34(3):276–80. doi:10.1109/TAP.1986.1143830
101. Han QQ. The Technology Research of multi-signal recognition and suppress inter-ference direction finding. Heilongjiang. Harbin Engineering University (2013) p. 16–7.
102. Roy R, Paulraj A, Kailath T. ESPRIT-A subspace rotation approach to estimation of parameters of cisoids in noise. IEEE Trans.on Acoustics, Speech, Signal Process. (1986) 34(5):1340–2. doi:10.1109/tassp.1986.1164935
103. Song HT, Zheng XD, Fan GW. Design of GNSS interference direction finding antenna. Digital Commun World (2017) (8) 5–7, 18. doi:10.3969/J.ISSN.1672-7274.2017.08.002
104. Zhu L. Research and implementation of in-terference recognition and direction finding technology in BeiDou navigation satellite system. Beijing: Beijing Jiaotong University (2019).
105. Li XX. Research and implementation of anti-jamming antenna direction finding technology for BeiDou navigation. Beijing: Beijing Jiaotong University (2019).
106. Fan GW, Yu BG, Deng ZX, Chen PL. Research on adaptive array elements calibration algorithm in GNSS interference direction detection. Computer Simulation (2012) 29(11):141–4. doi:10.3969/j.issn.1006-9348.2012.11.033
107. Jiang YL, Zhang W, Chen T, Zhang YX. A phase calibrationethod of passive direction-finding channel based on convolutional neural network. Res Exploration Lab (2023) 42(07):54–7. doi:10.19927/j.cnki.syyt.2023.07.011
108. Zou YF, Yang JP, Wang HC, Liu P. GNSS complex electromagnetic environment integrated monitoring direction finding and analysis assessment technology. Navigation Positioning and Timing (2001) 8(5):88–95. doi:10.19306/j.cnki.2095-8110.2021.05.012
109. Yin Z, Zhen WM, Jin RM, Lin ZY, Wang RZ, Che L. ulti-interference source direction finding and positioning technology for satellite navigation and its application. GNSS World of China (2023) 48(1):111–6. doi:10.12265/j.gnss.2023006
110. Yao ZC, Xu JN, Yang J, Wang HY. An improved interferometer-MUSIC joint direction finding algorithm. J Ordnance Equipment Eng (2023) 44(11):240–7. doi:10.11809/bqzbgcxb2023.11.030
111. Jin RH. Wireless direction finding technology based on TMA//national conference of antenna - NCANT,2023.
112. He C. Theory and application research for timeodulated array. Shanghai: Shanghai Jiao Tong University (2015) p. 50–2.
113. Chen JF. Research on timeodulated array: theory and its application in direction finding. Shanghai: Shanghai Jiao Tong University (2018) p. 84–93.
114. Du HM, Fu CN. A single channel DOA estimationethod based on timeodulated array. J Detect and Control (2017) 39(1):111–5.
115. Wang MQ, He C, Xiang D, Cao AJ, Yang Y, You YH. A full-angle direction finding method with a single channel based on time modulated multi-beam array. Chin J Radio Sci (2024) 39(2):296–304. doi:10.12265/j.cjors.2023079
116. Liu H, Chen JF, Yang L, Ni G, He C, Jin RH. ulti-harmonic direction-finding method in time-modulated array based on frequency spectrum property analysis. Chin J Radio Sci (2023) 38(1):123–9. doi:10.12265/j.cjors.2021275
117. Lin YL, Wang WJ, Wu JW, Cheng Q. High-precision direction finding based on timeodulation array with single radio frequency channel and composite baselines. J Electronics and Inf Technology (2024) 46:5. doi:10.11999/JEIT231137
118. Bian DP, Zhao XN, Liu ZJ, Chen JF, Jin RH. Single-channel amplitude-comparison wideband direction finding method with nonuniform time modulation. Chin J Ship Res (2024) 19(4):282–9. doi:10.19693/j.issn.1673-3185.03367
119. Jiang Y, Li HH, Shuai YM. Broadband DOA estimation based on timeodulated array. Ship Electron Eng (2019) 39(7):60–2. 91. doi:10.3969/j.issn.1672-9730.2019.07.015
Keywords: global navigation satellite system, interference monitoring system, interference detection, interference identification, interference direction finding
Citation: He Y, Li B, Chen J, Wang Z, Xiao W and Lu Z (2024) Overview of the development of satellite navigation blanket interference monitoring. Front. Phys. 12:1487384. doi: 10.3389/fphy.2024.1487384
Received: 28 August 2024; Accepted: 25 November 2024;
Published: 12 December 2024.
Edited by:
David Ruffolo, Mahidol University, ThailandCopyright © 2024 He, Li, Chen, Wang, Xiao and Lu. This is an open-access article distributed under the terms of the Creative Commons Attribution License (CC BY). The use, distribution or reproduction in other forums is permitted, provided the original author(s) and the copyright owner(s) are credited and that the original publication in this journal is cited, in accordance with accepted academic practice. No use, distribution or reproduction is permitted which does not comply with these terms.
*Correspondence: Zukun Lu, bHV6dWt1bkBudWR0LmVkdS5jbg==