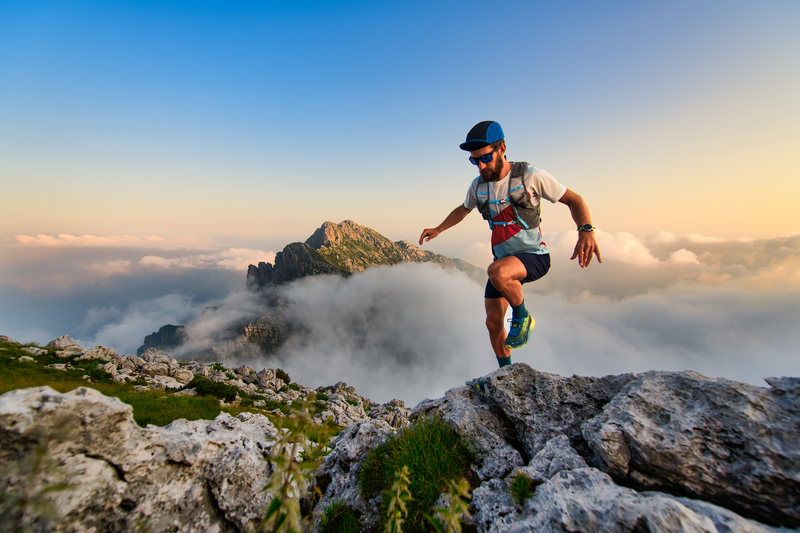
95% of researchers rate our articles as excellent or good
Learn more about the work of our research integrity team to safeguard the quality of each article we publish.
Find out more
ORIGINAL RESEARCH article
Front. Oncol. , 23 December 2024
Sec. Neuro-Oncology and Neurosurgical Oncology
Volume 14 - 2024 | https://doi.org/10.3389/fonc.2024.1449982
This article is part of the Research Topic Application of Emerging Technologies in the Diagnosis and Treatment of Patients with Brain Tumors: New Frontiers in Imaging for Neuro-oncology View all 9 articles
Introduction: Precision medicine refers to managing brain tumors according to each patient’s unique characteristics when it was realized that patients with the same type of tumor differ greatly in terms of survival, responsiveness to treatment, and toxicity of medication. Precision diagnostics can now be advanced through the establishment of imaging biomarkers, which necessitates quantitative image acquisition and processing. The VASARI (Visually AcceSAble Rembrandt Images) manual annotation methodology is an ideal and suitable way to determine the accurate association between genotype and imaging phenotype. Our work proposes an updated version of the VASARI score that is derived by changing the evaluation ranges of its components in an effort to increase the diagnostic accuracy of the VASARI manual annotation system and to find neuroimaging biomarkers in neuro-oncology with increasing reliability.
Materials and methods: We gathered the histological grade and molecular status of 126 patients with glioma (Men/Women = 75/51; mean age: 55.30) by a retrospective analysis. Two residents and three neuroradiologists blindedly examined each patient using all 25 VASARI characteristics, after having appropriately modified the reference ranges in order to implement an innovative VASARI lexicon (VASARI 2.0). It was determined how well the observers agreed. A box plot and a bar plot were used in a statistical analysis to assess the distribution of the observations. After that, we ran a Wald test and univariate and multivariate logistic regressions. To find cutoff values that are predictive of a diagnosis, we also computed the odds ratios, confidence intervals, and evaluation matrices using receiver operating characteristic curves for each variable. Finally, we performed a Pearson correlation test to evaluate whether the variable grades and IDH were correlated.
Results: An excellent Intraclass Correlation Coefficient (ICC) estimate was obtained. In this study, five features were part of the predictive model for determining glioma grade: F4, enhancement quality [area under the curve (AUC): 0.87]; F5, tumor-enhancing proportion (AUC: 0.70); F6, tumor–non-enhancing proportion (AUC: 0.89); F7, necrosis proportion (AUC: 0.79); and F17, diffusion characteristics (AUC: 0.75). Furthermore, six features were found to predict IDH mutation status: F4, enhancement quality (AUC: 0.904); F5, tumor-enhancing proportion (AUC: 0.73); F6, tumor–non-enhancing proportion (AUC: 0.91); F7, necrosis proportion (AUC: 0.84); F14, proportion of edema (AUC: 0.75); and diffusion characteristics F17 (AUC: 0.79). VASARI 2.0 models showed good performances according to the AUC values, which are also compared with traditional VASARI scores.
Discussion and conclusion: Glioma grade and isocitrate dehydrogenase (IDH) status can be predicted using specific magnetic resonance imaging (MRI) features, which have significant prognostic consequences. The accuracy of texture-derived metrics from preoperative MRI gliomas and machine learning analysis for predicting grade, IDH status, and their correlation can be enhanced by the suggested new and updated VASARI manual annotation system. To help with therapy selection and enhance patient care, we intend to create prediction models that incorporate these MRI findings with additional clinical data.
The cerebral glioma, a sizable and heterogeneous family of brain tumors with various features, is the most prevalent primary malignant brain tumor that exhibits variable treatment response and patient prognosis (1). Given the considerable variations in the care of these many glioma subtypes, an accurate diagnosis is essential. Glioblastoma and oligodendroglioma, for instance, respond very differently to treatment. Furthermore, tumors belonging to the same histologic subtype could exhibit distinct behaviors in other patient cohorts. Previously, the phenotypic characteristics of the cells were used to identify the tumor grade, but, today, this seems too simplistic (2), and it is not possible to identify these distinctions between the different glioma subtypes based on the purely histology-driven older classification system. Part of the challenge was that many gliomas can contain mixed cell types, which result in high inter-observer variability of diagnosis among neuropathologists (3).
Thus, a paradigm change in the diagnosis and categorization of gliomas has resulted from new discoveries on their genetic composition.
The updated glioma classification system incorporates molecular markers into tumor subgrouping, which has been shown to better correlate with tumor biology and behavior as well as patient prognosis than the previous purely histology-based classification system (4). The isocitrate dehydrogenase (IDH) gene changes and the co-deletion of chromosomal arms 1 and 19 (1p/19q) are the two main alterations taken into account when differentiating tumors in the World Health Organization (WHO) classification (5). It could be comparable to biomarkers that influence the prognosis and biological behavior of a patient. For instance, it has been demonstrated that IDH gene family mutations offer higher overall survival in high-grade gliomas than their IDH–wild-type counterparts, regardless of the histological grade (6–8). Additionally, the degree of cellular differentiation and the molecular state have an impact on the course of treatment. For instance, low-grade gliomas are often not treated with adjuvant radiotherapy and/or chemotherapy. Clinicians found that patients’ responses to treatment, the severity of side effects, and even prognosis could differ even when they shared the same tumor. This implies that therapeutic care tailored to the needs of particular people or “precision medicine” may be the direction of the future (9–11). The method most frequently used today for identifying glioma mutations is immunohistochemical analysis after biopsy or surgical resection (12). By categorizing radiological gliomas in a non-invasive way with relevant prognostic consequences, clinical therapeutic planning may be recommended (13). Magnetic resonance imaging (MRI) is used as a gold standard for radiological examination of gliomas. Because there are no objective measurements that can be extensively duplicated and validated, determining the tumor grade accurately is far from simple (14).
Whereas advanced MRI techniques (e.g., diffusion Magnetic Resonance Imaging (dMRI), Perfusion Magnetic Resonance Imaging (pMRI), and Magnetic Resonance Spectroscopy (MRS)) are more specific to biophysical, cellular, and microstructural processes, conventional MRI methods (e.g., T1-weighted and T2-weighed sequences) give macrostructural anatomical evidence. Unlike standard MRI techniques, which only yield relative image contrasts, these advanced techniques have the potential to be (semi)quantitative. For the purpose of acquiring imaging biomarkers, sensitivity, specificity, and quantification are crucial (15). While a number of recent investigations have concentrated on applying sophisticated MRI methods (such as perfusion, spectroscopy, and machine learning approaches) for radiogenomic purposes (16–18), standard MRI sequences continue to be the gold standard for the investigation and characterization of brain tumors.
An optimal and adequate method to identify the right correlation between imaging phenotype and genotype, based on the evaluation of specific radiological characteristics, mainly conventional MRI features, and, at the same time, to standardize the assessment of gliomas is represented by the VASARI manual annotation system.
A collection of standardized descriptors called VASARI (Visually AcceSAble Rembrandt Images) MRI characteristics is used to describe brain tumors on contrast-enhanced MRI imaging. These characteristics aid in the diagnosis, grading, and prognostication of gliomas by offering qualitative and quantitative information regarding the visual appearance and properties of the tumor (19). The location, shape, enhancement quality, necrosis proportion, edema proportion, and other geometric parameters of the tumor are all included in the VASARI features (20).
Since its development in 2016, VASARI score has undergone a development from the number of the features, now 25, to the field of application in neuro-oncology.
Certain specific MRI features [enhancement quality (F4), tumor-enhancing proportion (F5), tumor–non-enhancing proportion (F6), and necrosis proportion (F7)] have been shown in our previously published study (21) to be predictive of the grade and IDH status of gliomas, with significant prognostic implications.
Inter-observer agreement and multicenter collaborations are made possible by the reliability and consistency in the interpretation of MRI scans made possible by the standardization of the VASARI features (22, 23). The communication between radiologists, oncologists, and other medical professionals involved in the treatment of patients with glioma is improved when VASARI elements are used in structured reporting systems (24). To increase the precision of glioma grading, prognosis prediction, and treatment response assessment, they have been used in machine learning algorithms and radiomics studies (24, 25). Predictive models to inform therapy choices and patient care can be created by merging VASARI variables with additional imaging features and clinical data (26, 27).
Such a model’s potential resides in its capacity to evaluate tumor features objectively. In actuality, although VASARI started out as a visual assessment scale, a numerical estimate of the features under consideration can be obtained by using “regions of interest” (ROIs) to calculate the areas of the various tumor components. However, we think that such a system is not useful in terms of outpoint prediction and is too sophisticated and challenging for doctors to utilize, especially in light of the outcomes documented in the literature and our earlier study.
In this regard, we propose a new VASARI glioma score, which we refer to as VASARI 2.0. This system evaluates only those tumor features that can be objectively described by ROI (manual segmentation) and that predict the outpoint (IDH status and grade) with area under the curve (AUC) > 0.8. For this purpose, we modify the evaluation intervals/ranges as explained in the following (Table 1), all in order to provide the scientific community with a system suitable with clinical practice.
The Institutional Review Board accepted the study because the surgery was routinely carried out and was not considered experimental. Each patient completed and signed a proper written informed consent. There was no indication of a conflict of interest from the writers. No funding was given to support this study.
Retrospective analysis of patients who underwent MRI for pre-surgical glioma evaluation between 2018 and 2021 has been done on the database at our institution. Additionally, pathology reports were gathered to determine the glioma grade. According to the following criteria, a number of patients were disqualified from the study: (a) poor acquisition quality imaging; (b) no intravenous contrast; (c) medications prior to the MR examination, such as steroid medication that may alter edema and contrast enhancement; and (d) lack of a pathology report. In the end, 126 individuals with glioma were included. The study group included 51 women and 75 men, ages 14 to 84 (further information in Table 2).
MRI at 1.5 T (Magnetom Amyra; Siemens Medical Systems, Erlangen, Germany) was used for the imaging. In addition to T2-weighted images with dark fluid on the axial planes, the MR technique also includes T1-weighted images taken before and after the administration of gadolinium-based contrast media. In addition to this, we also carried out Diffusion Weighted Imaging (DWI) and Susceptibility Weighted Imaging (SWI) on the axial plane, as well as T1-w and T2-w sequences on additional planes. These were the precise imaging parameters: (1) axial T1-weighted MR: repetition time of 250 ms, echo time of 2.46 ms, slice thickness of 5 mm, matrix dimensions of 320 × 256, and field of view of 220 × 220 mm2; (2) axial T2-weighted MR: repetition time of 6000 ms, echo time of 93 ms, slice thickness of 5 mm, matrix dimensions of 320 × 288, and field of view of 198 × 220 mm; and (3) axial T2WI dark-fluid MR: repetition time of 8000 ms, echo time of 97 ms, slice thickness of 5 mm, matrix dimensions of 320 × 224, and field of view of 181 × 220 mm.
The VASARI lexicon can be easily understood by following the specific guide downloadable from the public website of The Cancer Imaging Archive, in the specific section “Supporting Documentation and Metadata” (https://wiki.cancerimagingarchive.net/display/Public/VASARI+Research+Project).
We considered that the entire lesion was made up of necrotic tissue, edema, enhancing area, and non-enhancing area in accordance with the VASARI approach. Furthermore, we extracted the score system and morphological features. Therefore, an enhancing area was defined as any region of the tumor that shows a discernible increase in signals on the post-contrast T1-weighted pictures in comparison to those in the pre-contrast. Any region displaying T2-weighted hyperintensity (less than the intensity of the Cerebral Spinal Fluid (CSF) fluid) and corresponding T1-weighted hypointensity, as well as a mass effect and architectural distortion, such as blurring of the gray-white interface, was deemed to be a non-enhancing area. An irregular border, a high signal on T2-weighted and proton density imaging, and either no enhancement at all or a significantly decreased enhancement are characteristics of a necrotic section of the tumor. By calculating the ratio of the total lesion area to the necrosis area (internal to it), a quantitative evaluation of the necrosis was produced. On the T2-, T1-, and SWI T2*–weighted sequences, a bleeding was detected and assessed in connection with the existence of hemoglobin breakdown products. Based on an apparent diffusion coefficient (ADC) map, the diffusion characteristics are classified as mostly facilitated or restricted in the enhancing or non-contrast–enhanced tumor (nCET) region of the tumor. They are described as mixed when there is a roughly equal amount of both limited and assisted diffusion.
Three neuroradiologists, two residents and one senior, independently evaluated the imaging characteristics.
The aims of the statistical analysis were as follows: 1) analyze the statistically significance of each variable with respect to the prediction of the variable levels; 2) analyze the statistically significance of each variable with respect to the prediction of the variable IDH; and 3) analyze the relationship between IDH and GRADE.
To consider only relevant columns in the dataset, a sub-dataset was created, only with the following columns: GRADE, F4, F5, F6, F7, F14, F15, and F24, and another column was added to the dataset named “levels.” This variable is binary, with level = 0 denoting a grade of 1 or 2 (low grade) and level = 1 denoting a grade of 3 or 4 (high grade). The variable “IDH mutate” has been turned into a dummy binary variable too (IDH 0 = non-mutate = neg; IDH 1 = mutate = pos).
To see whether the variable GRADE and IDH are correlated, we built a contingency table where, on one side, there are the levels of GRADE and, on the other, the IDH, negative or positive. Then, we also did the Pearson correlation test and built a correlation plot.
As is often the case in real datasets, the VASARI dataset that we analyzed is highly imbalanced (80% vs. 20%) and is of high grade, so we divided the dataset into train (70%) and test (30%), and, then, we balanced the training data in such a way that we obtained 2,000 observation and perfectly balanced classes (p = 0.5). Then, we proceeded to the analysis of the statistically significant variables in the prediction of levels. As the first step in this part of the analysis, we conducted a multiple logistic regression using the dichotomic variable levels as response variable and each variable as covariate on the balanced training set. We also conducted a Wald test on the categorical variables to confirm statistical significance.
We computed the odds ratios (OR) and confidence intervals for each variable and evaluated the matrices with ROC curves.
After the balancing of the classing, we finally conducted a multiple logistic regression using all the variables in the dataset to have an idea of which variable is statistically significant and to see how does our classifier performs having the whole set of information in the prediction of IDH. We then applied this model to the test set to evaluate the predictive performance in the analysis of the relationship between IDH and GRADE.
We compared the results with the same value obtained in our previous studies using the gold standard for VASARI score and traditional statistics method; in particular, we focused on the AUC value to compare diagnostic accuracy.
The analysis has been done on the software R, using the package ROSE for the balancing purpose.
Applying more or less complex machine learning methods to this type of data is very risky because the more unbalanced the classes, the greater the risk of having results biased by lack of observation in one class.
More specifically, the classification problem’s confusion matrix indicates how well our model classifies the target classes, and it is from this confusion matrix that we derive the model’s accuracy, which is determined by dividing the total number of predictions made by the model correctly by the total number of predictions. Thus, in cases where a class has few observations, it may be categorized as the most popular class, potentially yielding a high accuracy score. For example, one of the most often used parametric techniques for binary classification is logistic regression, which is heavily biased in cases when the classes are not balanced because it underestimates the conditional probabilities of the rare class. To solve these problems, many methods have been proposed in the literature, such as oversampling, undersampling, SMOTE (Synthetic Minority Oversampling Technique), and ROSE (Random Over-Sampling Examples). In this paper, we chose to use the most recent ROSE technique. It is a bootstrap-based method that helps with binary classification when there are uncommon classes present. By creating synthetic examples from a conditional density estimate of the two classes, it can handle both continuous and categorical data. We selected this approach because of the strong theoretical underpinnings of ROSE. It also draws synthetic examples from an estimate of the conditional density underlying the data, thus providing confidence that the distribution of the data into the classes has not changed because the balancement has been performed.
In previous studies, it has been demonstrated that some of that specific MRI features [enhancement quality (F4), tumor-enhancing proportion (F5), tumor–non-enhancing proportion (F6), and necrosis proportion (F7)] can be used to predict the grade and IDH status of gliomas, with important prognostic implications. The standardization and improvement of these data can be used for programming machine learning software (28).
We obtained a clear relation between a negative IDH and high GRADE. In fact, 75.2% of observations have a negative IDH and a high grade (= 3 or 4) with a p-value of 2.2e−16 < 0.05, so the coefficient is statistically significant. We obtained a correlation coefficient of −0.661, meaning that these two variables are significantly negatively correlated. The resulting confidence interval is [−0.750, −0.550], respectively, at 2.5% and 97.5%. These results are shown in Figure 1.
Figure 1. In our analysis, we obtained a clear relationship between negative IDH and high GRADE. In fact, 75.2% of the observations have negative IDH and high grade (= 3 or 4) with a p-value of 2.2e−16 < 0.05, so the coefficient is statistically significant. We obtained a correlation coefficient of −0.661, which means that these two variables are significantly negatively correlated. The resulting confidence interval is [−0.750, −0.550] at 2.5% and 97.5%, respectively. Statistical analysis by contingency table, which is then confirmed by Pearson’s test, obtained a statistically significant correlation between IDH mutation and glioma grade: In 75% of the observations, we found IDH WT and high grade.
We applied this model to the test set to evaluate the predictive performances, and we obtain accuracy = 0.811, sensitivity = 0.781, and specificity = 1, with AUC = 0.906. We proceeded with the analysis of the statistical significance of each variable in the prediction of levels. We obtained that variable F4 is significant with a p-value = 2.7e−10 < 0.05 with no significant differences between levels 1 and 3. For every one unit increase in F4 = 2, the odds of being in the level = 1 (high grade) increases by a factor of 4.699.
We calculated the predicted probabilities to be in the high-level grades (vs. low level) at each level of F4: F4 = 3 has 100% probability to be in the high level (grades 3 and 4), whereas the probability for the other two levels is much lower (5% and 20%). This model has accuracy = 0.811, sensitivity = 0.781, and specificity = 1.000, with AUC = 0.869, which is superior to that of the first study (0.73).
Variable F5 is statistically significant with a p-value < 2e−16 < 0.05 with significant differences between levels 2 and 6 of the F5 variable and cutoff level F5 = 4.
For every one unit increase in F5 = 3, the odds of being in the level = 1 (high grade) increases by a factor of 0.49.
F5 = 5 and F6 = 6 have a probability to be in the high level (grades 3 and 4) of 73% and 63%, whereas the probability for the other three levels is much lower (19%, 73%, and 63%).
This model has accuracy = 0.672, sensitivity = 0.908, and specificity = 0.438, with AUC = 0.712.
Variable F6 is statistically significant with a p-value = 0.01409 < 0.05.
For every one unit increase in F6 = 2, the odds of being in the level = 1 (high grade) increases by a factor of 1. We also obtained that there is a significant difference between the F6 = 3 and F6 = 4 (p-value = 0.0049 < 0.05). We calculated the predicted probabilities to be in the high-level grades (vs. low level) at each level of F6: F6 = 1, F6 = 2, and F6 = 3 have a probability to be in the high level (grades 3 and 4) of 100%, whereas the probability for the other levels is much lower (24.1%, 15.9%, and 0%, respectively). We can also clearly identify a cutoff level for this variable. This model has accuracy = 0.757, sensitivity = 0.719, and specificity = 1.000, with AUC = 0.8969. Also, this result is superior to that of our first study.
Variable F7 is statistically significant with a p-value = 2e−16 > 0.05, but none of the levels of F7 is statistically significant. For every one unit increase in F7 = 2, the odds of being in the level = 1 (high grade) increases by a factor of 3.35; whereas for every one unit increase in F7 = 4, the odds of being in the level = 1 (high grade) increases by a factor of 3.22e+09.
Moreover, we calculated the predicted probabilities to be in the high-level grades (vs. low level) at each level of F7: F7 = 4, F7 = 5, and F7 = 6 have the probability to be in the high level (grades 3 and 4) of 100%; whereas when F7 = 1 and F7 = 2, the probability to be in the high level is 8.9% and 24.6%, respectively. This model has accuracy = 0.837, sensitivity = 0.906, and specificity 0.400, with AUC = 0.8 (vs. an AUC of 0.738).
Variable F17 is statistically significant with a p-value = 3.634e−05 < 0.05. Moreover, there is a significant difference between levels F17 = 1 and F17 = 2 with a p-value of 0.017 < 0.05 and also between F17 = 1 and F17 = 3 with a p-value of 0.0017 < 0.05. We can then identify a cufoff in this case.
For every one unit increase in F17 = 2, the odds of being in the level = 1 (high grade) increases by a factor of 7.71; whereas for every one unit increase in F17 = 3, the odds of being in the level = 1 (high grade) increases by a factor of 18.48.
We can calculate the predicted probabilities to be in the high-level grades (vs. low level) at each level of F17: F17 = 3 has the probability to be in the high level (grades 3 and 4) of 74%; whereas when F17 = 1 and F17 = 2, the probability to be in the high level is 13.4% and 24.3%, respectively.
This model has accuracy = 0.840, sensitivity = 0.921, and specificity 0.500, with AUC = 0.8.
Variables F14, F15, F18, F19, and F20 were not statistically significant with a p-value < 0.05. These results are shown in Figure 2.
Figure 2. Through logistic regression using the dichotomous variable (high or low grade) as the response variable and F4 as the covariate, we obtained that variable F4 is significant with a p-value = 0.000157 < 0.05. We also compared the performance of VASARI 2.0 with that of traditional VASARI in predicting grade.
The pre-processing part is identical to the one described in part 2: We divided the dataset into train (70%) and test (30%), and, then, we balanced the training data in such a way that we obtained 2,000 observation and perfectly balanced classes (p = 0.5). Then, we conducted a multiple logistic regression and obtained that all the variables are statistically significant. We then applied this model to the test set to evaluate the predictive performances, and we obtained accuracy = 0.88, sensitivity = 0.6, and specificity = 0.95, with AUC = 0.93. We proceed with the analysis of the significance of each variable in the prediction of IDH. We obtained that variable F4 is significant with a p-value = 1.9e−05 < 0.05 with a significant difference between F4 = 2 and F4 = 3 (p-value 8.9e−05 < 0.05). The results of the OR have a key role in interpreting the role of the variable. In particular, the OR column means that, for every one unit increase in F4 = 2, the odds of having a positive IDH increases by a factor of 0.14; whereas for every one unit increase in F4 = 3, the odds of having a positive IDH increases by a factor of 0.0. Moreover, we can calculate the predicted probabilities of having a positive IDH (vs. negative) at each level of F4: F4 = 3 has 1.2% probability of having a positive IDH, whereas the probability for the other two levels is higher (76.9% and 45.2%). This model has accuracy = 0.90, sensitivity = 0.9, and specificity 0.90, with AUC = 0.904, which is superior to that of our first study (0.73).
Variable F5 is statistically significant with a p-value = 0.04386 < 0.05 with a significant difference also between F5 = 3 and F5 = 4 with a p-value = 3.6e−11 < 0.05 and between F5 = 3 and F5 = 5 with a p-value = 0.0077 < 0.05. Hence, we can conclude that the cutoff level for variable F5 is F5 = 3. The OR column showed that, for every one unit increase in F5 = 2, the odds of having a positive IDH increases by a factor of 4.978539e−08; whereas for every one unit increase in F5 = 7, the odds of having a positive IDH increases by a factor of 5.524675e−16. These values are so low because F5 = 1 is taken as baseline, where only positive values are observed.
Moreover, we calculated the predicted probabilities of having a positive IDH (vs. negative) at each level of F5: F5 = 1, F5 = 2, and F5 = 3 have a high probability to have a positive IDH of 100%, 67%, and 81%, respectively, whereas the probability for the other three levels is much lower (53.3%, 14%, and ~0%). This modes has accuracy = 0.808, sensitivity = 0.48, and specificity 0.890, with AUC = 0.73.
Variable F6 is statistically significant with a p-value = 5.162e−0 5< 0.05 with a significant difference between levels F3 and F4 with a p-value of 2e−16 < 0.05 and between F5 and F6 with a p-value of 1.1e−14 < 0.05. With these results, we may identify two different cutoffs.
The OR column showed that, for every one unit increase in F6 = 2, the odds of having a positive IDH increases by a factor of 1; whereas for every one unit increase in F6 = 4, the odds of having a positive IDH increases by a of 6.704338e+08.
Moreover, we calculated the predicted probabilities to be in the high-level grades (vs. low level) at each level of F6: F6 = 4, F6 = 5, and F6 = 6 have the highest probability to have a positive IDH: 68.1%, 75.5%, and 97.4%, respectively, whereas the probability for the other levels is 0%. This model has accuracy = 0.86, sensitivity = 0.90, and specificity = 0.85, with AUC = 0.9125 (vs. AUC = 0.7648).
We obtained that variable F7 is statistically significant with a p-value = 2.31e−06 < 0.05 and a significant difference between F7 = 3 and F4 = 4 (p-value = 0.00085 < 0.05). Another significant difference is between F7 = 2 and F7 = 4. We can then conclude that we have a cutoff for values higher than F7 = 3. The OR column showed that, for every one unit increase in F7 = 2, the odds having a positive IDH increases by a factor of 2.083333e−01. Moreover, we can calculate the predicted probabilities to have a positive IDH at each level of F7: F7 = 1, F7 = 2, and F7 = 3 have a probability of having a positive IDH of 61.5%, 25%, and 21.2%, respectively, whereas F7 = 4, F7 = 5, and F7 = 6 have a probability of having a positive IDH mush lower: 0%. This model has accuracy = 0.740, sensitivity = 1.000, and specificity = 0.675, with AUC = 0.835 (vs. AUC = 0.789).
Variable F14 is barely statistically significant with a p-value = 0.0648 > 0.05 with no significant difference between the levels in F14. The OR column showed that, for every one unit increase in F14 = 2, the odds having a positive IDH increases by a factor of 0.25; whereas for every one unit increase in F14 = 3, the odds of being in the odds having a positive IDH increases by a factor of 0.625. Moreover, we can calculate the predicted probabilities to have a positive IDH at each level of F14: F14 = 3 has the highest probability of having a positive IDH with the 38.5%; whereas F14 = 2, F14 = 4, and F14 = 5 have a probability of having a positive IDH much lower: 20%, 8.8%, and 12.5%, respectively. This model has accuracy = 0.780, sensitivity = 0.600, and specificity 0.825, with AUC = 0.75.
Variable F17 is statistically significant with a p-value = 9.372e−06 < 0.05 and a significant difference between the levels in F17 = 1 and F17 = 2 with a p-value of 0.00049 < 0.05. The OR column showed that, for every one unit increase in F17 = 2, the odds having a positive IDH increases by a factor of 0.11; whereas for every one unit increase in F17 = 3, the odds of being in the odds having a positive IDH increases by a factor of 0.05. Moreover, we can calculate the predicted probabilities to have a positive IDH at each level of F17: F17 = 3 has the lowest probability of having a positive IDH with the 8.7%; whereas F17 = 1 has a higher probability of having positive IDH: 65%. This modes has accuracy = 0.860, sensitivity = 0.400, and specificity 0.975, with AUC = 0.7925.
Other features, qualitative or non-statistically significant, were not taken into account. These results are shown in Figure 3.
Figure 3. Through univariate logistic regression to evaluate the influence of variables in predicting/IDH levels, we obtained that variable F6 is statistically significant with a p-value = 0.0032 < 0.05. We also performed a Wald test on variable F6, which concluded that this variable is statistically significant (p-value = 0.01409 < 0.05). We also compared the performance of VASARI 2.0 with that of traditional VASARI in predicting IDH status.
In our study, we confirm that there is a positive statistical evidence between some VASARI features and IDH and glioma grade (Figure 1). The most significant variables in the prediction of IDH are F4 (AUC: 0.904), F5 (AUC: 0.73), F6 (AUC: 0.91), F7 (AUC: 0.84), F14 (AUC: 0.75), and F17 (AUC: 0.79).
The most significant variables in the prediction of levels are F4 (AUC: 0.87), F5 (AUC: 0.7), F6 (AUC: 0.89), F7 (AUC: 0.79), and F17 (AUC: 0.75).
The statistical significance for all the features is increased using VASARI 2.0 compared to result obtained with traditional VASARI ranges, demonstrating how the new proposed VASARI lexicon promotes an increase in the sensitivity, specificity, and AUC of these features to increase the statistical significance with AUC > 0.8 and to make this system more suitable for clinical practice. We can distinguish low-grade gliomas from high-grade gliomas using this model. Numerous studies have assessed the application of the VASARI Lexicon in the categorization of cerebral gliomas (29–34). Due to a much more extensive destruction of the blood–brain barrier in high-grade gliomas compared to that in low-grade gliomas, our study, for instance, produced similar results to those in the work done by Su et al., in which the enhancement quality and the proportion enhancing were significantly higher in high-grade gliomas (33). High-grade gliomas exhibit much higher cell growth proliferation. In the context of tumor tissue, new, irregular, and aberrant vessels form very rapidly, without an adequate blood–brain barrier, a process known as neoangiogenesis. Newly formed vessels are much more permeable than normal vessels. Therefore, in an MRI study after intra venous of contrast agent, the solid tumor component appears much brighter or “enhanced” in the resulting images, given that the contrast agent can cross the blood–brain barrier very easily, escaping from the blood vessels into the surrounding tumor tissue. Furthermore, areas of necrosis can very often be found in high-grade gliomas, given that they have a very high rate of proliferation and cell growth, which is associated with an inadequate blood supply. These necrotic phenomena, therefore, contribute to the breakdown of the blood–brain barrier, allowing a greater leakage of contrast agents into the tumor tissue and promoting greater further enhancement (31, 32). In high-grade gliomas, there are often infiltrative lesional margins in the surrounding healthy tissue. In MRI, all this appears as an area of pathological alteration of the signal intensity, with hyperintensity in the Fluid Attenuated Inversion Recovery (FLAIR)-weighted sequences, much larger and more extensive than the pathological area visible on the T1-weighted MRI sequences. Our study supports this theory by showing that the infiltrative T1/FLAIR ratio has a high predictive value for glioma grade (OR = 41.99, p = 0.001). The fast and uncontrolled phenomenon of neoangiogenesis is indirectly indicated by the presence of hemorrhagic components and necrosis in the context of high-grade gliomas. The newly created blood vessels are easily injured and do not have an uneven morphology, which results in bleeding. In our study, the presence of necrosis was a strong predictive factor with an OR of 13.57 (p < 0.001) for high-grade glioma, a result that is consistent with previous research findings (30–34).
In our study, we found that edema proportion was a significant factor. These findings, in line with other recent studies, highlight that mass effect is an important predictor of astrocytoma grade. Tumor perilesional cerebral edema correlates with the WHO pathological grading as recently demonstrated (35). Measuring areas of non-enhancing tumor have been highlighted by The Response Assessment in Neuro-Oncology Working Group (36). Astrocytomas represent very heterogeneous neoplasms. The component characterized by contrast enhancement does not always contain anaplastic parts, unlike the component without contrast enhancement, which often contains both anaplastic parts and low-grade parts. The evaluation of the nCET component, therefore, is very important both in the diagnosis and in the follow-up to better assess the therapeutic monitoring of astrocytoma. However, understanding the real extent of a high-grade astrocytoma by evaluating the nCET proportion is very difficult given the extremely heterogeneous nature of the tumor and the extreme difficulty in delineating its peripheral margins. In reality, all this represents a false dichotomy, given that, in infiltrating gliomas, there is an “infiltrative edema,” consisting of tumor cells and edema in the background of the brain. Furthermore, even with the use of sophisticated techniques like T2 mapping, diffusion tensor imaging, and perfusion imaging, it remains difficult to differentiate pure vasogenic edema from infiltrative edema. In this work, using multivariate analysis based on VASARI, we showed that nCET percentage was a predictive factor of grade 4 astrocytoma. The new classification of tumors of the central nervous system published in 2021 highlights the importance of evaluating the molecular status, particularly, and first of all, the IDH mutation. Usually, one of the two IDH genes, IDH1 and IDH2, is affected by the mutation. The mutation most frequently found in gliomas is that affecting the IDH1 gene. Typically, a specific mutation (R123H) occurs that causes a single–amino acid change in the active enzyme site. The mutated IDH enzyme promotes the conversion of alpha ketoglutarate into 2-hydroxyglutarate, an oncological metabolite that induces cancer formation (6). The presence of the IDH mutation is associated with a significantly better prognosis compared to IDH–wild-type gliomas, and its identification is, therefore, very important for the classification of cerebral gliomas and for clinical therapeutic management. In our study, six VASARI features were found to predict IDH mutation status: F4, enhancement quality (AUC: 0.904); F5, tumor-enhancing proportion (AUC: 0.73); F6, tumor–non-enhancing proportion (AUC: 0.91); F7, necrosis proportion (AUC: 0.84); F14, proportion of edema (AUC: 0.75); and F17, diffusion characteristics (AUC: 0.79). Our study confirms the results of our recently published study for VASARI characteristics and demonstrates an increase in their diagnostic accuracy, especially regarding F4 and F6 with an AUC value greater than 85%, in line with the ESR statement on the validation of imaging biomarkers (European Society of Radiology, 2020). Olar et al. identified a significant correlation between proportion of enhancing tumor and IDH mutation. In the study, the researchers studied the role that the IDH mutation may have in the grading and mitotic index in grade II–III diffuse astrocytomas, and their results demonstrated that the IDH mutation determines the effect of mitotic index on patient outcome (37, 38). IDH–wild-type gliomas usually have a higher contrast enhancement than IDH-mutant gliomas (39–43), and IDH wild-type gliomas have undefined margins (44). Weighted FLAIR sequences are very useful for evaluating areas with pathological signal intensities. In the case of IDH–wild-type gliomas, the areas with pathological signal hyperintensity in the weighted FLAIR sequences, which extend beyond the margins of enhancement, usually represent the infiltrative edema component, characterized by the presence of infiltration of tumor cells in the peripheral tissue (31). Using multivariate analysis, we discovered that, in our cohort, the percentage of necrosis accurately predicts the status of IDH mutation. In our investigation, necrosis accounted for less than 25% of the total tumor volume in IDH-mutant cohorts and more than 50% in IDH-wild phenotypes. These results support the conclusions of multiple investigations. IDH-mutants were frequently linked to a cutoff necrosis of less than 33% of the tumor, according to Park et al. (45). Excessive tumor necrosis in IDH–wild-type gliomas is determined by increased hypoxia, which is brought on by intravascular thrombosis and the coagulation pathway activation (45–47). Furthermore, our results highlight two other VASARI features that can be used in the prediction of IDH status, which were not highlighted in our recently published work, namely, F14 (proportion of edema) and F17 (diffusion characteristics). In IDH-mutant gliomas, absent edema or edema with an extension smaller than that of the solid tumor component was found; whereas in IDH–wild-type gliomas, the extension of the edematous alteration was greater than or equal to the volume of the tumor. Similar results were documented in the studies by Lasocki et al. (40) and Patel et al. (48). Lasocki et al. found a cutoff value of 33% to distinguish IDH-mutant gliomas from IDH–wild-type gliomas. Furthermore, the presence of cysts was documented more frequently in IDH-mutant gliomas, in line with other published studies (49). In several other studies, IDH-mutant gliomas had higher average ADC values than IDH–wild-type gliomas, underlining that their edematous component is usually less infiltrative and destructive (50–54). Nowadays, the main attraction of the scientific interest is radiomic and machine learning that has been applied to tumor grading and diagnosis, tumor segmentation, non-invasive genomic biomarker identification, detection of progression, and patient survival prediction. It has been suggested that machine learning models are capable of more accurate prognosis prediction than histopathological categorization. These considerations could be the starting point for subsequent studies. The standardization and improvement of these data can be used for programming machine learning software (55). In the field of brain tumor, interest in machine learning methods is increasing, especially in diagnosis and pre-surgery planning though un-invasive histopathological categorization. Radiomics may be able to determine a tumor’s response to treatment, make an accurate diagnosis, and forecast a prognosis (56). In addition to offering extra prognostic data, radiomic analysis’ ability to non-invasively differentiate between various glioma molecular subtypes would aid in the choice of targeted chemotherapy for patients with numerous genetic mutations and possibly high-grade tumor types (57–59). Additionally, it would improve surgery, which is necessary to maintain median survival (60). Therefore, treatment responses, progression-free survival, and overall survival can all be more accurately predicted with the application of radiomic risk models (61, 62). It is possible to evaluate the effectiveness of anti-angiogenic therapy without endangering the patient by non-invasively acquiring the radio-genomic profile of a tumor (63). Our study has some limitations that need to be clarified and discussed: a single retrospective center study and a small sample size. This would not allow a validation of the new VASARI 2.0 method proposed by use on a large scale quickly. However, the number of cases is in line with for the type of analysis described. Future studies with multi-center data or larger cohorts needed for a full validation of the new VASARI lexicon that we proposes, in order to eliminate the risk of data bias, which could affect the generalizability of the study results. However, there are also strong points for the use of the proposed VASARI 2.0 lexicon in daily clinical radiological practice. It does not require specific software to automate the scoring process. There are methodological innovations in the evaluation of the MRI VASARI features, thanks to adequate changes in the reference intervals as reported in Table 1. Familiarization with this new lexicon is easy. It can be easily used in daily clinical practice also because it can be of valid help in capturing the most salient aspects to be described in the report. It can represent a valuable tool for producing a structured and standardized report with the aim of offering with simplicity and clarity all the salient information needed by the neuro-oncology core group (oncologist, radiotherapist, neurosurgeon, and neuoradiologist). It would be desirable to conduct a large-scale multi-center study to then draft a new VASARI lexicon guide based on the validation results.
The evaluation of gliomas with modified ranges/score of VASARI 2.0 allows the prediction of the outpoint (IDH status and grade) with AUC > 0.8, higher than that of traditional VASARI. Thus, the new score could be used in pre-surgical evaluation of gliomas in a method both suitable with clinical practice and that can also be the starting point for subsequent studies of radiomics and machine learning.
All relevant data is contained within the article. Additional data are available upon request to interested researchers.
The studies involving humans were approved by Institutional Review Board - ASL NA1 Centro. The studies were conducted in accordance with the local legislation and institutional requirements. The human samples used in this study were acquired from a by-product of routine care or industry. Written informed consent for participation was not required from the participants or the participants’ legal guardians/next of kin in accordance with the national legislation and institutional requirements. Written informed consent was obtained from the individual(s) for the publication of any potentially identifiable images or data included in this article.
AN: Conceptualization, Data curation, Formal analysis, Funding acquisition, Investigation, Methodology, Project administration, Resources, Software, Supervision, Validation, Visualization, Writing – original draft, Writing – review & editing. LG: Conceptualization, Data curation, Formal analysis, Investigation, Methodology, Project administration, Resources, Software, Supervision, Validation, Visualization, Writing – original draft, Writing – review & editing. MT: Conceptualization, Data curation, Formal analysis, Investigation, Methodology, Project administration, Resources, Software, Supervision, Validation, Visualization, Writing – original draft, Writing – review & editing. GP: Data curation, Methodology, Writing – review & editing. RI: Data curation, Methodology, Visualization, Writing – review & editing. MM: Data curation, Writing – review & editing. AE: Supervision, Writing – review & editing. FT: Supervision, Validation, Writing – review & editing. VD’A: Supervision, Validation, Visualization, Writing – review & editing. Members of ODM Multidisciplinary Neuro-Oncology Group: Data curation, Formal analysis, Resources, Software.
The author(s) declare that no financial support was received for the research, authorship, and/or publication of this article.
Authors want to recognize all members of ODM Multidisciplinary Neuro-Oncology Group as effective participants to the study. Ospedale del Mare, ASL NA1 Centro, Naples, Italy: Oncology Unit: Pasqualina Giordano and Bruno Daniele; Radiotherapy Unit: Maria Francesca Giugliano, Marcella Gaetano, and Cesare Guida; Nuclear Medicine Unit: Eugenio Di Giorgio and Marco Spadafora; Pathological Anatomy Unit: Alfredo D’Avino and Antonio D’Antonio; Neurosurgery Unit: Carmela Chiaramonte, Federico Frio, Francesco De Falco, Francesco Colaleo, and Giuseppe Catapano.
The authors declare that the research was conducted in the absence of any commercial or financial relationships that could be construed as a potential conflict of interest.
All claims expressed in this article are solely those of the authors and do not necessarily represent those of their affiliated organizations, or those of the publisher, the editors and the reviewers. Any product that may be evaluated in this article, or claim that may be made by its manufacturer, is not guaranteed or endorsed by the publisher.
1. Omuro A, DeAngelis LM. Glioblastoma and other Malignant gliomas: A clinical review. JAMA. (2013) 310:1842–50.
2. Parsons DW, Jones S, Zhang X, Lin JC-H, Leary RJ, Angenendt P, et al. An integrated genomic analysis of human glioblastoma multiforme. Science. (2008) 321:1807–12.
4. Bai J, Varghese J, Jain R. Adult glioma WHO classification update, genomics, and imaging: what the radiologists need to know. Top Magn Reson Imaging. (2020) 29:71–82.
5. Louis DN, Perry A, Wesseling P, Brat DJ, Cree IA, Figarella-Branger D, et al. The 2021 WHO classification of tumors of the central nervous system: A summary. Neuro Oncol. (2021) 23:1231–51.
6. Yan H, Parsons DW, Jin G, McLendon R, Rasheed BA, Yuan W, et al. IDH1 and IDH2 mutations in gliomas. N Engl J Med. (2009) 360:765–73.
7. Hartmann C, Meyer J, Balss J, Capper D, Mueller W, Christians A, et al. Type and frequency of IDH1 and IDH2 mutations are related to astrocytic and oligodendroglial differentiation and age: A study of 1010 diffuse gliomas. Acta Neuropathol. (2009) 118:469–74.
8. Houillier C, Wang X, Kaloshi G, Mokhtari K, Guillevin R, Laffaire J, et al. IDH1 or IDH2 mutations predict longer survival and response to temozolomide in low-grade gliomas. Neurology. (2010) 75:1560–6.
9. König IR, Fuchs O, Hansen G, Von Mutius E, Kopp MV. What is precision medicine? Eur Respir J. (2017) 50:1700391.
10. Yang K, Wu Z, Zhang H, Zhang N, Wu W, Wang Z, et al. Glioma targeted therapy: Insight into future of molecular approaches. Mol Cancer. (2022) 21:39.
11. Leibetseder A, Preusser M, Berghoff AS. New approaches with precision medicine in adult brain tumors. Cancers. (2022) 14:712.
12. Dunbar E, Yachnis AT. Glioma diagnosis: Immunohistochemistry and beyond. Adv Anat. Pathol. (2010) 17:187–201.
13. Nicholson JG, Fine HA. Diffuse glioma heterogeneity and its therapeutic implications. Cancer Discovery. (2021) 11:575–90.
14. Weller M, van den Bent M, Preusser M, Le Rhun E, Tonn JC, Minniti G, et al. EANO guidelines on the diagnosis and treatment of diffuse gliomas of adulthood. Nat Rev Clin Oncol. (2021) 18:170–86.
16. Habib A, Jovanovich N, Hoppe M, Ak M, Mamindla PR, Colen R, et al. MRI-based radiomics and radiogenomics in the management of low-grade gliomas: evaluating the evidence for a paradigm shift. J Clin Med. (2021) 1:1411.
17. Bhandari A, Sharma C, Ibrahim M, Riggs M, Jones R, Lasocki A. The role of 2-hydroxyglutarate magnetic resonance spectroscopy for the determination of isocitrate dehydrogenase status in lower grade gliomas versus glioblastoma: A systematic review and meta-analysis of diagnostic test accuracy. Neuroradiology. (2021) 63:1823–30.
18. Lu J, Li X, Li H. Perfusion parameters derived from MRI for preoperative prediction of IDH mutation and MGMT promoter methylation status in glioblastomas. Magn Reson Imag. (2021) 83:189–95.
19. You W, Mao Y, Jiao X, Wang D, Liu J, Lei P, et al. The combination of radiomics features and VASARI standard to predict glioma grade. Front Oncol. (2023) 13:1083216. doi: 10.3389/fonc.2023.1083216
20. The Cancer Imaging Archive. VASARI Research Project, in: The Cancer Genome Atlas (2020). Available online at: https://wiki.cancerimagingarchive.net/display/Public/VASARI+Research+Project (Accessed 4 Jun 2022).
21. Gemini L, Tortora M, Giordano P, Prudente ME, Villa A, Vargas O, et al. Vasari scoring system in discerning between different degrees of glioma and IDH status prediction: A possible machine learning application? J Imaging. (2023) 9:75.
22. Sampaio L, Linhares P, Fonseca J. Detailed magnetic resonance imaging features of a case series of primary gliosarcoma. Neuroradiol J. (2017) 30:546–53.
23. Setyawan NH, Choridah L, Nugroho HA, Malueka RG, Dwianingsih EK. Beyond invasive biopsies: using VASARI MRI features to predict grade and molecular parameters in gliomas. Cancer Imaging. (2024) 24:3.
24. Nam YK, Park JE, Park SY, Lee M, Kim M, Nam SJ, et al. Reproducible imaging-based prediction of molecular subtype and risk stratification of gliomas across different experience levels using a structured reporting system. Eur Radiol. (2021) 31(10):7374–85. doi: 10.1007/s00330-021-08015-4
25. Peeken JC, Goldberg T, Pyka T, Bernhofer M, Wiestler B, Kessel KA, et al. Combining multimodal imaging and treatment features improves machine learning-based prognostic assessment in patients with glioblastoma multiforme. Cancer Med. (2019) 8(1):128–36.
26. Rajput SR, Ravals MS. A review on end-to-end methods for brain tumor segmentation and overall survival prediction. Azerbaijan J High Perform Computs. (2020) 3(1):119–38.
27. Kocher M, Ruge MI, Galldiks N, Lohmann P. Applications of radiomics and machine learning for radiotherapy of malignant brain tumors. Strahlenther Onkol. (2020) 196(10):856–67.
28. Shen G, Wang R, Gao B, Zhang Z, Wu G, Pope W. The MRI features and prognosis of gliomas associated with IDH1 mutation: A single center study in southwest china. Front Oncol. (2020) 10:852. doi: 10.3389/fonc.2020.00852
29. Li X, Zhu Y, Kang H, et al. Glioma grading by microvascular permeability parameters derived from dynamic contrast-enhanced MRI and intratu moral susceptibility signal on susceptibility weighted imaging. Cancer Imaging. (2015) 15(1):4.
30. Hsu CCT, Watkins TW, Kwan GNC, Haacke EM. Susceptibility-weighted imaging of glioma: Update on current imaging status and future directions. J Neuroimaging. (2016) 26(4):383–90.
31. Smits M, van den Bent MJ. Imaging correlates of adult glioma geno types. Radiology. (2017) 284(2):316–31.
32. Jain R, Griffith B, Alotaibi F, et al. Glioma angiogenesis and perfusion imaging: Understanding the relationship between tumor blood volume and leakiness with increasing glioma grade. Am J Neuroradiol. (2015) 36(11):2030–5.
33. Su CQ, Lu SS, Han QY, Zhou MD, Hong XN. Intergrating conventional MRI, texture analysis of dynamic contrast-enhanced MRI, and susceptibility weighted imaging for glioma grading. Acta Radiol. (2019) 60(6):777–87.
34. Yu J, Wang M, Song J, Huang D, Hong X. Potential utility of visually AcceS able rembrandt images assessment in brain astrocytoma grading. J Comput Assist Tomogr. (2016) 40(2):301–6.
35. Rong JD, Ding F, Li J, Zhu GY, He Z. Correlation of influencing factors with the extent of peritumoral brain glioma edema. Zhonghua Yi Xue Za Zhi. (2013) 93:169–71.
36. Lacroix M, Abi-Said D, Fourney DR, Gokaslan ZL, Shi W, De Monte F, et al. A multivariate analysis of 416 patients with glioblastoma multiforme: prognosis, extent of resection and survival. JNeurosurg. (2001) 95:190–8.
37. Olar A, Wani KM, Alfaro-Munoz KD, Heathcock LE, van Thuijl HF, Gilbert MR, et al. IDH mutation status and role of WHO grade and mitotic index in overall survival in grade II–III diffuse gliomas. Acta Neuropathol. (2015) 129(4):585–96.
38. Zhang B, Chang K, Ramkissoon S, Tanguturi S, Bi WL, Reardon DA, et al. Multimodal MRI features predict isocitrate dehydrogenase genotype in high-grade gliomas. Neuro Oncol. (2017) 19(1):109–17.
39. Nakae S, Murayama K, Sasaki H, et al. Prediction of genetic subgroups in adult supra tentorial gliomas by pre- and intraopera tive parameters. J Neurooncol. (2017) 131:403–12.
40. Lasocki A, Tsui A, Gaillard F, Tacey M, Drummond K, Stuckey S. Reliability of noncontrast-enhancing tumor as a biomarker of IDH1 mutation status in glioblastoma. J Clin Neurosci. (2017) 39:170–5.
41. Wang K, Wang Y, Fan X, Wang J, Li G, Ma J, et al. Radiological features combined with IDH1 status for predicting the survival outcome of glioblastoma patients. Neuro Oncol. (2016) 18:589–97.
42. Qi S, Yu L, Li H, Ou Y, Qiu X, Ding Y, et al. Isocitrate dehydrogenase mutation is associated with tumor location and magnetic resonance imaging characteristics in astrocytic neoplasms. Oncol Lett. (2014) 7:1895–902.
43. Carrillo JA, Lai A, Nghiemphu PL, Kim HJ, Phillips HS, Kharbanda S, et al. Relationship between tumor enhancement, edema, IDH1 mutational status, MGMT promoter methylation, and survival in glioblastoma. AJNR Am J Neuroradiol. (2012) 33:1349–55.
44. Delfanti RL, Piccioni DE, Handwerker J, et al. Imaging correlates for the 2016 update on WHO classification of grade II/III gliomas: implications for IDH, 1p/19q and ATRX status. J Neurooncol. (2017) 135:601–60.
45. Yamashita K, Hiwatashi A, Togao O, Kikuchi K, Hatae R, Yoshimoto K, et al. MR imaging-based analysis of glioblastoma multiforme: Estimation of IDH1 mutation status. Am J Neuroradiol. (2016) 37:58–65.
46. Kickingereder P, Sahm F, Radbruch A, Wick W, Heiland S, Deimling AV, et al. IDH mutation status is associated with a distinct hypoxia/angiogenesis transcriptome signature which is non-invasively predictable with rCBV imaging in human glioma. Sci Rep. (2015) 5:16238.
47. Park YW, Han K, Ahn SS, Bae S, Choi YS, Chang JH, et al. Prediction of IDH1-mutation and 1p/19q-codeletion status using preoperative MR imaging phenotypes in lower grade gliomas. Am J Neuroradiol. (2018) 39:37–42.
48. Patel SH, Poisson LM, Brat DJ, Zhou Y, Cooper L, Snuderl M, et al. T2–FLAIR mismatch, an imaging biomarker for IDH and 1p/19q status in lower-grade gliomas: A TCGA/TCIA project. Clin Cancer Res. (2017) 23:6078–86.
49. Villanueva-Meyer JE, Wood MD, Choi B, Mabray MC, Butowski NA, Tihan T, et al. MRI features and IDH mutational status in grade II diffuse gliomas: Impact on diagnosis and prognosis. AJR Am J Roentgenol. (2018) 210:621.
50. Xing Z, Yang X, She D, Lin Y, Zhang Y, Cao D. Noninvasive assessment of IDH mutational status in world health organization grade II and III astrocytomas using DWI and DSC-PWI combined with conventional MR imaging. AJNR Am J Neuroradiol. (2017) 38:1134–44.
51. Leu K, Ott GA, Lai A, Nghiemphu PL, Pope WB, Yong WH, et al. Perfusion and diffusion MRI signatures in histologic and genetic subtypes of WHO grade II–III diffuse gliomas. J Neurooncol. (2017) 134:177–88.
52. Xiong J, Tan W, Wen J, Pan J, Wang Y, Zhang J, et al. Combination of diffusion tensor imaging and conventional MRI correlates with isocitrate dehydrogenase1/2 mutations but not 1p/19q genotyping in oligodendroglial tumours. Eur Radiol. (2016) 26:1705–15.
53. Wasserman JK, Nicholas G, Yaworski R, Wasserman AM, Woulfe JM, Jansen GH, et al. Radiological and pathological features associated with IDH1-R132H mutation status and early mortality in newly diagnosed anaplastic astrocytic tumours. PloS One. (2015) 10:e0123890.
54. Lee S, Choi SH, Ryoo I, et al. Evaluation of the micro-environmental heterogeneity in high-grade gliomas with IDH1/2 gene mutation using histogram analysis of diffusion-weighted imaging and dynamic-susceptibility contrast perfusion imaging. J Neurooncol. (2015) 121:141–50.
55. Merkaj S, Bahar RC, Zeevi T, Lin M, Ikuta I, Bousabarah K, et al. Machine learning tools for image-based glioma grading and the quality of their reporting: Challenges and opportunities. Cancers. (2022) 14:2623.
56. Rapisarda A, Melillo G. Overcoming disappointing results with antiangiogenic therapy by targeting hypoxia. Nat Rev Clin Oncol. (2012) 9:378–90.
57. Beig N, Patel J, Prasanna P, Hill V, Gupta A, Correa R, et al. Radiogenomic analysis of hypoxia pathway is predictive of overall survival in glioblastoma. Sci Rep. (2018) 8:7.
58. Kickingereder P, Burth S, Wick A, Götz M, Eidel O, Schlemmer H-P, et al. Radiomic profiling of glioblastoma: Identifying an imaging predictor of patient survival with improved performance over established clinical and radiologic risk models. Radiology. (2016) 280:880–9.
59. McGarry SD, Hurrell SL, Kaczmarowski AL, Cochran EJ, Connelly J, Rand SD, et al. Magnetic resonance imaging-based radiomic profiles predict patient prognosis in newly diagnosed glioblastoma before therapy. Tomography. (2016) 2:223–8.
60. Liu X, Li Y, Qian Z, Sun Z, Xu K, Wang K, et al. A radiomic signature as a non-invasive predictor of progression-free survival in patients with lower-grade gliomas. NeuroImage Clin. (2018) 20:1070–7.
61. Zhou H, Vallières M, Bai HX, Su C, Tang H, Oldridge D, et al. MRI features predict survival and molecular markers in diffuse lower-grade gliomas. Neuro Oncol. (2017) 19:862–70.
62. Kickingereder P, Götz M, Muschelli J, Wick A, Neuberger U, Shinohara RT, et al. Large-scale radiomic profiling of recurrent glioblastoma identifies an imaging predictor for stratifying anti-angiogenic treatment response. Clin Cancer Res. (2016) 22:5765–71.
Keywords: VASARI, MRI, glioma, IDH status, grade tumor, neuroradiology
Citation: Negro A, Gemini L, Tortora M, Pace G, Iaccarino R, Marchese M, Elefante A, Tortora F, D'Agostino V and members of ODM Multidisciplinary Neuro-Oncology Group (2024) VASARI 2.0: a new updated MRI VASARI lexicon to predict grading and IDH status in brain glioma. Front. Oncol. 14:1449982. doi: 10.3389/fonc.2024.1449982
Received: 16 June 2024; Accepted: 18 November 2024;
Published: 23 December 2024.
Edited by:
Domenico Aquino, IRCCS Carlo Besta Neurological Institute Foundation, ItalyCopyright © 2024 Negro, Gemini, Tortora, Pace, Iaccarino, Marchese, Elefante, Tortora, D'Agostino and members of ODM Multidisciplinary Neuro-Oncology Group. This is an open-access article distributed under the terms of the Creative Commons Attribution License (CC BY). The use, distribution or reproduction in other forums is permitted, provided the original author(s) and the copyright owner(s) are credited and that the original publication in this journal is cited, in accordance with accepted academic practice. No use, distribution or reproduction is permitted which does not comply with these terms.
*Correspondence: Alberto Negro, QWxiZXJ0by5OZWdyb0Bob3RtYWlsLml0
Disclaimer: All claims expressed in this article are solely those of the authors and do not necessarily represent those of their affiliated organizations, or those of the publisher, the editors and the reviewers. Any product that may be evaluated in this article or claim that may be made by its manufacturer is not guaranteed or endorsed by the publisher.
Research integrity at Frontiers
Learn more about the work of our research integrity team to safeguard the quality of each article we publish.