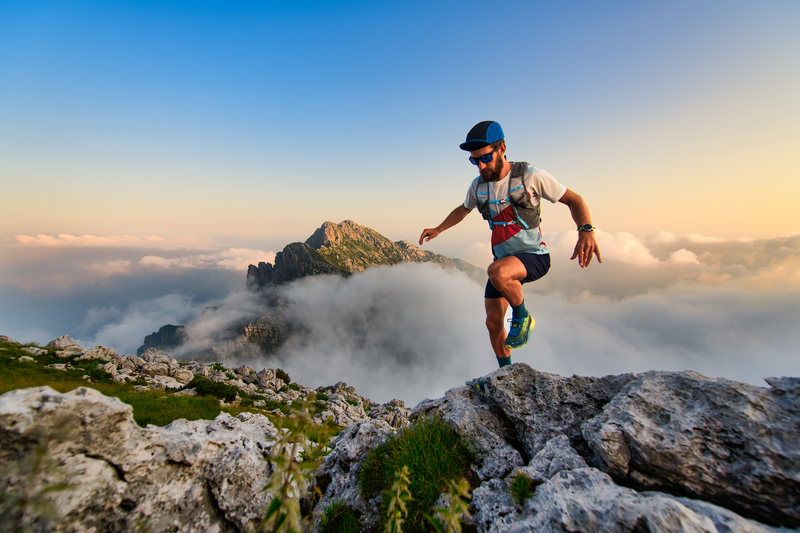
94% of researchers rate our articles as excellent or good
Learn more about the work of our research integrity team to safeguard the quality of each article we publish.
Find out more
ORIGINAL RESEARCH article
Front. Oncol. , 05 December 2024
Sec. Pediatric Oncology
Volume 14 - 2024 | https://doi.org/10.3389/fonc.2024.1445173
This article is part of the Research Topic Pediatric Myeloid Neoplasms: New Insights Into Diagnosis, Prognosis, and Treatment View all 4 articles
Introduction: Despite remarkable progress in Pediatric Acute Myeloid Leukemia (pAML) treatments, the relapsed disease remains difficult to treat, making it pertinent to identify novel biomarkers of prognostic/therapeutic significance.
Material and methods: Bone marrow samples from 21 pAML patients were analyzed using single cell RNA sequencing, functional assays with ARMH1 knockdown and overexpression were performed in leukemia cell lines to evaluate impact on proliferation and migration, and chemotherapy sensitivity. Mitochondrial function was assessed via Seahorse assay, ARMH1 interacting proteins were studied using co-immunoprecipitation. Bulk RNA-seq was performed on ARMH1knockdown and over expressing cell lines to evaluate the pathways and networks impacted by ARMH1.
Results: Our data shows that ARMH1, a novel cancer-associated gene, is highly expressed in the malignant blast cells of multiple pediatric hematologic malignancies, including AML, T/B-ALL, and T/B-MPAL. Notably, ARMH1 expression is significantly elevated in blast cells of patients who relapsed or have a high-risk cytogenetic profile (MLL) compared to standard-risk (RUNX1, inv (16)). ARMH1 expression is also significantly correlated with the pediatric leukemia stem cell score of 6 genes (LSC6) associated with poor outcomes. Perturbation of ARMH1 (knockdown and overexpression) in leukemia cell lines significantly impacted cell proliferation and migration. The RNA-sequencing analysis on multiple ARMH1 knockdown and overexpressing cell lines established an association with mitochondrial fatty acid synthesis and cell cycle pathways.The investigation of the mitochondrial matrix shows that pharmacological inhibition of a key enzyme in fatty acid synthesis regulation, CPT1A, resulted in ARMH1 downregulation. ARMH1 knockdown also led to a significant reduction in CPT1A and ATP production as well as Oxygen Consumption Rate. Our data indicates that downregulating ARMH1 impacts cell proliferation by reducing key cell cycle regulators such as CDCA7 and EZH2. Further, we also established that ARMH1 is a key physical interactant of EZH2, associated with multiple cancers.
Conclusion: Our findings underscore further evaluation of ARMH1 as a potential candidate for targeted therapies and stratification of aggressive pAML to improve outcomes.
Pediatric Acute myeloid leukemia (pAML) accounts for about 20% of childhood leukemia (1). The prognosis and treatment of pAML remain challenging because of disease heterogeneity, high relapse rates, and therapy-associated toxicity (2). It is important to identify novel risk stratification strategies that can be used to better guide therapy decisions and improve outcomes for pAML. A deeper characterization of the pAML is essential to overcome the above-mentioned limitations. Recent studies in AML indicated that dynamic changes in the bone marrow microenvironment from the time of disease diagnosis to relapse, including alterations in the immune microenvironment, might be responsible for poor outcomes (3, 4). The therapeutics for advanced AML are undergoing a paradigm shift with the advent of immune therapy, mainly Chimeric Antigen Receptor (CAR) T-cell therapy, resulting in sustained disease responses in a subset of previously incurable patients (5). While measurable residual disease (MRD) status is critical for post-therapy patient stratification and clinical assessment, it is not infallible, with relapse occurring in some cases, even after the intense end of consolidation therapy irrespective of MRD status. It is important to identify novel risk stratification strategies that can be used to better guide therapy decisions and improve clinical outcomes for pAML patients. To achieve deep characterization of pediatric AML, Therapeutically Applicable Research to Generate Effective Treatments (TARGET) AML and other large-scale genetic profiling initiatives have generated large-scale genomics data. These initiatives indicated that AML is a genetically diverse disease having various gene mutations, insertions, and fusions associated with high risk and poor prognosis (6–8). Alongside bulk genomic data, the utilization of single-cell RNA sequencing (scRNA-seq) for profiling bone marrow microenvironment (BMM) of AML patients has revealed the molecular diversity in the blast and non-blast cells. The single-cell profiling attained better molecular characterization of AML BMM along with the identification of biomarkers/targets that can distinguish AML blast cells (AML-blasts) from non-malignant cells (non-AML cells). Single-cell profiling approaches have helped in deciphering the cellular composition of the leukemic BMM, the cellular transcriptional states associated with leukemic processes at the time of diagnosis (Dx), and the presence of residual blast cells at the end of induction (EOI) post-chemotherapy (9–13). To understand the role of tumor cells and their microenvironment in pediatric AML prognosis, we performed scRNA-seq on 21 pAML BMM samples from diagnosis (Dx), end of induction (EOI), and relapse time points (14). Analysis of matched Dx and EOI scRNA-seq data along with healthy bone marrow, hematopoietic stem cells, and TARGET AML dataset revealed a novel pAML blast-associated 7-gene signature (i.e., CLEC11A, PRAME, AZU1, NREP, ARMH1, C1QBP, TRH). This novel signature depicted excellent performance for pAML blast cell identification on a publicly available dataset and an independent validation cohort (14). The comparative analysis of pAML-blast cell profiles at Dx between future relapse and complete remission (CR) samples depicted overexpression of a novel Armadillo Like Helical Domain Containing 1 (ARMH1) gene in the relapse samples with minimal or null expression in immune and healthy stromal cells from human single-cell atlas. Higher expression of ARMH1 was also observed in the therapy-resistant blast cells, suggesting potential involvement in the manifestation of therapeutic resistance as well as influencing outcomes. Additionally, publications from our group in characterizing T-cell acute lymphocyte leukemia (T-ALL) (15) and Mixed Phenotype leukemia (MPAL) also depicted higher expression of ARMH1 in the leukemic cells as compared to normal cells, indicating its association with other acute leukemias. The mechanistic involvement of ARMH1 in leukemogenesis warrants further functional and molecular studies. Correlative analysis of ARMH1 expression with enrichment of blast cells, and leukemia risk scores depicted a significant positive association indicating ARMH1’s role in leukemogenesis. Further in vitro knockdown and overexpression of the ARMH1 resulted in significant dysregulation in the cell proliferation and migration as well as modified sensitivity to a chemotherapy agent. The functional analysis using RNA-sequencing on ARMH1 perturbed cells depicted a significant association with cholesterol and fatty acid biosynthesis and cell cycle (i.e., CDCA7, P21) pathways. Further, inhibition of the fatty acid pathway using a chemical inhibitor resulted in a significant reduction in ARMH1 levels and cellular proliferation, supporting its role in disease progression. Additionally, the cellular respiration analysis using seahorse assay also depicts ARMH1’s impact on mitochondrial cellular respiration. Therefore, this study provides the first evidence about the molecular mechanism of ARMH1 in leukemogenesis that can be explored in future clinical trials to develop novel therapies.
Our group conducted a single-cell RNA sequencing (scRNA-seq) analysis of pediatric AML BMM samples for in-depth characterization of leukemic blasts and other tumor microenvironment cells (14). A unique AML-blast associated 7-gene signature was identified by analyzing integrated scRNA-seq data from matched Dx and EOI AML BM samples and TARGET AML bulk dataset by implementing a supervised machine learning technique, support vector machine (SVM) approach. The seven gene signature achieved excellent performance on training and independent validation sets in distinguishing AML blast cells from the immune microenvironment cells (AUC >0.78) (14). The signature also depicted elevated expression in malignant blast cells as compared to other non-blast (microenvironment cells) profiled in the 20 patient samples by the single cell assay (Figure 1A). Further analysis of AML samples at diagnosis revealed that ARMH1 expression not only blast-specific but the expression was also elevated in the blast cells from patients that relapsed (marked with dotted lasso) in comparison to blast cells from patients who achieved Clinical Remission (CR) (Figure 1B). In our previous study, ARMH1 expression was observed to be significantly higher in scRNA-seq data of AML Dx blast cells compared to EOI non-blast cells (P<0.001) (14). ARMH1, also known as C1orf228, has not been previously associated with AML or other pediatric cancers. ARMH1 encodes for Armadillo-like helical domain containing protein1 with unknown function and association with diseases. To investigate its involvement in AML, we performed further validation on large-scale TARGET AML RNA-seq data. ARMH1 depicted an association with clinical blast percentage, with expression slightly increasing as blast percentages increase from <30% to >60% (Figure 1C). This finding provides evidence of ARMH1 blast cells associated expression. Additionally, expression of ARMH1 in AML malignant cells from an external pediatric AML study (16) was correlated with the enrichment of a leukemia stem cell score of 6 genes (LSC6) that predicts AML outcome reliably with high LSC6 scores associated with poor outcome (17). When malignant cells are stratified by their LSC6 scores into three groups, ARMH1 expression incrementally increases from low to medium to high LSC6 groups (P<0.0001). The malignant AML cells from this study with the highest LSC6 scores also had the highest ARMH1 expression (Figure 1D). Besides AML, our group also performed in-depth characterization of other pediatric leukemias, Acute lymphocytic leukemia (T-ALL, B-ALL) and mixed phenotypic acute leukemia (T- MPAL, B-MPAL) (18). ARMH1 depicted significantly higher expression in T-ALL (P<0.0001), B-ALL (P<0.0001), T-MPAL (P<0.0001), B-MPAL (P<0.0001), blast cells as compared to normal non-blast cells (Figure 1E). This pattern of blast cell restrictive expression of ARMH1 indicates its potential association with leukemogenesis. Moreover, the investigation of levels of ARMH1 expression in samples from three patients with a high-risk cytogenetic profile (MLL) and six patients with a standard risk cytogenetic categorization [RUNX1, inv (16)] revealed that ARMH1 expression was significantly higher (P<0.0001) in high-risk (MLL) compared to standard-risk [RUNX1, inv (16)] group (Figure 1F). This is further validated in TARGET-AML BM diagnosis samples showing significantly (P=1.3e-05) higher ARMH1 expression in the high-risk cytogenetic subtypes (i.e., MLL) compared to standard-risk [e.g., RUNX1, inv (16)] (Figure 1G). Utilizing temporal AML samples collected at Dx, EOI, and Relapse timepoints of the same patients, we assessed ARMH1 expression in blast cells across disease stages. We observed ARMH1 expression was mostly localized to blast cells across time points, in blasts at diagnosis, as well as residual blasts at EOI (P=2.18e-34). (Figure 1H). A survival analysis of ARMH1 expression in pediatric AML data from TARGET depicted a significant association between ARMH1 (P=0.00199) overexpression and poor overall survival. These correlative analysis results provide substantial evidence for ARMH1 association with high-risk or aggressive pediatric acute leukemias, specifically AML. These lay the foundation to evaluate the functional impacts of ARMH1 through perturbation studies described in the following sections of the manuscript.
Figure 1. Differential ARMH1 expression in leukemic blasts revealed by scRNA-seq analysis. (A) Heatmap showing expression of the genes from the seven gene signatures across blast and non-blast cells in 20 AML samples collected at disease diagnosis. Patient IDs are represented by numbers along columns and are grouped into REL (relapse) and REM (remission) groups based on their outcome. Blue and red colors represent low and high expression, respectively. (B) UMAPs showing ARMH1 expression in scRNA-seq data of AML samples at diagnosis (left) with low to high expression colored light to intense orange colors. Blast clusters are lassoed on the feature plot. The UMAP plot (right) shows cellular grouping with non-blast cells shown in blue and blast cells from remission (REM) and relapse (REL) in orange and red colors, respectively. An inset violin plot shows the ARMH1 expression in non-blast immune microenvironment cells, remission, and relapse blast cells. (C) Violin plot with ARMH1 expression in the bulk RNA-seq TARGET-AML samples stratified based on clinical blast percentages. Samples are grouped based on diagnosis blast percentages [Low (<30%), Medium (30%-60%), High (>60%)]. Points represent median values per group. (D) AML blast cells from (16) study are grouped by pLSC6 score (left). ARMH1 expression is plotted for each pLSC6 group (low, mid, high) (right). (E) Mean and standard deviation of ARMH1 expression in blast (purple) and non-blast (pink) cells across different pediatric acute leukemias: AML (acute myeloid leukemia), B-MPAL (B-cell/Myeloid mixed phenotype acute leukemia), B-ALL (B-cell acute lymphoid leukemia), T-MPAL (T-cell/Myeloid MPAL), and T-ALL (T-cell ALL). (F) Violin plot of ARMH1 expression in diagnosis AML samples categorized by cytogenetic risk. High risk (purple) represents MLL patients, and standard risk (green) represents RUNX1 and inv (16) patients. (G) ARMH1 expression in TARGET-AML BM samples collected at disease diagnosis from patients with different cytogenetic risk categories [high risk=MLL, standard risk=RUNX1, inv (16)]. The significance of differences in expression levels throughout the analysis was determined using the Wilcoxon Rank-Sum test. The Bonferroni method was used to adjust p-values for multiple comparisons. If the p-value is below 0.0001, it is indicated on the plots. (H) UMAP with ARMH1 expression in the residual blast and non-blast immune microenvironment cells identified from comparison of DX and End of Induction (EOI) samples. Blast clusters are lassoed on the UMAP. The boxplot shows significantly higher expression of ARMH1 in residual blast cells at EOI compared to non-blast immune cells.
Following promising findings regarding the increased expression of ARMH1 (Figure 1) in malignant and therapy-resistance aggressive AML cells, we initiated functional studies to delve into the role of ARMH1 in leukemogenesis. To evaluate the functional impact of ARMH1, we modulated the expression of ARMH1 by knockdown and overexpression and measured the effect on cellular proliferation and migration. We achieved ARMH1 knockdown (shARMH1) vs. wild type of ARMH1 (shCrt) and overexpression (ARMH1 Oe) vs wild type of ARMH1 (empty vector=EV) in three different AML cell lines, MOLM14 (n=3), HEL92.1.7 (n=3), and only knockdown in Kasumi-1 (n=3). The knockdown and overexpression were confirmed by western blot in MOLM14 (Figure 2A), HEL92.1.7 (Figure 2B), and Kasumi-1 cell lines (Supplementary Figure 1A). We next performed cellular proliferation, migration, and dose-response assays. The cellular proliferation analysis revealed a substantial reduction in cell proliferation upon knockdown of ARMH1 in MOLM14 (P<0.0001), HEL92.1.7 (P=0.0012), whereas a significant increase in cell proliferation upon ARMH1 overexpression in MOLM14 (P<0.0001), HEL92.1.7 (P=0.0005) (Figures 2C, E) was observed. Likewise, we observed a significant decrease in cell proliferation in ARMH1 knockdown in Kasumi-1 (P<0.0001) (Supplementary Figure 1B) cell lines. Analysis of cell migration showed that the knockdown of ARMH1 led to a significant decrease in cell migration in MOLM14 (P=0.0016) and HEL92.1.7 (P<0.0001), while overexpression significantly increased cell migration in MOLM14 (P=0.005) and HEL92.1.7 (P=0.0077) cell lines (Figures 2D, F). A significant decrease in migration was also noted upon ARMH1 knockdown in Kasumi-1 (P<0.0001) (Supplementary Figure 1C). To verify the impact of ARMH1 on propagation in normal non-leukemic cells, we also performed the knockdown of ARMH1 in normal human cell lines, HEK293T, and Mesenchymal stromal cells (MSC) cells. After the knockdown of ARMH1, no significant alteration in cell proliferation was observed in MSCs (P=0.9358) (Figure 2G) and HEK293T (P=0.3925) (Figure 1D). Additionally, we investigated ARMH1’s potential role in altering the therapeutic index of traditional chemotherapy (i.e., Cytarabine) for pAML. We observed an improvement in sensitivity to Cytarabine in ARMH1 knockdown relative to wild-type in MOLM14 (n=3, p=0.0088), HEL92.1.7 shCrt vs shARMH1 (n=3) (p=0.0077), and Kasumi-1 shCrt vs shARMH1 (n=3) (p=0.0004) (Figure 2H). The survival analysis on ARMH1 expression in pAML data from the TARGET dataset performed using the Survival Genie tool (n=144) depicted a significant association between ARMH1 (HR=2.4, P=0.00199) overexpression and poor overall survival (19) (Figure 2I). These results underscore the involvement of ARMH1 in cancer cell proliferation, migration, and on mediating resistance to therapeutic regimens. To further understand the molecular mechanism of ARMH1, we performed RNA-seq on ARMH1 perturbed cells to measure genome-level transcriptome dysregulations.
Figure 2. Knockdown and overexpression of ARMH1 modulate AML cell proliferation and migration and better susceptibility to chemotherapy. (A) Left panel: Western blot of MOLM 14 cell lysates probed with ARMH1 antibody and total H3 antibody as loading control (3 independent replicates) with lentiviral transduced ARMH1 knockdown (shARMH1) and shControl (shCrt). Right panel: ARMH1 overexpressed (Oe) using lentiviral transduced in MOLM14 cells with ORF (pLX-317) or Empty Vector (EV). (B) Left panel: Western blot of HEL92.1.7 cell lysates probed with ARMH1 antibody and total H3 antibody as loading control in lentiviral transduced shARMH1 and shCrt cells. Right panel: ARMH1 Oe in lentiviral transduced ORF (pLX-317), and EV. (C) Bar plots showing cell proliferation measured by CCK-8 in MOLM14 shARMH1 cells along with shCrt in the top panel, and MOLM14 cells with ARMH1 EV along with ARMH1 Oe in the lower panel. Statistical analysis: Unpaired student’s t-test (n=3), data are means (absorbance in 450nm) +/-SEM of independent biological replicates (n=3). (D) Bar plots showing the migration assay results that were conducted by using the fluorometric format in MOLM14 cell lines with shARMH1, shCrt along with EV and ARMH1 Oe, the results of shARMH1 and ARMH1 Oe are shown in the top and bottom panels respectively. Statistical analysis: Unpaired student’s t-test (n=3), data are means of (Relative Florescence Unit (RFU) +/-SEM of independent biological replicates. (E) Bar plots showing cell proliferation measured by CCK-8 of HEL92.1.7 with shARMH1 cells along with shCrt in the top panel, and HEL92.1.7 cells with EV along with ARMH1 Oe HEL 92.1.7 cells in the lower panel. Statistical analysis: Unpaired student’s t-test (n=3), data are means (absorbance in 450nm) +/-SEM of independent biological replicates. (F) Bar plot showing the migration assay results of HEL92.1.7 cell line with shARMH1, shCrt along with EV, and ARMH1 Oe. The HEL92.1.7 migration assay results for shARMH1 and ARMH1 Oe are shown in the top and bottom panels, respectively. Statistical analysis: Unpaired student’s t-test (n=3), data are means of (Relative Florescence Unit (RFU) +/-SEM of independent biological replicates. (G) Left panel: Western blot of Mesenchymal Stromal Cell Line (MSC) lysate probed with ARMH1 antibody and total H3 antibody as loading control with lentiviral transduced shRNA for targeting ARMH1 and shCrt (3 independent replicates). The lower bands in H3 are non-specific bands. Right panel: Bar chart of cell proliferation measured by CCK-8 in MSC shARMH1, and shCrt. Statistical analysis: Unpaired student’s t-test (n=3), data are means (absorbance in 450nm) +/-SEM of independent biological replicates. (H) The bar graph shows individual IC50 values for each of the three independent biological replicates on the Y-axis. IC50 (Cytarabine treatment, nM) reported for shARMH1 and shCrt cells that were exposed to increasing concentration of Cytarabine in MOLM14 (p=0.0088), HEL92.1.7 (p=0.0077) and Kasumi-1 (p=0.0004). The p-value was calculated using a two-tailed Student’s t-test on graph pad prism 10. (I) Kaplan-Meier curve shows the overall survival in pAML patients of the TARGET dataset, grouped by ARMH1 expression. High expression of ARMH1 is associated with poor outcomes in this cohort (HR=2.4 (95% CI=1.4-4.1) log-rank p=0.000557). The Survival Genie platform (https://bhasinlab.bmi.emory.edu/SurvivalGenie2/home) was used to perform survival analysis. The samples were grouped into “low” and “high” groups based on an optimal threshold calculated using the cutP algorithm. Using these groupings, we calculate the survival probability and generate Kaplan Meier curves with corresponding log-rank p-value, which represents the significance of the difference between the curves for “high” and “low” expression groups. A Cox proportional hazard ratio (HR) is also calculated between the high and low samples; an HR>1 represents poor survival, whereas an HR<1 represents an association with better survival.
To characterize gene expression level alterations in ARMH1 perturbed cells and explore the affected pathways, bulk RNA sequencing was performed. Unsupervised Principal Component Analysis illustrates distinct separations among the three treatment groups (ARMH1 Oe, shCrt/EV, and shARMH1) for MOLM14 (Figure 3A) and HEL92.1.7 cell lines indicating distinct transcriptome profiles of groups (Figure 3B). The differential expression analysis on MOLM14 cell lines identified 2,924 and 3,276 significantly (Adj. P < 0.01) differentially expressed (upregulated as well as downregulated) genes, respectively upon ARMH1 knockdown and overexpression. Similar analysis on ARMH1 knockdown and over-expressing HEL92.1.7 cell lines identified 1,777 and 1,184 significantly (Adj. P < 0.01) differentially expressed genes, respectively. To identify genes that followed the same expression patterns as ARMH1 (i.e. increased with ARMH1 overexpression and decreased with ARMH1 knockdown), we performed a comparative analysis of differentially expressed genes using UpSet plots (20) (Figure 3C, top plot in red) and those that followed the opposite expression pattern as ARMH1 (i.e. decreased with ARMH1 overexpression and increased with ARMH1 knockdown; the bottom plot in blue). The analysis identified 106 genes that were significantly (P < 0.05) upregulated with ARMH1 overexpression and downregulated with ARMH1 knockdown in at least three of the four contrasts. Conversely, there was one gene, LIMD2, that was significantly downregulated with ARMH1 overexpression and upregulated with shARMH1 in all four contrasts (P-value: MOLM14 ARMH1 Oe = 0.0271, MOLM14 shARMH1 = 0.0196, HEL ARMH1 Oe= 0.0016, HEL shARMH1 = 0.0001), and 114 other genes that were significant in at least three of the four contrasts at P < 0.05. Therefore, we considered 106 genes that followed the same expression patterns as ARMH1 and 115 genes that followed the opposite expression patterns as an ARMH1 perturbed core signature. The expression levels of selected genes from this core signature were visualized in the heatmap depicting very concordant patterns across ARMH1 perturbed (i.e., knockdown, overexpression) and control cell lines (MOLM14, HEL92.1.7) (Figure 3D). Among the top genes co-regulated with ARMH1 include SLAIN1, CYFIP2, NEDD4L, CA2, ZNF738, FAHD2CP, PLOD3, SLITRK5, KDSR, TAF1D, DBF4B, WDR35, RPUSD3 and ZNF691. Several of these genes were studied by other teams, such as SLAIN1 (21) (previously reported to contribute to cancer cell proliferation and therapy resistance) as well as CYFIP2 (22) and CA2 (23) (currently being explored as prognostic biomarkers). Pathway analysis using the 106 genes (genes boxed in green in Figure 3C; co-regulated with ARMH1) depicts the significant enrichment of pathways related to cholesterol biosynthesis (P = 0.0023) and mitochondrial fatty acid synthesis (P = 0.0288). For the second group (genes boxed in yellow in Figure 3C; showing opposite trends (counter-regulated) to ARMH1 protein expression), the pathways relating to the overall regulation of inflammation (P = 0.0007) as well as the angiogenesis pathway through VEGFA and VEGFR2 (P = 0.0084) were significantly enriched (Figure 3E). Further gene set enrichment analysis on the normalized expression values of co-regulated genes depicted upregulation of TCA cycle in senescence (P-value: MOLM14 shARMH1 = 0.0024, HEL ARMH1 Oe = 0.0118, HEL shARMH1 = 0.0291) and short-chain fatty acid catabolic processes (P-value: MOLM14 ARMH1 Oe = 0.0450, MOLM14 shARMH1 = 0.0111, HEL ARMH1 Oe = 0.0014, HEL shARMH1 = 0.0254) while analysis on the genes with an opposite expression pattern depicted perturbation of death receptor signaling (P-value: MOLM14 ARMH1 Oe = 0.0050, MOLM14 shARMH1 = 3.04e-5, HEL ARMH1 Oe = 0.0013, HEL shARMH1 = 0.0007) and Histone H3 K4 Dimethylation (P-value: MOLM14 ARMH1 Oe = 0.0010, MOLM14 shARMH1 = 0.0029, HEL ARMH1 Oe = 0.0162, HEL shARMH1 = 0.0282) (Figure 3F). Further co-expression network analysis on the co-regulated genes revealed one gene, RAN binding protein 17 (RANBP17) that was identified as a central hub gene in both the MOLM14 and HEL co-expression Networks (Figure 3G). RANBP17 is a member of the importin-beta superfamily of nuclear transport receptors. The expression of RANBP17 has been implicated in the progression of many cancers (24, 25), and it was also reported as a potential prognostic biomarker for glioblastoma (26). Additionally, RANBP17 is reported to be a member of the ARMH1 superfamily, according to the Hugo Gene Nomenclature Committee, suggesting that it could be closely related to ARMH1 in both structure and function (27). To further investigate the overall impact of the ARMH1 co-expressed genes on AML outcomes, we performed a survival analysis using the TARGET-AML cohort. The survival analysis showed that higher expression levels of ARMH1 co-expressed genes (n=88 genes with valid gene symbols out of the 106 co-expressed genes) were significantly associated (HR=3.6, P=0.0003) with poorer overall survival (Figure 3H). In summary, the bulk RNA-seq data suggests ARMH1 is likely a key component of the cellular metabolic process, particularly in the cholesterol and fatty acid synthesis pathways that occur in the mitochondria. Next, we investigated the role of ARMH1 in fatty acid synthesis in mitochondria as well as in cell cycle regulation.
Figure 3. Transcriptome profiling of ARMH1 perturbed cells for exploration of gene and pathway alterations. (A) PCA plot with first two principal components derived from the normalized gene expression matrix using PCA for the seven samples obtained from the MOLM14 cell line. Each dot represents a sample, and distinct separation among treatment groups (ARMH1 Oe, shCrt/EV, and shARMH1) is observed. (B) Similar to (A), the PCA plot illustrates the first two principal components for the eight samples collected from the HEL cell line after the PCA transformation of the normalized gene expression matrix. Notable separation among treatment groups is evident. (C) UpSet plot displaying intersecting genes in MOLM14 and HEL92.1.7 cell lines that are perturbed by ARMH1 Oe and shARMH1. The top plot (red) shows co-expressing genes significantly upregulated in ARMH1 Oe and downregulated in shARMH1, and the bottom plot (blue) shows counter-regulated genes significantly downregulated in ARMH1 Oe and upregulated in shARMH1 at p < 0.05. Genes selected for downstream analyses (significant at P < 0.05 in at least three comparisons and consistent fold change) are highlighted with green and orange boxes. (D) Heatmap displaying relative expression values for selected ARMH co-expressed (genes from the green box in (C) (top) and counter-regulated (genes from the orange box in (C) (bottom). All genes were significant at p < 0.05 in at least three out of four comparisons. Gene expression is shown in pseudocolor with red denoting increase and blue decrease in gene expression. The rows and columns represent the genes and samples, respectively. (E) The top 10 pathways upregulated in ARMH1 co-expressed gene (red), as well as the top 10 pathways downregulated in ARMH1 counter-regulated genes. (F) Dot plot of the top 10 gene sets following a similar or co-expression pattern as ARMH1 (text in red) and gene sets following the opposite or counter-expression pattern as ARMH1 (text in blue). Gene sets are significant in at least three of the four contrasts at p < 0.05 and are ordered by the average of the absolute value log2FCs from the four comparisons listed on the x-axis. (G) Gene co-expression networks generated from ARMH1 co-expressed genes. Hub genes are labeled, with the gene common between both cell lines with the highest degree of connections. (H) Survival association analysis of ARMH1 co-expressed genes using pAML data from the TARGET initiative, with groups stratified based on enrichment score for ARMH1 co-expressed genes. Patients with higher enrichment scores for ARMH1 co-expressed genes have poor survival (HR=3.6 (95% CI=1.8-7.2) log-rank p=0.000076). The symbol ★ are notable pathways selected for further analysis.
AML cells exhibit an abnormal metabolic profile, which includes a higher need for Oxidative Phosphorylation and Fatty Acid Metabolism for survival and therapeutic resistant phenotype (28). Our RNA-seq analysis on ARMH1 knockdown and overexpression cells revealed that the mitochondrial fatty acid synthesis pathway was significantly (P=0.02875) dysregulated (Figure 3F) and provided clues for an underlying mechanism for ARMH1 association with leukemogenesis. To investigate ARMH1 association with Oxidative phosphorylation and ATP production, we first focused on studying the fatty acid Oxidative cycle by targeting a key enzyme associated with transferring long-chain fatty acids into mitochondria, which is located in the outer layer of the mitochondria membrane (29), Carnitine palmitoyl transferase 1A (CPT1A). By pharmaceutical inhibition of CPT1A, using Etomoxir (150uM), we observed that ARMH1 expression was decreased by inhibiting CPT1A in MOLM14 and HEL92.1.7 cell lines (Figure 4A). We also observed a reduction in the expression of CPT1A following the knockdown of ARMH1 in MOLM14 cell lines indicating potential co-regulations of ARMH1 and CPT1A (Figure 4B). In addition, we observed that CPT1A has significant co-expression with ARMH1 in AML samples from TARGET cohort (r=0.18, P=3.5e-14) (Supplementary Figure 2C). CPT1A has a significant role in mitochondrial fatty acid synthesis, and its expression is associated with increased cell proliferation and suppression of apoptosis in various cancers, including leukemia (30–32). Overexpression of CPT1A has been consistently linked to adverse outcomes in a variety of cancers, including AML (30, 33). Targeting CPT1A in leukemia cell lines leads to a decrease in cancer cell proliferation (34) and a reduction in apoptosis (35). To substantiate these findings, we performed the Seahorse assay to measure the Oxygen Consumption Rate (OCR) in both MOLM14 and HEL92.1.7 ARMH1 knockdown and control wild-type cells. The data demonstrated a notable reduction in OCR in ARMH1 knockdown in HEL 92.1.7 (P<0.0001) and MOLM14 (P<0.0001) cells compared to respective controls (Figures 4C, D). We also investigated the involvement of ARMH1 in the regulation of mitochondrial fatty acid synthesis by conducting a rescue study. We supplemented the cell culture media with an exogenous lipid mixture and conducted a cell proliferation assay in ARMH1 knockdown compared to wild-type conditions. The supplementation of lipid mixture restored cell proliferation of ARMH1 knockdown in MOLM14 and HEL92.1.7 cells to a level similar to control wild-type cells. This data indicated that the lipid mixture compensates for the reduction in proliferation due to ARMH1 knockdown (Figure 4E). In summary, these findings provide preliminary evidence of ARMH1 involvement in mitochondrial fatty acid synthesis in leukemia cells.
Figure 4. ARMH1 shows potential association with mitochondrial fatty acid synthesis and Cell cycle regulation. (A) Left panel: western blot shows the inhibition of CPT-1A and ARMH1 by Etomoxir (150uM) and DMSO control treatment of the MOLM14 cell line (3 independent replicates). Right panel: western blot shows the inhibition of CPT-1A and ARMH1 by Etomoxir (150uM) and DMSO control treatment of the HEL92.1.7 cell line (3 independent replicates). (B) Western blot of MOLM14 cell lysates probed with ARMH1, CPT1A antibody, and total H3 antibody as loading control with lentiviral transduced shARMH1 or shCrt (3 independent replicates). The CPT-1A expression is significantly lower in cells with ARMH1 knockdown cells. (C) Bar plot showing an Oxygen Consumption Rate (OCR) in shARMH1 and shCrt MOLM14 and HEL92.1.7 cells. Statistical analysis: Unpaired student’s t-test (n=15), data are means of [OCR (pmol/min)] +/-SEM of independent biological replicates. (D) Respiration stress test indicates the basal respiration and reflects the energy requirements under conditions, IA=Oligomycin (2.5uM), IIB=FCCP (0.5uM), and IIIC=Antimycin (2uM) and Rotenone (2uM). (E) Bar plot showing cell proliferation assay in shARMH1 and shCrt MOLM14 and HEL92.1.7 cells, the cell culture media supplemented with exogenous lipid mix (Lipid Mixture 1, Cat#10288, Sigma-Aldrich). The proliferation was measured at 72 hours. Statistical analysis: Unpaired student’s t-test, data are means (absorbance in 450nm) +/-SEM of independent biological replicates (n=4). (F) Left panel: Bar plot of normalized expression of CDCA7 and Right panel: c-MYC in shARMH1 and shCrt MOLM14 and HEL92.1.7 cells. Statistical analysis: Unpaired student’s t-test, data are normalized expression+/-SEM of c-MYC and CDCA7 from independent biological replicates (HEL92.1.7 Control/Knockdown, MOLM14 Control: n=3, HEL92.1.7 Overexpression, MOLM14 Knockdown/Overexpression: n=2). (G) Left Panel: Western blot of cell lysate probed with ARMH1, CDCA7, and total H3 as loading control with lentiviral transduced shCDCA7 and shCrt. (3 independent replicates). Right panel: Western blot of MOLM14 cell lysates probed with ARMH1 and CDCA7 antibody and total H3 antibody as loading control with lentiviral transduced shARMH1 and shCrt (3 independent replicates). (H) Log-normalized expression of CDKN1A in pAML bone marrow diagnosis (n=20) and healthy (n=4) samples. AML samples are grouped into REL (relapse), and REM (remission) based on future outcomes. Wilcoxon Rank-Sum test was used to compare expressions between groups, and p-values were reported (p<0.0001). (I) Upper panel: Kaplan-Meier curve shows overall survival (left) and event-free survival (EFS) (right) of pAML samples from the TARGET initiative by stratifying samples into high and low groups based on CPT1A expression. Lower panel: Kaplan-Meier curve shows overall survival (left) and event-free survival (EFS) (right) of pAML samples from the TARGET initiative by stratifying samples into high and low groups based on enrichment score for mitochondrial fatty acid synthesis gene set obtained from MsigDB via msigdbr (v7.5.1) from the Broad Institute. The Survival Genie platform (https://bhasinlab.bmi.emory.edu/SurvivalGenie2/home) was used to perform survival analysis. The samples were grouped into “low” and “high” groups based on an optimal threshold calculated with the cutP method. Using these groupings, we calculate the survival probability and generate Kaplan Meier curves with corresponding log-rank p-value, which represents the significance of the difference between the curves for “high” and “low” expression groups. A Cox proportional hazard ratio (HR) is calculated between the high and low samples, an HR>1 represents poor survival, whereas an HR<1 represents an association with better survival. The asterisk (-) means 0 concentration of drug.
In addition to impacting mitochondrial fatty acid synthesis, RNA-sequencing data also shows that ARMH1 is associated with the dysregulation of the cell cycle by affecting the expression of key cell cycle regulators such as Cell Division Cycle Associated 7 (CDCA7) and Cyclin-dependent kinase inhibitor 1A (CDKN1A or P21) genes. CDCA7 has been previously reported to play a significant role in cancer progression in diverse types of malignancies (36–41). CDCA7 shows periodic expression in the cell cycle, peaking between G1/S phases (42). Studies have also demonstrated a connection between CDCA7 and c-MYC, a potent cancer oncogene (43). In our RNA sequencing data, we identified that expression of CDCA7 and c-MYC significantly decreased in ARMH1 knockdown cell lines for CDCA7, MOLM14 wild type compared to shARMH1 (P=0.0035), and HEL92.1.7 wild type compared to shARMH1 (P=0.0002) and for c-MYC MOLM14 wild type compared to shRAMH1 (P=0.0005) and HEL92.1.7 wild type compared to shARMH1 (P=0.0002) (Figure 4F). Further, correlative analysis of using AML data from TARGET cohort revealed ARMH1 expression has a significant correlation with CDCA7 (r=0.29, P<2.2e-16) and c-MYC (r=0.2, P=4.6e-16) which suggests intersection between their regulatory pathways in leukemia cells (Supplementary Figure 2C). Through in vitro assays, we observed a co-expression between ARMH1 and CDCA7. Knockdown of ARMH1 led to a remarkable reduction in CDCA7 expression. Conversely, after the knockdown of CDCA7, the expression of ARMH1 also exhibited a similar reduction in MOLM14 (Figure 4G). A similar co-expression pattern was observed between ARMH1 and CDCA7 in HEL92.1.7, underscoring the co-regulation or interaction (Supplementary Figure 2D). In addition, we also investigated the association between ARMH1 and P21, which function as a suppressor of the cell cycle, thereby inhibiting cell growth (44, 45). Our Bulk RNA-seq analysis depicted an upregulation of P21 in ARMH1 knockdown in MOLM14 (P=0.0106), and in HEL 92.1.7 (P=0.0320) cell lines as compared to wild-type controls (Supplementary Figure 2E). The analysis of our single-cell data (14), including bone marrow samples of healthy control and patients at diagnosis who went on to experience relapse or remission, reveals significant overexpression of P21 in remission patients compared to relapse (P<0.0001) (Figure 4H). Further, survival analysis on CPT1A and mitochondrial fatty acid pathway (MSigDB, GO:0019395) expression in pediatric AML data from the TARGET initiative depicted a significant association between CPT1A (OS P=0.00249, EFS P=0.047) and mitochondrial fatty acid synthesis (OS P=0.000837, EFS P=0.00085) overexpression with poor overall and event-free survival in TARGET-AML cohort (Figure 4I).
Collectively, these findings indicate ARMH1 is involved in the modulation of the mitochondrial fatty acid synthesis pathway in pediatric AML. Further, in the absence of ARMH1, the cell cycle is arrested by upregulation of the checkpoint inhibitor, P21, and downregulation of the expression of CDCA7.
Studies have previously demonstrated that the enhancer of zeste homolog 2 (EZH2) plays a regulatory role in Lipid Metabolism, and simultaneous targeting of EZH2 and Fatty Acid Synthesis results in cancer cell death (46). Previous studies have shown that EZH2 increases lipid synthesis in a variety of cancers (47–49) and is associated with poor prognosis (50, 51). Moreover, EZH2 has been reported as a multifaced regulator across different types of cancers, potentially functioning both as an oncogene and a tumor-suppressor gene in cancer (52, 53). However, recent studies have underscored the remarkable role of EZH2 in hematologic malignancies and leukemogenesis (54, 55). Our data suggest that ARMH1 may serve as an interactant of EZH2 associated with high-risk and therapeutic resistance patients in pediatric AML. We observed that the knockdown of either EZH2 or ARMH1 in MOLM14 cells diminishes the expression of both EZH2 and ARMH1 proteins (Figure 5A). Additionally, in ARMH1 knockdown cells, we observed downregulation of Embryonic Ectoderm Development (EED) and Suppressor of Zest 12 (SUZ12) the core component of the Polycomb repressive complex 2 (PRC2) along with EZH2, which highlights ARMH1’s role in PRC2 complex destabilization (Figure 5B). Similar results were observed in the HEL92.1.7 cell line for EZH2 knockdown (Supplementary Figure 2F). To further support the possibility of EZH2 and ARMH1 physical interaction, we either inhibited the enzymatic activity of EZH2 using EPZ6438 (an inhibitor targeting the zest domain of EZH2) or destabilized EZH2 protein and PRC2 complex using DZNep (3-Deazaneplanocin A). After treatment of AML cells with EPZ6438, while protein level EZH2 was not altered, its enzymatic activity was downregulated as determined based on reduced levels of H3k27me3 (Figure 5C). We observed that the expression of ARMH1 remained at almost the same level or slightly changes. On the other hand, after DZNep treatment, a potent destabilizer of the EZH2, the ARMH1 protein expression was decreased significantly along with a decrease in levels of EZH2 (Figure 5C). This data indicates that ARMH1 and EZH2 may have physical interaction together, which occurs post-translationally. For further investigation of EZH2 and ARMH1 interaction, we performed Co-Immunoprecipitation (CO-IP) by pulling down EZH2 and EED in MOLM14 and Kasumi-1 cell lines. The pull-down product analysis using western blot showed a signal for both EZH2, EED, and ARMH1 proteins, suggesting a possible physical interaction among these proteins (Figure 5D). Also, the protein expression analysis of EZH2 and ARMH1 in patient samples has shown a higher expression for both in diagnostic samples from patients who experienced relapse (patient#1) as compared to those who achieved remission (patients#13,17,19,20) (Figure 5E). Moreover, the survival analysis on EZH2 expression in the TARGET AML dataset depicted a significant association between high expression of EZH2 and poor overall survival (P=0.00249), and event-free survival (P=0.033) (Figure 5F). Taken together, this highlights the importance of ARMH1 as EZH2 interactant at the post-translational level and provides insights into its involvement in high-risk factors and therapeutic resistance mechanisms, in pediatric AML.
Figure 5. ARMH1 is a novel interactant of EZH2 in leukemia. (A) Western blot of MOLM14 cell lysates probed with ARMH1 and EZH2 antibody and total H3 antibody as loading control with lentiviral transduced shEZH2 or shCrt (3 independent replicates). Left panel: replicates#1,2 of shEZH2 (the lower bands in H3 are non-specific bands), and right panel: shEZH2 replicate#3. (B) Western blot of cell lysates of MOLM14, Kasumi-1, and HEL, probed with ARMH1, EED, EZH2, and SUZ12 antibody and total H3 as loading control with lentiviral transduced shARMH1 or shCrt (3 independent replicates). (C) Western blot of detecting ARMH1, EZH2, and H3K27me3 in MOLM14 and HEL92.1.7 cell lines in (3 independent replicates) after treatment of EPZ6438 (150uM) and DZNep (0.5uM) over 72 hours. The membrane was first probed with H3K27me3 and then stripped and probed with total H3. (Lower bands in H3 are non-specific bands). (D) Western blot on Co-IP pull-down using EED (Upper Panel) and EZH2 (Lower Panel) antibodies for MOLM14 and Kasumi-1 cells extract. The membranes were probed with EZH2, EED, and ARMH1 antibodies. (E) Western blot on patient samples collected at disease diagnosis from patients with future relapse (patient#1) and remission (patients#13,17,19,20) probed with ARMH1, EZH2, and total H3 and Tubulin as loading control. (F) Kaplan-Meier curve shows overall survival (left), and event-free survival (EFS) (right) based on the expression of EZH2. The Survival Genie platform (https://bhasinlab.bmi.emory.edu/SurvivalGenie2/home) was used to perform survival analysis. The samples were grouped into “low” and “high” groups based on an optimal threshold calculated with the cutP method. Using these groupings, we calculate the survival probability and generate Kaplan Meier curves with corresponding log-rank p-value, which represents the significance of the difference between the curves for “high” and “low” expression groups. A Cox proportional hazard ratio (HR) is calculated between the high and low samples, an HR>1 represents poor survival, whereas an HR<1 represents an association with better survival. The asterisk (*) means control for replicates.
The emergence of resistance to conventional chemotherapies is increasing, and it can be stimulated by different mechanisms and finally leads to adverse outcomes in pAML patients, while cytogenetic assessments and molecular abnormalities provide pivotal prognostic indicators and predictive capabilities, pAML continues to exhibit high mortality rate due to lack of robust biomarker that reliably indicates a relapse (56, 57). In the current study, we report that ARMH1 may serve as a potential biomarker for relapse in pediatric AML. Recently, ARMH1 has been identified as a potential biomarker gene for the immunological score-based risk stratification signature in oral squamous cell carcinoma (58), but ARMH1 is still reported to be an unknown function gene and has not been studied in detail in cancer, especially leukemias. This is the first study attempt to characterize ARMH1 at both functional and molecular levels in pediatric AML. Our data suggests that the knockdown of ARMH1 leads to a significant reduction in cell proliferation and migration as well as slightly enhances response toward conventional chemotherapy. Conversely, overexpression of ARMH1 leads to increasing cell proliferation and migration rates. Interestingly, these effects are similar to very well-studied genes that play a critical role in tumorigenesis in AML, such as c-Kit proto-oncogene, Runt-related transcription factors, RUNX1, and FLT3 (59–61). In the current research, we observed that ARMH1 has a notable role in the progression of AML, correlating significantly with relapse and poor clinical outcomes. Results from another team reveal that the mitochondrial fatty acid synthesis pathway assumes a critical role in the progression of AML (62, 63). Furthermore, inhibition of the mitochondrial fatty acid synthesis pathway in combination with conventional chemotherapy protocol has shown a notable impact on inhibiting leukemia progression (64). Findings from other teams signify that AML exhibits a dependency on mitochondrial metabolism and mitochondrial fatty acid synthesis pathways (65). In this study, to characterize the molecular landscape of ARMH1 perturbation effects, we leveraged RNA-seq and Pathway analysis on ARMH1 knockout, overexpressing, and control cells. The RNA-seq analysis enrichment of the mitochondrial fatty acid synthesis pathway in the genes that are consistently positively correlated with ARMH1 expression across cell lines. Our Seahorse assay data also demonstrated that knockdown of ARMH1 leads to a significant reduction in mitochondrial OCR. This finding highlighted the potential linkage of ARMH1 to the mitochondrial fatty acid synthesis pathway in AML tumorigenesis. Additionally, our data indicated that ARMH1 has a role in the regulation of the cell cycle. CDCA7 has been consistently reported in many research studies to play a remarkable role in tumor progression in various types of cancers (38, 66–68). In the current paper, an analysis of differential gene expression using bulk RNA seq data has shown that CDCA7 ranks as the gene showing significant co-expression with ARMH1, during in vitro studies, ARMH1 knockdown leads to downregulation of CDCA7, and knockdown CDCA7 causes downregulation of ARMH1. This finding highlighted the regulatory role of ARMH1 in influencing the expression of CDCA7 in pediatric leukemia, which has never been reported. Also, our data indicated that the knockdown of ARMH1 leads to the upregulating of the checkpoint inhibitor gene, P21, resulting in cell cycle arrest. In the current study, we also identified that the knockdown of ARMH1 leads to the destabilization of the PRC2 complex and the degradation of EZH2 and EED, a core component of the PRC2 complex. Our data highlighted that ARMH1 and EZH2 may have protein-protein interactions, indicating functional interdependence between these two genes. Several studies have reported that EZH2 downregulation has been associated with poor prognosis (Stomper et al., 2021; Kempf et al., 2021; Göllner et al., 2017). On the other hand, multiple studies across solid tumors show contrary results, while EZH2 overexpression has been linked to poor prognosis (69–71). These studies highlight a dual and puzzling role of EZH2 in cancer progression that might be influenced by complex cancer genetics and microenvironment. Our finding suggests that in pediatric AML the downregulation of ARMH1 along with EZH2 has been associated with good outcomes. Taken together, the absence of ARMH1 is a good prognostic marker as its upregulation is associated with aggressive disease, so it can be used in addition to cytogenetics for identifying patients with high relapse probability. It is involved in two cancer hallmark pathways related to metabolism and cell cycle, making it a good therapeutic target. Fatty acid metabolism-based targeting might provide a novel way to treat high-risk pediatric AML.
In conclusion, our study identified ARMH1 as a novel biomarker for aggressive pediatric AML, demonstrating ARMH1’s significant association with disease progression and relapse. Through comprehensive analysis, we elucidated the impact of ARMH1 on leukemic cell proliferation, migration, and chemotherapy sensitivity, as well as its involvement in mitochondrial fatty acid synthesis and cell cycle regulation. These findings underscore ARMH1 as a potential prognostic indicator for patient stratification and a therapeutic target for enhancing clinical remission rates. Further investigation into ARMH1’s role in AML pathogenesis will be required to improve advancing personalized treatment strategies and improving pAML patient outcomes.
One limitation of this study is the lack of animal models to complement the findings obtained from in vitro studies. Consequently, the conclusion of findings solely from in vitro or clinical studies may not capture the entirety of the pathobiological role of ARMH1 in pediatric leukemia. Limited understanding of the ARMH1 effect on immune cells is another limitation of the study.
The single-cell AML dataset was generated in our lab previously, and ARMH1 was identified among a 7-gene signature that distinguished AML malignant cells (14). Viable frozen AML bone marrow samples were obtained from the Aflac Cancer and Blood Disorders Center, Children’s Healthcare of Atlanta, and processed for generating high-quality single-cell RNA sequencing data on 20 samples. These BM samples were viably thawed and processed for single-cell transcriptome profiling using the 10x Chromium Controller, followed by library preparation with 10x Genomics Single Cell 3`v3, and 5`v1 reagent kits. Sequencing was performed on the Novaseq 6000 platform. The raw scRNA-seq data was demultiplexed, filtered, and aligned to the human reference genome (hg38) using Cell Ranger software by 10x Genomics (v3). This output single-cell count matrices for each sample, which were then pre-processed, integrated, and normalized using Seurat package (v4.3) functions (72). To account for the two batches of data generated from our lab (batch 1: samples 1-15, batch 2: samples 16-20), the integration anchors method (Seurat) was used to integrate and perform batch correction. Log normalization, scaling, PCA, UMAP, and clustering were applied to generate processed single-cell expression data for downstream analysis. Among the 20 patients in our dataset, seven experienced relapse, and 13 experienced clinical remission. This dataset is publicly available on the Gene Expression Omnibus repository under accession GSE235923. In addition to our lab’s 20-sample AML dataset, we also included a publicly available 8-sample AML scRNA-seq dataset from the GEO repository (GSE154109) (12). Seurat functions were again used for log normalization, scaling, PCA, UMAP, and clustering to generate normalized single-cell expression data for downstream analysis. Among the patients from this dataset (12), six experienced remission, and two experienced relapse.
Total RNA was extracted from cell lines of different groups (HEL shCrt/EV (n=3), HEL shARMH1 (n=3), HEL ARMH1 Oe (n=2), MOLM14 shCrt/EV (n=3), MOLM14 shARMH1 (n=2) and MOLM14 ARMH1 Oe (n=2) using RNeasy Micro Kit (Cat# 74004, Qiagen) according to the instructions provided by the manufacturer. RNA concentration was evaluated by photometric measurement at 260/280 nm, and quality was assessed using the Agilent Bioanalyzer platform. For each group, sequencing was performed on two or three biological replicates. Double-stranded cDNA sequencing libraries were generated using the Illumina TruSeq kit per the manufacturer’s protocol. High-quality libraries were sequenced on an Illumina Novaseq 6000 platform. To achieve comprehensive coverage for each sample, we generated ~20–30 million paired-end reads.
The raw sequencing data was processed to remove any adaptor, PCR primers, and low-quality transcripts using FASTQC (73) and fastx. These provide a very comprehensive estimate of sample quality based on read quality, read length, GC content, sequence duplication, adaptor, and PCR primer contamination. These high-quality, clean reads were aligned against the human genome using the hisat2 aligner (74). We used GRCh38 human genome assembly as the reference genome for alignment. Gene expression measurement was performed from aligned reads by counting the unique reads using the htseq-count algorithm (75). The read count-based gene expression data was normalized based on library complexity and gene variation using the Voom algorithm (76). The normalized count data underwent comparison among groups using the limma method (77) to identify differentially expressed genes (raw p < 0.05). Principal Component Analysis (PCA) was used to capture the variation in the data across different biological groups. Supervised analyses focused on identifying differentially expressed genes through four distinct comparisons: overexpression vs. control and knockdown vs. control for each cell line. Our primary aim was to identify co-expressing genes that are upregulated and downregulated in ARMH1 Oe and shARMH1 cell lines respectively, but we were also interested in identifying counter-expressing genes that are downregulated in ARMH1 Oe and upregulated in shARMH1. Genes were selected for downstream analysis based on their consistency in differential expression patterns and statistical significance across the different contrasts. Due to limited overlap between the four groups, genes were included if they exhibited expression changes consistent with the direction of interest (logFC > 0 in overexpression and logFC < 0 in knockdown for co-expressed genes and the opposite for counter-expressed genes) and demonstrated statistical significance (P < 0.05) in at least three out of the four contrasts.
Pathway enrichment analysis was conducted using the Enrichr tool (77) to identify pathways significantly affected by ARMH1 perturbations. Specifically, enrichment analysis was carried out separately for ARMH1 co-expressed and counter-regulated genes utilizing the ‘WikiPathway 2023 Human database. Pathways with a P-value < 0.05 were determined to be significantly perturbed by ARMH1. To capture subtle pathway level perturbations due to ARMH1, Gene Set Variation Analysis (GSVA) was performed using the gsva R package (78). Using the Hallmark, C2: CP, C3: TFT, and C5: GO gene sets from MSigDB (79) as reference, the voom-derived normalized expression values were transformed through GSVA into sample-wise enrichment scores for each gene set. Subsequently, the limma package (77) was utilized to conduct differential testing on these enrichment scores obtained from GSVA. This step enabled the identification of gene sets that are significantly (P < 0.05) upregulated or downregulated in response to ARMH1 overexpression or knockdown, respectively, or vice versa (78–80). Next, the BioNERO package (81) was utilized to construct gene co-expression networks from genes that were co-expressed with ARMH1. Using the voom-derived normalized expression values, we constructed signed hybrid co-expression networks for each cell line employing Pearson’s correlation metric with a module merging threshold of 1.0. Hub genes within the resulting network were identified based on BioNERO’s principles of module membership and degree of nodes. To mitigate potential unwanted effects from genes with low log fold-change values, this process was iterated by iteratively removing the gene with the lowest average logFC among the four contrasts and re-running the network analysis with the same parameters as mentioned above. Hub genes that were common between the MOLM14 and HEL cell lines in any of these runs were selected for further investigation. Finally, we utilized Survival Genie (19) to conduct survival analysis on genes significantly (P < 0.05) upregulated in ARMH1 Oe and downregulated in shARMH1 in at least three contrasts. This analysis aimed to investigate whether disparities in gene expression levels correlated with survival probabilities in the TARGET-AML patient cohort. Employing the cutp method from the R package survMisc (https://cran.r-project.org/package=survMisc), we partitioned the TARGET-AML cohort into two distinct groups, characterized by relatively high or low expression levels of the ARMH1-perturbed genes. Subsequently, Kaplan-Meier survival curves were constructed, and a hazard ratio, indicative of the magnitude of differences between these high and low expression groups, was computed. The confidence interval and log-rank p-value for the hazard ratio were then determined to assess the significance of any observed differences. Additionally, we further utilized the TARGET-AML cohort to examine the association of ARMH1 expression with risk in AML. Primary BM samples from TARGET-AML were grouped into high-risk – MLL cytogenetic subtype – and standard risk – RUNX1, inv (16) cytogenetic subtypes. Then, voom-derived normalized expression values were obtained for ARMH1, and expression was compared in high-risk and standard-risk samples using a Wilcoxon rank-sum test.
Leukemia cells MOLM14, HEL92.1.7, Kasumi-1, and normal cell HEK293T were generously provided by Dr. Douglas K. Graham and Dr. Deborah DeRyckere’s labs (Emory University, Department of Pediatric, Hematology, and Oncology division) and Mesenchymal Stromal Cells (MSC) were kindly provided by Dr. Subra Kugathasan’s lab (Emory University, Pediatric Department, Children’s Healthcare of Atlanta Combined Center for Pediatric Inflammatory Bowel Disease). Leukemia cells were cultured in RPMI 1640 media supplemented with 10% FBS, 100U/ml penicillin/streptomycin, and 1% Glutomax. HEK293T and MSC cells were cultured in DMEM F12 media supplemented with 10% FBS 100U/ml penicillin/streptomycin and 1% Glutomax. All cell lines were checked periodically for Mycoplasma contamination using MycoAlert® Mycoplasma Detection Kit (Cat#LT07-418). During the in vitro experiments, the cells were free of Mycoplasma contamination.
To detect cell viability, we used Bio-Rad Trypan Blue (Cat#1450021) and automated cell counter TC20 (Cat#1450102). The cells with more than 90% viability were utilized for experiments.
To generate ARMH1, EZH2, and CDCA7 knockdown cells as well as ARMH1 overexpression cells, the lentivirus was generated with transfection of shRNAs (pLKO.1) and the Lentiviral Packaging Kit (Lenti-vpak, Cat#TR30037, OriGene) by following manufacturer protocol. Three shRNAs were used for ARMH1 knockdown, with clone IDs as follows: TRCN0000168648 with target sequence: GCTGAGGACTTGTACATGAAA in 3UTR, TRCN0000172632 with target sequence: GAGTCACATCCTCGACAAGTT in CDS, and TRCN0000336686 with target sequence: CAAGTGTATAAAGGTCTAATA in CDS. One validated shRNA was used for EZH2 knockdown; the clone ID was TRCN0000040077 with the target sequence: CCCAACATAGATGGACCAAAT in CDS. One validated shRNA was used for CDCA7 knockdown; the clone ID was TRCN0000017937 with target sequence: CCAGTTATTGGCGGAATTGAA in CDS. We utilized ARMH1 cloned in the Open Reading Frame plasmid (ORF_pLX-317) for overexpression of ARMH1. All plasmids were purchased from Millipore Sigma, Inc. Plasmid extraction: Bacteria derived from each glycerol stock of shRNA and ORF clones were distributed onto agar-ampicillin-positive plates, following the incubation of 18 hours in a 37°C dry incubator, a single colony was picked and grown in LB broth at 37°C and 200 rpm for 18 hours in a shaker incubator. Subsequently, the plasmids were extracted from bacteria utilizing PureLink™ HiPure Plasmid Midiprep Kit (Cat#K210004). Lentivirus particles and transduction to target cells: After reaching HEK293T cell confluency to 80% in 6 wells plate, the HEK293T cells were incubated with the lentivirus packaging plasmids and shRNA/ORF plasmids for 24 hours following the manufacturer’s protocol. After 24 hours, the media was changed to fresh media (complete RPMI 1% penicillin/streptomycin, 10% FBS and 1% Glutomax). During the 72 hours, lentiviruses were collected, filtered through a 0.45um filter, and stored at -80°C. The target leukemic cells were cultured in the presence of polybrene (8 ug/ml) and lentivirus for 24 hours; then, the targeted cells were washed with 1X PBS and grown for an additional 2 days. The transduced cells were selected with puromycin dihydrochloride (2 ug/ml) (Cat#A1113803, ThermoFisher) in two weeks. All experiments were conducted as three independent biological replicates.
On day 1, the total protein was extracted from the cell lines and protein concentration was measured with BCA assay (Cat#A55860, ThermoFisher). Separately, the 4ug of EZH2 antibody (Cat#A16846, ABclonal) was mixed with 50ul of protein G (Cat#16-266EDM Millipore). Increased volume to 500ul by adding 1X PBS and incubated overnight at 4C. On day 2, the lysate was prepared by mixing 1ml of 1X PBS with 50ul of protein G, which was pre-cleared, then centrifuged at 5000rpm for 2 minutes at 4C. The supernatant was aspirated, resuspended in 500ul of RIPA buffer, and centrifuged at 5000 rpm for 2 minutes at 4C. Then, the supernatant was collected, the protein G and antibody mixture were added, and incubated overnight at 4C. After overnight incubation on day 3, the lysate was centrifuged at 5000 rpm for 2 minutes at 4C, washed twice with cold PBS, resuspended in western blot elution buffer, and ran on SDS gel.
Cells were lysed in 1X RIPA Lysis Buffer (Cat#89900, ThermoFisher) with 1X Halt™ Protease Inhibitor Cocktail, EDTA-free (100X) (Cat#78425, ThermoFisher) for 30 min on ice. Protein concentration was measured by Pierce™ Dilution-Free™ Rapid Gold BCA Protein Assay (Cat#A55860, ThermoFisher). Protein extracts were resolved by SDS–PAGE on 4–20% Mini-PROTEAN® TGX™ Precast Protein Gels (Cat# 4561093, Bio-Rad). Post SDS-PAGE, the gels were blotted onto the membrane, 0.2 µm (Cat#1620112, Bio-Rad), and probed with the following antibodies: ARMH1 (Cat #PA5-71024, ThermoFisher), β-Actin mouse mAb (Cat #3700, Cell signaling), H3 (Cat#A2348, ABclonal), SUZ12 (A7786, AB clonal), EED (A5371, AB clonal) H3K27me3 (Cat#A2363, ABclonal), EZH2 (Cat#A16846, ABclonal), CPT1A (Cat#A5307, AB clonal), CDCA7 (Cat#A15472, ABclonal), GAPDH (Cat#14C10, cell signaling). Secondary antibodies used: anti-mouse IgG, HRP-linked Antibody (Cat#7076, Cell signaling) and Anti-rabbit IgG, HRP-linked Antibody (Cat# 7074, Cell signaling), TidyBlot secondary antibody (Cat#STAR209p, Bio-Rad). The signal was developed with SuperSignal™ West Pico PLUS Chemiluminescent Substrate (Cat#PI34578, FisherScentific), and images were detected with ChemiDoc MP instrument (Cat#12003154, Bio-Rad). We used TBST (Cat#40120065-2, BioWorld) for washing membranes. Primary antibody was diluted in 1X TBST/5%BSA, and secondary antibodies were diluted in 1X TBST/5% dry milk.
A total of 2500 cells with viability > 90% were cultured in 96-well cell culture plates in a total volume of 100ul with complete cell culture media. Following an incubation period of 72 hours (37°C, 5%CO2), 10ul of CCK-8 kit (Dojindo Cat#CK04-01) solution was added to each well. This was followed by further incubation for 3 hours at 37°C, 5% CO2, and the absorbance was measured at 450 nm in plate reader (BMG, Labteck, CLARIO Star).
The CytoSelectTM 24-Well Cell Migration Assay (3μm, Fluorometric Format; Cat# CBA-103, Cell Biolabs, Inc) was used for cell migration assay. We followed the manufacturer’s protocol, and the Relative Florescent Unit (RFU) was measured using a fluorescence plate reader (BMG, Labteck, CLARIO Star).
Cells were cultured at 2500 count per well with viability > 90% in a total volume of 50ul cell culture complete media. On the day of treatment, Cytarabine USP grade (Cat#1162002; Sigma Aldrich) was added to the medium at various concentrations (Control wells are 0 nanomolar (nM) concentration while Cytarabine treatment concentrations were 25,50,75,100,125,150,175,250,300 nM). The reaction was terminated after 72 hours by adding 100 μl/well of CellTiter-Glo® 2.0 (Cat#G9241, Promega), and cell viability was assessed by measuring the absorbance at 490nm in a 96-well plate reader (BMG, Labteck, CLARIO Star). The doses that decrease cell viability to 50% (IC50) were analyzed using a nonlinear regression log (inhibitor) vs. normalized response (three parameters) with GraphPad Prism (version 10) software.
We utilized the Seahorse XFe24 Analyzer (Agilent) to measure the OCR of live cells with more than 90% viability. A day before the assay: Turned on the Agilent Seahorse XFe/XF analyzer for warm-up overnight (minimum of five hours). Hydrate a sensor cartridge in Seahorse XF (Cat#102342-100, Agilent) calibrant at 37°C in a non-CO2 incubator overnight. On the day of assay, the first assay medium was made: Glucose (Cat#G7021-100mg, Sigma) 11mM, Sodium Pyruvate (Cat#SH3023901, Hyclone) 1mM, Glutamine (Cat#17-605E, Lonza) 2mM and XF Base medium (Cat#102353-100, Agilent) in volume of 97ml. After preparing the media,1N NaOH (Cat#3722-11, Baker) was prepared to adjust the pH of the medium to 7.4. Drug preparation: Oligomycin (Cat#495455, Sigma) 2.5uM, FCCP (Cat#C2920, Sigma) 0.5uM, Antimycin (Cat#A8674, Sigma), Rotenone (Cat#R8875, Sigma) each drug was 2uM. The Cell-Tak coated XF24 cell culture was prepared, and the assay was set up as described in the manufacturer’s protocol.
Statistical analysis of data was performed with GraphPad Prism 10 software (GraphPad Software Inc., La Jolla, CA) or with statistical functions developed using R language. All data are presented as the means ± SD or SEM. Significant differences were determined using a two-tailed student’s t-test, ANOVA test, or Wilcoxon rank-sum test.
The datasets presented in this study can be found in online repositories. The names of the repository/repositories and accession number(s) can be found in the article/Supplementary Material.
Ethical approval was not required for the studies on humans in accordance with the local legislation and institutional requirements because only commercially available established cell lines were used.
MBa: Conceptualization, Data curation, Formal analysis, Funding acquisition, Investigation, Methodology, Project administration, Resources, Software, Supervision, Validation, Visualization, Writing – original draft, Writing – review & editing. SJ: Formal analysis, Methodology, Writing – original draft. HM: Formal analysis, Methodology, Writing – original draft. RS: Methodology, Writing – review & editing. MS: Writing – review & editing. SB: Conceptualization, Data curation, Formal analysis, Funding acquisition, Investigation, Methodology, Project administration, Resources, Software, Supervision, Validation, Visualization, Writing – original draft, Writing – review & editing. MBh: Conceptualization, Data curation, Formal analysis, Funding acquisition, Investigation, Methodology, Project administration, Resources, Software, Supervision, Validation, Visualization, Writing – original draft, Writing – review & editing.
The author(s) declare financial support was received for the research, authorship, and/or publication of this article. Emory University Startup funds.
MBh serves on the board of Canomiks Inc. as chief scientific advisor and has equity in it. SB is the president of Anxomics Inc.
The remaining authors declare that the research was conducted in the absence of any commercial or financial relationships that could be construed as a potential conflict of interest.
All claims expressed in this article are solely those of the authors and do not necessarily represent those of their affiliated organizations, or those of the publisher, the editors and the reviewers. Any product that may be evaluated in this article, or claim that may be made by its manufacturer, is not guaranteed or endorsed by the publisher.
The Supplementary Material for this article can be found online at: https://www.frontiersin.org/articles/10.3389/fonc.2024.1445173/full#supplementary-material
1. Egan G, Chopra Y, Mourad S, Chiang KY, Hitzler J. Treatment of acute myeloid leukemia in children: A practical perspective. Pediatr Blood Cancer. (2021) 68:e28979. doi: 10.1002/pbc.28979
2. Chen J, Glasser CL. New and emerging targeted therapies for pediatric acute myeloid leukemia (AML). Children (Basel). (2020) 7(2):12. doi: 10.3390/children7020012
3. Medyouf H. The microenvironment in human myeloid Malignancies: emerging concepts and therapeutic implications. Blood J Am Soc Hematol. (2017) 129:1617–26. doi: 10.1182/blood-2016-11-696070
4. Bolandi SM, Pakjoo M, Beigi P, Kiani M, Allahgholipour A, Goudarzi N, et al. A role for the bone marrow microenvironment in drug resistance of acute myeloid leukemia. Cells. (2021) 10:2833. doi: 10.3390/cells10112833
5. Hofmann S, Schubert M-L, Wang L, He B, Neuber B, Dreger P, et al. Chimeric antigen receptor (CAR) T cell therapy in acute myeloid leukemia (AML). J Clin Med. (2019) 8:200. doi: 10.3390/jcm8020200
6. El Fakih R, Rasheed W, Hawsawi Y, Alsermani M, Hassanein M. Targeting FLT3 mutations in acute myeloid leukemia. Cells. (2018) 7:4. doi: 10.3390/cells7010004
7. Roboz GJ. Novel approaches to the treatment of acute myeloid leukemia. Hematol 2010 Am Soc Hematol Educ Program Book. (2011) 2011:43–50. doi: 10.1182/asheducation-2011.1.43
8. Thiede C, Steudel C, Mohr B, Schaich M, Schäkel U, Platzbecker U, et al. Analysis of FLT3-activating mutations in 979 patients with acute myelogenous leukemia: association with FAB subtypes and identification of subgroups with poor prognosis: Presented in part at the 42nd Annual Meeting of the American Society of Hematology, December 1-5, 2000, San Francisco, CA (abstract 2334). Blood J Am Soc Hematol. (2002) 99:4326–35. doi: 10.1182/blood.V99.12.4326
9. van Galen P, Hovestadt V, Wadsworth MH II, Hughes TK, Griffin GK, Battaglia S, et al. Single-cell RNA-seq reveals AML hierarchies relevant to disease progression and immunity. Cell. (2019) 176:1265–81.e24. doi: 10.1016/j.cell.2019.01.031
10. Chen J, Kao YR, Sun D, Todorova TI, Reynolds D, Narayanagari SR, et al. Myelodysplastic syndrome progression to acute myeloid leukemia at the stem cell level. Nat Med. (2019) 25:103–10. doi: 10.1038/s41591-018-0267-4
11. Stetson LC, Balasubramanian D, Ribeiro SP, Stefan T, Gupta K, Xu X, et al. Single cell RNA sequencing of AML initiating cells reveals RNA-based evolution during disease progression. Leukemia. (2021) 35:2799–812. doi: 10.1038/s41375-021-01338-7
12. Bailur JK, McCachren SS, Pendleton K, Vasquez JC, Lim HS, Duffy A, et al. Risk-associated alterations in marrow T cells in pediatric leukemia. JCI Insight. (2020) 5(16). doi: 10.1172/jci.insight.140179
13. Wu J, Xiao Y, Sun J, Sun H, Chen H, Zhu Y, et al. A single-cell survey of cellular hierarchy in acute myeloid leukemia. J Hematol Oncol. (2020) 13:128. doi: 10.1186/s13045-020-00941-y
14. Mumme H, Thomas BE, Bhasin SS, Krishnan U, Dwivedi B, Perumalla P, et al. Single-cell analysis reveals altered tumor microenvironments of relapse- and remission-associated pediatric acute myeloid leukemia. Nat Commun. (2023) 14:6209. doi: 10.1038/s41467-023-41994-0
15. Bhasin SS, Thomas BE, Summers RJ, Sarkar D, Mumme H, Pilcher W, et al. Pediatric T-cell acute lymphoblastic leukemia blast signature and MRD associated immune environment changes defined by single cell transcriptomics analysis. Sci Rep. (2023) 13:12556. doi: 10.1038/s41598-023-39152-z
16. Lambo S, Trinh DL, Ries RE, Jin D, Setiadi A, Ng M, et al. A longitudinal single-cell atlas of treatment response in pediatric AML. Cancer Cell. (2023) 41:2117–35.e12. doi: 10.1016/j.ccell.2023.10.008
17. Elsayed AH, Rafiee R, Cao X, Raimondi S, Downing JR, Ribeiro R, et al. A six-gene leukemic stem cell score identifies high risk pediatric acute myeloid leukemia. Leukemia. (2020) 34:735–45. doi: 10.1038/s41375-019-0604-8
18. Mumme HL, Raikar SS, Bhasin SS, Thomas BE, Lawrence T, Weinzierl EP, et al. Single-cell RNA sequencing distinctly characterizes the wide heterogeneity in pediatric mixed phenotype acute leukemia. Genome Med. (2023) 15:83. doi: 10.1186/s13073-023-01241-z
19. Dwivedi B, Mumme H, Satpathy S, Bhasin SS, Bhasin M. Survival Genie, a web platform for survival analysis across pediatric and adult cancers. Sci Rep. (2022) 12:3069. doi: 10.1038/s41598-022-06841-0
20. Conway JR, Lex A, Gehlenborg N. UpSetR: an R package for the visualization of intersecting sets and their properties. Bioinformatics. (2017) 33:2938–40. doi: 10.1093/bioinformatics/btx364
21. Fujita Y, Kojima T, Kawakami K, Mizutani K, Kato T, Deguchi T, et al. miR-130a activates apoptotic signaling through activation of caspase-8 in taxane-resistant prostate cancer cells. Prostate. (2015) 75:1568–78. doi: 10.1002/pros.v75.14
22. Peng Q, Ren B, Xin K, Liu W, Alam MS, Yang Y, et al. CYFIP2 serves as a prognostic biomarker and correlates with tumor immune microenvironment in human cancers. Eur J Med Res. (2023) 28:364. doi: 10.1186/s40001-023-01366-2
23. Zhang H, Zhuo C, Zhou D, Zhang F, Chen M, Xu S, et al. Association between the expression of carbonic anhydrase II and clinicopathological features of hepatocellular carcinoma. Oncol Lett. (2019) 17:5721–8. doi: 10.3892/ol.2019.10242
24. Mandic R, Marquardt A, Terhorst P, Ali U, Nowak-Rossmann A, Cai C, et al. The importin beta superfamily member RanBP17 exhibits a role in cell proliferation and is associated with improved survival of patients with HPV+ HNSCC. BMC Cancer. (2022) 22:1–18. doi: 10.1186/s12885-022-09854-0
25. Zhou M, Zhang P, Zhao Y, Liu R, Zhang Y. Overexpressed circRANBP17 acts as an oncogene to facilitate nasopharyngeal carcinoma via the miR-635/RUNX2 axis. J Cancer. (2021) 12:4322. doi: 10.7150/jca.55794
26. Tang H, Liu X, Li H, Huang H, Ran C, Wen B, et al. Comprehensive analysis reveals RANBP17 as a potential biomarker for prognosis and immunotherapy in glioblastoma. Res Sq. (2023). doi: 10.21203/rs.3.rs-2777142/v1
27. Seal RL, Braschi B, Gray K, Jones TE, Tweedie S, Haim-Vilmovsky L, et al. Genenames. org: the HGNC resources in 2023. Nucleic Acids Res. (2023) 51:D1003–9. doi: 10.1093/nar/gkac888
28. Tcheng M, Roma A, Ahmed N, Smith RW, Jayanth P, Minden MD, et al. Very long chain fatty acid metabolism is required in acute myeloid leukemia. Blood J Am Soc Hematol. (2021) 137:3518–32. doi: 10.1182/blood.2020008551
29. Lee K, Kerner J, Hoppel CL. Mitochondrial carnitine palmitoyltransferase 1a (CPT1a) is part of an outer membrane fatty acid transfer complex. J Biol Chem. (2011) 286:25655–62. doi: 10.1074/jbc.M111.228692
30. Shi J, Fu H, Jia Z, He K, Fu L, Wang W. High expression of CPT1A predicts adverse outcomes: a potential therapeutic target for acute myeloid leukemia. EBioMedicine. (2016) 14:55–64. doi: 10.1016/j.ebiom.2016.11.025
31. Ngo J, Choi DW, Stanley IA, Stiles L, Molina AJ, Chen PH, et al. Mitochondrial morphology controls fatty acid utilization by changing CPT1 sensitivity to malonyl-CoA. EMBO J. (2023) 42:e111901. doi: 10.15252/embj.2022111901
32. Joshi M, Stoykova GE, Salzmann-Sullivan M, Dzieciatkowska M, Liebman LN, Deep G, et al. CPT1A supports castration-resistant prostate cancer in androgen-deprived conditions. Cells. (2019) 8:1115. doi: 10.3390/cells8101115
33. Shao H, Mohamed EM, Xu GG, Waters M, Jing K, Ma Y, et al. Carnitine palmitoyltransferase 1A functions to repress FoxO transcription factors to allow cell cycle progression in ovarian cancer. Oncotarget. (2016) 7:3832. doi: 10.18632/oncotarget.6757
34. Lee EA, Angka L, Rota S-G, Hanlon T, Mitchell A, Hurren R, et al. Targeting mitochondria with avocatin B induces selective leukemia cell death. Cancer Res. (2015) 75:2478–88. doi: 10.1158/0008-5472.CAN-14-2676
35. Ricciardi MR, Mirabilii S, Allegretti M, Licchetta R, Calarco A, Torrisi MR, et al. Targeting the leukemia cell metabolism by the CPT1a inhibition: functional preclinical effects in leukemias. Blood J Am Soc Hematol. (2015) 126:1925–9. doi: 10.1182/blood-2014-12-617498
36. Wang H, Ye L, Xing Z, Li H, Lv T, Liu H, et al. CDCA7 promotes lung adenocarcinoma proliferation via regulating the cell cycle. Pathology-Research Pract. (2019) 215:152559. doi: 10.1016/j.prp.2019.152559
37. Yang CY, Wang L, Mu DC, Li FF, Shen H, Yang XR, et al. High expression of CDCA7 promotes cell proliferation, migration, invasion and apoptosis in non-small-cell lung cancer. J Nutr Oncol. (2020) 5:78–86. doi: 10.34175/jno202002004
38. Li S, Huang J, Qin M, Zhang J, Liao C. High expression of CDCA7 predicts tumor progression and poor prognosis in human colorectal cancer. Mol Med Rep. (2020) 22:57–66. doi: 10.3892/mmr.2020.11089
39. Cai C, Peng X, Zhang Y. Downregulation of cell division cycle-associated protein 7 (CDCA7) suppresses cell proliferation, arrests cell cycle of ovarian cancer, and restrains angiogenesis by modulating enhancer of zeste homolog 2 (EZH2) expression. Bioengineered. (2021) 12:7007–19. doi: 10.1080/21655979.2021.1965441
40. Tian Y, Huang C, Zhang H, Ni Q, Han S, Wang D, et al. CDCA7L promotes hepatocellular carcinoma progression by regulating the cell cycle. Int J Oncol. (2013) 43:2082–90. doi: 10.3892/ijo.2013.2142
41. Martín-Cortázar C, Chiodo Y, Jiménez RP, Bernabé M, Cayuela ML, Iglesias T, et al. CDCA7 finely tunes cytoskeleton dynamics to promote lymphoma migration and invasion. Haematologica. (2020) 105:730. doi: 10.3324/haematol.2018.215459
42. Osthus RC, Karim B, Prescott JE, Smith BD, McDevitt M, Huso DL, et al. The Myc target gene JPO1/CDCA7 is frequently overexpressed in human tumors and has limited transforming activity in vivo. Cancer Res. (2005) 65:5620–7. doi: 10.1158/0008-5472.CAN-05-0536
43. Prescott JE, Osthus RC, Lee LA, Lewis BC, Shim H, Barrett JF, et al. A novel c-Myc-responsive gene, JPO1, participates in neoplastic transformation. J Of Biol Chem. (2001) 276:48276–84. doi: 10.1074/jbc.M107357200
44. Harper JW, Adami GR, Wei N, Keyomarsi K, Elledge SJ. The p21 Cdk-interacting protein Cip1 is a potent inhibitor of G1 cyclin-dependent kinases. Cell. (1993) 75:805–16. doi: 10.1016/0092-8674(93)90499-G
45. Poole AJ, Heap D, Carroll RE, Tyner AL. Tumor suppressor functions for the Cdk inhibitor p21 in the mouse colon. Oncogene. (2004) 23:8128–34. doi: 10.1038/sj.onc.1207994
46. Zhang Y, Wu M-J, Lu W-C, Li Y-C, Chang CJ, Yang J-Y. Metabolic switch regulates lineage plasticity and induces synthetic lethality in triple-negative breast cancer. Cell Metab. (2024) 36:193–208.e8. doi: 10.1016/j.cmet.2023.12.003
47. Wan D, Liu C, Sun Y, Wang W, Huang K, Zheng L. MacroH2A1. 1 cooperates with EZH2 to promote adipogenesis by regulating Wnt signaling. J Mol Cell Biol. (2017) 9:325–37. doi: 10.1093/jmcb/mjx027
48. Yi SA, Um SH, Lee J, Yoo JH, Bang SY, Park EK, et al. S6K1 phosphorylation of H2B mediates EZH2 trimethylation of H3: a determinant of early adipogenesis. Mol Cell. (2016) 62:443–52. doi: 10.1016/j.molcel.2016.03.011
49. Dong Z, Li C, Yin C, Xu M, Liu S, Gao M. LncRNA PU. 1 AS regulates arsenic-induced lipid metabolism through EZH2/Sirt6/SREBP-1c pathway. J Environ Sci. (2019) 85:138–46. doi: 10.1016/j.jes.2019.05.019
50. Saramäki OR, Tammela TL, Martikainen PM, Vessella RL, Visakorpi T. The gene for polycomb group protein enhancer of zeste homolog 2 (EZH2) is amplified in late-stage prostate cancer. Genes Chromosomes Cancer. (2006) 45:639–45. doi: 10.1002/gcc.20327
51. Kleer CG, Cao Q, Varambally S, Shen R, Ota I, Tomlins SA, et al. EZH2 is a marker of aggressive breast cancer and promotes neoplastic transformation of breast epithelial cells. Proc Natl Acad Sci. (2003) 100:11606–11. doi: 10.1073/pnas.1933744100
52. Sauvageau M, Sauvageau G. Polycomb group proteins: multi-faceted regulators of somatic stem cells and cancer. Cell Stem Cell. (2010) 7:299–313. doi: 10.1016/j.stem.2010.08.002
53. Lund K, Adams P, Copland M. EZH2 in normal and Malignant hematopoiesis. Leukemia. (2014) 28:44–9. doi: 10.1038/leu.2013.288
54. Herrera-Merchan A, Arranz L, Ligos J, de Molina A, Dominguez O, Gonzalez S. Ectopic expression of the histone methyltransferase Ezh2 in haematopoietic stem cells causes myeloproliferative disease. Nat Commun. (2012) 3:623. doi: 10.1038/ncomms1623
55. Tanaka S, Miyagi S, Sashida G, Chiba T, Yuan J, Mochizuki-Kashio M, et al. Ezh2 augments leukemogenicity by reinforcing differentiation blockage in acute myeloid leukemia. Blood J Am Soc Hematol. (2012) 120:1107–17. doi: 10.1182/blood-2011-11-394932
56. Grimwade D, Walker H, Harrison G, Oliver F, Chatters S, Harrison CJ, et al. The predictive value of hierarchical cytogenetic classification in older adults with acute myeloid leukemia (AML): analysis of 1065 patients entered into the United Kingdom Medical Research Council AML11 trial. Blood J Am Soc Hematol. (2001) 98:1312–20. doi: 10.1182/blood.V98.5.1312
57. Grimwade D, Walker H, Oliver F, Wheatley K, Harrison C, Harrison G, et al. The importance of diagnostic cytogenetics on outcome in AML: analysis of 1,612 patients entered into the MRC AML 10 trial. Blood J Am Soc Hematol. (1998) 92:2322–33. doi: 10.1182/blood.V92.7.2322
58. Huang S-N, Li G-S, Zhou X-G, Chen X-Y, Yao Y-X, Zhang X-G, et al. Identification of an immune score-based gene panel with prognostic power for oral squamous cell carcinoma. Med Sci monitor. (2020) 26:e922854–1. doi: 10.12659/MSM.922854
59. Ikeda H, Kanakura Y, Tamaki T, Kuriu A, Kitayama H, Ishikawa J, et al. Expression and functional role of the proto-oncogene c-kit in acute myeloblastic leukemia cells. Blood. (1991) 78(11). doi: 10.1182/blood.V78.11.2962.bloodjournal78112962
60. Asou N. The role of a Runt domain transcription factor AML1/RUNX1 in leukemogenesis and its clinical implications. Crit Rev oncology/hematology. (2003) 45:129–50. doi: 10.1016/S1040-8428(02)00003-3
61. Lisovsky M, Estrov Z, Zhang X, Consoli U, Sanchez-Williams G, Snell V, et al. Flt3 ligand stimulates proliferation and inhibits apoptosis of acute myeloid leukemia cells: regulation of Bcl-2 and Bax. blood. (1996) 88(10). doi: 10.1182/blood.V88.10.3987.bloodjournal88103987
62. Mesbahi Y, Trahair TN, Lock RB, Connerty P. Exploring the metabolic landscape of AML: from haematopoietic stem cells to myeloblasts and leukaemic stem cells. Front Oncol. (2022) 12:807266. doi: 10.3389/fonc.2022.807266
63. Stuani L, Riols F, Millard P, Sabatier M, Batut A, Saland E, et al. Stable isotope labeling highlights enhanced fatty acid and lipid metabolism in human acute myeloid leukemia. Int J Mol Sci. (2018) 19:3325. doi: 10.3390/ijms19113325
64. Tabe Y, Saitoh K, Yang H, Sekihara K, Yamatani K, Ruvolo V, et al. Inhibition of FAO in AML co-cultured with BM adipocytes: mechanisms of survival and chemosensitization to cytarabine. Sci Rep. (2018) 8:16837. doi: 10.1038/s41598-018-35198-6
65. Wu Y, Hurren R, Maclean N, Gronda M, Jitkova Y, Sukhai MA, et al. Carnitine transporter CT2 (SLC22A16) is over-expressed in acute myeloid leukemia (AML) and target knockdown reduces growth and viability of AML cells. Apoptosis. (2015) 20:1099–108. doi: 10.1007/s10495-015-1137-x
66. Liu S, Wang Y, Miao C, Xing Q, Wang Z. High expression of CDCA7 predicts poor prognosis for clear cell renal cell carcinoma and explores its associations with immunity. Cancer Cell Int. (2021) 21:1–13. doi: 10.1186/s12935-021-01834-x
67. Zeng W, Tan H, Jamal M, Xie T, Li J, Song H, et al. Expression of CDCA7 is a prognostic biomarker and potential therapeutic target in non-small cell lung cancer. Res Sq. (2021). doi: 10.21203/rs.3.rs-170510/v1
68. Li H, Weng Y, Wang S, Wang F, Wang Y, Kong P, et al. CDCA7 facilitates tumor progression by directly regulating CCNA2 expression in esophageal squamous cell carcinoma. Front Oncol. (2021) 11:734655. doi: 10.3389/fonc.2021.734655
69. Deb G, Thakur VS, Gupta S. Multifaceted role of EZH2 in breast and prostate tumorigenesis: epigenetics and beyond. Epigenetics. (2013) 8:464–76. doi: 10.4161/epi.24532
70. Zhang Y, Yu X, Chen L, Zhang Z, Feng S. EZH2 overexpression is associated with poor prognosis in patients with glioma. Oncotarget. (2017) 8:565. doi: 10.18632/oncotarget.13478
71. Liu Y, Liu T, Bao X, He M, Li L, Yang X. Increased EZH2 expression is associated with proliferation and progression of cervical cancer and indicates a poor prognosis. Int J gynecological Pathol. (2014) 33:218–24. doi: 10.1097/PGP.0b013e31829c6574
72. Satija R, Farrell JA, Gennert D, Schier AF, Regev A. Spatial reconstruction of single-cell gene expression data. Nat Biotechnol. (2015) 33:495–502. doi: 10.1038/nbt.3192
73. Andrews S. FastQC: a quality control tool for high throughput sequence data. Cambridge, United Kingdom: Babraham Bioinformatics, Babraham Institute (2010).
74. Kim D, Paggi JM, Park C, Bennett C, Salzberg SL. Graph-based genome alignment and genotyping with HISAT2 and HISAT-genotype. Nat Biotechnol. (2019) 37:907–15. doi: 10.1038/s41587-019-0201-4
75. Anders S, Pyl PT, Huber W. HTSeq—a Python framework to work with high-throughput sequencing data. bioinformatics. (2015) 31:166–9. doi: 10.1093/bioinformatics/btu638
76. Law CW, Chen Y, Shi W, Smyth GK. voom: Precision weights unlock linear model analysis tools for RNA-seq read counts. Genome Biol. (2014) 15:1–17. doi: 10.1186/gb-2014-15-2-r29
77. Ritchie ME, Phipson B, Wu D, Hu Y, Law CW, Shi W, et al. limma powers differential expression analyses for RNA-sequencing and microarray studies. Nucleic Acids Res. (2015) 43:e47–7. doi: 10.1093/nar/gkv007
78. Hänzelmann S, Castelo R, Guinney J. GSVA: gene set variation analysis for microarray and RNA-seq data. BMC Bioinf. (2013) 14:1–15. doi: 10.1186/1471-2105-14-7
79. Liberzon A, Subramanian A, Pinchback R, Thorvaldsdóttir H, Tamayo P, Mesirov JP. Molecular signatures database (MSigDB) 3.0. Bioinformatics. (2011) 27:1739–40. doi: 10.1093/bioinformatics/btr260
80. Yu G, Wang L-G, Han Y, He Q-Y. clusterProfiler: an R package for comparing biological themes among gene clusters. Omics: J Integr Biol. (2012) 16:284–7. doi: 10.1089/omi.2011.0118
Keywords: AML, cell cycle, fatty acid metabolic pathway, EZH2, CDCA7
Citation: Bakhtiari M, Jordan SC, Mumme HL, Sharma R, Shanmugam M, Bhasin SS and Bhasin M (2024) ARMH1 is a novel marker associated with poor pediatric AML outcomes that affect the fatty acid synthesis and cell cycle pathways. Front. Oncol. 14:1445173. doi: 10.3389/fonc.2024.1445173
Received: 06 June 2024; Accepted: 04 November 2024;
Published: 05 December 2024.
Edited by:
Teresa de Souza Fernandez, National Cancer Institute (INCA), BrazilReviewed by:
Bruno Lopes, National Cancer Institute (INCA), BrazilCopyright © 2024 Bakhtiari, Jordan, Mumme, Sharma, Shanmugam, Bhasin and Bhasin. This is an open-access article distributed under the terms of the Creative Commons Attribution License (CC BY). The use, distribution or reproduction in other forums is permitted, provided the original author(s) and the copyright owner(s) are credited and that the original publication in this journal is cited, in accordance with accepted academic practice. No use, distribution or reproduction is permitted which does not comply with these terms.
*Correspondence: Manoj Bhasin, bWFub2ouYmhhc2luQGVtb3J5LmVkdQ==
Disclaimer: All claims expressed in this article are solely those of the authors and do not necessarily represent those of their affiliated organizations, or those of the publisher, the editors and the reviewers. Any product that may be evaluated in this article or claim that may be made by its manufacturer is not guaranteed or endorsed by the publisher.
Research integrity at Frontiers
Learn more about the work of our research integrity team to safeguard the quality of each article we publish.