- 1Graduate School, Changchun University of Chinese Medicine, Changchun, Jilin, China
- 2Geriatric Department, Suzhou Hospital of Integrated Traditional Chinese and Western Medicine, Suzhou, Jiangsu, China
- 3Respiratory Disease Department, Affiliated Hospital of Changchun University of Chinese Medicine, Changchun, Jilin, China
Objective: Lung cancer, the most prevalent malignancy, is typically diagnosed at an advanced stage. Smoking is a pivotal risk factor for NSCLC, yet the impact of various smoking statuses on NSCLC remains unclear. Thus, this study aims to explore whether different smoking statuses can causally influence NSCLC through effects on predictive targets, offering a novel perspective for NSCLC treatment.
Methods: Employing dual-sample MR, MVMR, and TSMR approaches, we assessed the causal relationships between 13 distinct smoking statuses and NSCLC, using predicted potential therapeutic targets as mediators to further elucidate the causal interplay among them.
Results: Among the 13 smoking statuses, current tobacco smoking, exposure to tobacco smoke outside the home, past tobacco smoking, and never smoked demonstrated causal relationships with NSCLC. MVMR analysis reveals that Current tobacco smoking is an independent risk factor for NSCLC. Utilizing NCAPD2, IL11RA, and MLC1 as mediators, IL11RA (22.2%) was found to potentially mediate the relationship between past tobacco smoking and NSCLC.
Conclusion: This study, integrating bioinformatics and MR analysis, identified three potential predictive targets as mediators to investigate the causal relationships between different smoking statuses and NSCLC through potential therapeutic targets, providing new insights for the treatment and prevention of NSCLC.
1 Introduction
Lung cancer stands as one of the principal causes of mortality among all diseases globally (1), with both its incidence and mortality rates on the rise (2). In 2022, lung cancer accounted for one-eighth of all global cancer cases, making it the leading cause of cancer-related deaths worldwide. It ranks first in incidence and mortality among men and second among women (3), with non-small cell lung cancer (NSCLC) comprising 80%-85% of all lung cancer cases (4). The primary risk factors for lung cancer include smoking, occupational exposure, air pollution, and genetic susceptibility, each of which can either individually or synergistically elevate the risk of developing lung cancer (5). Recent extensive research has focused on the genetic factors of lung adenocarcinoma, such as comparisons between the lung cancer genes of East Asian and European populations (6), potential therapeutic targets for lung cancer (7, 8), potential susceptibility to lung cancer in Asian populations (9), and the determination of lung cancer susceptibility genes in European, East Asian, and African populations (10). Additionally, rare molecular subtypes of lung cancer have been explored (11). Consequently, further research is imperative to elucidate the underlying mechanisms of lung cancer, enhance our understanding of the disease, and improve early diagnosis and prevention strategies.
The survival rate for advanced lung cancer remains low (12), and there is a definitive causal relationship between the immunological alterations caused by smoking and lung cancer (13). Over 50% of lung cancer cases occur in individuals who have previously smoked (14), with 40% occurring more than 15 years after cessation of smoking (15). With the widespread adoption of health education, an increasing number of lung cancer cases are unrelated to smoking (16). Smoking can increase the risk and mortality rate of lung cancer, establishing a clear causal link (17). However, the specific causal relationships between different smoking statuses and lung cancer remain unclear. Therefore, it is crucial to further explore how various smoking statuses influence lung cancer, which could provide new perspectives for personalized treatment strategies.
Mendelian randomization (MR) allows for the assessment of causal relationships between exposure factors and outcomes from a genetic perspective (18). Single eQTLs may present a risk of biased results and false positives (19), whereas summary data-based Mendelian Randomization (SMR) can explore the pleiotropy between gene expression levels and complex traits (20, 21). Consequently, this study predicts potential etiological and therapeutic targets for NSCLC from a bioinformatics perspective combined with MR analysis. It confirms these potential therapeutic targets through differential analysis in a validation cohort and SMR analysis. By using these potential therapeutic targets as mediators, the study explores whether different smoking statuses can influence NSCLC through the mediation of these targets. Thus, we aim to elucidate the causal relationships between different smoking statuses, potential predictive targets, and NSCLC through MR analysis, thereby offering new therapeutic perspectives for NSCLC.
2 Methods
2.1 Study design
This study initially employs two-sample MR analysis, using 13 different smoking statuses as exposures. These include Current tobacco smoking, Past tobacco smoking, Ever smoked, Exposure to tobacco smoke outside the home, and Never smoked, as the primary exposures. NSCLC serves as the outcome factor. The research aims to explore the causal relationships and underlying mechanisms between various smoking statuses and NSCLC. After adjusting for the confounding factor of BMI, Multivariable Mendelian Randomization (MVMR) analysis is conducted to ascertain which smoking status independently contributes to the risk of NSCLC. To ensure the credibility of the results, the findings of the MR analysis are validated through the Bayesian Weighted Mendelian Randomization (BWMR) method, confirming the accuracy of the causal relationships established.
Concurrently, we downloaded datasets related to “non-small cell lung cancer” from the GEO database (https://www.ncbi.nlm.nih.gov/geo/) to identify differentially expressed genes associated with NSCLC. Utilizing gene eQTLs as exposure data (https://www.eqtlgen.org/) and NSCLC as outcome data, we conducted MR analyses to identify genes causally linked to NSCLC. By intersecting these genes, we identified co-expressed genes, which were then subjected to enrichment analysis, immune cell infiltration studies, GSEA enrichment analysis, and validation group differential analysis. Additionally, SMR analysis was performed on gene eQTLs and NSCLC to verify the causal relationship of the intersected co-expressed genes.
Ultimately, we employ identified potential therapeutic targets for NSCLC as mediators, utilizing two-step MR analysis to further elucidate whether the predicted potential therapeutic targets play a significant mediating role in the causal relationship between various smoking statuses and NSCLC (Figure 1).
2.2 Data sources
The genetic information for the 13 smoking statuses and BMI is sourced from the GWAS database (https://gwas.mrcieu.ac.uk/), pertaining to a European population. The datasets for differentially expressed genes in the transcriptome are identified by the numbers GSE21933, GSE23066, GSE27262, and GSE118370, while the validation group is denoted by GSE74706. Data on NSCLC are derived from the FinnGen database (https://www.finngen.fi/en) (22), with the specific identifier finngen_R10_C3_LUNG_NONSMALL_EXALLC, also originating from a European cohort. The gene eQTLs data are obtained from peripheral blood of 31,864 European individuals (23, 24) (Table 1).
2.3 Selection of instrumental variables and mendelian randomization analysis
The selection of instrumental variables must satisfy several assumptions (25): the instrumental variables must be closely associated with different smoking statuses, independent of confounding factors in the exposure-outcome relationship, and must influence NSCLC solely through different smoking statuses (26). To ensure their relevance (27), we conduct association analyses for 13 distinct smoking statuses using a threshold of P<5×10−6, and for plasma protein eQTLs, a threshold of P<5×10−8 is applied. Concurrently, SNPs exhibiting linkage disequilibrium are eliminated using criteria of R2<0.001 and a distance of 10,000 Kb (28), followed by the calculation of the F-statistic for the selected SNPs to exclude weak instrumental variables, considering an F-value greater than 10 as indicative of the absence of weak instrumental variables (29, 30).
We employed five methods to assess the causal relationship between the variables: Inverse Variance Weighted (IVW), MR-Egger, Weighted Median, Simple Mode, and Weighted Mode methods, with IVW serving as the primary method (26, 31). A P-value less than 0.05 indicates a causal relationship (32), while the other four methods serve as supplementary approaches (33). Additionally, we utilized the BWMR method to further verify the reliability of the causal relationship. To evaluate the robustness of the causal relationship, a “leave-one-out” sensitivity analysis was conducted (34). Additionally, Cochran’s Q test and the MR-Egger intercept test were utilized to examine pleiotropy and heterogeneity (35, 36), with a P-value greater than 0.05 indicating the absence of both pleiotropy and heterogeneity.
2.4 Acquisition of differentially expressed genes and enrichment analysis
We utilized the R programming language to process data from GSE21933, GSE23066, GSE27262, and GSE118370, performing individual data corrections and merging. Using a threshold of P<0.05 and LogFC > 0.585, we filtered differentially expressed genes (DEGs) with the “limma” package, while employing “sva” and PCA to mitigate batch effects. Genes demonstrating a causal relationship between gene eQTLs and NSCLC were extracted and intersected with DEGs to identify co-expressed genes. These intersected genes were then subject to individual MR analyses, along with sensitivity, pleiotropy, and heterogeneity tests, to assess the reliability of the results.
Simultaneously, we conducted GO and KEGG enrichment analyses on the intersected genes using R, aiming to elucidate potential functional pathways and pathogenic mechanisms related to NSCLC. Additionally, GSEA enrichment analysis was employed to explore related functions within the gene expression profiles. We utilized CIBERSORT to analyze the infiltration levels of 22 types of immune cells in NSCLC (37), examining the differential expression of immune cells between two groups. Through Lasso regression, relevant immune cells associated with NSCLC were identified. By intersecting these, core immune cells were determined, further investigating the correlation between intersected genes and immune cells in NSCLC, as well as the regulatory effects of intersected genes on immune cells.
2.5 Analysis of differences in the validation group and SMR analysis
We validated the differential expression of intersected genes between the control group and the NSCLC group using data from GSE74706, comparing these findings with the results from MR analysis. Concurrently, we conducted SMR analysis using gene eQTLs data and NSCLC to further ascertain the causal relationship between the intersected genes and NSCLC (20). The SMR software (version 1.3.1) was employed for SMR analysis and HEIDI testing (21), utilizing the default settings of SMR (38). Linkage disequilibrium estimation was based on the European 1000 Genomes as a reference (39). An SMR P-value <0.05 and a HEIDI P-value >0.05 indicate a causal relationship between the exposure and the outcome.
2.6 Mediation analysis
Utilizing the TSMR method, we initially calculated the total effect (β0) of different smoking statuses on NSCLC, the effect (β1) of different smoking statuses on potential therapeutic targets, and the effect (β2) of potential therapeutic targets on NSCLC. The mediating effect was computed as β1*β2, and the direct effect was determined by subtracting the mediating effect from the total effect. The mediation proportion was calculated as (β1×β2)/β0 (40), further elucidating whether the predicted potential therapeutic targets play a significant mediating role in the causal relationship between different smoking statuses and NSCLC. All analyses were conducted using R (version 4.3.3).
3 Results
3.1 The causal relationship between 13 different smoking states and NSCLC
We conducted an association analysis on 13 different smoking statuses, removing linkage disequilibrium and weak instrumental variables, and identified 917 SNPs across these smoking statuses, with the smallest F-statistic being 20.86 and the largest 204.69. Univariate MR analysis supported a causal relationship between NSCLC and various smoking statuses, including Current tobacco smoking, Exposure to tobacco smoke outside the home, Past tobacco smoking, and Never smoked. IVW analysis results indicated a positive correlation between NSCLC and Current tobacco smoking (OR=5.218, 95% CI 2.95-9.373; P=0.000) and Exposure to tobacco smoke outside the home (OR=2.467, 95% CI 1.166-5.220; P=0.018). Conversely, a negative correlation was found with Past tobacco smoking (OR=0.722, 95% CI 0.607-0.860; P=0.000) and Never smoked (OR=0.588, 95% CI 0.348-0.995; P=0.048). Simultaneously, we employed the BWMR method to validate and further ensure the reliability of the causal relationship. Additionally, reverse MR analysis, using NSCLC as the exposure and the four different smoking statuses as outcomes, revealed no reverse causal relationships (P>0.05).
According to the results of the univariate MR analysis, current tobacco smoking, exposure to tobacco smoke outside the home, past tobacco smoking, and never having smoked all exhibit causal relationships with NSCLC. By adjusting for the confounding factor of BMI and conducting MVMR analysis, we found that the causal relationship between current tobacco smoking (OR=6.487, 95% CI 2.670-15.765; P=0.000) and NSCLC persists, affirming that current tobacco smoking is an independent risk factor for NSCLC. In contrast, past tobacco smoking, exposure to tobacco smoke outside the home, and never having smoked are not independent risk factors for NSCLC.
To evaluate the robustness of our analysis results, we employed Cochran’s Q test, the MR-Egger intercept test, and MR-PRESSO to assess pleiotropy and heterogeneity. No evidence of pleiotropy or heterogeneity was detected (P>0.05). Furthermore, a leave-one-out sensitivity analysis indicated that the exclusion of any single SNP would not significantly affect the estimates of causal relationships, suggesting that the MR analysis results are robust (Tables 2, 3, Figure 2).
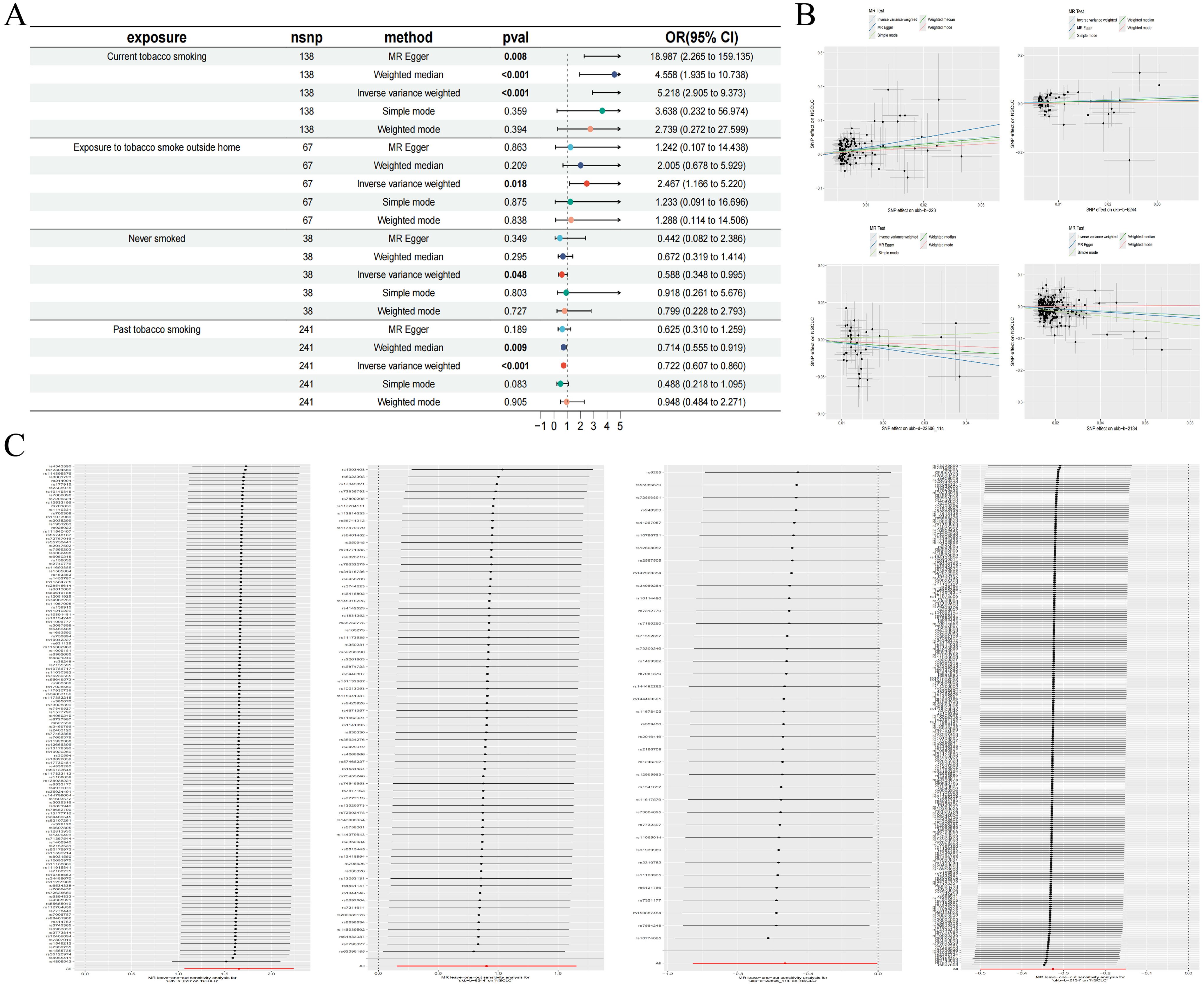
Figure 2. Forest plot of MR analysis for different smoking statuses and NSCLC (A); MR scatter plot for different smoking statuses and NSCLC (B); Results of the leave-one-out sensitivity analysis for different smoking statuses and NSCLC (C).
3.2 Acquisition of DEGs in NSCLC and MR analysis of gene eQTLs and NSCLC
We retrieved transcriptomic data from the GEO database, specifically datasets GSE21933, GSE23066, GSE27262, and GSE118370, which included samples from 57 normal lung tissues and 57 NSCLC lung tissues. Each dataset was corrected and merged to eliminate batch effects, resulting in the identification of 740 differential genes, comprising 343 upregulated and 397 downregulated genes. Concurrently, we conducted an association analysis on gene eQTLs, removing linkage disequilibrium and weak instrumental variables, and identified 19,739 SNPs associated with gene eQTLs, with the smallest F-statistic being 29.71 and the largest 14,522.86. MR analysis revealed causal relationships between 163 gene eQTLs and NSCLC, with 81 showing a negative correlation and 82 a positive correlation.
In our MR analysis, we identified 163 genes with causal relationships and intersected these with the 740 genes identified as DEGs, revealing co-expressed genes related to NSCLC. Among these, five upregulated genes were identified: CA4 (OR=1.337, 95% CI 1.005-1.779; P=0.046), ZFP28 (OR=1.355, 95% CI 1.042-1.759; P=0.023), NCAPD2 (OR=1.195, 95% CI 1.005-1.422; P=0.044), FBN2 (OR=1.225, 95% CI 1.037-1.447; P=0.017), and PI16 (OR=1.337, 95% CI 1.052-1.699; P=0.018). Additionally, three downregulated genes were identified: IL11RA (OR=0.781, 95% CI 0.650-0.937; P=0.007), RFC5 (OR=0.641, 95% CI 0.422-0.974; P=0.037), and MLC1 (OR=0.632, 95% CI 0.414-0.966; P=0.034). Simultaneously, we employed the BWMR method to validate and further ensure the reliability of the causal relationships identified. To assess the robustness of our analytical results, we employed Cochran’s Q test and the MR-Egger intercept test to examine pleiotropy and heterogeneity, detecting neither (P > 0.05). The leave-one-out analysis indicated that the exclusion of any single SNP would not significantly affect the estimates of causal association, suggesting that the MR analysis is robust (Table 4, Figure 3).
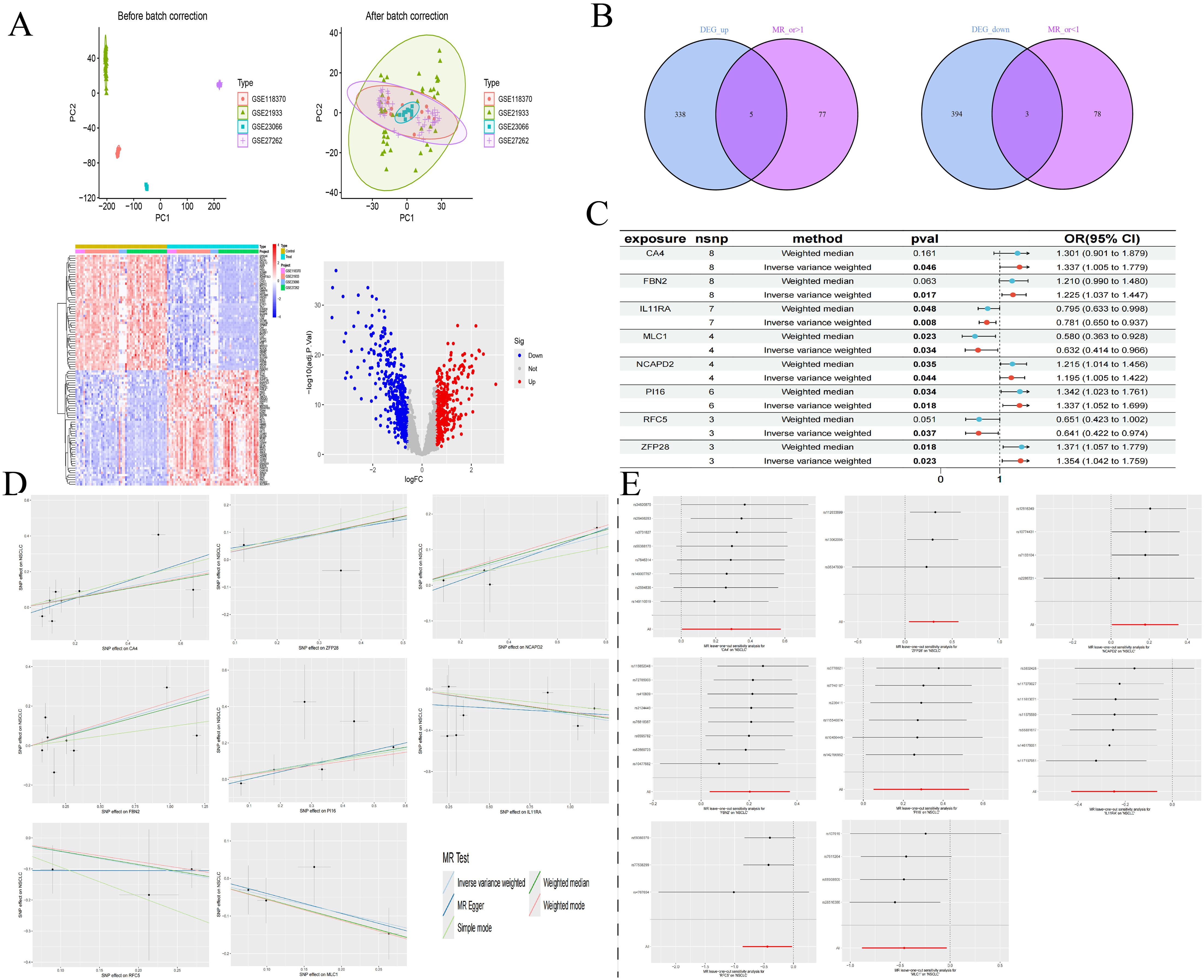
Figure 3. DEGs of NSCLC (A); Intersection of co-expressed genes (B); MR forest plot of co-expressed genes (C); MR scatter plot of co-expressed genes (D); Results of the leave-one-out sensitivity analysis of co-expressed genes (E).
3.3 Enrichment analysis and immune infiltration of co-expressed genes
We visualized the co-expressed genes to elucidate their distribution in staining. Subsequently, we conducted GO and KEGG enrichment analyses on these genes to explore their potential roles. The GO enrichment analysis revealed that the co-expressed genes predominantly affect biological functions such as cytokine receptor activity and hormone activity, through processes occurring at the extracellular side of the plasma membrane, microfibrils, plasma membrane rafts, caveolae, and involving caveolin-mediated endocytosis, extracellular regulation of signal transduction, and regulation of response to osmotic stress. The KEGG enrichment analysis focused on nucleotide excision repair, mismatch repair, DNA replication, and base excision repair. Additionally, single-gene GSEA analysis was performed on the co-expressed genes to investigate the biological functions or pathway activity levels in the high expression group. For instance, IL11RA is associated with a defense response to other organisms, innate immune response, and vesicle membrane in low expression, and with regulation of cell differentiation and metanephros development in high expression. MLC1, in high expression, is associated with circulatory system processes, multicellular organism processes, and sodium ion transport, while in low expression, it is linked to chromosome organization and chromosomal regions.
We utilized the CIBERSORT algorithm to infer the characteristics of immune cells and explore the correlation between NSCLC co-expressed genes and immune cells. Compared to the control group, differences were observed in 13 types of immune cells (P < 0.05). In NSCLC samples, the proportions of Plasma cells, T cells CD8, T cells CD4 naive, T cells regulatory (Tregs), Macrophages M0, Macrophages M1, Dendritic cells resting, and Neutrophils were elevated, whereas the proportions of T cells follicular helper, Dendritic cells activated, Monocytes, Mast cells resting, and Eosinophils were reduced. Through Lasso regression, 12 types of immune cells were selected, including Plasma cells, T cells CD8, T cells CD4 naive, T cells CD4 memory activated, T cells follicular helper, and Eosinophils. An intersection of these identified nine core immune cells: Plasma cells, T cells CD8, T cells CD4 naive, and Eosinophils, among others, further suggesting a significant correlation with NSCLC. Exploring the correlation between co-expressed genes and immune cells, it was found that ZFP28 and B cells naive were negatively correlated, as were ZFP28 and Macrophages M2; NCAPD2 was negatively correlated with Dendritic cells resting and Mast cells activated; FBN2 was negatively correlated with T cells CD4 memory activated and Monocytes; PI16 was positively correlated with Dendritic cells activated, MLC1 with T cells CD4 naive; IL11RA was positively correlated with T cells CD8 and negatively with Macrophages M0; CA4 and RFC5 showed no correlation with immune cells (Figure 4).
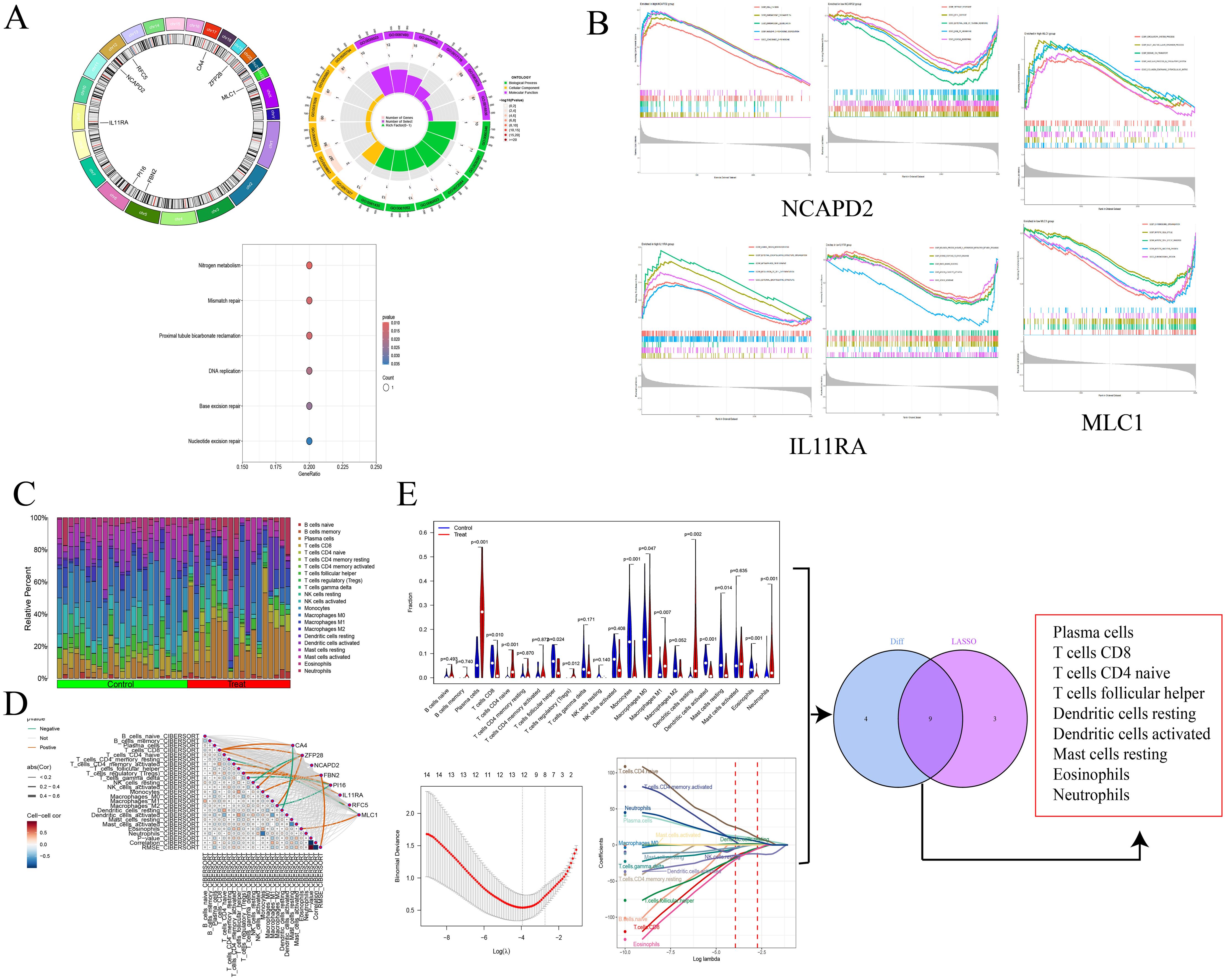
Figure 4. Circos plot and enrichment analysis of co-expressed genes (A); GAEA enrichment analysis for NCAPD2, IL11RA, and MLC1 (B); Immune infiltration analysis (C); Correlation between genes and immune cells (D); Screening of core immune cells (E).
3.4 Differential analysis in the validation cohort and SMR analysis
We further validated the co-expressed genes using data from the validation cohort GSE74706, which included 18 samples of normal lung tissue and 18 samples of NSCLC lung tissue. We observed that CA4, PI16, IL11RA, and MLC1 were highly expressed in the control group, while NCAPD2 and RFC5 were expressed at lower levels (P < 0.001). Compared to our MR analysis, the findings for IL11RA, NCAPD2, and MLC1 were consistent with the MR results, further affirming the potential of these three genes in regulating NSCLC.
We employed SMR to further ascertain the causal relationships between gene eQTLs and NSCLC, revealing that 552 genes have a causal association with NSCLC, with 287 showing a negative correlation and 265 a positive correlation. Among the eight co-expressed genes related to NSCLC, IL11RA, NCAPD2, and MLC1 passed both the SMR analysis and HEIDI tests (PSMR < 0.05 and PHEIDI > 0.05), further substantiating the potential of IL11RA, NCAPD2, and MLC1 as prospective therapeutic targets for NSCLC (Table 5, Figure 5).
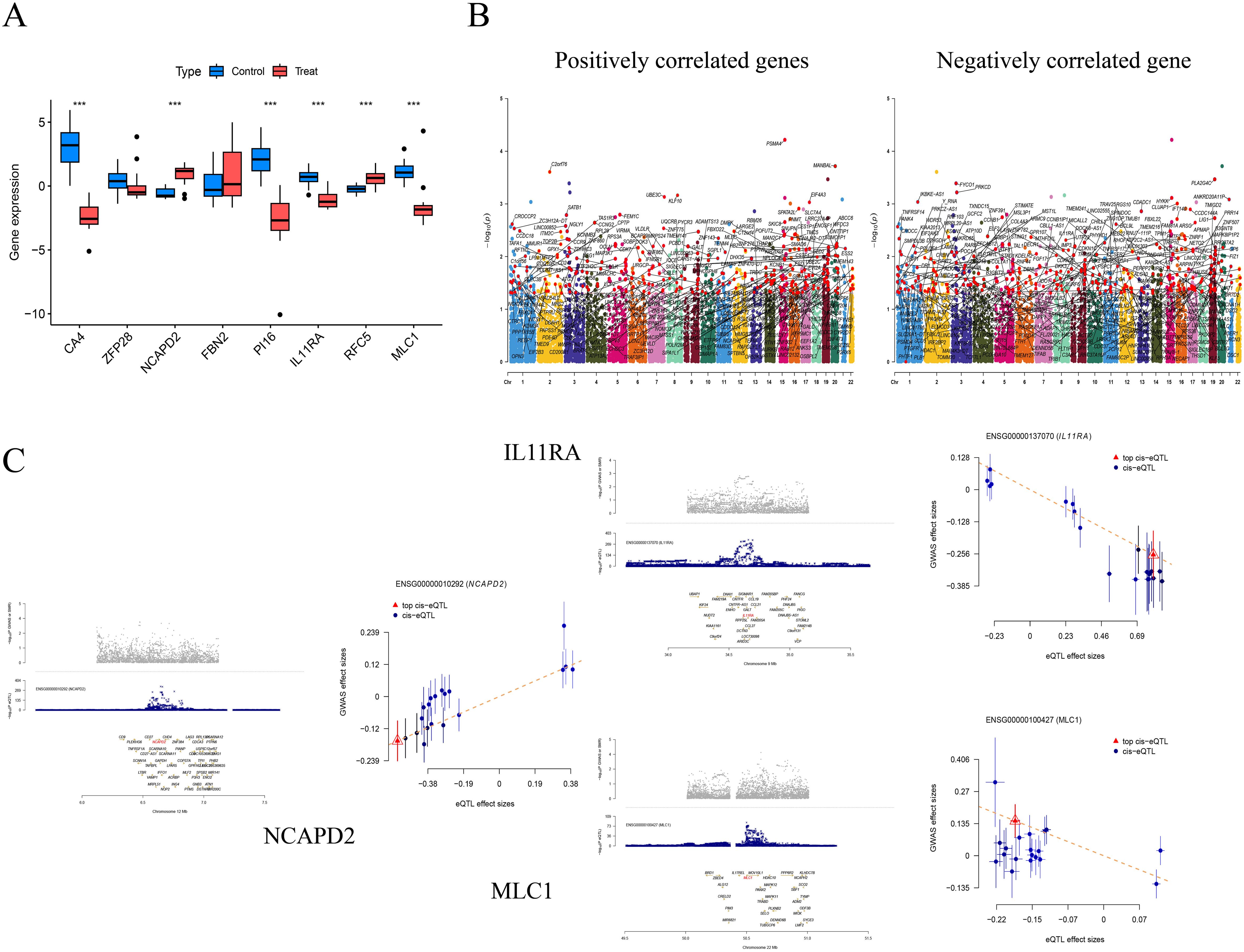
Figure 5. Differential analysis in the validation group (***P<0.001) (A); Manhattan Plots for SMR Analysis (Positive and Negative Correlations) (B); Scatter Plots of Single Genes as Potential Therapeutic Targets for NSCLC (MLC1 and IL11RA are negatively correlated, while NCAPD2 is positively correlated) (C).
3.5 Mediation analysis
We conducted a mediation analysis focusing on NCAPD2, IL11RA, and MLC1 as potential therapeutic targets for NSCLC, to further explore whether these targets mediate the impact of various smoking statuses on NSCLC. Our aim was to elucidate the proportion of the causal effects of four distinct smoking statuses on NSCLC mediated by these three predicted potential targets. We discovered that only IL11RA mediated the impact of past tobacco smoking on NSCLC, with a mediation effect of -0.253, a direct effect of -0.072, and a mediation proportion of 22.2% (Figure 6).
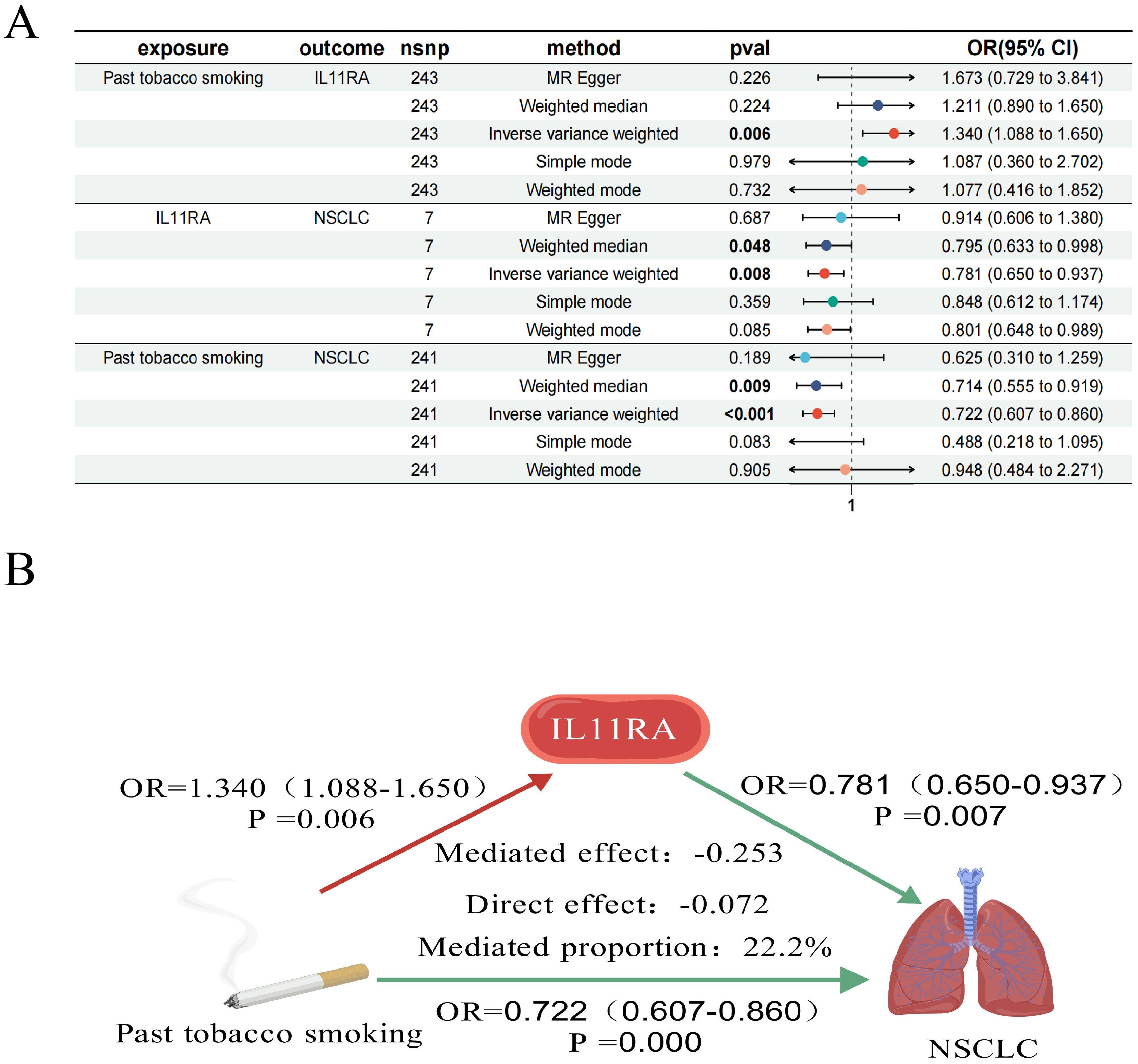
Figure 6. Forest plot of past tobacco smoking, IL11RA, and NSCLC (A); IL11RA Mediates the Causal Relationship between Past Tobacco Smoking and NSCLC (Red Indicates Risk Factors, Green Indicates Protective Factors) (B).
4 Discussion
In our study, univariate MR analysis revealed causal relationships between current tobacco smoking, exposure to tobacco smoke outside the home, past tobacco smoking, never smoked, and NSCLC. After adjusting for BMI, further MVMR analysis identified current tobacco smoking as an independent risk factor for NSCLC. Utilizing bioinformatics, MR analysis, and SMR analysis, we identified three potential therapeutic targets for NSCLC (NCAPD2, IL11RA, and MLC1). Further investigation using these three potential targets as mediators revealed that IL11RA might partially mediate the causal relationship between past tobacco smoking and NSCLC. Our findings offer a novel perspective for the treatment of NSCLC.
Smoking is the principal cause of lung cancer (41), with an increased mortality rate associated with smoking (42). Smoking drives the aggregation of pro-inflammatory macrophages (43), induces the expression of CCNA (44), accumulates M2-TAMs (45), and causes oxidative damage (46), thereby promoting the development of NSCLC from multiple angles. This aligns with our research, which genetically demonstrates that the longer the duration of current tobacco smoking, the higher the risk of NSCLC. Compared to active smoking, the mechanisms of passive smoking remain unclear (47); however, systematic reviews have found that exposure to secondhand smoke at home significantly increases the risk of lung cancer (48). Secondhand smoke affects lung function and the production of inflammatory cytokines (49), inducing inflammation and impairing immunity (50). Our genetic research confirms that exposure to tobacco smoke outside the home increases the risk of NSCLC, suggesting that encouraging family members to quit smoking and avoid secondhand smoke is one of the measures to reduce the risk of NSCLC. Numerous studies have found that quitting smoking earlier and for longer durations can reduce the mortality rate of NSCLC (51), and quitting smoking, whether before or after diagnosis, is beneficial for the survival of NSCLC patients (52, 53), enhancing overall survival (54). However, there is no significant difference in survival between smokers and non-smokers with NSCLC (55). Our MR analysis found a negative correlation between past tobacco smoking and NSCLC, consistent with most observational studies and systematic reviews. The incidence of NSCLC in never-smokers is increasing (56), with variations due to geography, environment, gender, and ethnicity (57). Our study indicates a causal relationship between never smoking and NSCLC, further emphasizing the genetic susceptibility to NSCLC.
Through bioinformatics and MR analysis, we have predicted eight genes potentially relevant for the prevention and treatment of NSCLC, with five showing positive correlations (CA4, ZFP28, NCAPD2, FBN2, and PI16) and three exhibiting negative correlations (IL11RA, RFC5, and MLC1). Cytokine receptor activity can augment the chemotherapeutic immune response in NSCLC (58), playing a synergistic role in combating tumors (59). There is a notable association between hormone activity and NSCLC (60); women receiving estrogen therapy have a lower mortality rate from lung cancer compared to men (61), and estrogen can influence the immune suppression response in NSCLC (62), inhibiting the growth of lung cancer cells through hormone activity (63). Nucleotide excision repair can fix mutated DNA bases, serving as a marker for early lung cancer cells (64). We conducted an immune infiltration analysis, identifying nine core immune cells in NSCLC through differential immune cells and Lasso regression, and explored the co-expression of genes and their correlation with immune cells. Plasma cells, which produce specific antibodies to initiate an immune response, are prognostically relevant to NSCLC (65) and contribute significantly to the efficacy of PD-1 blockade in NSCLC treatment (66). Enhancing the immune infiltration of T cells CD8 can improve the therapeutic efficacy against NSCLC (67), promoting anti-tumor activity (68). Eosinophils can predict the response and prognosis of NSCLC treatment (69) and may serve as predictive biomarkers (70). Neutrophils can be linked to new therapeutic approaches in NSCLC (71).
Further validation groups and SMR have identified three potential targets for the prevention and treatment of NSCLC: NCAPD2, IL11RA, and MLC1. NCAPD2, located on chromosome 12, regulates the structure and separation of chromosomes (72) and heterochromatin recombination (73), affecting cellular function abnormalities and playing a role in tumor development. Downregulation of NCAPD2 can inhibit tumor growth in vivo (74), promote the release of pro-inflammatory factors (75), enhance cell cycle progression and invasive capabilities (76), regulate autophagy (77), and serve as a prognostic biomarker in various cancers (78). It is often overexpressed in pan-cancer settings, associated with poor outcomes (79).MLC1, located on chromosome 22, is typically associated with the white matter of the central nervous system and is highly expressed in vascular astrocytes (80). It determines the proliferation and invasive state of brain cancer glioma cells (81). MLC1 expression is negatively correlated with tumor metastasis and has an anti-tumor effect (82). MLC1 enhances the sensitivity of cells to apoptosis, improving the effectiveness of NSCLC treatment (83).IL11RA, located on chromosome 9, initiates signaling pathways that affect cell growth, proliferation, and differentiation. IL11RA interacts with IL11 (84) and can regulate inflammatory responses, bone metabolism, and tumor development through the IL-11 signaling pathway (85). IL11RA is associated with T cells CD8 and can inhibit tumor growth (86), enhance drug resistance (87), and has potential immunomodulatory effects (88), improving survival outcomes (89). Research on the relationship between IL11RA, past tobacco smoking, and NSCLC is limited. However, through MR analysis, bioinformatics, and mediation analysis, we discovered that past tobacco smoking could exert a mediating effect on NSCLC through the mediation of IL11RA. This provides a fresh perspective for the treatment of NSCLC.
5 Conclusion
This study employs bioinformatics and MR analysis to identify three potential predictive targets, using them as mediators to explore the causal relationship between past tobacco smoking in various smoking statuses and NSCLC through the potential therapeutic target IL11RA. This approach offers a novel perspective for the treatment and prevention of NSCLC.
Data availability statement
The original contributions presented in the study are included in the article/supplementary material. Further inquiries can be directed to the corresponding authors.
Ethics statement
Ethical approval was not required for the study involving humans in accordance with the local legislation and institutional requirements. Written informed consent to participate in this study was not required from the participants or the participants’ legal guardians/next of kin in accordance with the national legislation and the institutional requirements.
Author contributions
ZC: Conceptualization, Data curation, Methodology, Writing – original draft, Writing – review & editing. SZ: Data curation, Formal analysis, Writing – original draft. TW: Data curation, Formal analysis, Software, Writing – original draft. HD: Data curation, Formal analysis, Writing – review & editing. ZT: Data curation, Validation, Writing – original draft. FS: Data curation, Writing – original draft. ZF: Data curation, Writing – original draft. SH: Conceptualization, Funding acquisition, Writing – original draft, Writing – review & editing. LS: Conceptualization, Funding acquisition, Supervision, Writing – original draft, Writing – review & editing.
Funding
The author(s) declare financial support was received for the research, authorship, and/or publication of this article. This study was funded by the Science and Technology Development Plan Project of Jilin Province (20200201608JC) and the Jilin Provincial Department of Education Science and Technology Project (JJKH20200887KJ). We gratefully acknowledge the funding of the above projects. The funders had no role in the study design, data collection and analysis, decision to publish, or preparation of the manuscript.
Acknowledgments
We want to acknowledge the participants and investigators of the FinnGen study and GWAS, as well as all the authors for their contributions to this article.
Conflict of interest
The authors declare that the research was conducted in the absence of any commercial or financial relationships that could be construed as potential conflicts of interest.
Publisher’s note
All claims expressed in this article are solely those of the authors and do not necessarily represent those of their affiliated organizations, or those of the publisher, the editors and the reviewers. Any product that may be evaluated in this article, or claim that may be made by its manufacturer, is not guaranteed or endorsed by the publisher.
References
1. Diseases GBD, Injuries C. Global burden of 369 diseases and injuries in 204 Countries and Territories, 1990-2019: a systematic analysis for the global burden of disease study 2019. Lancet. (2020) 396(10258):1204–22. doi: 10.1016/S0140-6736(20)30925-9
2. Collaborators GBDRTC. Global, regional, and national burden of respiratory tract cancers and associated risk factors from 1990 to 2019: A systematic analysis for the global burden of disease study 2019. Lancet Respir Med. (2021) 9:1030–49. doi: 10.1016/S2213-2600(21)00164-8
3. Bray F, Laversanne M, Sung H, Ferlay J, Siegel RL, Soerjomataram I, et al. Global cancer statistics 2022: globocan estimates of incidence and mortality worldwide for 36 cancers in 185 countries. CA Cancer J Clin. (2024) 74:229–63. doi: 10.3322/caac.21834
4. Jha SK, De Rubis G, Devkota SR, Zhang Y, Adhikari R, Jha LA, et al. Cellular senescence in lung cancer: molecular mechanisms and therapeutic interventions. Ageing Res Rev. (2024) 97:102315. doi: 10.1016/j.arr.2024.102315
5. Malhotra J, Malvezzi M, Negri E, La Vecchia C, Boffetta P. Risk factors for lung cancer worldwide. Eur Respir J. (2016) 48:889–902. doi: 10.1183/13993003.00359-2016
6. Shi J, Shiraishi K, Choi J, Matsuo K, Chen TY, Dai J, et al. Genome-wide association study of lung adenocarcinoma in East Asia and comparison with a european population. Nat Commun. (2023) 14:3043. doi: 10.1038/s41467-023-38196-z
7. Zhang L, Xiong Y, Zhang J, Feng Y, Xu A. Systematic proteome-wide mendelian randomization using the human plasma proteome to identify therapeutic targets for lung adenocarcinoma. J Transl Med. (2024) 22:330. doi: 10.1186/s12967-024-04919-z
8. Li H, Du S, Dai J, Jiang Y, Li Z, Fan Q, et al. Proteome-wide mendelian randomization identifies causal plasma proteins in lung cancer. iScience. (2024) 27:108985. doi: 10.1016/j.isci.2024.108985
9. Fan J, Zhu M, Wang Y, Li Z, Zhang J, Wang L, et al. Genome-wide analysis of expression quantitative trait loci identified potential lung cancer susceptibility variants among asian populations. Carcinogenesis. (2019) 40:263–8. doi: 10.1093/carcin/bgy165
10. Byun J, Han Y, Li Y, Xia J, Long E, Choi J, et al. Cross-ancestry genome-wide meta-analysis of 61,047 cases and 947,237 controls identifies new susceptibility loci contributing to lung cancer. Nat Genet. (2022) 54:1167–77. doi: 10.1038/s41588-022-01115-x
11. Harada G, Yang SR, Cocco E, Drilon A. Rare molecular subtypes of lung cancer. Nat Rev Clin Oncol. (2023) 20:229–49. doi: 10.1038/s41571-023-00733-6
12. Wang X, Zhang Z, Ding Y, Chen T, Mucci L, Albanes D, et al. Impact of individual level uncertainty of lung cancer polygenic risk score (Prs) on risk stratification. Genome Med. (2024) 16:22. doi: 10.1186/s13073-024-01298-4
13. de Biase MS, Massip F, Wei TT, Giorgi FM, Stark R, Stone A, et al. Smoking-associated gene expression alterations in nasal epithelium reveal immune impairment linked to lung cancer risk. Genome Med. (2024) 16:54. doi: 10.1186/s13073-024-01317-4
14. Siegel RL, Miller KD, Jemal A. Cancer statistics, 2020. CA Cancer J Clin. (2020) 70:7–30. doi: 10.3322/caac.21590
15. Tindle HA, Stevenson Duncan M, Greevy RA, Vasan RS, Kundu S, Massion PP, et al. Lifetime smoking history and risk of lung cancer: results from the framingham heart study. J Natl Cancer Inst. (2018) 110:1201–7. doi: 10.1093/jnci/djy041
16. Shiels MS, Graubard BI, McNeel TS, Kahle L, Freedman ND. Trends in smoking-attributable and smoking-unrelated lung cancer death rates in the United States, 1991-2018. J Natl Cancer Inst. (2024) 116:711–6. doi: 10.1093/jnci/djad256
17. Went M, Sud A, Mills C, Hyde A, Culliford R, Law P, et al. Phenome-wide mendelian randomisation analysis of 378,142 cases reveals risk factors for eight common cancers. Nat Commun. (2024) 15:2637. doi: 10.1038/s41467-024-46927-z
18. Bowden J, Holmes MV. Meta-analysis and mendelian randomization: A review. Res Synth Methods. (2019) 10:486–96. doi: 10.1002/jrsm.1346
19. Li N, Yi H, Sun W, Sundquist J, Sundquist K, Zhang X, et al. Revealing genes associated with cervical cancer in distinct immune cells: A comprehensive mendelian randomization analysis. Int J Cancer. (2024) 155:149–58. doi: 10.1002/ijc.34911
20. Zhu Z, Zhang F, Hu H, Bakshi A, Robinson MR, Powell JE, et al. Integration of summary data from gwas and eqtl studies predicts complex trait gene targets. Nat Genet. (2016) 48:481–7. doi: 10.1038/ng.3538
21. Wu Y, Zeng J, Zhang F, Zhu Z, Qi T, Zheng Z, et al. Integrative analysis of omics summary data reveals putative mechanisms underlying complex traits. Nat Commun. (2018) 9:918. doi: 10.1038/s41467-018-03371-0
22. Kurki MI, Karjalainen J, Palta P, Sipila TP, Kristiansson K, Donner KM, et al. Finngen provides genetic insights from a well-phenotyped isolated population. Nature. (2023) 613:508–18. doi: 10.1038/s41586-022-05473-8
23. Vosa U, Claringbould A, Westra HJ, Bonder MJ, Deelen P, Zeng B, et al. Large-scale cis- and trans-eqtl analyses identify thousands of genetic loci and polygenic scores that regulate blood gene expression. Nat Genet. (2021) 53:1300–10. doi: 10.1038/s41588-021-00913-z
24. Sun BB, Maranville JC, Peters JE, Stacey D, Staley JR, Blackshaw J, et al. Genomic atlas of the human plasma proteome. Nature. (2018) 558:73–9. doi: 10.1038/s41586-018-0175-2
25. Burgess S, Scott RA, Timpson NJ, Davey Smith G, Thompson SG, Consortium E-I. Using published data in mendelian randomization: A blueprint for efficient identification of causal risk factors. Eur J Epidemiol. (2015) 30:543–52. doi: 10.1007/s10654-015-0011-z
26. Davies NM, Holmes MV, Davey Smith G. Reading mendelian randomisation studies: A guide, glossary, and checklist for clinicians. BMJ. (2018) 362:k601. doi: 10.1136/bmj.k601
27. He M, Xu C, Yang R, Liu L, Zhou D, Yan S. Causal relationship between human blood metabolites and risk of ischemic stroke: A mendelian randomization study. Front Genet. (2024) 15:1333454. doi: 10.3389/fgene.2024.1333454
28. Yuan J, Xiong X, Zhang B, Feng Q, Zhang J, Wang W, et al. Genetically predicted C-reactive protein mediates the association between rheumatoid arthritis and atlantoaxial subluxation. Front Endocrinol (Lausanne). (2022) 13:1054206. doi: 10.3389/fendo.2022.1054206
29. Choi KW, Chen CY, Stein MB, Klimentidis YC, Wang MJ, Koenen KC, et al. Assessment of bidirectional relationships between physical activity and depression among adults: A 2-sample mendelian randomization study. JAMA Psychiatry. (2019) 76:399–408. doi: 10.1001/jamapsychiatry.2018.4175
30. Cheng ZX, Hua JL, Jie ZJ, Li XJ, Zhang J. Genetic insights into the gut-lung axis: mendelian randomization analysis on gut microbiota, lung function, and copd. Int J Chron Obstruct Pulmon Dis. (2024) 19:643–53. doi: 10.2147/COPD.S441242
31. Pierce BL, Burgess S. Efficient design for mendelian randomization studies: subsample and 2-sample instrumental variable estimators. Am J Epidemiol. (2013) 178:1177–84. doi: 10.1093/aje/kwt084
32. Sanderson E. Multivariable mendelian randomization and mediation. Cold Spring Harb Perspect Med. (2021) 11. doi: 10.1101/cshperspect.a038984
33. Burgess S, Thompson SG. Erratum to: interpreting findings from mendelian randomization using the mr-egger method. Eur J Epidemiol. (2017) 32:391–2. doi: 10.1007/s10654-017-0276-5
34. Burgess S, Bowden J, Fall T, Ingelsson E, Thompson SG. Sensitivity analyses for robust causal inference from mendelian randomization analyses with multiple genetic variants. Epidemiology. (2017) 28:30–42. doi: 10.1097/EDE.0000000000000559
35. Bowden J, Davey Smith G, Burgess S. Mendelian randomization with invalid instruments: effect estimation and bias detection through egger regression. Int J Epidemiol. (2015) 44:512–25. doi: 10.1093/ije/dyv080
36. Verbanck M, Chen CY, Neale B, Do R. Detection of widespread horizontal pleiotropy in causal relationships inferred from mendelian randomization between complex traits and diseases. Nat Genet. (2018) 50:693–8. doi: 10.1038/s41588-018-0099-7
37. Chen B, Khodadoust MS, Liu CL, Newman AM, Alizadeh AA. Profiling tumor infiltrating immune cells with cibersort. Methods Mol Biol. (2018) 1711:243–59. doi: 10.1007/978-1-4939-7493-1_12
38. Liu D, Yang J, Feng B, Lu W, Zhao C, Li L. Mendelian randomization analysis identified genes pleiotropically associated with the risk and prognosis of Covid-19. J Infect. (2021) 82:126–32. doi: 10.1016/j.jinf.2020.11.031
39. Zheng-Bradley X, Flicek P. Applications of the 1000 genomes project resources. Brief Funct Genomics. (2017) 16:163–70. doi: 10.1093/bfgp/elw027
40. Carter AR, Sanderson E, Hammerton G, Richmond RC, Davey Smith G, Heron J, et al. Mendelian randomisation for mediation analysis: current methods and challenges for implementation. Eur J Epidemiol. (2021) 36:465–78. doi: 10.1007/s10654-021-00757-1
41. Schabath MB, Cote ML. Cancer progress and priorities: lung cancer. Cancer Epidemiol Biomarkers Prev. (2019) 28:1563–79. doi: 10.1158/1055-9965.EPI-19-0221
42. Dai X, Gil GF, Reitsma MB, Ahmad NS, Anderson JA, Bisignano C, et al. Health effects associated with smoking: A burden of proof study. Nat Med. (2022) 28:2045–55. doi: 10.1038/s41591-022-01978-x
43. Krishna C, Tervi A, Saffern M, Wilson EA, Yoo SK, Mars N, et al. An immunogenetic basis for lung cancer risk. Science. (2024) 383:eadi3808. doi: 10.1126/science.adi3808
44. He Q, Qu M, Xu C, Wu L, Xu Y, Su J, et al. Smoking-induced ccna2 expression promotes lung adenocarcinoma tumorigenesis by boosting at2/at2-like cell differentiation. Cancer Lett. (2024) 592:216922. doi: 10.1016/j.canlet.2024.216922
45. Cheng C, Wang P, Yang Y, Du X, Xia H, Liu J, et al. Smoking-induced M2-tams, via circeml4 in evs, promote the progression of nsclc through alkbh5-regulated M6a modification of socs2 in nsclc cells. Adv Sci (Weinh). (2023) 10:e2300953. doi: 10.1002/advs.202300953
46. Franci L, Vallini G, Bertolino FM, Cicaloni V, Inzalaco G, Cicogni M, et al. Mapk15 controls cellular responses to oxidative stress by regulating nrf2 activity and expression of its downstream target genes. Redox Biol. (2024) 72:103131. doi: 10.1016/j.redox.2024.103131
47. Mochizuki A, Shiraishi K, Honda T, Higashiyama RI, Sunami K, Matsuda M, et al. Passive smoking-induced mutagenesis as a promoter of lung carcinogenesis. J Thorac Oncol. (2024). doi: 10.1016/j.jtho.2024.02.006
48. Hori M, Tanaka H, Wakai K, Sasazuki S, Katanoda K. Secondhand smoke exposure and risk of lung cancer in Japan: A systematic review and meta-analysis of epidemiologic studies. Jpn J Clin Oncol. (2016) 46:942–51. doi: 10.1093/jjco/hyw091
49. Flouris AD, Metsios GS, Carrillo AE, Jamurtas AZ, Gourgoulianis K, Kiropoulos T, et al. Acute and short-term effects of secondhand smoke on lung function and cytokine production. Am J Respir Crit Care Med. (2009) 179:1029–33. doi: 10.1164/rccm.200812-1920OC
50. Bhat TA, Kalathil SG, Bogner PN, Miller A, Lehmann PV, Thatcher TH, et al. Secondhand smoke induces inflammation and impairs immunity to respiratory infections. J Immunol. (2018) 200:2927–40. doi: 10.4049/jimmunol.1701417
51. Lai H, Liu Q, Ye Q, Liang Z, Long Z, Hu Y, et al. Impact of smoking cessation duration on lung cancer mortality: A systematic review and meta-analysis. Crit Rev Oncol Hematol. (2024) 196:104323. doi: 10.1016/j.critrevonc.2024.104323
52. Caini S, Del Riccio M, Vettori V, Scotti V, Martinoli C, Raimondi S, et al. Quitting Smoking at or around Diagnosis Improves the Overall Survival of Lung Cancer Patients: A Systematic Review and Meta-Analysis. J Thorac Oncol. (2022) 17:623–36. doi: 10.1016/j.jtho.2021.12.005
53. Wang X, Romero-Gutierrez CW, Kothari J, Shafer A, Li Y, Christiani DC. Prediagnosis smoking cessation and overall survival among patients with non-small cell lung cancer. JAMA Netw Open. (2023) 6:e2311966. doi: 10.1001/jamanetworkopen.2023.11966
54. Fares AF, Li Y, Jiang M, Brown MC, Lam ACL, Aggarwal R, et al. Association between duration of smoking abstinence before non-small-cell lung cancer diagnosis and survival: A retrospective, pooled analysis of cohort studies. Lancet Public Health. (2023) 8:e691–700. doi: 10.1016/S2468-2667(23)00131-7
55. Steuer CE, Jegede OA, Dahlberg SE, Wakelee HA, Keller SM, Tester WJ, et al. Smoking behavior in patients with early-stage nsclc: A report from ecog-acrin 1505 trial. J Thorac Oncol. (2021) 16:960–7. doi: 10.1016/j.jtho.2020.12.017
56. Pelosof L, Ahn C, Gao A, Horn L, Madrigales A, Cox J, et al. Proportion of never-smoker non-small cell lung cancer patients at three diverse institutions. J Natl Cancer Inst. (2017) 109. doi: 10.1093/jnci/djw295
57. LoPiccolo J, Gusev A, Christiani DC, Janne PA. Lung cancer in patients who have never smoked - an emerging disease. Nat Rev Clin Oncol. (2024) 21:121–46. doi: 10.1038/s41571-023-00844-0
58. Yi L, Xu Z, Ma T, Wang C, Wei P, Xiao B, et al. T-cell subsets and cytokines are indicative of neoadjuvant chemoimmunotherapy responses in nsclc. Cancer Immunol Immunother. (2024) 73:99. doi: 10.1007/s00262-024-03687-5
59. Li Y, Sharma A, Hoffmann MJ, Skowasch D, Essler M, Weiher H, et al. Discovering single cannabidiol or synergistic antitumor effects of cannabidiol and cytokine-induced killer cells on non-small cell lung cancer cells. Front Immunol. (2024) 15:1268652. doi: 10.3389/fimmu.2024.1268652
60. Chen J, Zhao R, Wang Y, Xiao H, Lin W, Diao M, et al. G protein-coupled estrogen receptor activates pi3k/akt/mtor signaling to suppress ferroptosis via srebp1/scd1-mediated lipogenesis. Mol Med. (2024) 30:28. doi: 10.1186/s10020-023-00763-x
61. Bouchardy C, Benhamou S, Schaffar R, Verkooijen HM, Fioretta G, Schubert H, et al. Lung cancer mortality risk among breast cancer patients treated with anti-estrogens. Cancer. (2011) 117:1288–95. doi: 10.1002/cncr.25638
62. Anobile DP, Salaroglio IC, Tabbo F, La Vecchia S, Akman M, Napoli F, et al. Autocrine 17-beta-estradiol/estrogen receptor-alpha loop determines the response to immune checkpoint inhibitors in non-small cell lung cancer. Clin Cancer Res. (2023) 29:3958–73. doi: 10.1158/1078-0432.CCR-22-3949
63. Gao D, Li Y, Xiang S, Zhang J. Key targets and molecular mechanisms of the fat-soluble components of ginseng for lung cancer treatment. Appl Biochem Biotechnol. (2023) 195:6495–515. doi: 10.1007/s12010-023-04409-w
64. Limpose KL, Trego KS, Li Z, Leung SW, Sarker AH, Shah JA, et al. Overexpression of the base excision repair nthl1 glycosylase causes genomic instability and early cellular hallmarks of cancer. Nucleic Acids Res. (2018) 46:4515–32. doi: 10.1093/nar/gky162
65. Edlund K, Madjar K, Mattsson JSM, Djureinovic D, Lindskog C, Brunnstrom H, et al. Prognostic impact of tumor cell programmed death ligand 1 expression and immune cell infiltration in nsclc. J Thorac Oncol. (2019) 14:628–40. doi: 10.1016/j.jtho.2018.12.022
66. Patil NS, Nabet BY, Muller S, Koeppen H, Zou W, Giltnane J, et al. Intratumoral plasma cells predict outcomes to pd-L1 blockade in non-small cell lung cancer. Cancer Cell. (2022) 40:289–300.e4. doi: 10.1016/j.ccell.2022.02.002
67. Luo J, Cheng K, Ji X, Gao C, Zhu R, Chen J, et al. Anlotinib enhanced cd8(+) T cell infiltration via induction of ccl5 improves the efficacy of pd-1/pd-L1 blockade therapy in lung cancer. Cancer Lett. (2024) 591:216892. doi: 10.1016/j.canlet.2024.216892
68. Hao Z, Xin Z, Chen Y, Shao Z, Lin W, Wu W, et al. Jaml promotes the antitumor role of tumor-resident cd8(+) T cells by facilitating their innate-like function in human lung cancer. Cancer Lett. (2024) 590:216839. doi: 10.1016/j.canlet.2024.216839
69. Huai Q, Luo C, Song P, Bie F, Bai G, Li Y, et al. Peripheral blood inflammatory biomarkers dynamics reflect treatment response and predict prognosis in non-small cell lung cancer patients with neoadjuvant immunotherapy. Cancer Sci. (2023) 114:4484–98. doi: 10.1111/cas.15964
70. Takeuchi E, Ogino H, Kondo K, Okano Y, Ichihara S, Kunishige M, et al. An increased relative eosinophil count as a predictive dynamic biomarker in non-small cell lung cancer patients treated with immune checkpoint inhibitors. Thorac Cancer. (2024) 15:248–57. doi: 10.1111/1759-7714.15191
71. Takeuchi E, Kondo K, Okano Y, Ichihara S, Kunishige M, Kadota N, et al. Pretreatment eosinophil counts as a predictive biomarker in non-small cell lung cancer patients treated with immune checkpoint inhibitors. Thorac Cancer. (2023) 14:3042–50. doi: 10.1111/1759-7714.15100
72. Ball AR Jr., Schmiesing JA, Zhou C, Gregson HC, Okada Y, Doi T, et al. Identification of a chromosome-targeting domain in the human condensin subunit cnap1/hcap-D2/eg7. Mol Cell Biol. (2002) 22:5769–81. doi: 10.1128/MCB.22.16.5769-5781.2002
73. Huang B, Wang B, Yuk-Wai Lee W, Pong UK, Leung KT, Li X, et al. Kdm3a and kdm4c regulate mesenchymal stromal cell senescence and bone aging via condensin-mediated heterochromatin reorganization. iScience. (2019) 21:375–90. doi: 10.1016/j.isci.2019.10.041
74. He J, Gao R, Yang J, Li F, Fu Y, Cui J, et al. Ncapd2 promotes breast cancer progression through E2f1 transcriptional regulation of cdk1. Cancer Sci. (2023) 114:896–907. doi: 10.1111/cas.15347
75. Yuan CW, Sun XL, Qiao LC, Xu HX, Zhu P, Chen HJ, et al. Non-smc condensin I complex subunit D2 and non-smc condensin ii complex subunit D3 induces inflammation via the ikk/nf-kappab pathway in ulcerative colitis. World J Gastroenterol. (2019) 25:6813–22. doi: 10.3748/wjg.v25.i47.6813
76. Zhang Y, Liu F, Zhang C, Ren M, Kuang M, Xiao T, et al. Non-smc condensin I complex subunit D2 is a prognostic factor in triple-negative breast cancer for the ability to promote cell cycle and enhance invasion. Am J Pathol. (2020) 190:37–47. doi: 10.1016/j.ajpath.2019.09.014
77. Jing Z, He X, Jia Z, Sa Y, Yang B, Liu P. Ncapd2 inhibits autophagy by regulating ca(2+)/camkk2/ampk/mtorc1 pathway and parp-1/sirt1 axis to promote colorectal cancer. Cancer Lett. (2021) 520:26–37. doi: 10.1016/j.canlet.2021.06.029
78. Dong X, Liu T, Li Z, Zhai Y. Non-smc condensin I complex subunit D2 (Ncapd2) reveals its prognostic and immunologic features in human cancers. Aging (Albany NY). (2023) 15:7237–57. doi: 10.18632/aging.204904
79. Feng L, Yang Y, Lin Z, Cui M, Jin A, Cui A. Correction: ncapd2 is a favorable predictor of prognostic and immunotherapeutic biomarker for multiple cancer types including lung cancer. Genes Environ. (2024) 46:6. doi: 10.1186/s41021-024-00301-z
80. Brignone MS, Lanciotti A, Michelucci A, Mallozzi C, Camerini S, Catacuzzeno L, et al. The camkii/mlc1 axis confers ca(2+)-dependence to volume-regulated anion channels (Vrac) in astrocytes. Cells. (2022) 11. doi: 10.3390/cells11172656
81. De A, Lattier JM, Morales JE, Kelly JR, Zheng X, Chen Z, et al. Glial cell adhesion molecule (Glialcam) determines proliferative versus invasive cell states in glioblastoma. J Neurosci. (2023) 43:8043–57. doi: 10.1523/JNEUROSCI.1401-23.2023
82. Wu Z, Ni J, Zhang H, Zhang Y, Lv C, Wang Y, et al. Mlc1 overexpression inhibits tumor progression through pi3k/akt signal pathway in prostate cancer. Adv Biol (Weinh). (2024) 8:e2300060. doi: 10.1002/adbi.202300060
83. Shen Q, Li J, Mai J, Zhang Z, Fisher A, Wu X, et al. Sensitizing non-small cell lung cancer to bcl-xl-targeted apoptosis. Cell Death Dis. (2018) 9:986. doi: 10.1038/s41419-018-1040-9
84. Metcalfe RD, Aizel K, Zlatic CO, Nguyen PM, Morton CJ, Lio DS, et al. The structure of the extracellular domains of human interleukin 11alpha receptor reveals mechanisms of cytokine engagement. J Biol Chem. (2020) 295:8285–301. doi: 10.1074/jbc.RA119.012351
85. Eun YG, Shin IH, Kim MJ, Chung JH, Song JY, Kwon KH. Associations between promoter polymorphism -106a/G of interleukin-11 receptor alpha and papillary thyroid cancer in Korean population. Surgery. (2012) 151:323–9. doi: 10.1016/j.surg.2011.07.014
86. Winship A, Van Sinderen M, Rainczuk K, Dimitriadis E. Therapeutically blocking interleukin-11 receptor-alpha enhances doxorubicin cytotoxicity in high grade type I endometrioid tumours. Oncotarget. (2017) 8:22716–29. doi: 10.18632/oncotarget.15187
87. Cheng B, Li L, Luo T, Wang Q, Luo Y, Bai S, et al. Single-cell deconvolution algorithms analysis unveils autocrine il11-mediated resistance to docetaxel in prostate cancer via activation of the jak1/stat4 pathway. J Exp Clin Cancer Res. (2024) 43:67. doi: 10.1186/s13046-024-02962-8
88. Huynh J, Baloyan D, Chisanga D, Shi W, O'Brien M, Afshar-Sterle S, et al. Host il11 signaling suppresses cd4(+) T cell-mediated antitumor responses to colon cancer in mice. Cancer Immunol Res. (2021) 9:735–47. doi: 10.1158/2326-6066.CIR-19-1023
Keywords: different smoking statuses, NSCLC, Mendelian randomization, bioinformatics, mediation analysis
Citation: Cao Z, Zhao S, Wu T, Ding H, Tian Z, Sun F, Feng Z, Hu S and Shi L (2024) The causal nexus between diverse smoking statuses, potential therapeutic targets, and NSCLC: insights from Mendelian randomization and mediation analysis. Front. Oncol. 14:1438851. doi: 10.3389/fonc.2024.1438851
Received: 26 May 2024; Accepted: 17 October 2024;
Published: 04 November 2024.
Edited by:
Panagiota Economopoulou, University General Hospital Attikon, GreeceCopyright © 2024 Cao, Zhao, Wu, Ding, Tian, Sun, Feng, Hu and Shi. This is an open-access article distributed under the terms of the Creative Commons Attribution License (CC BY). The use, distribution or reproduction in other forums is permitted, provided the original author(s) and the copyright owner(s) are credited and that the original publication in this journal is cited, in accordance with accepted academic practice. No use, distribution or reproduction is permitted which does not comply with these terms.
*Correspondence: Li Shi, c2hpbGkwNjQ4QDE2My5jb20=; Shaodan Hu, NzM0MTY4MzAwQHFxLmNvbQ==