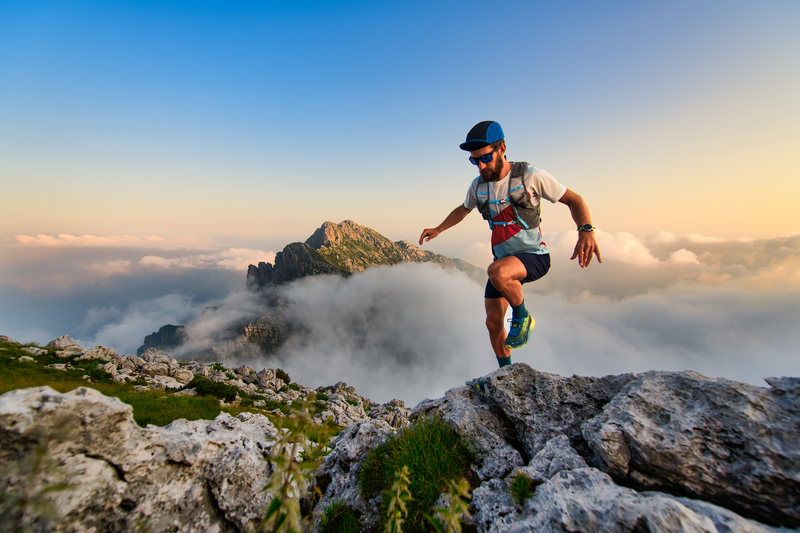
94% of researchers rate our articles as excellent or good
Learn more about the work of our research integrity team to safeguard the quality of each article we publish.
Find out more
ORIGINAL RESEARCH article
Front. Oncol. , 29 November 2024
Sec. Cancer Imaging and Image-directed Interventions
Volume 14 - 2024 | https://doi.org/10.3389/fonc.2024.1395502
Quantitative dynamic contrast-enhanced (DCE) MRI as a promising method for the prediction of breast cancer response to neoadjuvant chemotherapy (NAC) has been demonstrated mostly in single-center and single-vendor platform studies. This preliminary study reports the initial experience in implementing quantitative breast DCE-MRI in multi-center (MC) and multi-vendor platform (MP) settings to predict NAC response. MRI data, including B1 mapping, variable flip angle (VFA) measurements of native tissue R1 (R1,0), and DCE-MRI, were acquired during NAC at three sites using 3T systems with Siemens, Philips, and GE platforms, respectively. High spatiotemporal resolution DCE-MRI was performed using similar vendor product sequences with k-space undersampling during acquisition and view sharing during reconstruction. A breast phantom was used for quality assurance/quality control (QA/QC) across sites. The Tofts model (TM) and shutter-speed model (SSM) were used for pharmacokinetic (PK) analysis of the DCE data. Additionally, tumor region of interest (ROI)- vs. voxel-based analyses in combination with the use of VFA-measured R1,0 vs. fixed, literature-reported R1,0 were investigated to determine the optimal analysis approach. Results from 15 patients who completed the study are reported. Voxel-based PK analysis using fixed R1,0 was deemed the optimal approach, which allowed the inclusion of data from one vendor platform where VFA measurements produced ≥100% overestimation of R1,0. The semi-quantitative signal enhancement ratio (SER) and quantitative PK parameters outperformed the tumor longest diameter (LD) in the prediction of pathologic complete response (pCR) vs. non-pCR after the first NAC cycle, whereas Ktrans consistently provided more accurate predictions than both SER and LD after the first NAC cycle and at the NAC midpoint. Both TM and SSM Ktrans and kep were excellent predictors of response at the NAC midpoint with ROC AUC >0.90, while the SSM parameters (AUC ≥0.80) performed better than their TM counterparts (AUC <0.80) after the first NAC cycle. The initial experience of this ongoing study indicates the importance of QA/QC using a phantom and suggests that deploying voxel-based PK analysis using a fixed R1,0 may mitigate random errors from R1,0 measurements across platforms and potentially eliminate the need for B1 and VFA acquisitions in MC and MP trials.
Neoadjuvant chemotherapy (NAC) is frequently used in the standard of care (SoC) to treat patients with locally advanced breast cancer to downstage the disease and facilitate breast-conserving surgery. In addition, the setting of NAC systemic treatment affords the opportunity to assess the pathologic response to NAC. Studies have shown that pathologic complete response (pCR) or minimal residual disease following NAC is prognostic for survival (1–6). However, since assessment of pathologic response can only be ascertained from surgical tumor specimens after NAC has already been completed, the treating clinician’s options to tailor therapy regimens during NAC to improve pathologic response outcome and consequently survival are limited. Therefore, minimally invasive methods that can provide an accurate prediction of response in the early stages of NAC are urgently needed. With many innovative treatment regimens using targeted therapies and/or immunotherapies being tested in clinical trials for breast cancer treatment, improved capability of accurate and early prediction of pathologic response to NAC may allow rapid individualized regimen de-escalation/alteration for responding/non-responding breast cancer patients in the future, facilitating precision medicine and leading to improved treatment outcomes.
In current SoC and clinical trials, the measurement of imaging tumor size change according to the Response Evaluation Criteria in Solid Tumors (RECIST) guidelines (7, 8) is the standard approach to assess tumor response to therapy. However, many studies have shown that tumor size changes in response to therapy, especially targeted therapies, often lag well behind changes in the underlying tumor biological functions (9–11), such as perfusion/permeability, cellularity, and metabolism. Therefore, the anatomic imaging approach of tumor size measurement is generally less effective for the early prediction of therapeutic response compared to functional imaging methods. As a noninvasive method for evaluation of microvascular perfusion and permeability, dynamic contrast-enhanced (DCE) MRI has been increasingly used in research settings, including early phase clinical trials, to evaluate breast cancer responses to NAC. There are usually three approaches in the analysis of DCE-MRI time-course data: qualitative curve shape description, calculation of semi-quantitative metrics such as contrast agent (CA) uptake and wash-out slopes, and quantitative pharmacokinetic (PK) modeling to extract parameters, such as Ktrans (CA volume transfer rate constant) and ve (extravascular, extracellular volume fraction), which are more directly reflective of the underlying biological functions and are in principle independent of scanner vendor platforms and data acquisition details. Thus, compared with qualitative and semi-quantitative DCE-MRI, quantitative DCE-MRI is hypothetically a more desirable approach for the assessment of cancer therapy response. Using summary receiver operating characteristic (SROC) analysis, a recent meta-analysis (12) of 14 published studies including 739 patients showed that the area under the curve (AUC), sensitivity, and specificity of Ktrans for early discrimination of pCR and non-pCR after one to two NAC cycles were 0.90, 84%, and 83%, respectively. However, the promise of Ktrans as an imaging biomarker for the prediction of breast cancer response to NAC has been demonstrated mostly by single-site and single-vendor platform DCE-MRI studies (13) that often sacrificed spatial resolution and/or coverage for the high temporal resolution necessary for PK modeling of the time-course data. There remain significant technical challenges in implementing quantitative DCE-MRI in multi-center (MC) and multi-vendor platform (MP) settings to assess breast cancer response to NAC, as many technical aspects in data acquisition and analysis, from temporal resolution to selection of the PK model and software tool, can affect the accuracy and precision of the derived PK parameters (14–17). Therefore, it is of paramount importance to standardize data acquisition and analysis strategies in MC and MP study settings (14, 15).
In this study, through preliminary analysis of data from an ongoing project, we report our initial experience in implementing quantitative DCE-MRI with simultaneous high spatial and temporal resolutions in an MC and MP setting to predict breast cancer response to NAC, compare predictive performances among quantitative and semi-quantitative DCE-MRI parameters, and tumor size measurement, and make initial best-practice recommendations with regard to PK analysis of breast DCE-MRI data acquired from different sites with different vendor platforms.
With local internal review board (IRB) approval, breast cancer patients treated with SoC NAC were enrolled with informed consent at three sites—Oregon Health & Science University (OHSU), University of Washington (UW), and University of Iowa (UI)—to participate in a longitudinal research MRI study. Four MRI sessions were performed before, during, and after the NAC course: Visit 1 (V1, before NAC), Visit 2 (V2, after the first cycle of NAC), Visit 3 (V3, at the midpoint of NAC; generally, after the completion of the first drug regimen but before the start of the second drug regimen), and Visit 4 (V4, after NAC but before surgery). Each patient’s pCR (defined as no residual invasive disease in the breast or axilla) or non-pCR status after NAC was determined by pathological analysis of the surgical tumor specimens as per the SoC procedures.
Fifteen patients across the three sites completed NAC treatment and MRI studies with pathologic response outcomes to date. Table 1 lists the clinicopathological characteristics of these 15 patients, with six of them achieving pCR (40%). The imaging results and correlations with the pathological response outcomes are reported below.
A 3T MRI scanner was used at each site for MRI data acquisition with each site employing a unique vendor platform, including Siemens (Siemens Healthineers, Erlangen, Germany), General Electric (GE Healthcare, Waukesha, WI), and Philips (Philips Healthcare, Best, the Netherlands). The vendor platform and software versions are listed in Table 2. Each MRI session consisted of a scout scan and the following scans in the axial plane with bilateral full breast coverage: T2-weighted MRI with fat suppression, T1-weighted MRI without fat suppression, axial diffusion-weighted MRI (DWI), B1 mapping, variable flip angle (VFA) gradient-echo (GRE) MRI for mapping of native tissue T1 (T1,0) (18), and DCE-MRI. Since this study mainly reports the results of DCE-MRI for the prediction of breast cancer response to NAC, only the sequences and acquisition parameters relevant to DCE-MRI quantification on the three vendor platforms are summarized in Table 2.
To achieve simultaneous high spatial and high temporal resolution DCE-MRI, similar product 3D GRE-based sequences employing Cartesian k-space undersampling in acquisition and view-sharing in reconstruction were used at the three sites for DCE-MRI data acquisition: Time-resolved angiography WIth Stochastic Trajectories (TWIST) (19–21), 4D Time-Resolved Angiography using keyhole (4D-TRAK) (22), and DIfferential Subsampling with Cartesian Ordering (DISCO) (23, 24) on Siemens, Philips, and GE platforms, respectively. Except for the first DCE time frame where full k-space data were acquired, the k-space data acquired for each remaining frame included the center region of the k-space and a portion of the peripheral k-space. For each of the three vendor sequences, the center region of the k-space was set at 15% of the full k-space, and the peripheral portion was set at 20% of the peripheral k-space. For VFA acquisitions, full k-space GRE MRI data were acquired, and three FAs of 3°, 9°, and 15° were used with the estimated Ernst angle positioned between the largest and smallest angles. The selection of these three FAs was automatically determined by a product T1 mapping sequence from one vendor following the entry of literature breast tumor R1 (= 1/T1) value of approximately 0.6 s−1 at 3T (25–27). B1 mapping, VFA-MRI, and DCE-MRI were spatially aligned during postprocessing.
For DCE-MRI, the same gadolinium-based CA, Prohance (Bracco Diagnostics Inc., Township, NJ, USA), dose (0.1 mmol/kg), injection rate (2 mL/s using a programmable power injector), and injection site (antecubital vein) were used at all three institutions. Intravenous administration of CA was initiated at the beginning of the third DCE frame acquisition, followed by a 20-mL saline flush at the same injection rate.
For quality assurance and quality control (QA/QC) of this MC and MP study, a bilateral breast phantom with a diffusion side and T1 side (CaliberMRI, Boulder, CO, USA; https://qmri.com/product/premium-single-wall-breast/) was scanned monthly at the three sites with the same DWI, B1 mapping, and VFA-MRI protocols used for the patient study. Data from the T1 side with compartments containing breast fibroglandular tissue- and adipose tissue-mimicking materials were used for the QA/QC of quantitative DCE-MRI. B1 maps and VFA data from the phantom T1 side were used to generate B1-corrected R1 maps, which were compared with the known ground-truth R1 values of the phantom at the experimental temperature.
Breast tumor longest diameter (LD) was measured by a site radiologist from post-contrast DCE-MRI images according to the RECIST 1.1 guidelines (8) for cases of a single primary tumor or the presence of multiple tumors in the same breast. Under the supervision of the site radiologist, tumor regions of interest (ROIs) were manually drawn by the site investigators on each image slice containing the contrast-enhanced tumor. If multiple tumors were present, ROIs were drawn independently for each tumor.
Together with the ROIs, de-identified B1 mapping, VFA-MRI, and DCE-MRI data from the patients enrolled at the two sites (UW and UI), as well as from the phantoms (not de-identified), were submitted to a secure server at the management site (OHSU) for further centralized analysis.
In a large multicenter clinical trial (28, 29), functional breast tumor volume calculated based on a semi-quantitative DCE-MRI parameter, the signal enhancement ratio (SER), was shown to be a promising imaging biomarker for predicting breast cancer response to NAC and survival. Following a similar approach, voxel-based SER values within the tumor ROIs were derived from the DCE time-course data using the following equation:
where S2 is the signal intensity from the second DCE frame, a pre-contrast baseline frame, and S9 and S26 are the post-contrast signal intensities from the 9th (early phase, approximately 110 s after contrast injection) and 26th (delayed phase, approximately 380 s after contrast injection) DCE frames, respectively.
To estimate the intrinsic tissue R1 (R1,0) value before CA arrival for each voxel, a B1-corrected R1 map was computed from the VFA data for both the phantom and de-identified patient data. Briefly, B1 DICOM images were first converted to a B1 ratio map based on vendor-provided formalisms. Therefore, voxel-based ratio value quantifies the fractional FA deviation from the nominal input value prescribed in the sequence. For example, a value of 1.0 reflects perfect agreement between the actual and prescribed FA values and 1.2 reflects an FA that is 20% larger than the nominal FA prescribed in the sequence. Each B1 acquisition had one or more accompanying image sets (used to calculate B1) which provided more anatomical details than the actual B1 map; therefore, we used these to co-register the B1 maps to the VFA images with the most similar contrast using publicly available ANTs software (30). B1 maps were interpolated to allow voxel-wise FA correction in the VFA data. When fitting an R1 value for each voxel against the VFA data, B1-corrected FA was used instead of the nominal value. Voxel-by-voxel fitting was performed using the standard nonlinear fitting approach using (Equation 2),
where is the B1-corrected FA that acts as the independent variable in the VFA R1 fitting and TR is the repetition time. R1 and S0 in (Equation 2) are the fitting parameters. The R1 in Equation 2 becomes R1,0 when the patient VFA data collected before DCE-MRI are fitted to the equation. In addition, it has been shown that potentially different scaling factors may be applied to image intensities across the three different FAs in VFA acquisition on a particular vendor platform (31). Before quantifying phantom R1 or tumor R1,0 from the VFA data obtained from that platform, corrections of signal intensities were made if differences in scaling factors were observed in the DICOM tags (31).
A generalized MRI modeling fitting package written in Python was developed at OHSU’s Advanced Imaging Research Center (AIRC). This package includes several DCE PK models. Using this package and several other software tools, a processing workflow was developed specifically for for this MC and MP study. Although the goal of the near future is to make the software package available to all three sites in this study (and eventually to the broader research community) for localized data processing, centralized data processing was used for this initial effort. The PK models used in data analysis are the fast-exchange-limit (FXL) Tofts model (TM) (32, 33) and the simplest fast-exchange-regime (FXR) exchange-sensitized shutter-speed models (SSM) (34, 35). The CA volume transfer rate constant, Ktrans, and extravascular extracellular space (EES) volume fraction, ve, were modeled using the TM. For SSM, the unidirectional cellular water efflux rate constant, kio, is modeled in addition to Ktrans and ve. The rate constant kep was calculated as Ktrans/ve in both models. The population-averaged arterial input function (AIF) (36) measured from an axillary artery in a previous single-breast DCE study in the sagittal plane was adopted for all PK modeling in this study. The PK modeling details are provided in the Supplementary Material.
In addition to the use of TM and SSM for PK data analysis, some additional practical considerations in PK modeling were also investigated. These included ROI- vs. voxel-based PK analysis in combination with the use of fixed, literature-reported R1,0 (fR1,0 = 0.60 s−1) (25–27) vs. VFA-measured R1,0 (mR1,0) for four analysis conditions for each PK model: ROI_ fR1,0, ROI_mR1,0, voxel_ fR1,0, and voxel_ mR1,0. When mR1,0 is used, the VFA series with FA (= 9°) closest to that of the DCE were used to coregister the measured R1,0 maps to DCE baseline data. For ROI-based analysis, multi-slice tumor ROIs were concatenated to form a 3D tumor ROI with a single averaged DCE time course for PK modeling. For voxel-based (within ROIs) analysis, each voxel DCE time course underwent PK modeling. The ROI-based analysis has the advantage of significantly increasing the signal-to-noise ratio (SNR) of the time-course data and much less computing time required for PK modeling, whereas the use of fR1,0 reduces the imaging time for data acquisition and simplifies data post-processing. Ktrans has been shown by many research studies to be the best quantitative DCE-MRI biomarker for prediction of breast cancer response to NAC (13). For this MC and MP study, Ktrans was used as the reference biomarker to investigate the effects of these four different quantitative DCE-MRI analysis approaches using either TM or SSM for PK modeling.
The bolus arrival time (BAT) is defined as the delay in the arrival of the CA in the tissue of interest from the artery where the AIF is measured. In general, it is assumed that the CA concentration time-course in the blood plasma (or AIF), Cp(t), is temporally aligned to match the CA concentration time-course in the tissue, Ct(t). Typically, this is performed manually or by convention (e.g., based on when the injection occurs), and a single global alignment is chosen. However, the time at which the CA bolus arrives at any given voxel is different owing to differing blood transit times. Even in studies where a high-quality AIF can be measured directly from some arterial voxels visible in the DCE acquisition, it is likely that the AIF needs to be time-shifted for accurate PK analysis of the tissue time-course data of any given voxel. Misalignment can cause biases in all estimated PK model parameters. To reduce these biases (and reduce manual work in time-shifting AIF) we adopted a model-based approach to align the AIF for each tissue voxel curve. To achieve this, we fit a linearized version of TM (37), wrapped in a nonlinear optimizer that solves for a single parameter: BAT. Because this nonlinear problem can have multiple local minima, we performed the optimization in two phases, starting with a brute force search using a coarse grid, followed by an iterative solver (using Powell’s method) for fine-tuning.
The sensitivity of DCE-MRI data to water exchange depends on many factors such as CA dosage, CA extravasation kinetics, and DCE-MRI pulse sequence parameters (38). With the standard CA dose and a DCE-MRI sequence optimized for better SNR, that is also inherently water exchange sensitive (38), such as the case in this study, the most important factor that drives the DCE data sensitivity to kio is tissue-specific CA extravasation. It has been shown that when a DCE-MRI time-course is insensitive to water exchange and kio is still fitted as a variable, the returned kio parameter often hits the FXL-limit fitting boundaries (39). In this study, the fitting upper boundary for kio was set at 1,000 s−1 to minimize the occurrence of fitting procedures stopping at a parameter boundary prematurely. Because the fitting sensitivity of kio for each voxel-based DCE time-course within the tumor ROIs strongly depends on voxel-based CA extravasation, which was unknown before PK modeling, voxel-based SSM modeling was initially performed for all voxel data within the tumor ROIs. These fitted kio values were then filtered with a biologically meaningful and DCE-MRI achievable range of 0.1 s−1–20 s−1. A kio of 0.1 s−1 or lower reflects that its reciprocal, the mean intracellular lifetime, is on the order of 10 s or larger. This is an unrealistically large value for relatively small sizes of breast tissue cells. For example, based on a spherical cell model, Sehy et al. estimated that for an intracellular water lifetime of 10 s, the “cell size” is on the order of ~300 μm (40), at least an order of magnitude higher than that of breast cancer cells (41). For the upper limit, a kio value of 20 s−1 or higher indicates that the transmembrane water molecule exchange process represented by k (= kio + koi, where koi is the rate constant defining the process of water molecules entering the intracellular space from EES (42)) is even greater. An in vivo system with k >20 s−1 will appear to be closer to the FXL than the FXR condition in a breast DCE experiment with a single-dose CA administered intravenously. After this simple voxel-based kio filtering, the fraction of tumor voxels with kio within the range of 0.1 s−1–20 s−1 was recorded, and descriptive statistics were then used to summarize the filtered kio results.
For each patient at each MRI visit, the tumor LD, SER, and quantitative parameters from PK modeling were reported. For voxel-based analysis (SER and PK parameters), the mean tumor parameter value was calculated by averaging voxel parameter values. In addition, the median and width of the interquartile range (iqr = 75 percentile voxel parameter value − 25 percentile voxel parameter value) from the histogram analysis of the voxel parameter distribution were also obtained. For ROI-based analysis (PK parameters only), the derived parameter values from PK modeling of the single DCE time course were reported as the mean tumor parameter values. If multiple tumors were present, the average of the parameter value from each tumor was reported.
Descriptive statistical analysis was performed for each MRI metric at each visit, as well as the percent change relative to baseline (V1), such as V21% (percent change at V2 relative to V1) and V31% (percent change at V3 relative to V1), for each response group (pCR and non-pCR). Differences between the groups were assessed using the Wilcoxon rank-sum test. Student’s t-test was used to evaluate the differences in Ktrans among the four PK analysis approaches of ROI- and voxel-based analysis in combination with fR1,0 and mR1,0, and between TM and SSM. Statistical significance was set at P-values <0.05.
In this preliminary study, the discriminative performances of V21% and V31% of each MRI metric for early prediction of pCR vs. non-pCR were evaluated using univariate logistic regression with ROC curves, and AUC values were calculated with 95% confidence intervals (CIs). All statistical analyses were performed using R: A Language and Environment for Statistical Computing (43).
The results of the tumor mean Ktrans from V1 to V3 (two patients missed V3 scans) and its performance for early prediction of NAC response are reported here. Figure 1 shows column graphs of TM and SSM mean ± SD Ktrans of the patient cohort under the analysis conditions of ROI_ fR1,0, ROI_mR1,0, voxel_ fR1,0, and voxel_ mR1,0. The VFA-measured phantom R1 and in vivo breast tumor R1,0 values from the two vendor platforms were in excellent agreement with the ground truth R1 of the fibroglandular tissue mimicking material and literature reported breast tumor R1,0 at 3T (25–27), respectively. However, due to technical reasons still under investigation, the corresponding VFA-measured R1 and R1,0 values from the other vendor platforms were overestimated by ≥100%, resulting in failure in PK modeling of patient DCE data from that platform. Thus, patient data from that platform (N = 2) were not included under the mR1,0 condition in Figure 1. SSM Ktrans was significantly (P <0.05) larger than TM Ktrans under all conditions, while Ktrans from the voxel-based analysis was significantly (P <0.05) larger than that from the ROI-based analysis. There was no statistically significant difference in Ktrans between fR1,0 and mR1,0 for either ROI- or voxel-based PK analysis using TM or SSM. Figure 2 shows voxel-based V1 Ktrans parametric maps of pCR and non-pCR tumors obtained from TM and SSM PK analyses using fR1,0. For each patient, color Ktrans maps from the same image slice are shown for comparison of the TM and SSM analyses. It can be clearly observed that SSM Ktrans was substantially greater than TM Ktrans in both tumors.
Figure 1. Column graphs of mean TM (blue) and SSM (orange) Ktrans of the patient cohort under the analysis conditions of ROI_ fR1,0, ROI_mR1,0, voxel_ fR1,0, and voxel_ mR1,0 from Visit 1 (V1) to Visit 3 (V3). Error bars represent the positive standard deviation (SD). N: patient number; *statistically significant (P <0.05, t-tests) difference in Ktrans between TM and SSM under the same analysis conditions; #statistically significant (P <0.05, t-tests) difference in Ktrans between ROI- and voxel-based analyses under the same R1,0 and PK model conditions.
Figure 2. Voxel-based tumor Ktrans color parametric maps for 43-year old pCR (left breast cancer, top row) and 53-year old non-pCR (left breast cancer, bottom row) patients at V1, obtained from TM (left column) and SSM (right column) PK analysis, respectively. For each patient, the Ktrans maps (overlaid on post-contrast DCE images) from the same slice are shown, and the color scale is kept the same to allow the comparison of TM and SSM Ktrans. It can be clearly observed that SSM Ktrans was substantially greater than TM Ktrans in both tumors.
Table 3 lists the ROC AUC values of Ktrans percent changes, V21% and V31%, for the early prediction of pCR (N = 6) vs. non-pCR (N = 9). SSM Ktrans from voxel-based analysis exhibited a better predictive performance than TM Ktrans, with SSM Ktrans under the condition of voxel_fR1,0 showing the highest predictive accuracy. Overall, for both TM and SSM Ktrans, voxel-based PK analysis using fR1,0 was the optimal approach for early prediction of NAC response at both V2 and V3. Additionally, the use of fR1,0 for PK analysis also allowed for inclusion of patient data from the vendor platform that produced substantial errors in VFA R1 measurement, which would otherwise be discarded if mR1,0 was used for PK analysis. Therefore, we proceeded to compare the quantitative DCE-MRI parameters derived with the voxel_fR1,0 approach with SER and tumor LD for early prediction of NAC response.
Table 3. Early prediction of breast cancer response to neoadjuvant chemotherapy using Ktrans percent change under different analysis conditions.
Table 4 shows the percent changes in MRI metrics (V21% and V31%) of the pCR and non-pCR groups, P-values from the Wilcoxon test comparing the two groups, and ROC AUC values for the early prediction of pCR vs. non-pCR. For the semi-quantitative SER parameter, percent changes in tumor mean SER showed higher AUC values than those of median SER and are listed in Table 4. The results for the PK parameters reported here were all obtained using the voxel_fR1,0 approach, and only those with percent changes showing AUC ≥0.80, indicating good predictive performance, are summarized in Table 4. After the first NAC cycle, V21% of LD was a poor predictor of response with AUC = 0.56, whereas V21% of SER and several SSM PK parameters showed fair to good predictive performance with SSM Ktrans (mean), ve (mean), Ktrans (iqr), and kep (iqr) showing AUC values of 0.83, 0.81, 0.80, and 0.81, respectively. None of the TM parameters demonstrated an AUC ≥0.80 after the first NAC cycle. At NAC midpoint, while V31% of both LD and SER demonstrated similar good predictive performances with AUC = 0.86 and 0.83, respectively, V31% of TM and SSM Ktrans and kep, whether the mean or median value, were excellent predictors with AUC >0.90. Furthermore, both SSM Ktrans (iqr) and kep (iqr) percent changes separated the two groups completely, with AUC = 1. The associated, representative ROC curves are shown in Figure 3. Figure 4 shows the tumor SSM Ktrans parametric color maps at V1, V2, and V3 for a non-pCR and pCR patient. There were no noticeable changes in Ktrans of the non-pCR tumor from V1 to V3. However, the decrease in Ktrans was substantial in the pCR tumor from V1 to V2, and the values remained low at V3. Figure 5 shows histograms of voxel SSM Ktrans values for a pCR and a non-pCR tumor, from V1 to V3. The Ktrans iqr values indicated by widths of the grey columns were 0.33 min−1, 0.44 min−1, and 0.21 min−1 for the non-pCR and 0.42 min−1, 0.21 min−1, and 0.0072 min−1 for the pCR, respectively, from V1 to V3. The difference in iqr changes at V2 and V3 relative to V1 is striking between these two tumors: a 33% increase at V2 and 36% decrease at V3 for the non-pCR, while a 50% decrease at V2 and 98% decrease at V3 for the pCR group.
Figure 3. ROC curves of V21% (left) and V31% (right) of LD, SER (mean), TM Ktrans (mean), SSM Ktrans (mean), and SSM Ktrans (iqr) for the early discrimination of pCR and non-pCR. AUC values are shown in parentheses in the figure legends.
Figure 4. Voxel-based tumor SSM Ktrans parametric maps in color (overlaid on a cropped post-contrast DCE image slice through the center of the tumor) at V1, V2, and V3 from a 52-year old non-pCR patient with left breast cancer (top) and 57-year old pCR patient with right breast cancer (bottom). For each tumor, the Ktrans color scale was kept the same from V1 to V3 to allow the visual assessment of Ktrans changes.
Figure 5. V1–V3 histograms of voxel SSM Ktrans within the tumor ROIs from a non-pCR patient (top; the same patient as shown in Figure 4) and a 42-year old pCR patient with right breast cancer (bottom). The width of the gray column in each panel represents the iqr value. For each patient, the x-axis (Ktrans) scale was kept the same from V1 to V3 to demonstrate longitudinal changes in Ktrans iqr. For the V3 histogram of the pCR patient, an inset with a much smaller Ktrans scale (0 min−1–0.1 min−1) is shown for better visualization of this histogram.
Figure 6 summarizes the fraction means (SD error bars) of the filtered kio within the tumor ROIs for the two response groups. The fractions of voxels with filtered, meaningful kio generally decreased from V1 to V4. The difference between V1 and V4 was substantial for both the non-pCR (gray) and pCR (blue) groups. Furthermore, the V4 fractions of pCRs were much smaller than those of non-pCRs. In addition, fraction means showed little R1,0-selection dependence between the use of fR1,0 and mR1,0 (data not shown). Both V21% and V31% of filtered kio mean or median had ROC AUC values <0.80 for early prediction of NAC response.
Figure 6. Fraction means (SD error bars) of the filtered kio within the tumor ROIs for the two response groups were plotted. The fraction of voxels with filtered kio values generally decreased from V1 to V4. The difference between V1 and V4 was highly substantial for both the non-pCR (gray) and pCR (blue) groups. Furthermore, the V4 fraction of filtered kio was much smaller for pCRs than for non-pCRs.
In Figure 7, filtered tumor ROI kio results at two visits (V1, V3) for a non-pCR (A, B) and a pCR (C, D) patient are shown. In each panel, the filtered kio color map overlaid on a zoomed post-contrast DCE image is shown on the left, and the voxel-based Σ[CAo] map on the right shows the summation of the EES CA concentration, [CAo], over the entire DCE time-course within the tumor ROI. In all four panels, the results from the center slice of the respective tumors are shown. The white arrows in (C) and (D) indicate artifacts caused by a metal biopsy clip. In the pCR tumor, a larger area of kio was filtered out at V3 (D, orange arrow) compared to a smaller filtered-out area at V1 (C, orange arrow) due to kio filtering, in addition to our built-in quality control that masked out unenhanced voxels. Σ[CAo] approximates the total CA extravasation during the entire DCE acquisition and serves as a simple quantitative surrogate for monitoring the sensitivity of the voxel DCE time-course to water exchange kinetics. Cold spots in the Σ[CAo] maps, where CA extravasation was low, matched the areas of the filtered-out kio quite well, supporting the validity of our filtering approach to a certain degree.
Figure 7. Filtered tumor ROI kio results at two visits (V1 and V3) for a non-pCR (A, B) and a pCR (C, D) patient are shown. In each panel, kio color map overlaid on a zoomed post-contrast DCE image is shown on the left, and the Σ[CAo] map on the right shows the voxel-based summation of the EES CA concentration, [CAo], over the entire DCE time course within the tumor ROI. In all four panels, results from the center slice of the respective tumors are shown. The white arrows in (C, D) point to the artifacts caused by a metal biopsy clip. A larger area of the pCR tumor was filtered out in the V3 kio map [(D), orange arrow] compared to a smaller filtered-out area in the V1 kio map [(C), orange arrow]. There were no noticeable filtered kio areas in the non-pCR tumor at either V1 or V3.
To the best of our knowledge, this is the first MC and MP study using quantitative DCE-MRI to predict breast cancer response to NAC, where GRE-based product sequences of k-space undersampling during acquisition and view-sharing during reconstruction from Siemens, Philips, and GE platforms were used for high spatial and temporal resolution breast DCE-MRI. The data acquisition scheme used for DCE-MRI in this MC and MP setting allows for bilateral full breast coverage with adequate spatial resolution for accurate morphological evaluation, as well as sufficient temporal resolution for quantitative PK analysis of the breast DCE time-course data (14, 15). In today’s SoC breast DCE-MRI protocols, owing to trade-offs between spatial and temporal resolution in conventional sequences, the necessity for accurate tumor morphology assessment with high spatial resolution and spatial coverage results in low temporal resolutions between 60 s and 120 s, which precludes meaningful PK modeling of time-course data with acceptable accuracy (15, 16). With potentially faster dynamic imaging methods available from vendors through combinations of accelerated data acquisition approaches and advanced reconstruction algorithms (15), the ability to acquire breast DCE-MRI with simultaneous high spatial and temporal resolutions using vendor product sequences may facilitate the translation of quantitative DCE-MRI into clinical workflow.
With inherent differences in hardware and software among the three major MRI vendor platforms, standardizations in VFA and DCE acquisition parameters were implemented across the three sites to minimize differences in the acquired data and consequently, variations in results from data analysis (14, 15, 17). These included the use of the same three FAs in VFA acquisition for R1,0 mapping, and the same center and peripheral portions of the k-space in the three vendor-specific k-space undersampling and view-sharing product sequences for DCE-MRI acquisition. In addition, the same FA (10°), minimal TE (0.9 ms–2.9 ms), and similar TR in the range of 5.0 ms–6.2 ms were used across the platforms to ensure DCE data sensitivity to the water exchange effects (38), supporting the use of the SSM for PK analysis. Despite efforts in data acquisition standardization and centralized data analysis using a single software tool for both VFA R1 fitting and DCE-MRI PK modeling, VFA measurements of phantom R1 and patient tumor R1,0 on one vendor platform resulted in substantial biases compared to ground truth values in the phantom and measurements on the other two platforms, as well as literature reported breast tumor R1,0 values (25–27). The ≥100% overestimation of tumor R1,0 on this platform also caused patient data fitting failures when using either the TM or SSM. Therefore, PK modeling of patient data from this vendor platform was performed using fR1,0 only, excluding the use of mR1,0 from the analysis. The sources of errors in VFA R1 mapping on this platform are still under investigation. VFA fitting is inherently challenging owing to the need to fit multiple variables, which requires numerous individual images of unique FAs. Because this is not practical owing to the scan time, several assumptions are typically made to simplify the fitting, including the linearity and spatial uniformity of B1 over multiple transmitted power settings, as well as the direct correspondence between the delivered FA of the independent B1 mapping sequence and the VFA sequence. These assumptions rely on the use of non-clinically validated research tools from vendor platforms. However, these assumptions may not always hold true on specific vendor implementations. Furthermore, each vendor uses a different method to map the FA. There are no standards for the reporting of FA or B1 maps, including units, presenting challenges when assimilating data across multivendor platforms. One valuable lesson learned here for implementing MC and MP quantitative DCE-MRI is the importance of QA/QC scans of phantoms with ground truth R1 values to determine whether all vendor platforms provide reliable R1 measurements. If this is not the case, either error sources should be identified and corrective actions taken, or an alternative solution, such as the use of fR1,0 for PK analysis, should be found.
In this study, using Ktrans as the reference imaging biomarker for the prediction of breast cancer response to NAC, we found that voxel-based analysis using a literature-reported fR1,0 value was the optimal approach for PK analysis of DCE-MRI data collected from the MC and MP settings, whether the TM or SSM was used for data modeling. This approach allowed the inclusion of data from one platform, where the mR1,0 values calculated from VFA measurements were unreliable. The statistically insignificant differences in Ktrans between the use of fR1,0 and mR1,0 (Figure 1) suggest that it is reasonable and practical to use fR1,0 for PK modeling (44), which can mitigate random and systematic errors from R1,0 measurement across vendor platforms and potentially eliminate the need for B1 mapping and VFA acquisition in MC and MP trials. Although the use of fR1,0 instead of mR1,0 in PK analysis is expected to cause systematic errors in the estimated PK parameters, unlike random errors, the impact of systematic errors is lessened when percent changes in PK parameters, such as V21% and V31%, are used in a longitudinal study to predict breast cancer response to NAC. Compared with voxel-based analysis, ROI-based analysis dilutes tumor heterogeneity in perfusion and permeability, resulting in significantly smaller Ktrans values and a narrower range of Ktrans changes in response to therapy. The latter may potentially reduce the predictive performance of Ktrans for NAC response. Another disadvantage of ROI-based analysis is the inability to assess changes in PK parameter heterogeneity in response to treatment.
The preliminary results from this MC and MP study show that after only one NAC cycle, semi-quantitative and quantitative DCE-MRI metrics outperformed tumor size measurement in the early prediction of breast cancer response to NAC. The quantitative parameter Ktrans consistently provided a more accurate prediction of NAC response than both size measurement (LD) and SER after the first NAC cycle and at the NAC midpoint. These findings agree with many similar studies that used DCE-MRI to assess the breast cancer response to NAC (12, 13). The larger decreases in Ktrans (iqr) and kep (iqr) in pCRs compared to non-pCRs at V2 and especially V3 indicate greater decreases in tumor perfusion/permeability heterogeneity in patients responding to NAC regimens.
Consistent with previous studies (36, 45), SSM Ktrans was substantially greater than TM Ktrans in this cohort of malignant breast tumors when DCE-MRI acquisition was sensitive to the water-exchange effect (38). Since there is no significant difference between SSM and TM Ktrans in benign breast lesions or normal tissue (36, 45), SSM Ktrans potentially has better predictive performance than TM Ktrans due to the former’s greater dynamic ranges of change in response to therapy, assuming that microvascular properties of a responding tumor shift towards those of a benign lesion or normal tissue. This is manifested by the fact that, after the first NAC cycle, while V21% of mean SSM Ktrans was a good predictor of NAC response with an ROC AUC value of 0.83, V21% of mean TM Ktrans was only a fair predictor with ROC AUC = 0.70 (not shown in Table 4). Although at the NAC midpoint, both SSM and TM V31% Ktrans (and kep) are excellent predictors of NAC response, the percent changes of SSM Ktrans and kep were larger than those of the TM counterparts, and the P-values from comparing the two response groups were generally smaller for the SSM parameters.
Our initial experience shows that when the water exchange effect is explicitly modeled in the SSM analysis of DCE-MRI data, the kio parameter may provide complementary information to the more commonly modeled Ktrans parameter, which only focuses on CA kinetics. Recent studies have shown that kio is an imaging biomarker of metabolic activity (46). In fact, V31% of the tumor mean kio was a fair early predictor of NAC response with an ROC AUC value of 0.71 (not shown in Table 4). Combining Ktrans and kio in a multivariate predictive model may further improve the predictive accuracy for NAC response. NAC regimens often reduce tumor permeability/vascularity (21), resulting in reduced interstitium [CA] during a DCE study. This, in turn, makes kio quantification less reliable. In other words, reduced CA extravasation results in a smaller R1 difference between extracellular and intracellular spaces. This decreases the DCE-MRI sensitivity to the water exchange effect and, consequently, negatively impacts the accuracy and precision of SSM quantification of kio. Therefore, caution should be exercised when evaluating the estimated kio values from the SSM analysis. Unreliable voxel kio values should be filtered out. The smaller fraction of filtered kio at V4 in pCRs compared to non-pCRs quantitatively reflected lower CA extravasation in the former, consistent with the Ktrans and kep results. The right side of each panel in Figure 7 represents a simple method for quantitatively estimating the extent of CA extravasation using Σ[CAo]. As expected, the pCR tumor at V3 showed low Σ[CAo] owing to reduced perfusion and permeability in response to NAC treatment, resulting in large areas of unreliable kio being filtered out. A more accurate measure of voxel DCE data sensitivity to water exchange should include an estimation of the extent and duration of |R1i − R1o| absolute difference between intracellular and extracellular R1 exceeding (or at least close to) the exchange kinetics defined by k, the transmembrane water molecule exchange process (42). However, this may add complications in translational studies, such as this MC and MP study.
Our model-based approach for estimating BAT eliminated the need to manually align the AIF with voxel-based tissue DCE curves in PK analysis, which improves automation in the entire data processing workflow. Manual inspection of the model-selected BATs showed robust and qualitatively good performance. Future work will include quantifying the performance using a digital phantom for comparison with manual alignment.
There are several limitations to this preliminary MC and MP study. The main limitation is the small sample size of 15 patients in total, which caused large 95% CI ranges of the ROC AUC values for the prediction of NAC response and may artificially inflate the predictive performances of the quantitative PK parameters. The small sample size at each site also renders cross-vendor platform comparison of results unreliable, and therefore, was not performed. Second, for correlation analysis between MRI metrics and pathologic response outcomes, the small sample size precluded meaningful analysis stratified by breast cancer subtypes, as shown in Table 1. Therefore, the results presented here are more reflective of those from the general breast cancer population treated with SoC NAC regimens. Third, we did not perform a multivariate analysis by combining clinicopathological features with individual MRI metrics with high predictive performance, which may further enhance the capability of early prediction of NAC response, especially after the first NAC cycle. Fourth, we only used the iqr of voxel-based parameters to characterize changes in tumor heterogeneity in response to NAC, without performing a more comprehensive radiomics analysis (47), which may provide better predictive performance. Lastly, only three FAs within a relatively narrow range were used in VFA measurements of phantom R1 and breast tumor R1,0. In clinical practice, where breast tumor R1,0 values are unknown and could vary greatly, using more FAs over a larger range may result in more accurate R1,0 mapping, and consequently, more accurately estimated PK parameters. However, the usual time constraint for a clinical MRI protocol makes it difficult to add more FAs to the VFA acquisition. Furthermore, the small number of FAs used in this study is unlikely to be the reason why one vendor platform returned substantially biased R1 and R1,0 values.
In conclusion, the initial results from this MC and MP study validate findings from many single-site studies that quantitative DCE-MRI is superior to tumor size measurement for the prediction of breast cancer response to NAC. Both SSM and TM Ktrans showed better predictive performance than the semi-quantitative SER metric. Furthermore, Ktrans and kep derived from the SSM, using DCE-MRI data acquired with sensitivity to the water exchange effect, generally performed better than the TM counterparts in the prediction of NAC response, especially after only one cycle of NAC. Due to potential large variations in the accuracy of VFA-measured R1,0 on different vendor platforms, SSM PK analysis using a fixed, literature-reported breast tumor R1,0 could be a best-practice approach in quantitative DCE-MRI prediction of breast cancer response to NAC in an MC and MP setting.
The raw data supporting the conclusions of this article will be made available by the authors, without undue reservation.
The studies involving humans were approved by Internal Review Boards of Oregon Health & Science University (OHSU), University of Washington (UW), and University of Iowa (UI). The studies were conducted in accordance with the local legislation and institutional requirements. The participants provided their written informed consent to participate in this study.
BM: Data curation, Investigation, Software, Writing – original draft, Writing – review & editing, Methodology, Visualization. XL: Data curation, Investigation, Methodology, Software, Writing – original draft, Writing – review & editing, Supervision, Conceptualization. MH: Data curation, Investigation, Writing – review & editing, Visualization. AS: Data curation, Investigation, Visualization, Writing – review & editing. JL: Formal Analysis, Investigation, Writing – review & editing, Methodology. DB: Data curation, Investigation, Writing – review & editing, Visualization. AK: Data curation, Investigation, Writing – review & editing, Visualization. AT: Data curation, Investigation, Project administration, Writing – review & editing. IL: Data curation, Investigation, Project administration, Writing – review & editing. MB: Data curation, Investigation, Project administration, Writing – review & editing. CW: Data curation, Investigation, Project administration, Writing – review & editing. CP: Data curation, Investigation, Project administration, Writing – review & editing. HR: Data curation, Investigation, Validation, Writing – review & editing. SH: Data curation, Investigation, Validation, Writing – review & editing. TR: Data curation, Investigation, Validation, Writing – review & editing. SD: Data curation, Investigation, Validation, Writing – review & editing. AB: Data curation, Investigation, Validation, Writing – review & editing. EH: Data curation, Investigation, Validation, Writing – review & editing. AZ: Data curation, Investigation, Validation, Writing – review & editing. JS: Data curation, Investigation, Validation, Writing – review & editing. SP: Data curation, Investigation, Validation, Writing – review & editing. NF: Data curation, Investigation, Validation, Writing – review & editing. JH: Conceptualization, Data curation, Funding acquisition, Investigation, Methodology, Project administration, Resources, Supervision, Writing – original draft, Writing – review & editing. SP: Conceptualization, Data curation, Funding acquisition, Investigation, Methodology, Project administration, Resources, Supervision, Writing – original draft, Writing – review & editing. WH: Conceptualization, Data curation, Funding acquisition, Investigation, Methodology, Project administration, Resources, Supervision, Writing – original draft, Writing – review & editing.
The author(s) declare financial support was received for the research, authorship, and/or publication of this article. Grant support from National Institutes of Health (NIH): R01 CA248192, R01 CA190299, UM1TR004403, S10OD025025, and P30 CA086862.
This work was supported by NIH S10OD021701 for the 3T Siemens Prisma MRI instrument housed at OHSU’s Advanced Imaging Research Center. This work was supported by NIH S10OD018224 for the High-Performance Computing Cluster, housed at OHSU’s Advanced Imaging Research Center.
The authors declare that the research was conducted in the absence of any commercial or financial relationships that could be construed as a potential conflict of interest.
All claims expressed in this article are solely those of the authors and do not necessarily represent those of their affiliated organizations, or those of the publisher, the editors and the reviewers. Any product that may be evaluated in this article, or claim that may be made by its manufacturer, is not guaranteed or endorsed by the publisher.
The Supplementary Material for this article can be found online at: https://www.frontiersin.org/articles/10.3389/fonc.2024.1395502/full#supplementary-material
1. Fisher B, Bryant J, Wolmark N, Mamounas E, Brown A, Fisher ER, et al. Effect of preoperative chemotherapy on the outcome of women with operable breast cancer. J Clin Oncol. (1998) 16:2672–85. doi: 10.1200/JCO.1998.16.8.2672
2. Mauri D, Pavlidis N, Ioannidis JP. Neoadjuvant versus adjuvant systemic treatment in breast cancer: a meta-analysis. J Natl Cancer Inst. (2005) 97:188–94. doi: 10.1093/jnci/dji021
3. Symmans WF, Peintinger F, Hatzis C, Rajan R, Kuerer H, Valero V, et al. Measurement of residual breast cancer burden to predict survival after neoadjuvant chemotherapy. J Clin Oncol. (2007) 25:4414–22. doi: 10.1200/JCO.2007.10.6823
4. Redden MH, Fuhrman GM. Neoadjuvant chemotherapy in the treatment of breast cancer. Surg Clin North Am. (2013) 93:493–9. doi: 10.1016/j.suc.2013.01.006
5. Bonnefoi H, Litiere S, Piccart M, MacGrogan G, Fumoleau P, Brain E, et al. Pathological complete response after neoadjuvant chemotherapy is an independent predictive factor irrespective of simplified breast cancer intrinsic subtypes: a landmark and two-step approach analyses from the EORTC 10994/BIG 1-00 phase III trial. Ann Oncol. (2014) 25:1128–36. doi: 10.1093/annonc/mdu118
6. von Minckwitz G, Untch M, Blohmer JU, Costa SD, Eidtmann H, Fasching PA, et al. Definition and impact of pathologic complete response on prognosis after neoadjuvant chemotherapy in various intrinsic breast cancer subtypes. J Clin Oncol. (2012) 30:1796–804. doi: 10.1200/JCO.2011.38.8595
7. Therasse P, Arbuck SG, Eisenhauer EA, Wanders J, Kaplan RS, Rubinstein L, et al. New guidelines to evaluate the response to treatment in solid tumors. European Organization for Research and Treatment of Cancer, National Cancer Institute of the United States, National Cancer Institute of Canada. J Natl Cancer Inst. (2000) 92:205–16. doi: 10.1093/jnci/92.3.205
8. Eisenhauer EA, Therasse P, Bogaerts J, Schwartz LH, Sargent D, Ford R, et al. New response evaluation criteria in solid tumours: revised RECIST guideline (version 1.1). Eur J Cancer. (2009) 45:228–47. doi: 10.1016/j.ejca.2008.10.026
9. O'Connor JP, Jackson A, Parker GJ, Roberts C, Jayson GC. Dynamic contrast-enhanced MRI in clinical trials of antivascular therapies. Nat reviewsClinical Oncol. (2012) 9:167–77. doi: 10.1038/nrclinonc.2012.2
10. Yankeelov TE, Mankoff DA, Schwartz LH, Lieberman FS, Buatti JM, Mountz JM, et al. Quantitative imaging in cancer clinical trials. Clin Cancer Res. (2016) 22:284–90. doi: 10.1158/1078-0432.CCR-14-3336
11. Leach MO, Morgan B, Tofts PS, Buckley DL, Huang W, Horsfield MA, et al. Imaging vascular function for early stage clinical trials using dynamic contrast-enhanced magnetic resonance imaging. Eur radiology. (2012) 22:1451–64. doi: 10.1007/s00330-012-2446-x
12. Jun W, Cong W, Xianxin X, Daqing J. Meta-analysis of quantitative dynamic contrast-enhanced MRI for the assessment of neoadjuvant chemotherapy in breast cancer. Am Surg. (2019) 85:645–53. doi: 10.1177/000313481908500630
13. Huang W. Quantitative DCE-MRI of the breast. In: Pinker K, Mann R, Partridge S, editors. Breast MRI – State of the Art and Future Directions. Elsevier Academic Press, London UK (2022). p. 425–58.
14. Shukla-Dave A, Obuchowski NA, Chenevert TL, Jambawalikar S, Schwartz LH, Malyarenko D, et al. Quantitative imaging biomarkers alliance (QIBA) recommendations for improved precision of DWI and DCE-MRI derived biomarkers in multicenter oncology trials. J Magn Reson Imaging. (2019) 49:e101–e21. doi: 10.1002/jmri.26518
15. Li X, Huang W, Holmes JH. Dynamic contrast-enhanced (DCE) MRI. Magn Reson Imaging Clin N Am. (2024) 32:47–61. doi: 10.1016/j.mric.2023.09.001
16. Khalifa F, Soliman A, El-Baz A, Abou El-Ghar M, El-Diasty T, Gimel'farb G, et al. Models and methods for analyzing DCE-MRI: a review. Med Phys. (2014) 41:124301. doi: 10.1118/1.4898202
17. Huang W, Li X, Chen Y, Li X, Chang MC, Oborski MJ, et al. Variations of dynamic contrast-enhanced magnetic resonance imaging in evaluation of breast cancer therapy response: a multicenter data analysis challenge. Trans Oncol. (2014) 7:153–66. doi: 10.1593/tlo.13838
18. Deoni SC, Peters TM, Rutt BK. Determination of optimal angles for variable nutation proton magnetic spin-lattice, T1, and spin-spin, T2, relaxation times measurement. Magn Reson Med. (2004) 51:194–9. doi: 10.1002/mrm.10661
19. Song T, Laine AF, Chen Q, Rusinek H, Bokacheva L, Lim RP, et al. Optimal k-space sampling for dynamic contrast-enhanced MRI with an application to MR renography. Magn Reson Med. (2009) 61:1242–8. doi: 10.1002/mrm.21901
20. Tudorica LA, Oh KY, Roy N, Kettler MD, Chen Y, Hemmingson SL, et al. A feasible high spatiotemporal resolution breast DCE-MRI protocol for clinical settings. Magnetic Resonance Imaging. (2012) 30:1257–67. doi: 10.1016/j.mri.2012.04.009
21. Tudorica A, Oh KY, Chui SY, Roy N, Troxell ML, Naik A, et al. Early prediction and evaluation of breast cancer response to neoadjuvant chemotherapy using quantitative DCE-MRI. Transl Oncol. (2016) 9:8–17. doi: 10.1016/j.tranon.2015.11.016
22. Willinek WA, Hadizadeh DR, von Falkenhausen M, Urbach H, Hoogeveen R, Schild HH, et al. 4D time-resolved MR angiography with keyhole (4D-TRAK): more than 60 times accelerated MRA using a combination of CENTRA, keyhole, and SENSE at 3. 0T J Magn Reson Imaging. (2008) 27:1455–60. doi: 10.1002/jmri.21354
23. Saranathan M, Rettmann DW, Hargreaves BA, Clarke SE, Vasanawala SS. DIfferential Subsampling with Cartesian Ordering (DISCO): a high spatio-temporal resolution Dixon imaging sequence for multiphasic contrast enhanced abdominal imaging. J Magn Reson Imaging. (2012) 35:1484–92. doi: 10.1002/jmri.23602
24. Morrison CK, Henze Bancroft LC, DeMartini WB, Holmes JH, Wang K, Bosca RJ, et al. Novel high spatiotemporal resolution versus standard-of-care dynamic contrast-enhanced breast MRI: comparison of image quality. Invest Radiol. (2017) 52:198–205. doi: 10.1097/RLI.0000000000000329
25. Pineda FD, Medved M, Fan X, Karczmar GS. B1 and T1 mapping of the breast with a reference tissue method. Magn Reson Med. (2016) 75:1565–73. doi: 10.1002/mrm.25751
26. Rakow-Penner R, Daniel B, Yu H, Sawyer-Glover A, Glover GH. Relaxation times of breast tissue at 1.5T and 3T measured using IDEAL. J Magn Reson Imaging. (2006) 23:87–91. doi: 10.1002/jmri.20469
27. Meng T, He N, He H, Liu K, Ke L, Liu H, et al. The diagnostic performance of quantitative mapping in breast cancer patients: a preliminary study using synthetic MRI. Cancer Imaging. (2020) 20:88. doi: 10.1186/s40644-020-00365-4
28. Hylton NM, Gatsonis CA, Rosen MA, Lehman CD, Newitt DC, Partridge SC, et al. Neoadjuvant chemotherapy for breast cancer: functional tumor volume by MR imaging predicts recurrence-free survival-results from the ACRIN 6657/CALGB 150007 I-SPY 1 TRIAL. Radiology. (2016) 279:44–55. doi: 10.1148/radiol.2015150013
29. Hylton NM, Blume JD, Bernreuter WK, Pisano ED, Rosen MA, Morris EA, et al. Locally advanced breast cancer: MR imaging for prediction of response to neoadjuvant chemotherapy–results from ACRIN 6657/I-SPY TRIAL. Radiology. (2012) 263:663–72. doi: 10.1148/radiol.12110748
30. Avants BB, Tustison NJ, Song G, Cook PA, Klein A, Gee JC. A reproducible evaluation of ANTs similarity metric performance in brain image registration. Neuroimage. (2011) 54:2033–44. doi: 10.1016/j.neuroimage.2010.09.025
31. Chenevert TL, Malyarenko DI, Newitt D, Li X, Jayatilake M, Tudorica A, et al. Errors in quantitative image analysis due to platform-dependent image scaling. Trans Oncol. (2014) 7:65–71. doi: 10.1593/tlo.13811
32. Tofts PS, Brix G, Buckley DL, Evelhoch JL, Henderson E, Knopp MV, et al. Estimating kinetic parameters from dynamic contrast-enhanced T(1)-weighted MRI of a diffusable tracer: standardized quantities and symbols. J magnetic resonance Imaging JMRI. (1999) 10:223–32. doi: 10.1002/(SICI)1522-2586(199909)10:3<223::AID-JMRI2>3.0.CO;2-S
33. Tofts PS, Benton CE, Weil RS, Tozer DJ, Altmann DR, Jager HR, et al. Quantitative analysis of whole-tumor Gd enhancement histograms predicts Malignant transformation in low-grade gliomas. J magnetic resonance Imaging JMRI. (2007) 25:208–14. doi: 10.1002/jmri.20800
34. Yankeelov TE, Rooney WD, Li X, Springer CS Jr. Variation of the relaxographic "Shutter-speed" for transcytolemmal water exchange affects the CR bolus-tracking curve shape. Magnetic Resonance Med. (2003) 50:1151–69. doi: 10.1002/mrm.10624
35. Li X, Rooney WD, Springer CS Jr. A unified magnetic resonance imaging pharmacokinetic theory: Intravascular and extracellular contrast reagents. Magnetic Resonance Med. (2005) 54:1351–9. doi: 10.1002/mrm.20684
36. Huang W, Tudorica LA, Li X, Thakur SB, Chen Y, Morris EA, et al. Discrimination of benign and Malignant breast lesions by using shutter-speed dynamic contrast-enhanced MR imaging 1. Radiology. (2011) 261:394–403. doi: 10.1148/radiol.11102413
37. Murase K. Efficient method for calculating kinetic parameters using T1-weighted dynamic contrast-enhanced magnetic resonance imaging. Magnetic resonance Med. (2004) 51:858–62. doi: 10.1002/mrm.20022
38. Li X, Huang W, Rooney WD. Signal-to-noise ratio, contrast-to-noise ratio and pharmacokinetic modeling considerations in dynamic contrast-enhanced magnetic resonance imaging. Magnetic Resonance Imaging. (2012) 30:1313–22. doi: 10.1016/j.mri.2012.05.005
39. Li X, Priest RA, Woodward WJ, Siddiqui F, Beer TM, Garzotto MG, et al. Cell membrane water exchange effects in prostate DCE-MRI. J magnetic resonance (San Diego Calif: 1997). (2012) 218:77–85. doi: 10.1016/j.jmr.2012.03.019
40. Sehy JV, Banks AA, Ackerman JJ, Neil JJ. Importance of intracellular water apparent diffusion to the measurement of membrane permeability. Biophys J. (2002) 83:2856–63. doi: 10.1016/S0006-3495(02)75294-6
41. Xu J, Jiang X, Li H, Arlinghaus LR, McKinley ET, Devan SP, et al. Magnetic resonance imaging of mean cell size in human breast tumors. Magn Reson Med. (2020) 83:2002–14. doi: 10.1002/mrm.28056
42. Li X, Mangia S, Lee JH, Bai R, Springer CS Jr. NMR shutter-speed elucidates apparent population inversion of (1) H2 O signals due to active transmembrane water cycling. Magn Reson Med. (2019) 82:411–24. doi: 10.1002/mrm.27725
43. R Core Team. R: A Language and Environment for Statistical Computing. Vienna, Austria: R Foundation for Statistical Computing. (2020)
44. Huang W, Wang Y, Panicek DM, Schwartz LH, Koutcher JA. Feasibility of using limited-population-based average R10 for pharmacokinetic modeling of osteosarcoma dynamic contrast-enhanced magnetic resonance imaging data. Magn Reson Imaging. (2009) 27:852–8. doi: 10.1016/j.mri.2009.01.020
45. Huang W, Li X, Morris EA, Tudorica LA, Seshan VE, Rooney WD, et al. The magnetic resonance shutter speed discriminates vascular properties of Malignant and benign breast tumors in vivo. Proc Natl Acad Sci U S A. (2008) 105:17943–8. doi: 10.1073/pnas.0711226105
46. Zhang Y, Poirier-Quinot M, Springer CS Jr., Balschi JA. Active trans-plasma membrane water cycling in yeast is revealed by NMR. Biophys J. (2011) 101:2833–42. doi: 10.1016/j.bpj.2011.10.035
Keywords: breast cancer, therapy response, dynamic contrast-enhanced (DCE) MRI, pharmacokinetics, Ktrans, water exchange, multi-center, multi-vendor platform
Citation: Moloney B, Li X, Hirano M, Saad Eddin A, Lim JY, Biswas D, Kazerouni AS, Tudorica A, Li I, Bryant ML, Wille C, Pyle C, Rahbar H, Hsieh SK, Rice-Stitt TL, Dintzis SM, Bashir A, Hobbs E, Zimmer A, Specht JM, Phadke S, Fleege N, Holmes JH, Partridge SC and Huang W (2024) Initial experience in implementing quantitative DCE-MRI to predict breast cancer therapy response in a multi-center and multi-vendor platform setting. Front. Oncol. 14:1395502. doi: 10.3389/fonc.2024.1395502
Received: 04 March 2024; Accepted: 28 October 2024;
Published: 29 November 2024.
Edited by:
Maria Evelina Fantacci, University of Pisa, ItalyReviewed by:
Qiao Hu, The People’s Hospital of Guangxi Zhuang Autonomous Region, ChinaCopyright © 2024 Moloney, Li, Hirano, Saad Eddin, Lim, Biswas, Kazerouni, Tudorica, Li, Bryant, Wille, Pyle, Rahbar, Hsieh, Rice-Stitt, Dintzis, Bashir, Hobbs, Zimmer, Specht, Phadke, Fleege, Holmes, Partridge and Huang. This is an open-access article distributed under the terms of the Creative Commons Attribution License (CC BY). The use, distribution or reproduction in other forums is permitted, provided the original author(s) and the copyright owner(s) are credited and that the original publication in this journal is cited, in accordance with accepted academic practice. No use, distribution or reproduction is permitted which does not comply with these terms.
*Correspondence: Wei Huang, aHVhbmd3ZUBvaHN1LmVkdQ==; Xin Li, bHhpbkBvaHN1LmVkdQ==
†Present address: Wei Huang, Department of Radiation Oncology, Corewell Health William Beaumont University Hospital, Royal Oak, MI, United States
Disclaimer: All claims expressed in this article are solely those of the authors and do not necessarily represent those of their affiliated organizations, or those of the publisher, the editors and the reviewers. Any product that may be evaluated in this article or claim that may be made by its manufacturer is not guaranteed or endorsed by the publisher.
Research integrity at Frontiers
Learn more about the work of our research integrity team to safeguard the quality of each article we publish.