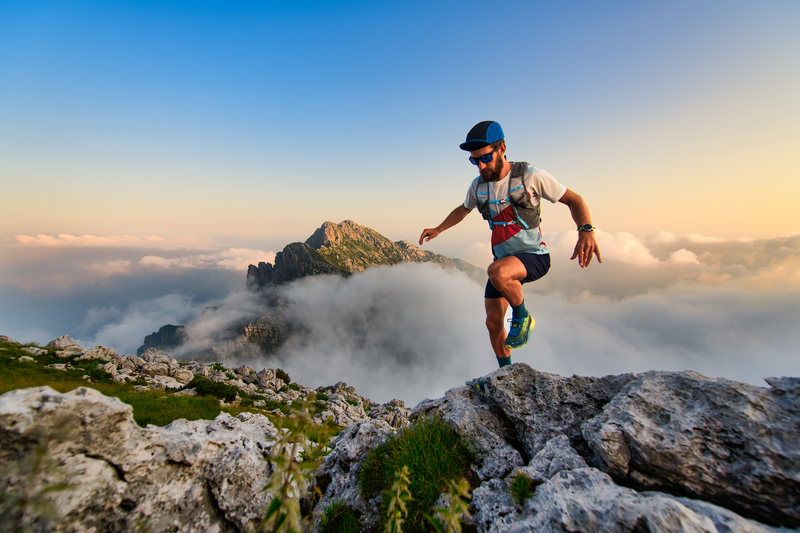
94% of researchers rate our articles as excellent or good
Learn more about the work of our research integrity team to safeguard the quality of each article we publish.
Find out more
ORIGINAL RESEARCH article
Front. Oncol. , 23 March 2023
Sec. Cancer Genetics
Volume 13 - 2023 | https://doi.org/10.3389/fonc.2023.916568
This article is part of the Research Topic Application and Innovation of Multiomics Technologies in Clinical Oncology View all 44 articles
Objective: To investigate the impact that TTN mutation had on the gene heterogeneity expression and prognosis in patients with lung adenocarcinoma.
Methods: In this study, the Cancer Genome Atlas (TCGA) dataset was used to analyze the TTN mutations in lung adenocarcinoma. Lung adenocarcinoma data was collected from the TCGA database, clinical information of patients was analyzed, and bioinformatics statistical methods were applied for mutation analysis and prognosis survival analysis. The results were verified using the GEO dataset.
Results: The incidence of TTN mutations in lung adenocarcinoma was found to be 73%, and it was related to the prognosis of lung adenocarcinoma. Ten genes were screened with significant contributions to prognosis. A prognosis model was constructed and verified by LASSO COX analysis in the TCGA and GEO datasets based on these ten beneficial factors. The independent prognostic factor H2BC9 for TTN mutation-driven gene heterogeneity expression was screened through multi-factor COX regression analysis.
Conclusion: Our data showed that the gene heterogeneity expression, which was driven by TTN mutations, prolonged the survival of lung adenocarcinoma patients and provided valuable clues for the prognosis of TTN gene mutations in lung adenocarcinoma.
Lung cancer remains the leading type of cancer-related death, and gene mutations have a very important impact on prognosis in NSCLC (1). TTN and TP53 are two of the most commonly mutated genes in NSCLC samples from The Cancer Genome Atlas (TCGA) official website. TP53 plays a crucial biological role in the development of various tumors and has received widespread attention (2–4). Co-mutation analyses of TP53 and cancer driver genes in clinical studies can often be used to provide specific molecular typing for molecularly targeted therapies (5). The coding gene TTN, also known as cardiomyopathy dilated 1G, is the longest-reported gene to date (6). TTN has a high mutation rate in several types of tumor tissue (7). However, TTN is controversial as it is considered a tumor-associated gene. This controversy focuses on the large and complex DNA structure and the false-positive results associated with tumor heterogeneity. In addition, the biological function of TTN mutations in the development of cancer has been questioned, as TTN mutations have been strongly associated with cardiac and skeletal muscle diseases but rarely with oncological diseases (6, 8, 9). To date, only a few studies have specifically explored the relevance of TTN to tumors. The complexity of the TTN gene structure compared to TP53 and the aforementioned controversies regarding TTN have greatly hindered researchers from analyzing this gene in depth. To date, studies related to TTN mutations in tumors are rare.
Using the TCGA database, this paper focuses on the correlation between TTN mutations and the clinicopathological characteristics of patients with LUSC and the impact of these mutations on the survival of these patients. It also focuses on the impact of TTN co-mutations on the prognosis survival of these patients to identify the main prognostic factors that affect TTN mutations in LUSC and thus provide new possible treatment targets for the disease. The main prognostic factors affecting TTN mutations in LUSC are SLC34A2, BARX1, and HOXC10. In this study, we elucidated the prognostic impact of heterogeneous gene expression caused by TTN mutations on LUSC and screened the main prognostic factors of heterogeneous gene expression driven by TTN mutations using the least absolute shrinkage and selection operator (LASSO) Cox analysis. The results could provide new possible targets for the prevention and treatment of LUSC.
RNA sequencing data (level 3), expression profile data, and corresponding clinical information were obtained from a TCGA dataset (https://portal.gdc.com) for 488 patients with LUSC. The GSE73403 datasets was from the Gene Expression Omnibus (GEO) database (https://www.ncbi.nlm.nih.gov/geo).
The data of 488 patients with LUSC got from TCGA were analyzed, and somatic mutations in patients with LUSC were visualized using the maftools package in R software.
A univariate analysis of the clinicopathological characteristics was performed using the chi-square test and nonparametric tests. This was followed by screening for statistically significant independent influences associated with TTN mutations using binary logistic regression models. P values < 0.05 were considered significant.
RNAseq and corresponding clinical information of 393 TTN mutations in LUSC were came from TCGA dataset (https://portal.gdc.com). Progression-free survival (PFS), disease-free survival (DFS), and disease-specific survival (DSS) were assessed by overall survival (OS). The impact of TTN mutation on the prognostic survival of LUSC was assessed. Survival analyzes were performed using Cox multiple survival regression model analysis as the primary method, and the Kaplan–Meier (KM) method as an adjunct method. The endpoint of OS was defined as the time from randomization to death from any cause. The endpoint of DFS was defined as the time from the start of the initial treatment to the onset of a new tumor-related event or death from any cause. All the above analysis methods were implemented with the R package. P values < 0.05 were considered significant.
The DESeq2 package of R software was used for expression profiling, differential analysis, gene volcano mapping, and heat mapping. For gene microarray screening, corrected p-values and |log1.5FC| p < 0.05 and |log1.5FC| ≥ 1.0 were calculated for differentially expressed genes. Gene ontology (GO) functional enrichment and Kyoto Encyclopedia of Genes and Genomes (KEGG) pathway analysis enrichment analysis were visualized using the ClusterProfiler package in R software, and a false discovery rate of < 0.01 was considered significant.
The relationship between prognostic immune-related gene expression and OS was analyzed. A prognostic risk prediction model for LUSC was calculated. Patients with LUSC were divided into high-risk and low-risk groups using the median risk score as the cut-off. KM curves were plotted to compare these groups. Receiver operating characteristic (ROC) survival analysis was performed using the R package SURVIVAL, and decision curve analysis was performed using the rmda package. The association between risk score models and tumor immune-infiltrating cells was also investigated using Spearman correlation analysis, and statistical significance was set to P < 0.05.
To make sure the frequency of mutations in genes in LUSC, we imported data from the TCGA database for analysis. A total of 488 patients with LUSC were imported, containing 478 samples with detected mutations, of which the mapping samples contained a total of 478 (97.75%). The results showed that the TTN mutation rate was 73.00% (Figures 1A, B).
Figure 1 Downloading and visualizing somatic mutations in patients with LUSC using the maftools package in R software for TCGA data. (A) Lollipop plot of TTN gene mutation distribution with 73% mutation rate in somatic cells. (B) Somatic cell landscape of LUSC patient cohort with genes sorted by mutation frequency and samples sorted by disease histology; sidebar plot showing log10 transformed Q-values estimated by MutSigCV. The waterfall plot shows mutation information for each gene in each sample.
Subsequently, our univariate analysis of TTN gene mutations and clinicopathological characteristics showed that survival status, pN stage, smoking history, and history of neoadjuvant treatment were associated with TTN mutations, while gender, age, pathological stage, new tumor event type, and radiation/chemotherapy were not associated with TTN mutations in LUSC (Table 1).
There were 211 deaths among 489 patients with LUSC (Table 1); according to the Cox proportional risk regression model, TTN mutation was associated with longer OS (multivariate Cox model P=0.000212, HR=0.547 [95% CI, 0.398–0.753], Figure 2A), PFS (multivariate Cox model P=0.0323, HR=0.649 [95% CI, 0.437–0.964], Figure 2B), and DSS (multivariate Cox model P=0.0181, HR=0.555 [95% CI, 0.341–0.904], Figure 2D), TTN mutations was not associated with DFS (Figure 2C). Therefore, it can be concluded that TTN mutations are associated with survival in LUSC.
Figure 2 Analysis of the effect of TTN mutation on prognostic survival in LUSC using the Cox regression model. (A) Overall survival (OS). (B) Progression-free survival (PFS). (C) Disease-free survival (DFS). (D) disease-specific survival (DSS). HR represents the risk coefficient of the high-expression group relative to the samples in the low-expression group; 95% CI represents the HR confidence interval; Median time represents the survival rate of the two groups (high-expression group and low-expression group) at 50% (i.e., median survival time) corresponding to the time in years; and the test between the two groups was performed by log-rank and was statistically significant at P < 0.05.
To determine the role of gene expression heterogeneity driven by TTN mutations in LUSC in the construction of prognostic models, we screened differentially expressed genes (DEGs) in 393 LUSC TTN mutant tissues and 96 LUSC tissues in TCGA. A total of 50 up-regulated genes and 7 down-regulated genes were screened, and the volcano are shown in Figures 3A, B showed the gene expression heat map of each sample, respectively. For DEGs, GO function enrichment and KEGG differential analyzes were performed on DEGs (Figure 3C).
Figure 3 DEGs screening. (A) Volcano plot. Red dots represent significantly differentially upregulated genes, blue dots represent significantly differentially downregulated genes, and gray dots represent insignificant genes. (B) Heat map of the top 50 significantly DEGs. Different colors represent expression trends in different tissues. (C) GO functional enrichment and KEGG pathway analysis of upregulated genes.
To further discuss the main genes that prolong the survival of lung adenocarcinoma patients with TTN mutations, 57 significant differential genes were analyzed for their correlation with the prognosis of lung adenocarcinoma patients, and 10 significant differential genes with prognostic differences were obtained. Among them, low expression of C12orf56, DLX5, DSC3, FEZF1, GSTA1, H2BC9, IGSF11 and high expression of EREG, CEACAM6, SLC34A2 in lung adenocarcinoma were found to predict poor prognosis for patients (Figure 4A). The results of differential expression analysis showed that C12orf56, DLX5, DSC3, FEZF1, GSTA1, H2BC9, IGSF11 were highly expressed in TTN mutation patients, and EREG, CEACAM6, SLC34A2 were lowly expressed in TTN mutation patients (Figure 4B). These data indicate that these 10 significant differential expression prognostic genes are beneficial factors for the prognosis and survival of patients with prolonged TTN mutations.
Figure 4 Figure 4 was shown to present the expression of ten beneficial factors and their impact on patient prognosis. (A) The high or low expression of the ten beneficial factors (C12orf56, DLX5, DSC3, FEZF1, GSTA1, H2BC9, IGSF11, EREG, CEACAM6, SLC34A2) was demonstrated to have an impact on the prognosis of lung squamous cell carcinoma patients. (B) The expression of the ten beneficial factors (C12orf56, DLX5, DSC3, FEZF1, GSTA1, H2BC9, IGSF11, EREG, CEACAM6, SLC34A2) in patients with TTN mutations and wild-type TTN patients was shown to be presented. t test was used for comparison between groups, with * indicating p<0.05, ** indicating p<0.01, and *** indicating p<0.001.
Subsequently, a prognostic feature was established based on the 10 beneficial factors using LASSO Cox analysis (Figures 5A, B). The lung adenocarcinoma patients were divided into high-risk and low-risk groups using the risk score Riskscore = (-4e-04)*DLX5 + (-0.0772)*FEZF1 + (-0.0933)*H2BC9 + (0.0637)*EREG + (0.0298)*SLC34A2 (Figure 5C), and their survival status was displayed in Figure 5D. The difference in survival between the high-risk and low-risk groups was shown in Figure 5E (P=0.00619). Prognostic survival prediction was performed for 1 year, 3 years, and 5 years, and the prognostic model showed good sensitivity and specificity (Figure 5F).
Figure 5 Construction of prognostic features of TTN mutation DEGs in LUSC. (A, B) Distribution of LASSO coefficients of DEGs to obtain the adjustment parameter. λ.min = 0.0329, the vertical black dashed line in B defines the optimal λ value. (C) Distribution of risk score of TTN mutation in LUSC. (D) Survival status and duration of TTN mutation in LUSC patients. (E) KM survival curves for high- and low-risk groups. (F) 1-year, 3-year, and 5-year ROC curves.
To validate the accuracy of our screened independent prognostic factors, we constructed risk prognosis models in GSE73403 datasets and obtained. RiskScore=(-0.61828)*IGSF11+(-0.13167)*DLX5+(0.31607)*EREG+(-0.41127)*SLC34A2 risk assessment models (Figures 6A, B), which divided patients into two groups of high risk and low risk (Figures 6C, D), with significant differences in prognosis (Figure 6E). Prognostic survival was predicted for 1, 3, and 5 years, and this prognostic model showed preferably sensitivity and specificity (Figure 6F).
Figure 6 GEO independent dataset to validate the risk model. (A, B) Distribution of LASSO coefficients in the GSE73403 datasets, obtained by adjusting the parameters λ.min = 0.0146. (C, D) Distribution of risk scores of the constructed models for the GSE73403 datasets. (E) KM survival curves for the high-and low-risk groups. (F) 1-year, 3-year, and 5-year ROC curves.
To determine the prognostic factors of TTN mutations on OS in LUSC, we performed univariate and multivariate Cox regression analysis of prognostic correlates, and the results are shown in Figures 7A, B. In the univariate Cox regression analysis, DLX5, FEZF1, H2BC9, EREG, SLC34A2, pT-stage and pTNM-stage were significantly correlated with the OS of the TTN mutations in LUSC patients (Figure 6A). Then, DLX5, FEZF1, H2BC9, EREG, SLC34A2, pT-stage and pTNM-stage were analyzed to determine the independent prognostic factors based on a regression analysis of TTN mutations in patients with LUSC by multivariateial Cox analysis. H2BC9 was found to be significantly associated with the OS of the TTN mutation in the patients (Figure 7B). All independent factors were combined to create nomogram prediction plots predicting 1-, 3-, and 5-year OS (Figures 7C, D).
Figure 7 Univariate and multivariate Cox screening prognostic factors. (A, B) Analysis of clinical factors and risk scores using univariate Cox analysis, and multivariate Cox analysis was used to analyze significant factors. (C) Construction of clinical diagnosis column line graphs based on clinical characteristics and risk scores. (D) Calibration plots for predicting recurrence at 1, 3, and 5 years. The x-axis indicates the recurrence probability predicted from the column line graphs, and the y-axis indicates the actual recurrence probability.
Lung carcinogenesis and progression are complex biological processes with multi-gene involvement and multiple steps. Few driver mutations have been identified in LUSC that can be used as drug targets, and, unlike with lung adenocarcinoma, the process of development does not depend on classical driver mutations, but more on epigenetic alterations (10–12). Therefore, because of its unique molecular pathology, LUSC cannot benefit significantly from molecularly targeted therapies as lung adenocarcinoma can. The TCGA database maps a large number of human tumor genomic variants through genomic analysis techniques. By mining this database with large samples of data, valuable research subjects can be derived and further targeted studies can be conducted. This database can provide a theoretical basis for clinical treatment. In LUSC, exploring the molecular-level determinants of the immunotherapeutic response is challenging, and many studies are underway to address this issue. The results of the TCGA study of mutations in the genome of 178 LUSC patients revealed potential therapeutic targets mainly associated with PI3K/AKT signaling pathways and signaling pathways mediated by receptor tyrosine kinases (RTKs), including PIK3CA mutations (16% of cases); FGFR amplification or mutations (18% of cases); PTEN mutations or deletions (15% of cases); EGFR amplification and PDGFRA amplification or mutation (9% each); and ERBB2 amplification, DDR2 mutation, and BRAF mutation (4% each) (13).
In recent years, it has been shown that single or double mutations in TP53 and TTN, two genes with high mutation frequency in NSCLC, have different prognostic effects, and TTN gene mutations were significantly associated with LUSC (14). Meanwhile, a study analyzed somatic mutations in LUSC samples based on datasets obtained from TCGA and the International Cancer Genome Consortium (ICGC) database and found that TTN mutations were associated with tumor mutational burden (TMB) and positively correlated with LUSC prognosis (15). And in a study by Xie et al. (16), TTN with mutations in higher TMB was found to be associated with prognostic outcome, and TTN mutations were found to enhance anti-tumor immune responses according to the CIBERSORT algorithm. In the above study, the prognostic impact of TTN mutation or double mutation with TP53 on LUSC was systematically described, and the relationship between TTN mutated genes and TMB was analyzed based on TCGA and ICGC databases. In our study, we investigated the prognostic impact of heterogeneous expression of genes due to TTN mutations on LUSC from the TCGA database and screened for their independent prognostic factors.
In our study, it was found that the prolonged survival of lung squamous cell carcinoma patients was caused by TTN mutation. Based on this, the heterogeneous expression of genes driven by TTN mutation was analyzed, and 57 differential genes were identified. After performing a prognostic analysis of the differential genes combined with their expression in TTN wild-type and TTN mutation patients, 10 genes were found to have contributed significantly to the prognostic potential of prolonged survival. A prognostic model was then constructed based on these 10 prognostic beneficial factors, and a prognostic model with DLX5, FEZF1, H2BC9, EREG, and SLC34A2 as the main factors was obtained.
SLC34A2 can be highly expressed in the lung (17, 18). In human lungs, SLC34A2 is expressed only in type II alveolar epithelial cells (AT-II) and is required for the synthesis of AT-II lung surfactant (19). AT-II cells may transform into cancer stem cells in response to exogenous or endogenous factors and eventually induce oncogenic effects and NSCLC carcinogenesis (20–22). SLC34A2 may have a physiological function in AT-II, and its mutation or abnormal expression will certainly affect the normal function of AT-II, which is associated with lung tumorigenesis. Also, recent studies have reported that SLC34A2 plays a key role in lung cancer. SLC34A2 expression was increased during fetal lung development and early embryonic development, but it was decreased in NSCLC tissues compared with surrounding normal lung tissue (23). In addition, studies have reported that SLC34A2 may inhibit the growth, invasion, and migration of lung cancer cells through PI3K/Akt and Ras/Raf/MEK signaling pathways, by acting as a tumor suppressor (23, 24). In some cancers, SLC34A2 is considered a potential prognostic marker of tumor disease. SLC34A2 mutations in breast and thymic tumors reduce the life expectancy of patients, and SLC34A2 overexpression was found to reduce the life expectancy of patients with brain tumors, ovarian tumors, and pancreatic tumors (25). High SLC34A2 expression was present in approximately 75% of lung adenocarcinoma samples and was associated with significantly improved overall patient survival (26). A recent study showed that SLC34A2 (hazard ratio: 0.86, P = 0.03, coefficient = -0.1551) could be a potential prognostic immune-related gene by validating the characteristics of immune infiltration analysis in patients with NSCLC and its correlation with survival outcomes (27). It has been revealed by other studies that the high expression of EREG in lung adenocarcinoma is detrimental to the survival of individuals (28). In gastric cancer, it was found that the EREG mRNA expression was an independent predictor of poor survival, and that the overall survival was significantly reduced in patients with gastric cancer with high expression (29). DLX homeobox genes, which belong to the NK family, play a dual role in development and cancer (30). In human ovarian cancer cells, it has been found that DLX5 is essential for regulating AKT signaling, thereby promoting cell proliferation and survival. FEZF1, which is a highly conserved transcription factor belonging to the C2H2 zinc finger protein family, has been shown to reduce cell proliferation and migration in human cervical cancer cell lines when it is knockdown and to act as an independent predictor of cervical cancer recurrence (31). H2BC9, which belongs to the H2B aggregator histone gene family, is commonly recognized as a molecular biomarker and an independent prognostic factor for H2BC9 cervical cancer in cervical cancer and glioma (32, 33).
In conclusion, a study was conducted in which the gene mutations in lung squamous cell carcinoma with TTN mutations were analyzed. Ten prognostic beneficial factors of the heterogeneous expression of lung squamous cell carcinoma genes driven by TTN mutations were screened for and DLX5, FEZF1, H2BC9, EREG, and SLC34A2 were identified as the main factors of the prognostic models. These factors were validated on external data sets. However, it was noted that a public database was used for the analysis and the screened prognostic factors were not experimentally validated. Additionally, data from a large clinical cohort was lacking for testing. It was planned that in a follow-up study, data collected from multiple cases would be analyzed, the correlation of TTN mutation-driven gene heterogeneous expression would be validated based on clinical trials, and the mechanism of TTN mutation regulation of gene expression would be investigated through cellular assays.
In conclusion, in our study, we identified SLC34A2, BARX1, and HOXC10 as the major prognosis-related genes by constructing a prognostic model constructed by the differential expression of TTN mutation-driven genes through bioinformatics analysis. These findings could provide prognostic biomarkers for LUSC and possible new targets for its clinical treatment.
The datasets presented in this study can be found in online repositories. The names of the repository/repositories and accession number(s) can be found in the article/supplementary material.
YZ, GZ, XP and CZ designed the study. ZL, XZ and XT completed the analysis. ZL and XT wrote the manuscript. RW proofread the manuscript and put forward constructive opinions, and all the authors have read and approved the submission of the manuscript. All authors contributed to the article and approved the submitted version.
ZL, XT and YZ were employed by Biotrans Technology Co., LTD.
The remaining authors declare that the research was conducted in the absence of any commercial or financial relationships that could be construed as a potential conflict of interest.
All claims expressed in this article are solely those of the authors and do not necessarily represent those of their affiliated organizations, or those of the publisher, the editors and the reviewers. Any product that may be evaluated in this article, or claim that may be made by its manufacturer, is not guaranteed or endorsed by the publisher.
1. Bray F, Ferlay F, Soerjomataram I, Siegel SL, Torre LA, Jemal A. Global cancer statistics 2018: GLOBOCAN estimates of incidence and mortality worldwide for 36 cancers in 185 countries. CA Cancer J Clin (2018) 68(6):394–424.
2. Harrison PT, Vyse S, Huang PH. Rare epidermal growth factor receptor (EGFR) mutations in non-small cell lung cancer. Semin Cancer Biol (2020) 61:167–79. doi: 10.1016/j.semcancer.2019.09.015
3. Yang C, Huang X, Li Y, Chen J, Lv Y, Dai S. Prognosis and personalized treatment prediction in TP53-mutant hepatocellular carcinoma: An in silico strategy towards precision oncology. Brief Bioinform (2021) 22(3):bbaa164. doi: 10.1093/bib/bbaa164
4. Duffy MJ, Synnott NC, O'Grady S, Crown J. Targeting p53 for the treatment of cancer. Semin Cancer Biol (2022) 79:58–67. doi: 10.1016/j.semcancer.2020.07.005
5. VanderLaan PA, Rangachari D, Mockus SM, Spotlow V, Reddi HV, Malcolm J, et al. Mutations in TP53, PIK3CA, PTEN and other genes in EGFR mutated lung cancers: Correlation with clinical outcomes. Lung Cancer (2017) 106:17–21. doi: 10.1016/j.lungcan.2017.01.011
6. Chauveau C, Rowell J, Ferreiro A. A rising titan: TTN review and mutation update. Hum Mutat (2014) 35(9):1046–59. doi: 10.1002/humu.22611
7. Kim N, Hong Y, Kwon D, Yoon S. Somatic mutaome profile in human cancer tissues. Genomics Inform (2013) 11(4):239–44. doi: 10.5808/GI.2013.11.4.239
8. Rees M, Nikoopour R, Fukuzawa A, Kho AL, Fernandez-Garcia MA, Wraige E, et al. Making sense of missense variants in TTN-related congenital myopathies. Acta Neuropathol (2021) 141(3):431–53. doi: 10.1007/s00401-020-02257-0
9. Tharp CA, Haywood ME, Sbaizero O, Taylor MRG, Mestroni L. The giant protein titin's role in cardiomyopathy: Genetic, transcriptional, and post-translational modifications of TTN and their contribution to cardiac disease. Front Physiol (2019) 10:1436. doi: 10.3389/fphys.2019.01436
10. Wang BY, Huang JY, Chen HC, Lin CH, Lin SH, Hung WH, et al. The comparison between adenocarcinoma and squamous cell carcinoma in lung cancer patients. J Cancer Res Clin Oncol (2020) 146(1):43–52. doi: 10.1007/s00432-019-03079-8
11. Ruiz-Cordero R, Devine WP. Targeted therapy and checkpoint immunotherapy in lung cancer. Surg Pathol Clin (2020) 13(1):17–33. doi: 10.1016/j.path.2019.11.002
12. Zeng Z, Yang F, Wang Y, Zhao H, Wei F, Zhang P, et al. Significantly different immunological score in lung adenocarcinoma and squamous cell carcinoma and a proposal for a new immune staging system. Oncoimmunology (2020) 9(1):1828538. doi: 10.1080/2162402X.2020.1828538
13. Rooney M, Devarakonda S, Govindan R. Genomics of squamous cell lung cancer. Oncologist (2013) 18(6):707–16. doi: 10.1634/theoncologist.2013-0063
14. Cheng X, Yin H, Fu J, Chen C, An J, Guan J, et al. Aggregate analysis based on TCGA: TTN missense mutation correlates with favorable prognosis in lung squamous cell carcinoma. J Cancer Res Clin Oncol (2019) 145(4):1027–35. doi: 10.1007/s00432-019-02861-y
15. Zou S, Ye J, Hu S, Wei Y, Xu J. Mutations in the TTN gene are a prognostic factor for patients with lung squamous cell carcinomas. Int J Gen Med (2022) 15:19–31. doi: 10.2147/IJGM.S343259
16. Xie X, Tang Y, Sheng J, Shu P, Zhu X, Cai X, et al. Titin mutation is associated with tumor mutation burden and promotes antitumor immunity in lung squamous cell carcinoma. Front Cell Dev Biol (2021) 9:761758. doi: 10.3389/fcell.2021.761758
17. Wagner CA, Hernando N, Forster IC, Biber J. The SLC34 family of sodium-dependent phosphate transporters. Pflugers Arch (2014) 466(1):139–53. doi: 10.1007/s00424-013-1418-6
18. Takeda E, Yamamoto H, Nashiki K, Sato T, Arai H, Taketani Y. Inorganic phosphate homeostasis and the role of dietary phosphorus. J Cell Mol Med (2004) 8(2):191–200. doi: 10.1111/j.1582-4934.2004.tb00274.x
19. Hashimoto M, Wang DY, Kamo T, Zhu Y, Tsujiuchi T, Konishi Y, et al. Isolation and localization of type IIb Na/Pi cotransporter in the developing rat lung. Am J Pathol (2000) 157(1):21–7. doi: 10.1016/S0002-9440(10)64512-9
20. Jacob A, Vedaie M, Roberts DA, Thomas DC, Villacorta-Martin C, Alysandratos KD, et al. Derivation of self-renewing lung alveolar epithelial type II cells from human pluripotent stem cells. Nat Protoc (2019) 14(12):3303–32. doi: 10.1038/s41596-019-0220-0
21. Desai TJ, Brownfield DG, Krasnow MA. Alveolar progenitor and stem cells in lung development, renewal and cancer. Nature (2014) 507(7491):190–4. doi: 10.1038/nature12930
22. Cho HC, Lai CY, Shao LE, Yu J. Identification of tumorigenic cells in Kras(G12D)-induced lung adenocarcinoma. Cancer Res (2011) 71(23):7250–8. doi: 10.1158/0008-5472
23. Kopantzev EP, Monastyrskaya GS, Vinogradova TV, Zinovyeva MV, Kostina MB, Filyukova OB, et al. Differences in gene expression levels between early and later stages of human lung development are opposite to those between normal lung tissue and non-small lung cell carcinoma. Lung Cancer (2008) 62(1):23–34. doi: 10.1016/j.lungcan.2008.02.011
24. Wang Y, Yang W, Pu Q, Yang Y, Ye S, Ma Q, et al. The effects and mechanisms of SLC34A2 in tumorigenesis and progression of human non-small cell lung cancer. J BioMed Sci (2015) 22(1):52. doi: 10.1186/s12929-015-0158-7
25. Vlasenkova R, Nurgalieva A, Akberova N, Bogdanov M, Kiyamova R. Characterization of SLC34A2 as a potential prognostic marker of oncological diseases. Biomolecules (2021) 11(12):1878. doi: 10.3390/biom11121878
26. Zhang Z, Ye S, Zhang M, Wu J, Yan H, Li X, et al. High expression of SLC34A2 is a favorable prognostic marker in lung adenocarcinoma patients. Tumour Biol (2017) 39(7):1010428317720212. doi: 10.1177/1010428317720212
27. Mi K, Chen F, Qian Z, Chen J, Lv D, Zhang C, et al. Characterizing heterogeneity of non-small cell lung tumour microenvironment to identify signature prognostic genes. J Cell Mol Med (2020) 24(24):14608–18. doi: 10.1111/jcmm.16092
28. Wang W, Xu C, Ren Y, Wang S, Liao C, Fu X, et al. A novel cancer stemness-related signature for predicting prognosis in patients with colon adenocarcinoma. Stem Cells Int (2021) 2021:7036059. doi: 10.1155/2021/7036059
29. Suematsu H, Hashimoto I, Hiroshima Y, Watanabe H, Kano K, Takahashi K, et al. Clinical significance of EREG gene expression in gastric cancer tissue after curative surgery. Anticancer Res (2022) 42(8):3873–8. doi: 10.21873/anticanres.15880
30. Tan Y, Testa JR. DLX Genes: Roles in development and cancer. Cancers (Basel) (2021) 13(12):3005.
31. Lan Y, Xiao X, Luo Y, He Z, Song X. FEZF1 is an independent predictive factor for recurrence and promotes cell proliferation and migration in cervical cancer. J Cancer (2018) 9(21):3929–38. doi: 10.7150/jca.26073
32. Li X, Tian R, Gao H, Yang Y, Williams BRG, Gantier MP, et al. Identification of a histone family gene signature for predicting the prognosis of cervical cancer patients. Sci Rep (2017) 7(1):16495. doi: 10.1038/s41598-017-16472-5
Keywords: bioinformatics, lung squamous carcinoma, TTN, gene mutation, prognostic survival
Citation: Liu Z, Zhao X, Wang R, Tang X, Zhao Y, Zhong G, Peng X and Zhang C (2023) Heterogeneous pattern of gene expression driven by TTN mutation is involved in the construction of a prognosis model of lung squamous cell carcinoma. Front. Oncol. 13:916568. doi: 10.3389/fonc.2023.916568
Received: 09 April 2022; Accepted: 09 February 2023;
Published: 23 March 2023.
Edited by:
Xinmin Li, University of California, Los Angeles, United StatesReviewed by:
Jianyu Rao, University of California, Los Angeles, United StatesCopyright © 2023 Liu, Zhao, Wang, Tang, Zhao, Zhong, Peng and Zhang. This is an open-access article distributed under the terms of the Creative Commons Attribution License (CC BY). The use, distribution or reproduction in other forums is permitted, provided the original author(s) and the copyright owner(s) are credited and that the original publication in this journal is cited, in accordance with accepted academic practice. No use, distribution or reproduction is permitted which does not comply with these terms.
*Correspondence: Guanghui Zhong, emdoMjAwNDA3MTJAMTI2LmNvbQ==; Xin Peng, cGVuZ3hAbml0LnpqdS5lZHUuY24=; Chunlin Zhang, cWR6Y2wyMDExQDE2My5jb20=
Disclaimer: All claims expressed in this article are solely those of the authors and do not necessarily represent those of their affiliated organizations, or those of the publisher, the editors and the reviewers. Any product that may be evaluated in this article or claim that may be made by its manufacturer is not guaranteed or endorsed by the publisher.
Research integrity at Frontiers
Learn more about the work of our research integrity team to safeguard the quality of each article we publish.