- 1Center of Uterine Cancer Diagnosis & Therapy Research of Zhejiang Province, Department of Obstetrics and Gynecology, The Second Affiliated Hospital of Wenzhou Medical University, Wenzhou, Zhejiang, China
- 2Department of Obstetrics and Gynecology, Taizhou Women and Children’s Hospital of Wenzhou Medical University, Taizhou, Zhejiang, China
Purpose: Aimed to identify the anti-uterine corpus endometrial carcinoma (UCEC) function and characterize the mechanism of quercetin in the treatment of patients infected with COVID-19 via integrated in silico analysis.
Methods: The Cancer Genome Atlas and Genotype Tissue Expression databases were applied to obtain differentially expressed genes of UCEC and non-tumor tissue. Several in silico methods such as network pharmacology, functional enrichment analysis, Cox regression analyses, somatic mutation analysis, immune infiltration and molecular docking were used to investigate and analysis the biological targets, functions and mechanisms of anti-UCEC/COVID-19 of quercetin. Multiple methods such as CCK8 assay, Transwell assay and western blotting were performed to test proliferation, migration, and protein level of UCEC (HEC-1 and Ishikawa) cells.
Results: Functional analysis disclosed that quercetin against UCEC/COVID-19 mainly by ‘biological regulation’, ‘response to stimulus’, and ‘regulation of cellular process’. Then, regression analyses indicated that 9 prognostic genes (including ANPEP, OAS1, SCGB1A1, HLA‐A, NPPB, FGB, CCL2, TLR4, and SERPINE1) might play important roles in quercetin for treating UCEC/COVID-19. Molecular docking analysis revealed that the protein products of 9 prognostic genes were the important anti-UCEC/COVID-19 biological targets of quercetin. Meanwhile, the proliferation and migration of UCEC cells were inhibited by quercetin. Moreover, after treatment with quercetin, the protein level of ubiquitination-related gene ISG15 was decreased in UCEC cells in vitro.
Conclusions: Taken together, this study provides new treatment option for UCEC patients infected with COVID-19. Quercetin may work by reducing the expression of ISG15 and participating in ubiquitination-related pathways.
1 Introduction
Severe acute respiratory syndrome coronavirus 2 (SARS-CoV-2) is a rapidly emerging global virus which leads to coronavirus disease in 2019 (COVID-19). The search for COVID-19-fighting drugs has been a long and arduous one for researchers. Despite promising results from in vitro trials, the antimalarial drug hydroxychloroquine couldn’t prevent symptomatic infection caused by SARS-CoV-2 (1). Similarly, a recent report found amubarvimab plus romlusevimab showed no effectiveness in improving clinical outcomes for hospitalized COVID-19 patients (2). Although nonsteroidal anti-inflammatory drugs could provide partial relief, they may increase the risk of bacterial infection (3). Therefore, it is still imperative to continue the search for drugs treating COVID-19.
Uterine corpus endometrial carcinoma (UCEC) is the most common gynecologic cancer in the high-income countries. In 2021, the numbers of new cases and deaths in the United States were approximately at 66,570 and 12,940, respectively (4). A recent study found that severe events were more likely to occur in cancer patients with COVID-19, compared with those who without cancer in China (5). During the hospitalization of COVID-19 patients, the 30-day mortality was 30% for cancer patients (6) and 21% for non-cancer patients (7). Cancer patients were more susceptible to COVID-19 (8). Theoretically, patients with UCEC are at high risk for COVID-19 infection. The prevalence of COVID-19 in UCEC patients will have a great possibility to diminish patient treatment outcomes and affect prognosis. Consequently, it is necessary to screen and develop medicine for anti-UCEC/COVID-19.
Quercetin is a natural compound finding in flowers, leaves, and seeds of plants. Lin et al. (9) showed that quercetin had strong virus inhibition and anti-inflammatory activity. Quercetin may intervene in events associated with cancer development and progression by promoting apoptosis, inhibiting cell proliferation and cancer-related inflammation (10). Our previous study found quercetin could enhance the effectiveness of cisplatin in cervical cancer (11). Additionally, it was found that a reduction in UCEC risk with quercetin intake (12). Unfortunately, the molecular mechanisms of quercetin on UCEC remains poorly understood.
Dysregulation of ubiquitination leads to uncontrolled cell proliferation and aberrant changes of suppressor or oncogene expression (13). ISG15, the first discovered ubiquitin-like protein, was identified to contribute to cancer progression through regulating the ubiquitination (14). Zhao et al. (15) found ISG15, which related to poor prognosis, was observed overexpression in UCEC. Interestingly, quercetin treatment was reported to modulate ISG15 expression (16).
Network pharmacology analyzes the link between drugs, targets, and diseases in the same network. For example, Zhang et al. (17) discovered 76 active ingredients of Xihuang pill through network analysis and identified genes and pathways associated with the triple-negative Breast Cancer. Liu et al. (18) screened 8 active ingredients in honeysuckle associated with acute lung injury by constructing a network of ingredients and targets. Furthermore, our previous study found the common targets between drugs for the treatment of cervical cancer through synergistic action (11). These existing studies suggest that it will be possible to explore quercetin for treating UCEC and COVID-19 with the use of network pharmacology. By network pharmacology analysis, this work investigated quercetin’s targets for treating UCEC/COVID-19 and elucidated its mechanisms. Our study provides a theoretical basis for the treatment of quercetin in UCEC/COVID-19 patients. Figure 1 illustrates the process.
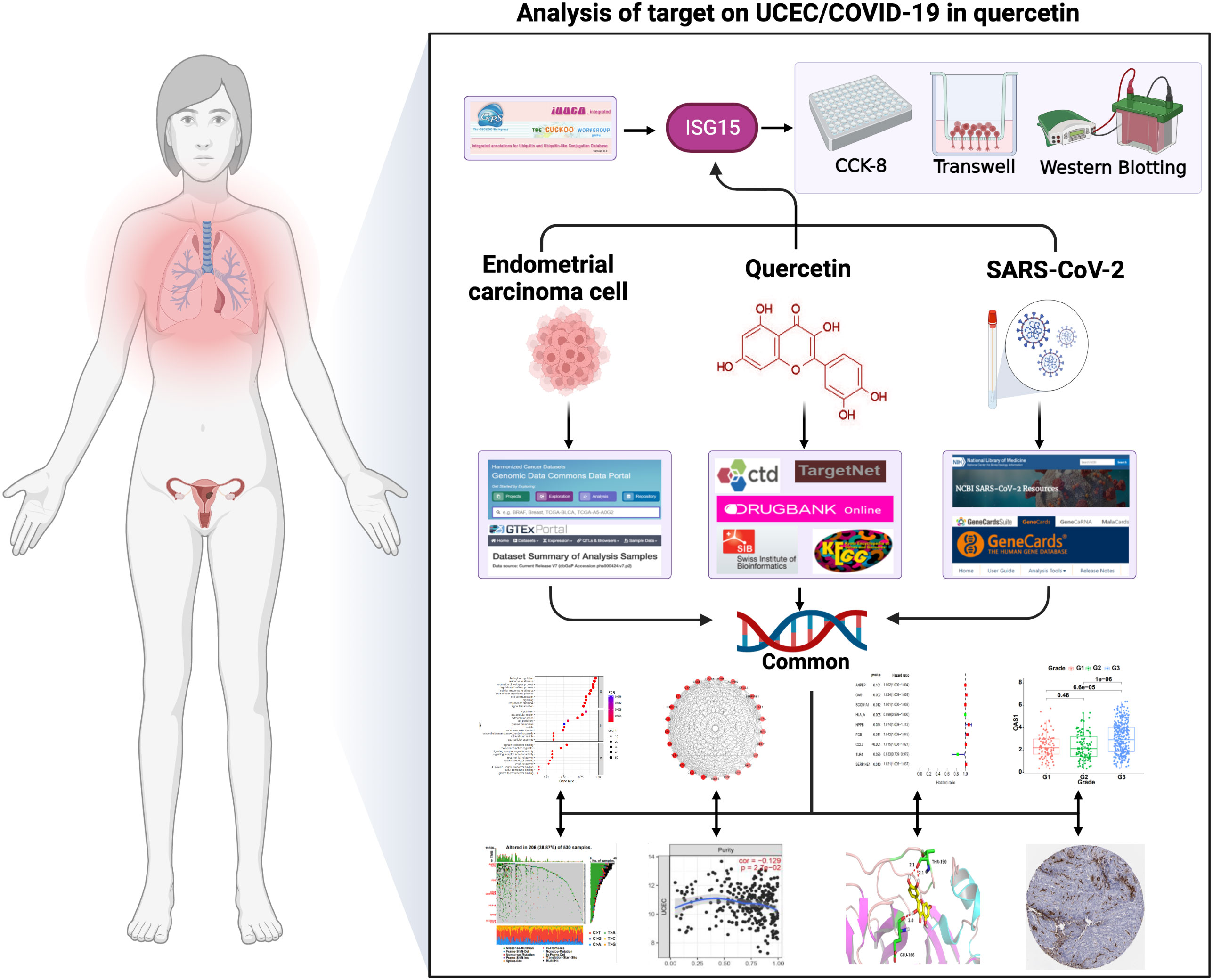
Figure 1 The graphical abstract of this article. The figure represents the antiviral action and mechanism of quercetin against UCEC/COVID-19 using the network pharmacology and computational bioinformatics analysis approach.
2 Materials and methods
2.1 Collecting data
The RNA expression profiles, somatic mutation data, and clinical information were downloaded from The Cancer Genome Atlas (TCGA-UCEC cohort). The dataset included 552 UCEC samples and 35 paracancer tissues. Data with missing clinical information were excluded from the corresponding analyses. As a supplement, RNA expression profiles of 142 normal uterus samples from Genotype Tissue Expression (GTEx) were also downloaded.
2.2 Identification of UCEC DEGs and COVID-19 related genes
After removing batch effects, differentially expressed genes (DEGs) of UCEC samples and non-tumor tissues were screened by using ‘DEseq2’ package in R language. Additionally, NCBI SARS-CoV-2 Data and Genecards were searched to find COVID-19 related genes (CRGs). Then, the overlapping genes were obtained by comparing the UCEC-DEGs with CRGs.
2.3 Acquisition of quercetin targets in UCEC/COVID-19
Five online databases, including Comparative Toxicogenomics Database (CTD), SwissTargetPrediction, DrugBank, Kyoto Encyclopedia of Genes and Genomes (KEGG), and TargetNet were used to identify quercetin targets. After deduplication, the common genes among targets of quercetin, UCEC-DEGs, and CRGs were identified as the main targets of quercetin for anti-UCEC/COVID-19.
2.4 Construction of protein-protein interaction (PPI) network
In order to construct the interaction network, PPI prediction was performed by the STRING. Then, the PPI network was evaluated and visualized using Cytoscape (v3.6.1) (19) software. As shown in Supplementary Table 1, the MCODE algorithm in Cytoscape was further used to identify the hub genes.
2.5 Functional enrichment analyses
In order to investigate the biological function of therapeutic targets of quercetin on UCEC/COVID-19, Gene Ontology (GO) and KEGG pathway analyses were performed. GO enrichment analysis consists of three parts: molecular functional analysis (MF), biological process analysis (BP), and cellular component analysis (CC). KEGG analysis helps us to discover the potential disease-specific pathways. In this study, the genes were entered into the g:Profiler online site to obtain the results of GO and KEGG analyses.
2.6 Clinical analysis of core targets for quercetin and UCEC/COVID-19
Univariate Cox analysis was conducted to evaluate the relationship between each quercetin target in UCEC/COVID-19 and survival status of UCEC patients. Subsequently, the obtained genes were analyzed by multivariate Cox analysis. For each patient, there is a formula for calculating their risk score. (Coef: coefficients, Gene: expression level of gene). The UCEC patients were classified into two risk groups based on the prognostic risk score at the median. Moreover, the overall survival (OS) time of two subgroups were compared utilizing the R packages ‘survival’. The ROC analysis was conducted to test the sensitivity and specificity of prognostic signatures using the ‘timeROC’ package. Additionally, we analyzed the relationship between risk scores and different clinical characteristics, such as age (≤ 65 and > 65), pathological grade, and clinical stage.
2.7 Somatic variation, immune infiltration, and protein level analysis of risk signatures
First, somatic mutations of the risk signatures in UCEC were analyzed and visualized by ‘maftools’ package. Then, TIMER database was performed to evaluate the relationship between infiltration of immune cell and risk signatures through xcell algorithm. In order to explore the different protein levels in UCEC tissue and normal endometrial tissue, immunohistochemistry staining images were acquired from Human Protein Atlas (HPA).
2.8 Molecular docking analysis
As a critical technique of network pharmacology, molecular docking could predict the binding activity of proteins and molecule compounds (20). Through Protein Data Bank (PDB) database, crystal structure of SARS-CoV-2 main protease (PDB code: 7BQY) was obtained. The Grid Box parameter in AutoDockTools was set to include all receptor areas. AutoDock Vina was used to proceed molecular docking. Docking result between quercetin and 7BQY was visualized by PyMOL (21) software.
2.9 Identification of ubiquitination related genes (URGs) in quercetin and UCEC/COVID-19
URGs were obtained from IUUCD database. Then, the intersecting genes were obtained by comparing the URGs and the PPI hub genes.
2.10 Cell culture
UCEC cells (HEC-1 and Ishikawa) were obtained from the Cell Bank of the Chinese Academy of Sciences (Shanghai, China). HEC-1 cells were cultured in McCoy’s 5A medium (Gibco, USA) and Ishikawa cells were cultured in dulbecco’s modified Eagle’s medium (DMEM, Gibco, USA), both supplemented with 10% fetal bovine serum (FBS; Gibco, USA) at 37°C with 5% CO2.
2.11 Cell viability measurement
CCK-8 kit (meilunbio, China) was used to assess the viability of UCEC cells. Quercetin (Sigma, USA) was dissolved in dimethylsufoxide (DMSO) previously stored at -20 °C. The HEC-1 and Ishikawa cells (5 × 103 cells/well) were dispensed in 96-well flat-bottomed plates and treated with different quercetin concentrations (0, 20, 40 and 80 μM for HEC-1 cells, 0, 25, 50 and 100 μM for Ishikawa cells). The Microplate Reader (Bio Tek Instruments, USA) was applied to determine the absorbance at 450 nm.
2.12 Transwell migration assay
For migration assay, the transwell chambers with polycarbonate membranes (8 µm pore, Corning, USA) were used. After treated with quercetin for 48h, the HEC-1 cells (8 × 104 cells/well) and Ishikawa cells (4 × 104 cells/well) were planted in the upper compartment in the 24-well plate with 100 µL FBS-free medium, while the lower compartment was using DMEM with 10% FBS. The membranes were fixed with 4% paraformaldehyde after incubating 24 h, and then stained with 0.1% crystal violet dye for 10 min. By using the inverted routine microscope (Leica, Japan), migrated cells were counted in five random fields.
2.13 Western blotting
The lysis buffer containing radioimmunoprecipitation (RIPA) and 1 mM phenylmethanesulfonyl fluoride (PMSF, Beyotime Biotechnology, China) were used for the extraction of protein from HEC-1 cells and Ishikawa cells treated with various concentrations of quercetin. After centrifugation with 12000 g for 20 min at 4°C, the total protein in each group was measured with BCA Protein Assay Kit (Beyotime Biotechnology, China). Then proteins were fractionated in SDS-polyacrylamide gels and moved to blotting membrane (polyvinylidene fluoride, PVDF). Then, the membrane was incubated with the primary antibody at 4°C overnight, followed by 1 h at room temperature with the second antibody. Rabbit anti-human ISG15 (1:1000, Proteintech, China) and rabbit anti-human GAPDH (1:2000, Affinity Biosciences, China) were used as primary antibodies. The second goat anti-rabbit and conjugated with peroxidase were purchased from Biosharp (1:3000, China). Lastly, ECL reagent was applied to visual the protein bands.
2.14 Statistical methods
The data were analyzed using SPSS 24.0 and R 3.6.3. The experiments were conducted at least three times, and the results were expressed as mean ± standard deviation. The Student’s t test was used in two groups, and one-way ANOVA was applied to determine the differences in multiple groups. P < 0.05 represented statistical significance.
3 Results
3.1 Identification of UCEC DEGs and CRGs
TCGA-UCEC and GTEx cohorts were used to identify DEGs between normal uterus tissues and UCEC samples. A total of 6067 up-regulated genes and 2881 down-regulated genes were obtained by the criteria of |log2(FC)| > 1 and P< 0.05. Then, we obtained 492 CRGs. Finally, 138 overlapping genes were discovered in the UCEC DEGs and CRGs. Besides, 98 DEGs were up-regulated, while the other 40 DEGs were down-regulated in UCEC (Figure 2).
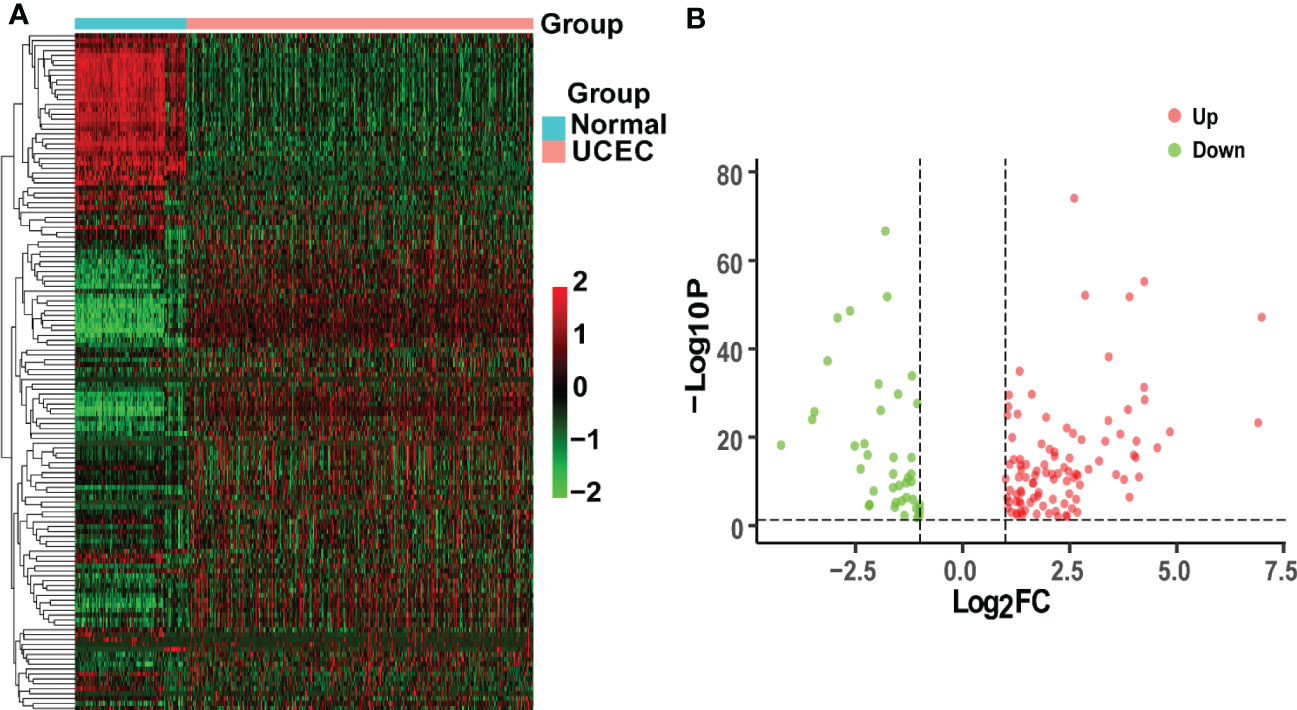
Figure 2 Analysis of intersecting genes in UCEC/COVID-19. (A) The heatmap of differential expression about intersecting genes in UCEC/COVID-19. (B) The volcano plot depicting 138 DGEs. Red dots and green dots represent differentially expressed up-regulated and down-regulated genes, respectively.
3.2 Recognition of quercetin’s targets
A total of 4237 drug targets for quercetin were predicted from KEGG, CTD, SwissTargetPrediction, DrugBank, and TargetNet. Then, the UCEC-DEGs/CRGs and quercetin target genes were analyzed, and 57 core target genes were acquired (Figure 3A). Moreover, 43 genes in core targets were up-regulated and 14 genes were down-regulated.
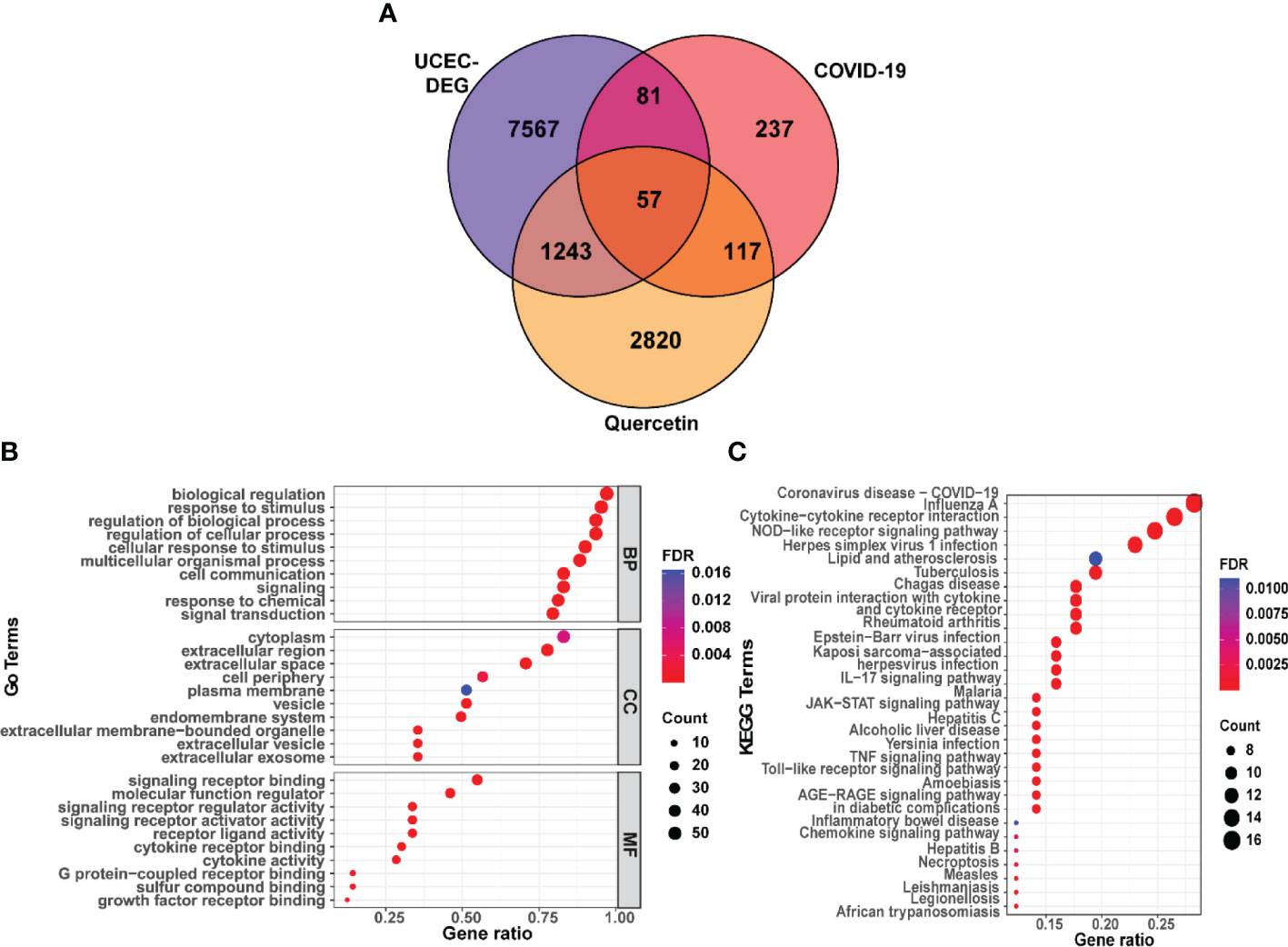
Figure 3 Functional characterization of quercetin against UCEC/COVID-19 intersecting genes. (A) Venn diagram describing intersecting genes of quercetin and UCEC/COVID-19. (B) GO analysis of intersecting genes of quercetin and UCEC/COVID-19. (C) KEGG pathway analysis of intersecting genes of quercetin and UCEC/COVID-19.
3.3 Functional enrichment analysis of core targets
Subsequently, functional enrichment analyses were performed by g:Profiler online tool. The results of GO found that the top BP terms were ‘biological regulation’, ‘response to stimulus’, and ‘regulation of cellular process’ (Supplementary Table 2). Besides, GO analysis identified the top CC terms, including ‘cytoplasm’, ‘extracellular region’, and ‘extracellular space’. Moreover, the top MF terms were ‘signaling receptor binding’ and ‘molecular function regulator’ (Figure 3B). In addition, the KEGG analysis results showed a significant relationship between 57 genes and ‘coronavirus disease-COVID-19’. (Figure 3C).
3.4 Construction of target network for quercetin’s core genes
Based on STRING platform, PPI network, which contained 57 nodes and 431 edges, was established to explore the mechanism of quercetin for treating UCEC/COVID-19 (Supplementary Figure 1). The cluster analysis of the PPI network was performed through MCODE function in Cytoscape 3.6.1 software and two clusters were found (Table 1). As shown in Figure 4A, cluster 1 consisted of 25 nodes and 236 edges. Then, GO analysis demonstrated cluster 1 was significantly enriched in ‘defense response’, ‘inflammatory response’, and ‘response to biotic stimulus’ (Supplementary Table 3 and Figure 4B). The KEGG pathway analysis showed cluster 1 was significantly enriched in ‘NOD-like receptor signaling pathway’, ‘Cytokine-cytokine receptor interaction’, and ‘IL-17 signaling pathway’ (Figure 4C). It is worth mentioning that two genes (ISG15, SOCS3) in cluster 1 were involved in the ubiquitination processes (Figure 4D). As shown in Figure 5, the diseases-drug-targets network contained 60 nodes and 171 edges.
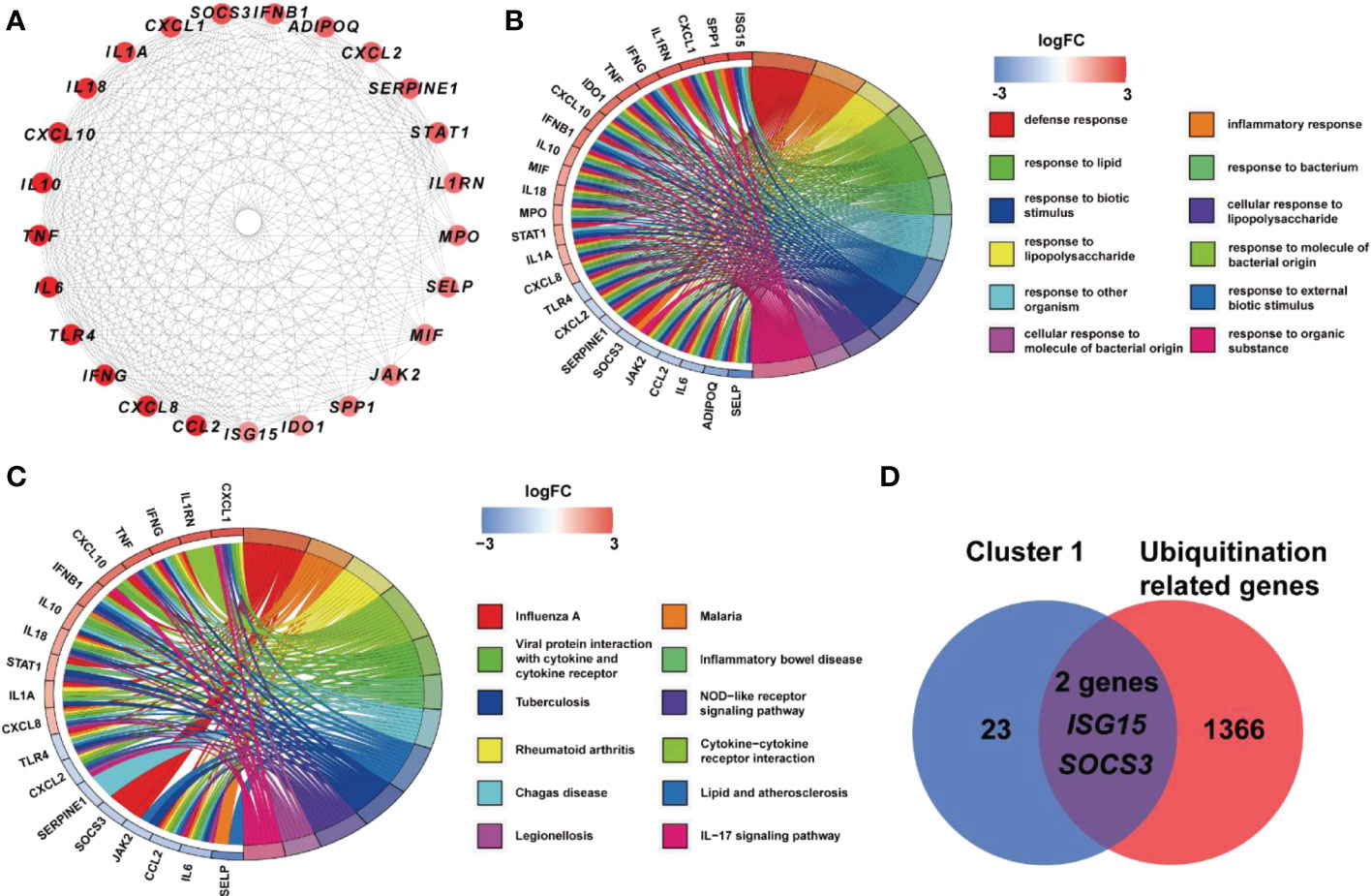
Figure 4 Analysis of cluster 1 from PPI network. (A) Cluster 1 contained 25 genes. (B) BP results from GO enrichment analysis of Cluster 1. (C) KEGG pathway analysis of genes in Cluster 1. (D) Two genes in Cluster 1 were URGs.
3.5 Clinical prognostic analysis of target genes of quercetin in UCEC DEGs and CRGs
According to univariate Cox analysis, 15 genes out of 57 drug targets in UCEC-DEGs/CRGs were significantly associated with patient prognosis (Table 2). Subsequently, the multivariate Cox analysis was conducted on the 15 prognostic genes. Nine risk signatures (ANPEP,OAS1, SCGB1A1, HLA‐A, NPPB, FGB, CCL2, TLR4, and SERPINE1) were obtained, and the risk scores of 9 genes were performed (Table 3). Next, the UCEC patients were categorized into two distinct groups based on the median risk score (Figure 6A). The formula of risk signature was as follow: ANPEP expression * 0.00202 + OAS1 expression * 0.02377 + SCGB1A1 expression * 0.00129 + HLA-A expression * (-0.00086) + NPPB expression * 0.07108 + FGB expression * 0.04111 + CCL2 expression * 0.01445 + TLR4 expression * (-0.18252) + SERPINE1 expression * 0.02068. As shown in Figure 6B, OS time was longer in group at low risk. In addition, ROC curves demonstrated the AUC values were 0.680 at 1 year, 0.681 at 2 years, and 0.673 at 3 years (Figure 6C). And there were significant differences in grade and age between the high and low risk groups (Figure 7).
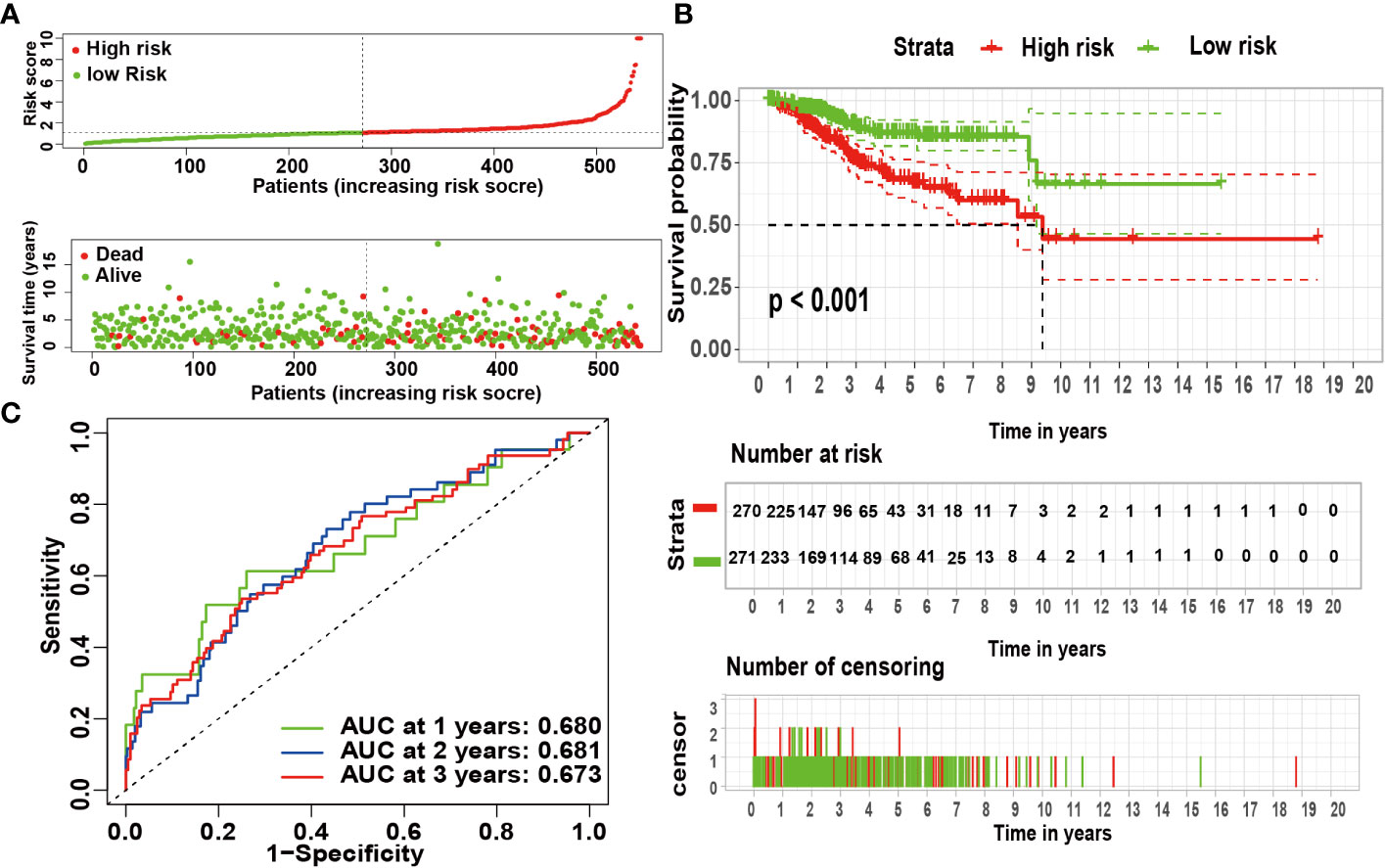
Figure 6 The risk coefficient model of UCEC predicted outcome. (A) The distribution of the risk scores of UCEC patients. (B) A Kaplan-Meier curve was constructed based on the survival status of the high- and low-risk groups. (C) The time-dependent curve showed that the AUC values at year 1, 2, and 3 were separated 0.680, 0.681, and 0.673, respectively.
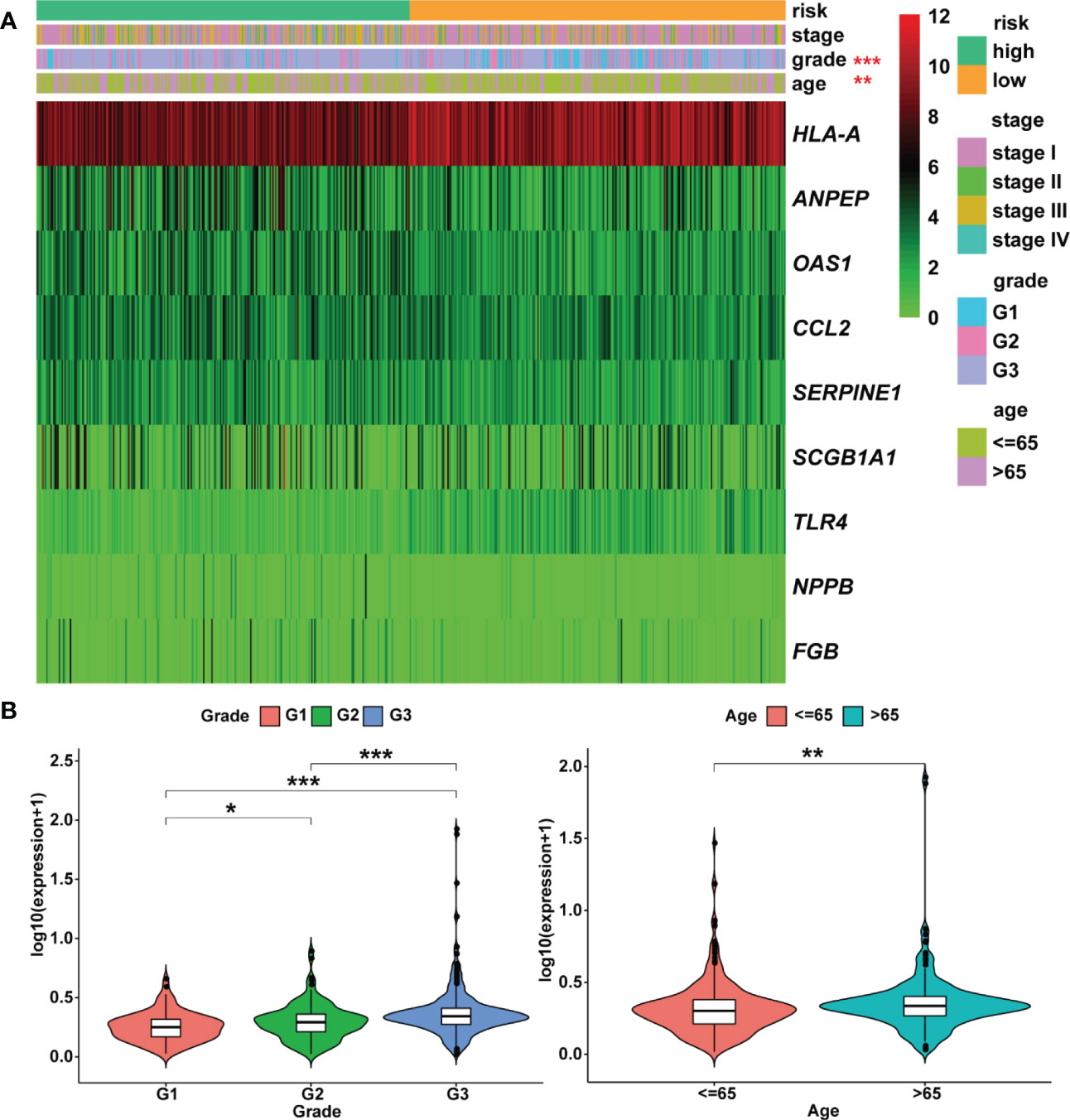
Figure 7 Heatmap and clinicopathologic parameters. (A) The clinical heatmap of 9 risk prognostic genes. (B) The risk scores were higher in the grade 3 and older patients. * means P< 0.05; ** means P< 0.01; *** means P< 0.001.
Moreover, the expression levels of risk signatures of UCEC patients with distinct clinical features were compared. According to the results, OAS1 was significantly increased in grade 3, stage III/IV, and older age (> 65) groups than that in grade 1/2, stage I/II, and younger (≤ 65) groups, respectively (Figures 8A–C). In contrast, the level of SCGB1A1 was significantly lower in grade 3 and stage III/IV than that in grade 1/2 and stage I/II, respectively (Figures 8D, E). Besides, the results showed that HLA‐A level was significantly higher in grade 1 and stage I/II than that in grade 2/3 and stage III/IV, respectively (Figures 8F, G). In grade 3, stage III/IV, and older age groups, the level of TLR4 was significantly lower than that in grade 1/2, stage I/II, and younger groups, respectively (Figures 8H–J). In addition, the SERPINE1 was lowly expressed in grade 1 and older age groups than that in grade 2/3 and younger age groups, respectively (Figures 8K, L).
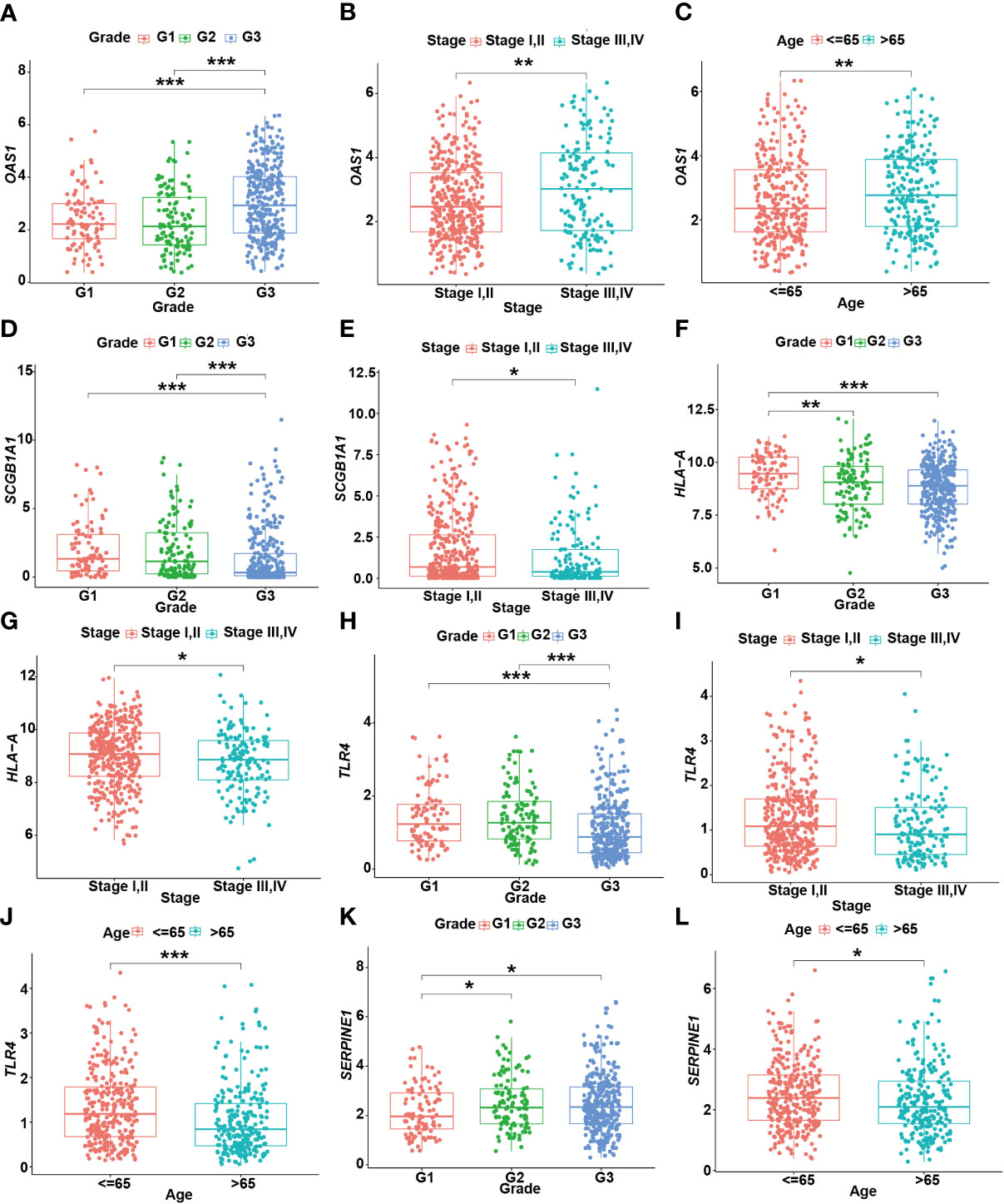
Figure 8 The relationship between prognostic gene levels and clinical characteristics. The relationship between the expression of OAS1 and grade (A), stage (B), and age (C) of UCEC patients. The relationship between the expression of SCGB1A1 and grade (D), stage (E) of UCEC patients. The relationship between the expression of HLA-A and grade (F), stage (G) of UCEC patients. The relationship between the expression of TLR4 and grade (H), stage (I), and age (J) of UCEC patients. The relationship between the expression of SERPINE1 and grade (K), age (L) of UCEC patients. * means P< 0.05; ** means P< 0.01; *** means P< 0.001.
3.6 Mutation profiles of gene signatures in risk model
Furthermore, R package ‘maftools’ was used to analyze and visualize the mutation frequencies of 9 risk signatures. As shown in Figure 9, ANPEP (8%), TLR4 (8%) and FGB (5%) were found commonly mutated. Mutations in genes including OAS1 (3%), SERPINE1 (3%), HLA-A (2%), NPPB (1%), and SCGB1A1 (1%) were also observed.
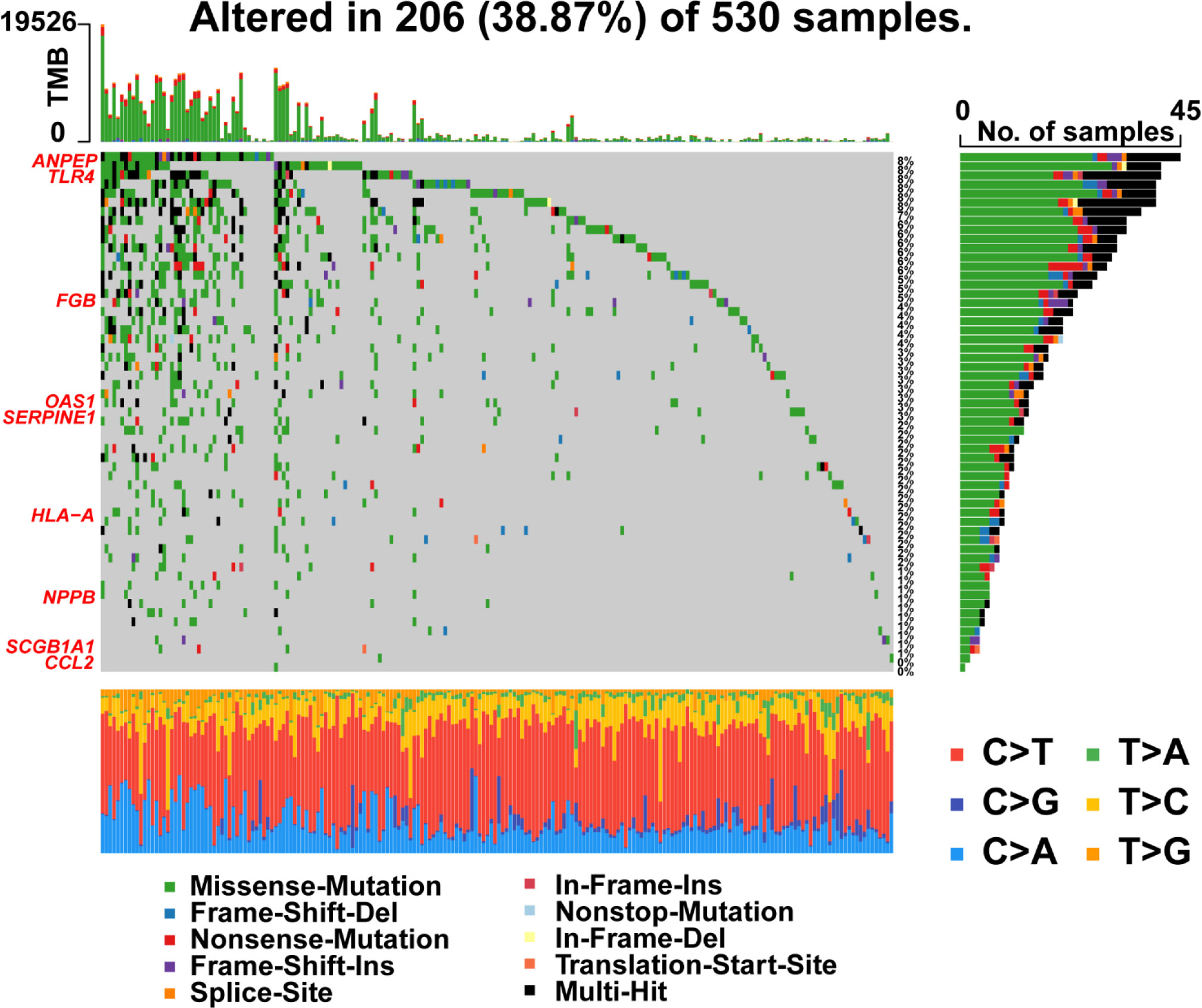
Figure 9 Landscape of mutations in 57 genes in UCEC. The left panel represents 9 prognostic genes which ordered by their mutation frequencies. The bottom panel represents 10 types of mutation.
3.7 Immune landscape of gene signatures in risk model
The immune landscape of the risk signatures in UCEC was further investigated via TIMER. Based on the results, the level of ANPEP, HLA‐A, CCL2, TLR4 and SERPINE1 had statistically significant association with tumor purity in UCEC tissues (Figures 10A–I). In addition, the correlations were also observed for 5, 4, 4, 4, 8, and 6 risk signatures, corresponding to the B cells, CD8+ T cells, CD4+ T cells, macrophages, neutrophils, and dendritic cells infiltration levels, respectively. Moreover, as shown in Figure 11, copy number variation of risk signatures had association with the infiltration of certain immune cells.
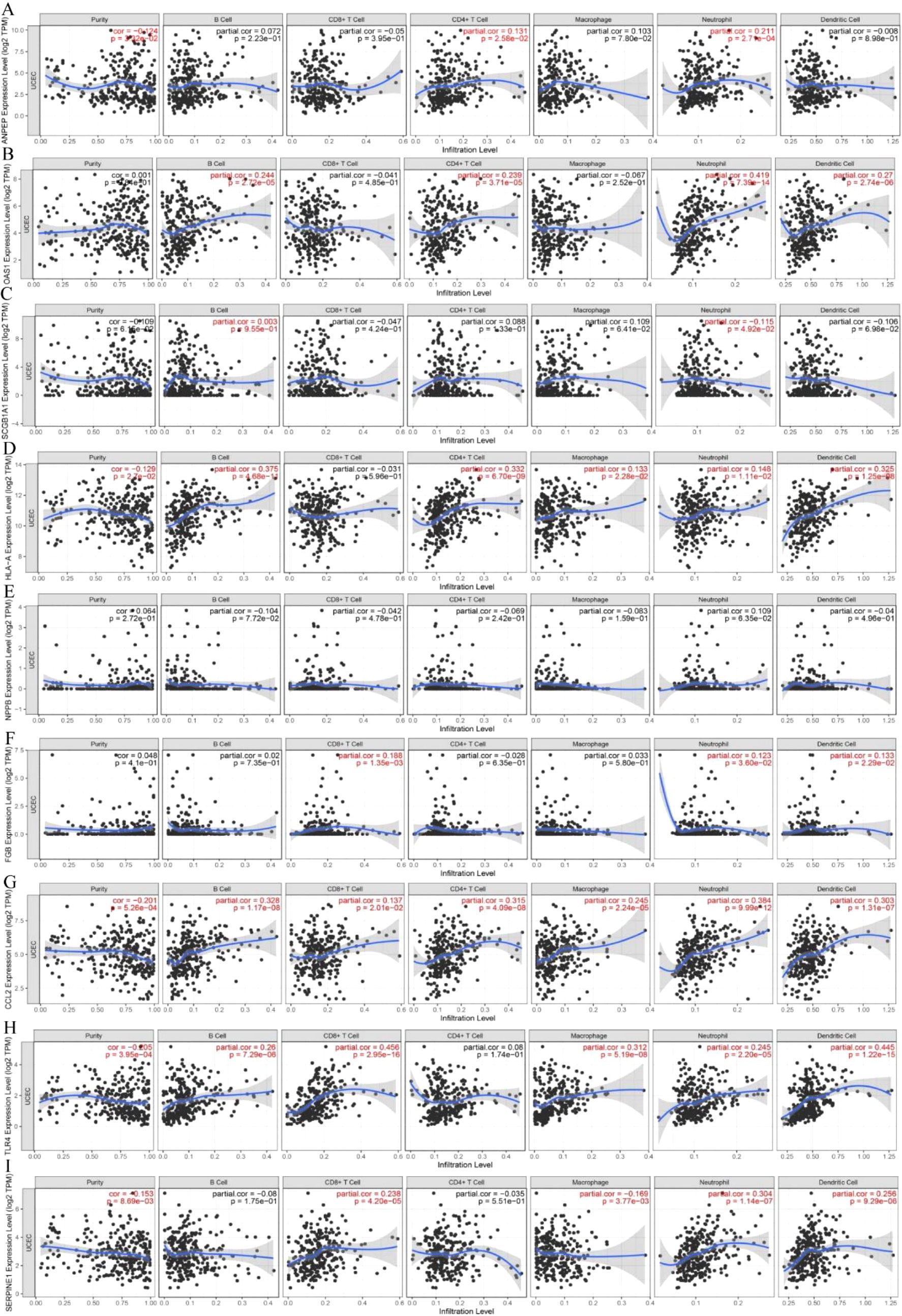
Figure 10 Correlation of 9 prognostic genes with immune cells in UCEC. (A) ANPEP, (B) OAS1, (C) SCGB1A1, (D) HLA‐A, (E) NPPB, (F) FGB, (G) CCL2, (H) TLR4, and (I) SERPINE1.
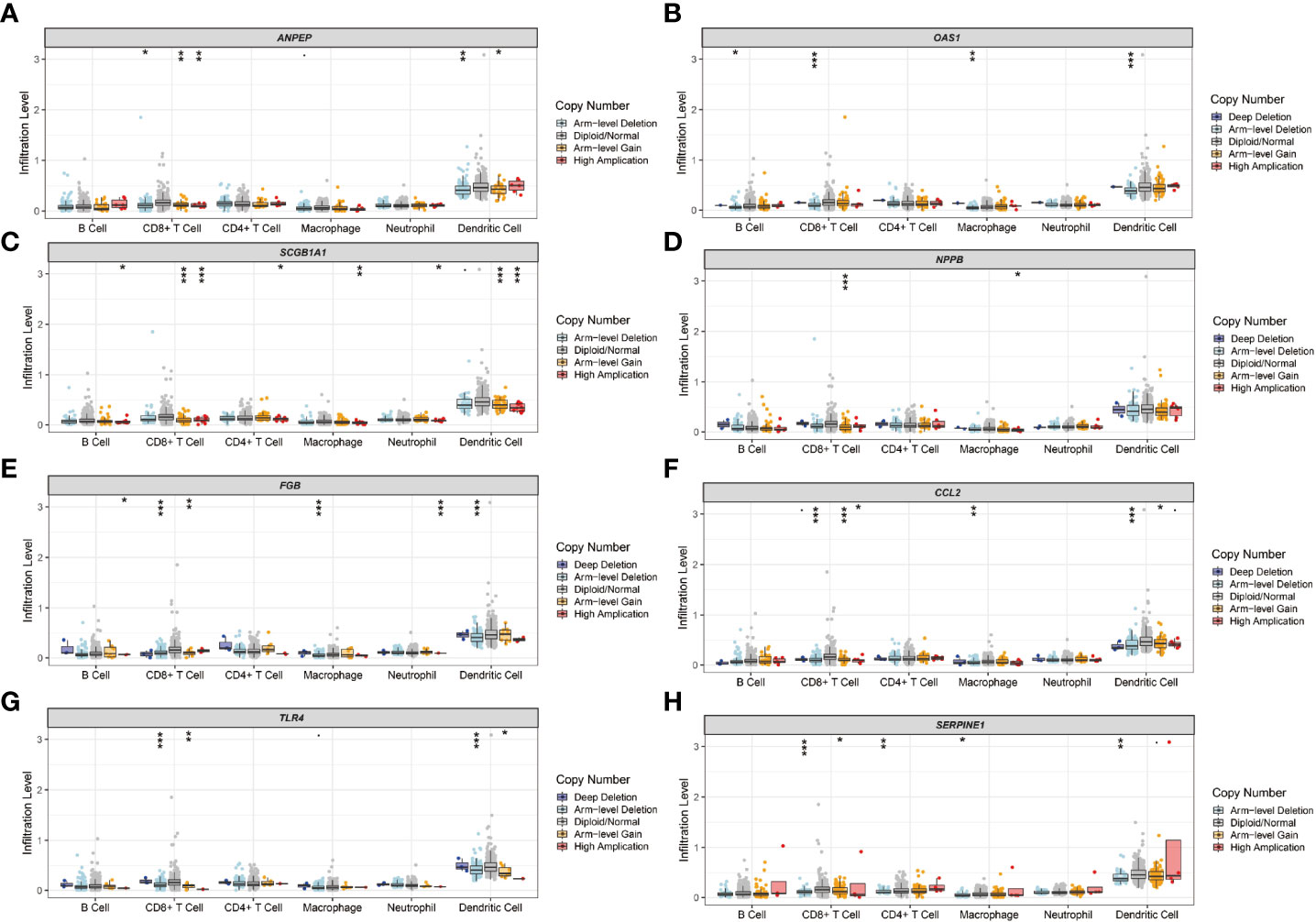
Figure 11 Immune cell infiltration influenced by copy number variation of prognostic genes. (A) ANPEP, (B) OAS1, (C) SCGB1A1, (D) NPPB, (E) FGB, (F) CCL2, (G) TLR4, and (H) SERPINE1.
3.8 The protein levels of gene signatures in risk model
HPA was applied to detect the protein levels of 9 risk signatures in human tissues. Notably, the expression of ANPEP, OAS1, SCGB1A1, and FGB could be detected in UCEC tissues. However, the protein levels of these 4 genes were not detected in human normal endometrial tissues. Additionally, medium protein level of CCL2 was observed in UCEC tissues. But the level of CCL2 was low in normal endometrial tissues (Figure 12).
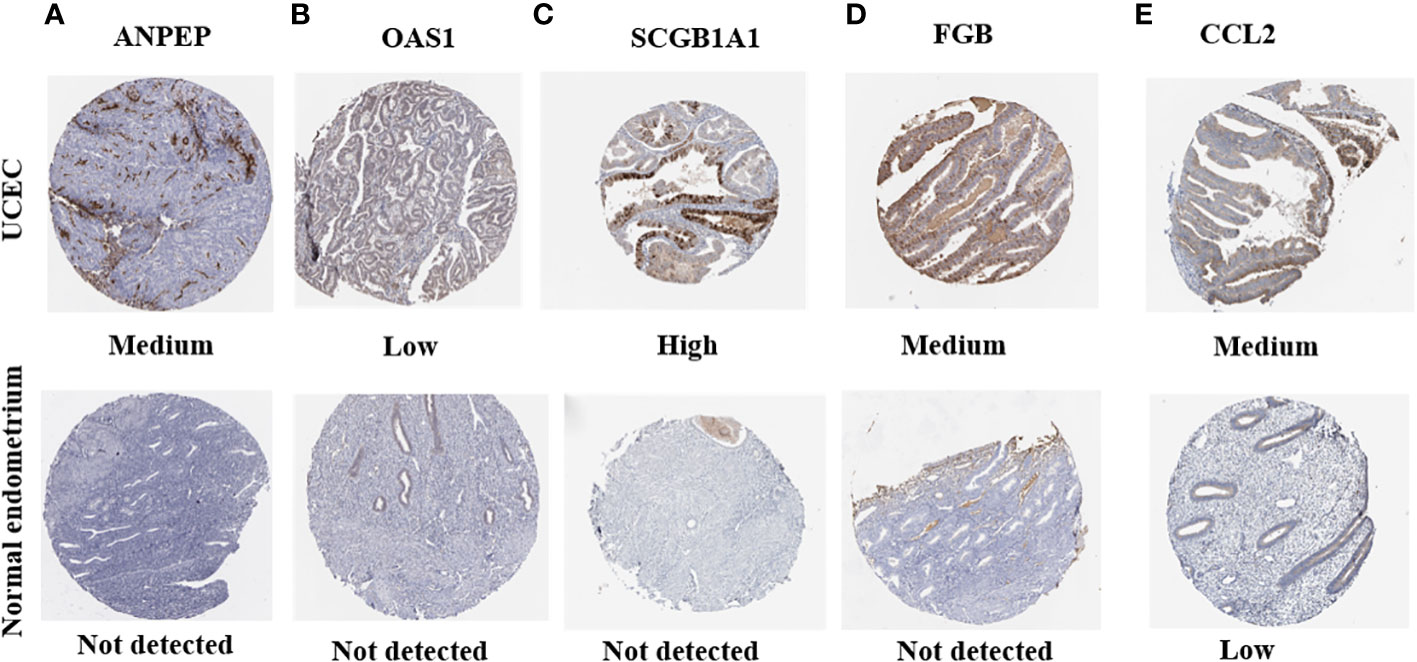
Figure 12 The protein expression of prognostic genes in UCEC tissues and normal endometrial tissues. (A) ANPEP, (B) OAS1, (C) SCGB1A1, (D) FGB, and (E) CCL2.
3.9 Molecular docking results
Molecular docking was applied to verify whether quercetin docked well with the main protease of SARS-CoV-2. The result showed that three hydrogen bonds formed at acid residues (Figure 13). The predicted binding energy of quercetin and main protease was -6.0 kcal/mol, indicating a strong affinity of quercetin and the main protease of SARS-CoV-2. To further explore potential targets in UCEC, molecular docking analyses between quercetin and 9 targets (ANPEP, OAS1, SCGB1A1, HLA‐A, NPPB, FGB, CCL2, TLR4, and SERPINE1) were conducted. As listed in Table 4, the molecular docking between quercetin and SERPINE1 had the least binding energy (-6.7 kcal/mol). It was followed by HLA-A (-6.2 kcal/mol) and OSA1 (-6.1 kcal/mol). In addition, docking affinity score between quercetin and ISG15 was -7.2 kcal/mol. And five hydrogen bonds were present in the conformation of quercetin and ISG15 molecular docking (Supplementary Figure 2).
3.10 Quercetin inhibited the proliferation and migration of UCEC cells
The CCK-8 analysis indicated that both HEC-1 and Ishikawa cells were significantly inhibited by quercetin in a dose-dependent manner at concentration from 0 to 80 μM and 0 to 100 μM, respectively (P< 0.001, Figure 14A). The IC50 values for inhibition of HEC-1 and Ishikawa cells were 67.67 and 48.29 μM at 48 h, respectively. The migration abilities of these two cells were evaluated using transwell migration assay. As shown in Figure 14B, compared with controls, the migration of HEC-1 and Ishikawa cells were markedly decreased under quercetin treatment at concentration of 60 and 50 μM, respectively.
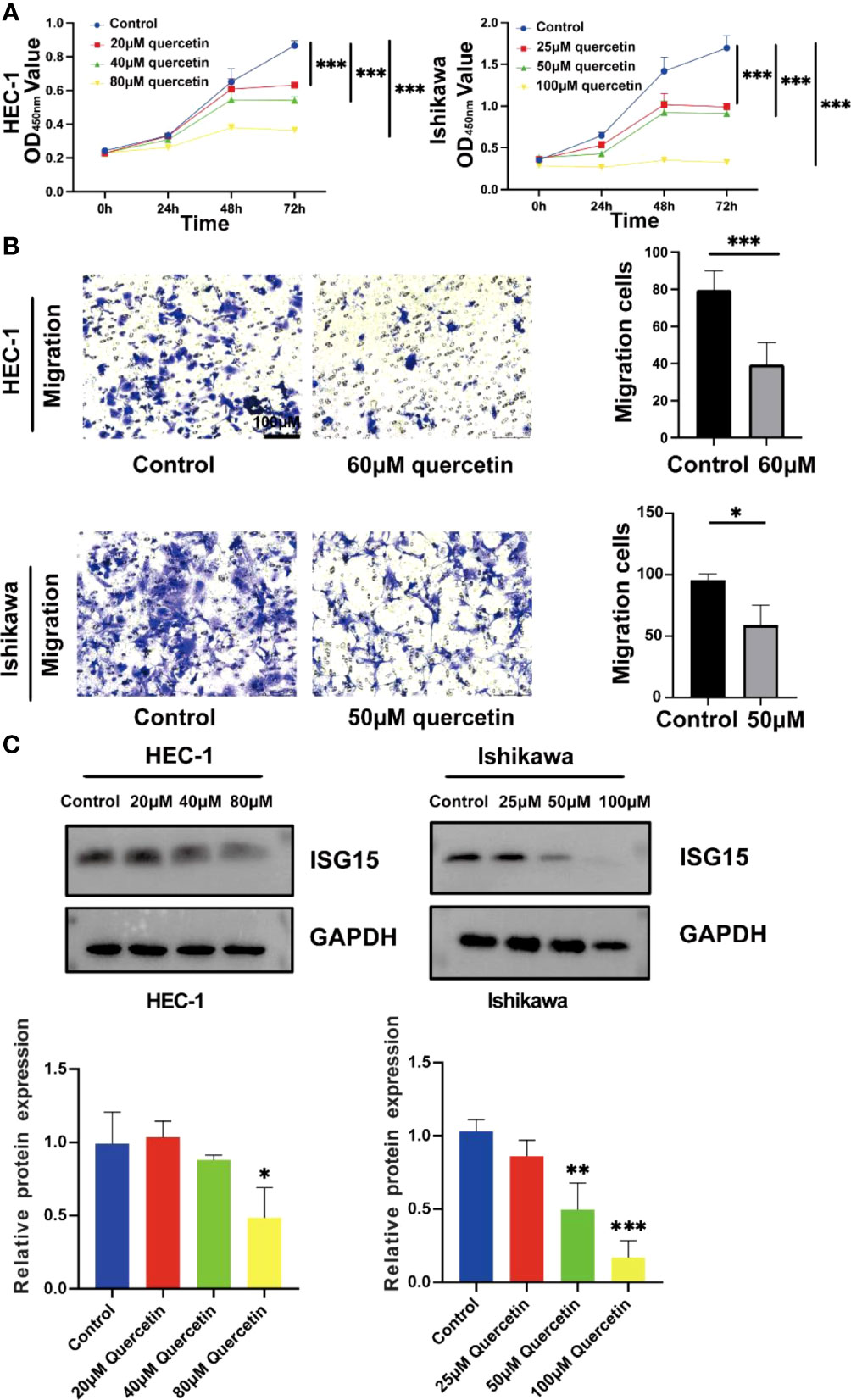
Figure 14 Quercetin inhibits the proliferation and migration of UCEC cells and reduces the expression of ISG15. (A) Quercetin suppressed viability of HEC-1 and Ishikawa cells by CCK-8 assay. (B) Left panel: Transwell assays were used in HEC-1 and Ishikawa cells with or without quercetin. Right panel: Quantitative analyses for Transwell assays. (C) Western blotting showed quercetin reduced the expression of ISG15. The bar graph showed the ratio of ISG15 level between quercetin treatment group and control group. * means P< 0.05; ** means P< 0.01; *** means P< 0.001.
3.11 Quercetin decreased the expression of ISG15 in UCEC cells
By analyzing TCGA and GTEx cohorts, we found that ISG15 was significantly elevated in UCEC patients compared to controls (Supplementary Figure 3). In order to further investigate the role of ISG15 in the process of quercetin for treating UCEC patients, the protein level of ISG15 was detected. Our results demonstrated that ISG15 tended to dose-dependently decrease by quercetin treatment in UCEC cells in vitro (P< 0.05, Figure 14C).
4 Discussion
During radiation and chemotherapy, many cancer patients might be immunocompromised and their general health might decline (22). It is likely that cancer patients are highly susceptible to COVID-19 during the hospitalization. Quercetin inhibits angiogenesis and affects autophagy in cancer cells to exert its anti-tumor effects (10). Additionally, quercetin could theoretically interfere with SARS-CoV-2 replication (9). Therefore, UCEC patients infected with COVID-19 might benefit from quercetin’s anticancer and antiviral properties. Our study constructed a quercetin-UCEC/COVID-19 network, composed of 57 genes based on UCEC DEGs, CRGs, and quercetin target genes. Functional enrichment analysis showed that quercetin worked mainly by ‘biological regulation’, ‘response to stimulus’, and ‘regulation of cellular process’ against UCEC and COVID-19.
Further, the hub cluster from the quercetin-UCEC/COVID-19 network identified ISG15, a gene connected with ubiquitination. The ISG15, which was implicated in the pathogenesis of cancer, formed covalent bonds with its target substrates using a cascade of enzymes (E1, E2, and E3) (23). Desai et al. showed that increasing ISG15 levels in cancer cells exerted pro-tumor effect through ubiquitination-mediated protein regulation (24). Another study found that the elevated level of ISG15 contributed to immune escape in UCEC, as well as activation of MYC proto-oncogene signaling pathway (15). In the current study, ISG15 was also elevated in UCEC patients compared to normal controls. Previous study has shown that quercetin inhibited tumor progression by inducing ubiquitination and down-regulating HER2 expression in breast cancer cells (25). Our experiments showed quercetin inhibited UCEC cells proliferation and migration, and quercetin reduced the expression of ISG15 dose-dependently. It is necessary to further explore the role of ubiquitination-related gene ISG15 in UCEC patients, especially those with COVID-19 infection.
By Cox regression analyses, we identified 9 risk prognostic genes (ANPEP, OAS1, SCGB1A1, HLA‐A, NPPB, FGB, CCL2, TLR4, and SERPINE1) for UCEC. Interestingly, different types of mutations of 9 risk prognostic genes such as nonsense mutation, missense mutation, and multi-hit were also found in UCEC. The high frequency of mutations in ANPEP, TLR4 and FGB were observed. However, the specific mechanisms of these mutations on UCEC are not clear now. Our present study revealed that the dysregulation of 9 risk prognostic genes served as an important part in UCEC. A previous study reported that HLA-A might enhance immune response against tumor mediated by T cells in upper gastrointestinal cancer (26). Allhorn et al. (27) found TLR4 potentially interacted with hyaluronic acid to activate CD44-mediated signaling, thereby promoting endometrial repair. According to this study, with higher stage and grade of UCEC, the levels of HLA-A and TLR4 were lower, which were significantly associated with longer survival. The results of our study confirm that HLA-A and TLR4 act as tumor suppressor genes during tumorigenesis and tumor progression.
ANPEP is a specific ectopeptidase and related to solid tumor growth (28). We confirmed that ANPEP associated with poor prognosis and highly expressed in UCEC tissues. A previous study proved that OAS1 was associated with poor prognosis in pancreatic cancer (29). Our results demonstrated OAS1 expression in UCEC was positively correlated with stage and grade. The risk of UCEC was also positively associated with SERPINE1 (30). In addition, Wilson et al. (31) found SERPINE1 could promote endometrial invasion via driving ARID1A gene mutant. In this study, there was a significant increase in SERPINE1 expression in the grade 2/3, compared to the grade 1. Thus, we hypothesized that ANPEP, OAS1 and SERPINE1 contribute to the carcinogenesis of UCEC.
Researchers discovered that NPPB played an important role in epithelial ovarian cancer progression (32). Besides, FGB was reported to contribute to tumor angiogenesis and metastasis (33). CCL2 had also been shown to promote tumors and metastases by polarizing macrophages M2 (34). In our study, we also observed that NPPB, FGB, and CCL2 were related to poor prognosis in UCEC patients.
It was believed that SCGB1A1 modulated inflammation and tumorigenesis (35). Furthermore, SCGB1A1 was considered as a biomarker for ovarian cancers with poor outcomes (36). Similarly to past studies, SCGB1A1 was considered a risk factor for UCEC prognosis in this work. However, SCGB1A1 level in grade 3 and stage III/IV was significantly lower than in grade 1/2 and stage I/II of the UCEC, respectively. Further investigation is needed into the complicated mechanism of SCGB1A1 in UCEC.
Unfortunately, several limitations exist in this study. Additional experiments need to be conducted on cells, animals, and humans to validate the results of this study. Besides, quercetin’s role in mediating proliferation and migration of tumor cells in UCEC patients with COVID-19 through ISG15-related pathways needs to be further explored.
In conclusion, our findings highlight the feasibility of quercetin for anti-UCEC/COVID-19 by ‘biological regulation’, ‘response to stimulus’, and ‘regulation of cellular process’. Furthermore, network pharmacology identified 57 targets of quercetin that were potentially useful in treating UCEC/COVID-19. The Cox regression analyses suggested that 9 prognostic genes (ANPEP, OAS1, SCGB1A1, HLA‐A, NPPB, FGB, CCL2, TLR4, and SERPINE1) might act critical roles in the treatment of UCEC/COVID-19. Additionally, quercetin could retard the cells proliferation and migration in UCEC and reduce ubiquitination related gene ISG15 expression (Figure 15). This study enriched treatment options for UCEC patients with COVID-19 and provided rationale for strategies for further study of quercetin treatment in UCEC patients infected with COVID-19.
Data availability statement
The original contributions presented in the study are included in the article/Supplementary Material. Further inquiries can be directed to the corresponding author.
Author contributions
Conceptualization: KL, XZ, and HL. Data analysis and performing experiments: KL, YL, LG, and XX. Investigation and writing: KL and HL. Revising manuscript: YL, LG, and XX. Data supervision and editing manuscript: XZ. All authors contributed to the article and approved the submitted version.
Funding
This work was supported by Zhejiang Key Laboratory of Traditional Chinese Medicine for Diagnosis and Treatment of Gynecological Cancers (2022-11).
Acknowledgments
The Figures 1 and 15 was created with BioRender.com. The authors would like to thank the TCGA, GTEx, NCBI, CTD, SwissTargetPrediction, DrugBank, TargetNet, KEGG, IUUCD, TIMER, STRING and g:Profiler databases for open access in this section.
Conflict of interest
The authors declare that the research was conducted in the absence of any commercial or financial relationships that could be construed as a potential conflict of interest.
Publisher’s note
All claims expressed in this article are solely those of the authors and do not necessarily represent those of their affiliated organizations, or those of the publisher, the editors and the reviewers. Any product that may be evaluated in this article, or claim that may be made by its manufacturer, is not guaranteed or endorsed by the publisher.
Supplementary material
The Supplementary Material for this article can be found online at: https://www.frontiersin.org/articles/10.3389/fonc.2023.1151434/full#supplementary-material
References
1. Boulware DR, Pullen MF, Bangdiwala AS, Pastick KA, Lofgren SM, Okafor EC, et al. A randomized trial of hydroxychloroquine as postexposure prophylaxis for covid-19. N Engl J Med (2020) 383(6):517–25. doi: 10.1056/NEJMoa2016638
2. Self WH, Sandkovsky U, Reilly CS, Vock DM, Gottlieb RL, Mack M, et al. Efficacy and safety of two neutralising monoclonal antibody therapies, sotrovimab and brii-196 plus brii-198, for adults hospitalised with covid-19 (Tico): A randomised controlled trial. Lancet Infect Dis (2022) 22(5):622–35. doi: 10.1016/s1473-3099(21)00751-9
3. Sestili P, Fimognari C. Paracetamol-induced glutathione consumption: Is there a link with severe covid-19 illness? Front Pharmacol (2020) 11:579944. doi: 10.3389/fphar.2020.579944
4. Siegel RL, Miller KD, Fuchs HE, Jemal A. Cancer statistic. CA Cancer J Clin (2021) 71(1):7–33. doi: 10.3322/caac.21654
5. Liang W, Guan W, Chen R, Wang W, Li J, Xu K, et al. Cancer patients in sars-Cov-2 infection: A nationwide analysis in China. Lancet Oncol (2020) 21(3):335–7. doi: 10.1016/s1470-2045(20)30096-6
6. Desai A, Gupta R, Advani S, Ouellette L, Kuderer NM, Lyman GH, et al. Mortality in hospitalized patients with cancer and coronavirus disease 2019: A systematic review and meta-analysis of cohort studies. Cancer (2021) 127(9):1459–68. doi: 10.1002/cncr.33386
7. Richardson S, Hirsch JS, Narasimhan M, Crawford JM, McGinn T, Davidson KW, et al. Presenting characteristics, comorbidities, and outcomes among 5700 patients hospitalized with covid-19 in the new York city area. Jama (2020) 323(20):2052–9. doi: 10.1001/jama.2020.6775
8. Lee KA, Ma W, Sikavi DR, Drew DA, Nguyen LH, Bowyer RCE, et al. Cancer and risk of covid-19 through a general community survey. Oncologist (2021) 26(1):e182–5. doi: 10.1634/theoncologist.2020-0572
9. Lin HY, Zeng YT, Lin CJ, Harroun SG, Anand A, Chang L, et al. Partial carbonization of quercetin boosts the antiviral activity against H1n1 influenza a virus. J Colloid Interface Sci (2022) 622:481–93. doi: 10.1016/j.jcis.2022.04.124
10. Tang SM, Deng XT, Zhou J, Li QP, Ge XX, Miao L. Pharmacological basis and new insights of quercetin action in respect to its anti-cancer effects. BioMed Pharmacother (2020) 121:109604. doi: 10.1016/j.biopha.2019.109604
11. Ji H, Li K, Xu W, Li R, Xie S, Zhu X. Prediction of the mechanisms by which quercetin enhances cisplatin action in cervical cancer: A network pharmacology study and experimental validation. Front Oncol (2021) 11:780387. doi: 10.3389/fonc.2021.780387
12. Bandera EV, Williams MG, Sima C, Bayuga S, Pulick K, Wilcox H, et al. Phytoestrogen consumption and endometrial cancer risk: A population-based case-control study in new Jersey. Cancer Causes Control (2009) 20(7):1117–27. doi: 10.1007/s10552-009-9336-9
13. Dastsooz H, Cereda M, Donna D, Oliviero S. A comprehensive bioinformatics analysis of Ube2c in cancers. Int J Mol Sci (2019) 20(9):2228. doi: 10.3390/ijms20092228
14. Han HG, Moon HW, Jeon YJ. Isg15 in cancer: Beyond ubiquitin-like protein. Cancer Lett (2018) 438:52–62. doi: 10.1016/j.canlet.2018.09.007
15. Zhao X, Wang J, Wang Y, Zhang M, Zhao W, Zhang H, et al. Interferon−Stimulated gene 15 promotes progression of endometrial carcinoma and weakens antitumor immune response. Oncol Rep (2022) 47(6):110. doi: 10.3892/or.2022.8321
16. Huang L, Li M, Wei H, Yu Q, Huang S, Wang T, et al. Research on the indirect antiviral function of medicinal plant ingredient quercetin against grouper iridovirus infection. Fish Shellfish Immunol (2022) 124:372–9. doi: 10.1016/j.fsi.2022.04.013
17. Zhang YZ, Yang JY, Wu RX, Fang C, Lu H, Li HC, et al. Network pharmacology-based identification of key mechanisms of xihuang pill in the treatment of triple-negative breast cancer stem cells. Front Pharmacol (2021) 12:714628. doi: 10.3389/fphar.2021.714628
18. Liu C, Yin Z, Feng T, Zhang M, Zhou Z, Zhou Y. An integrated network pharmacology and rna-seq approach for exploring the preventive effect of lonicerae japonicae flos on lps-induced acute lung injury. J Ethnopharmacol (2021) 264:113364. doi: 10.1016/j.jep.2020.113364
19. Shannon P, Markiel A, Ozier O, Baliga NS, Wang JT, Ramage D, et al. Cytoscape: A software environment for integrated models of biomolecular interaction networks. Genome Res (2003) 13(11):2498–504. doi: 10.1101/gr.1239303
20. Xia QD, Xun Y, Lu JL, Lu YC, Yang YY, Zhou P, et al. Network pharmacology and molecular docking analyses on lianhua qingwen capsule indicate Akt1 is a potential target to treat and prevent covid-19. Cell Prolif (2020) 53(12):e12949. doi: 10.1111/cpr.12949
21. Seeliger D, de Groot BL. Ligand docking and binding site analysis with pymol and Autodock/Vina. J Comput Aided Mol Des (2010) 24(5):417–22. doi: 10.1007/s10822-010-9352-6
22. Al-Quteimat OM, Amer AM. The impact of the covid-19 pandemic on cancer patients. Am J Clin Oncol (2020) 43(6):452–5. doi: 10.1097/coc.0000000000000712
23. Jiménez Fernández D, Hess S, Knobeloch KP. Strategies to target Isg15 and Usp18 toward therapeutic applications. Front Chem (2019) 7:923. doi: 10.3389/fchem.2019.00923
24. Desai SD, Haas AL, Wood LM, Tsai YC, Pestka S, Rubin EH, et al. Elevated expression of Isg15 in tumor cells interferes with the Ubiquitin/26s proteasome pathway. Cancer Res (2006) 66(2):921–8. doi: 10.1158/0008-5472.Can-05-1123
25. Jeong JH, An JY, Kwon YT, Li LY, Lee YJ. Quercetin-induced ubiquitination and down-regulation of her-2/Neu. J Cell Biochem (2008) 105(2):585–95. doi: 10.1002/jcb.21859
26. Mimura K, Shiraishi K, Mueller A, Izawa S, Kua LF, So J, et al. The mapk pathway is a predominant regulator of hla-a expression in esophageal and gastric cancer. J Immunol (2013) 191(12):6261–72. doi: 10.4049/jimmunol.1301597
27. Allhorn S, Böing C, Koch AA, Kimmig R, Gashaw I. Tlr3 and Tlr4 expression in healthy and diseased human endometrium. Reprod Biol Endocrinol (2008) 6:40. doi: 10.1186/1477-7827-6-40
28. Wickström M, Larsson R, Nygren P, Gullbo J. (Cd13) as a target for cancer chemotherapy. Cancer Sci (2011) 102(3):501–8. doi: 10.1111/j.1349-7006.2010.01826.x
29. Lu L, Wang H, Fang J, Zheng J, Liu B, Xia L, et al. Overexpression of Oas1 is correlated with poor prognosis in pancreatic cancer. Front Oncol (2022) 12:944194. doi: 10.3389/fonc.2022.944194
30. Trabert B, Eldridge RC, Pfeiffer RM, Shiels MS, Kemp TJ, Guillemette C, et al. Prediagnostic circulating inflammation markers and endometrial cancer risk in the prostate, lung, colorectal and ovarian cancer (Plco) screening trial. Int J Cancer (2017) 140(3):600–10. doi: 10.1002/ijc.30478
31. Wilson MR, Reske JJ, Holladay J, Neupane S, Ngo J, Cuthrell N, et al. Arid1a mutations promote P300-dependent endometrial invasion through super-enhancer hyperacetylation. Cell Rep (2020) 33(6):108366. doi: 10.1016/j.celrep.2020.108366
32. Lawrenson K, Grun B, Lee N, Mhawech-Fauceglia P, Kan J, Swenson S, et al. Nppb is a novel candidate biomarker expressed by cancer-associated fibroblasts in epithelial ovarian cancer. Int J Cancer (2015) 136(6):1390–401. doi: 10.1002/ijc.29092
33. Wang M, Zhang G, Zhang Y, Cui X, Wang S, Gao S, et al. Fibrinogen alpha chain knockout promotes tumor growth and metastasis through integrin-akt signaling pathway in lung cancer. Mol Cancer Res (2020) 18(7):943–54. doi: 10.1158/1541-7786.Mcr-19-1033
34. Xu M, Wang Y, Xia R, Wei Y, Wei X. Role of the Ccl2-Ccr2 signalling axis in cancer: Mechanisms and therapeutic targeting. Cell Prolif (2021) 54(10):e13115. doi: 10.1111/cpr.13115
35. Saha A, Lee YC, Zhang Z, Chandra G, Su SB, Mukherjee AB. Lack of an endogenous anti-inflammatory protein in mice enhances colonization of B16f10 melanoma cells in the lungs. J Biol Chem (2010) 285(14):10822–31. doi: 10.1074/jbc.M109.083550
Keywords: COVID-19, uterine corpus endometrial carcinoma (UCEC), quercetin, ubiquitination, network pharmacology (NP)
Citation: Li K, Liu H, Lin Y, Gu L, Xiang X and Zhu X (2023) Discovery of therapeutic targets of quercetin for endometrial carcinoma patients infected with COVID-19 through network pharmacology. Front. Oncol. 13:1151434. doi: 10.3389/fonc.2023.1151434
Received: 26 January 2023; Accepted: 17 February 2023;
Published: 08 March 2023.
Edited by:
Lixia Wang, Soochow University, ChinaReviewed by:
Jianhua Yang, Zhejiang University, ChinaHaiyan Zhu, Shanghai First Maternity and Infant Hospital, China
Xipeng Wang, Shanghai Jiao Tong University, China
Copyright © 2023 Li, Liu, Lin, Gu, Xiang and Zhu. This is an open-access article distributed under the terms of the Creative Commons Attribution License (CC BY). The use, distribution or reproduction in other forums is permitted, provided the original author(s) and the copyright owner(s) are credited and that the original publication in this journal is cited, in accordance with accepted academic practice. No use, distribution or reproduction is permitted which does not comply with these terms.
*Correspondence: Xueqiong Zhu, d3p6eHFAd3poZWFsdGguY29t
†These authors have contributed equally to this work