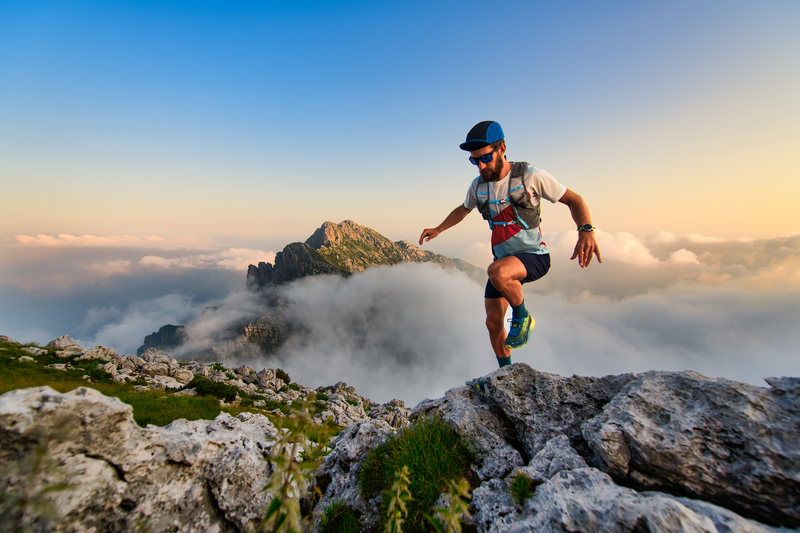
94% of researchers rate our articles as excellent or good
Learn more about the work of our research integrity team to safeguard the quality of each article we publish.
Find out more
REVIEW article
Front. Oncol. , 21 April 2023
Sec. Gynecological Oncology
Volume 13 - 2023 | https://doi.org/10.3389/fonc.2023.1133491
Background: In recent years, AI has been applied to disease diagnosis in many medical and engineering researches. We aimed to explore the diagnostic performance of the models based on different imaging modalities for ovarian cancer.
Methods: PubMed, EMBASE, Web of Science, and Wanfang Database were searched. The search scope was all published Chinese and English literatures about AI diagnosis of benign and malignant ovarian tumors. The literature was screened and data extracted according to inclusion and exclusion criteria. Quadas-2 was used to evaluate the quality of the included literature, STATA 17.0. was used for statistical analysis, and forest plots and funnel plots were drawn to visualize the study results.
Results: A total of 11 studies were included, 3 of them were modeled based on ultrasound, 6 based on MRI, and 2 based on CT. The pooled AUROCs of studies based on ultrasound, MRI and CT were 0.94 (95% CI 0.88-1.00), 0.82 (95% CI 0.71-0.93) and 0.82 (95% Cl 0.78-0.86), respectively. The values of I2 were 99.92%, 99.91% and 92.64% based on ultrasound, MRI and CT. Funnel plot suggested no publication bias.
Conclusion: The models based on ultrasound have the best performance in diagnostic of ovarian cancer.
Ovarian cancer (OC) is the malignant tumor with the highest death rate in the female reproductive system, with a high incidence rate and mortality (1). Among gynecological tumors, the incidence rate ranks third and the mortality ranks first, surpassing cervical cancer and endometrial cancer, posing a serious threat to the health of women (2). Unnecessary surgery leads to reduced fertility, therefore, accurate preoperative assessment of the risk of malignancy can help physicians provide individualized treatment for patients (3).
At present, the diagnosis of OC mainly relies on pathological examination and medical imaging techniques which can assist in the diagnosis and treatment of OC (4). However, due to the insidious nature of OC, the physician’s visual inspection of medical images can’t provide enough information to personalize the treatment for the patient (5). Artificial intelligence (AI) can automatically recognize complex patterns in imaging data, extract potential information from medical images, and provide quantitative assessment of radiographic characteristics (6). The application of artificial intelligence in medical imaging mainly includes two categories of radiomics and deep learning (7). This non-invasive approach reduces patient pain and helps physicians personalize treatment for patients.
For OC patients, proper and accurate preoperative imaging is very important for the treatment of cancer. Ultrasound is mainly used for early screening of ovarian cancer (8). The computed tomography (CT) imaging is the standard for preoperative evaluation of patients with OC; magnetic resonance imaging (MRI) focuses on imaging small peritoneal deposits in difficult-to-resect areas (9). To our knowledge, a recent study conducted Mata-analysis of studies which early predicted different kinds of diseases based on AI, demonstrating the important role of AI in disease diagnosis (10). Another study evaluated the diagnostic performance of artificial intelligence for lymph node metastasis in abdominopelvic malignancies and found that the diagnostic ability of artificial intelligence was higher than the subjective judgment of physicians (11). However, these studies have ignored the impact of different imaging modalities on artificial intelligence diagnostic results.
The purpose of this study was to conduct a systematic review and meta-analysis of published data on ovarian cancer to assess the accuracy of artificial intelligence in the application of multiple imaging modalities for OC.
In this study, the Preferred Reporting Item of the Guidelines for Systematic Reviews and Meta-Analysis (PRISMA) was used as the search rule (12), and the databases used for the search were PubMed, EMBASE, Web of Science, and Wanfang Database. Table 1 shows the method of search. The search was conducted using subject terms including “radiomics,” “deep learning,” “Artificial intelligence,” “ovarian cancer,” and “malignant ovarian tumors”. Combine the results of different queries by using the Boolean operator AND. Any eligible studies were considered preliminary search results. To get all relevant literatures, we searched the reference list of relevant studies by manual search.
The inclusion and exclusion criteria for studies were as follows. Inclusion criteria: (1) retrospective or prospective studies evaluating the diagnostic efficacy of AI in identifying ovarian tumors of patients; and (2) patients with ovarian tumor. Exclusion criteria: (1) animal studies, case reports, conference literature; (2) insufficient computable data; and (3) duplicate reports or studies based on the same data. Two researchers used Covidence software to screen studies and identified titles and abstracts. Disagreements in the process of study screening were arbitrated and agreed upon by a third author.
Data were extracted from all eligible studies, and information extracted included:first author, country, year of publication, type of AI model, number of patients, age of patients, type of tumor, type of stu1dy and imaging modality. The area under the receiver operating characteristic curve (AUROC), sensitivity (SEN), specificity (SPE), and accuracy are used to evaluate the performance of the models, with AUROC being considered the most important metric. The data we extracted was used for data processing and forest map production.
The Quality Assessment of Diagnostic Accuracy Studies Scale (QUADAS-2) was used to assess the risk of bias of included studies (13). First, the two researchers responded to each study’s landmark questions using three options: “yes”, “no”, and “uncertain”. Then the third researcher used the QUADAS-2 to rate the risk of bias into three categories, “low,” “high,” or “uncertain”.
Meta-analysis of the included literature was implemented in this study using STATA 17.0. If the study population is divided into training and test sets, only the test set data are included as metrics. If multiple models were used simultaneously in a given study, we only select the model with the median AUROC value. Continuous variables were described using mean difference (MD) as well as 95% confidence interval (CI), and were considered statistically significant when P<0.05. Heterogeneity was assessed according to discordance index (I2) (14). If I2<50%, it indicated low heterogeneity of Meta-analysis results and a fixed-effect model could be selected. Contrary, if I2≧50% that indicated high heterogeneity of Meta-analysis results and a random-effect model could be selected. Funnel plots and Egger tests (15) were used to assess whether there was publication bias in the results of Meta-analysis. When publication bias existed, the results of Meta-analysis were further analyzed for stability and reliability using the cut-and-patch method. In addition, sensitivity analysis was used to assess the robustness of the results of Meta-analysis. Sensitivity analyses excluding one study at a time were conducted to clarify whether the results were driven by one large study or a study with an extreme result.
In total, 71 studies were identified after removing duplicates, but 20 studies with non-compliant titles and abstracts were excluded. After full-text screening of the remaining 51 studies, only 26 studies met the requirements, but 15 of them had insufficient data, and the last 11 studies were used in our Meta-analysis (16–25). Figure 1 shows the selection process of our study.
We finally selected 11 studies for meta-analysis, and the characteristics of each study are summarized in Table 2. All of the studies we screened were retrospective, and two of them had independent validation set. Four studies built deep learning models and seven built radiomics models. In addition, the gold standard of diagnosis in most studies is pathology. In these studies, 3 types of medical imaging were used, 3 with ultrasound, 6 with MRI, and only 2 with CT. The results of the Meta-analysis of the AUROC values are presented in the form of forest plots in Figure 2.
QUADAS-2 was used to assess the risk of bias in the study, and the results are shown in Figure 3. For patient selection, all studies were low risk of bias. However, risk of bias was unclear of flow and timing for all 11 studies. For index test, 9 studies (81.82%) with high risk of bias, 2 studies (18.18%) with low. 9 studies (81.82%) with low and 2 (18.18%) with unclear risk of bias in reference standard. Table S1 shown individual evaluation of the risk of bias and applicability. For applicability concerns, overall risk is low. Funnel plot (Figure S1) and Egger test were used to evaluate whether publication bias existed in the results of the meta-analysis. When publication bias exists, shear and supplement method is used to further analyze whether the results of meta-analysis are stable and reliable (Figure 4). In addition, sensitivity analysis was used to evaluate whether the results of the meta-analysis were robust (Figure S2).
In these studies, the AUROC, sensitivity and specificity were used to assess the diagnostic performance of models. The categorized data extraction for each study report is shown in Table 3. As shown in Figure 2, AI models based on ultrasound had the best diagnostic performance, followed by MRI, and CT was the worst. The pooled AUROC of studies based on ultrasound, MRI and CT were 0.94 (95% CI 0.88-1.00), 0.82 (95% CI 0.71-0.93) and 0.82 (95% Cl 0.78-0.86), respectively. In addition, the heterogeneity of all these studies was high, the values of I2 reached 99.92%, 99.91% and 92.64% based on ultrasound, MRI and CT. The combined AUROC of all 11 included studies was 0.85 (95%CI 0.81-0.89) and I2 was 99.88%.
Medical imaging is the most effective way to assist clinical diagnosis and analyzing the condition for doctors. Imaging method is important for patients with OC because different images help to determine the feasibility of surgical approach and treatment (4). Our review is the first meta-analysis to evaluate the ability of artificial intelligence to identify benign and malignant ovarian cancer under different imaging modalities.
AI based medical imaging break through the technical barriers of traditional methods which have used in clinical practice, assisting physicians in lesion identification and diagnosis, efficacy assessment, and survival prognosis to improve diagnostic efficiency of doctors (26). Diagnosis of ovarian tumors still requires surgical removal, and the surgeon’s decision making is sometimes challenging in cases where preoperative examination finds atypical. Therefore, if AI can calculate the probability of ovarian cancer based on the results of preoperative examination and predict the final diagnosis, the management level of ovarian cancer will be improved (27). Benign ovarian tumors can avoid unnecessary surgery, and early diagnosis of ovarian cancer can improve the prognosis. In addition, for preoperative diagnosis, patients can receive a more informative probabilistic numerical interpretation (28). Preoperative diagnosis is more accurate and specific in the probability of ovarian tumor management decisions (29). AI extracts features from different types of images differently, and our study shows that the features extracted based on ultrasound images are better overall for the diagnosis of OC (27). Our results are consistent with a previous study which confirmed ultrasound was effective tools to characterize ovarian masses (30).
A total of 11 studies were included in our analysis, of which three were based on ultrasound, six were based on MRI and two based on CT. However, only three of them built deep learning models. This may be due to the fact that deep learning techniques are relatively new and prone to bias. In a recent study, the authors selected the best performed model to extract data for meta-analysis, but in our study, if multiple models were built in a study, we chose the model with the median AUROC value, which may better reflect the overall diagnostic performance of the models in a study. Finally, although most studies divided patients into training and test sets, most of them were monocentric and external validation was particularly important in the study.
However, there are some limitations to our study. First, scanning parameters (including field intensity, contrast agent type, injection velocity, etc.) are not uniform, and the analysis software is different. Then studies that only include Chinese and English literature may have some linguistic bias; In addition, the vast majority of the study’s first authors were from China, as were most of the cases, so there may be some bias. We should also critically consider some methodological issues. Modern information processing techniques to develop radiology report databases can improve report retrieval and help radiologists make diagnoses (31). We need to advocate for Internet networks to identify patient data from all over the world, and large-scale training of AI based on different patient demographics, geographic regions, diseases, and so on. In addition, we highlight the need for a more diverse database of images for rare cancers, including OC.
AI can play an adjunctive role in identifying benign and malignant ovarian tumors, and the models based on ultrasound has the best diagnostic ability, but due to the limitations of the number and quality of included studies, the above conclusions need to be viewed with caution, and more standardized and prospective studies need to be conducted to confirm them.
In conclusion, AI algorithms show good performance in diagnosing OC through medical imaging. Stricter reporting standards that address specific challenges in AI research could improve future research.
All authors had full access to all the data in the study and take responsibility for the integrity of the data and the accuracy of the data analysis. QX designed the study. ND, HL and ZL acquired the study data. CY and HW analyzed and interpreted the data. ML wrote the first draft of the manuscript. All authors revised the manuscript and approved it for publication.
Sichuan Medical Association project (Grant No. 2019HR65).
The authors declare that the research was conducted in the absence of any commercial or financial relationships that could be construed as a potential conflict of interest.
All claims expressed in this article are solely those of the authors and do not necessarily represent those of their affiliated organizations, or those of the publisher, the editors and the reviewers. Any product that may be evaluated in this article, or claim that may be made by its manufacturer, is not guaranteed or endorsed by the publisher.
The Supplementary Material for this article can be found online at: https://www.frontiersin.org/articles/10.3389/fonc.2023.1133491/full#supplementary-material
CT, Computer tomography; MRI, Magnetic resonance imaging; AI, Artificial intelligence; ML, machine learning; QUADAS-2, Quality Assessment of Diagnostic Accuracy Studies tool 2; AUROC, Area under the receiver operating characteristic curve.
1. Stewart C, Ralyea C, Lockwood S. Ovarian cancer: An integrated review. Semin Oncol Nurs (2019) 35(2):151–6. doi: 10.1016/j.soncn.2019.02.001
2. Eisenhauer EA. Real-world evidence in the treatment of ovarian cancer. Ann Oncol (2017) 28(suppl_8):viii61–65. doi: 10.1093/annonc/mdx443
3. Christiansen F, Epstein EL, Smedberg E, Åkerlund M, Smith K, Epstein E. Ultrasound image analysis using deep neural networks for discriminating between benign and malignant ovarian tumors: comparison with expert subjective assessment. Ultrasound Obstet Gynecol (2021) 57(1):155–63. doi: 10.1002/uog.23530
4. Mironov S, Akin O, Pandit-Taskar N, Hann LE. Ovarian cancer. Radiol Clin North Am (2007) 45(1):149–66. doi: 10.1016/j.rcl.2006.10.012
5. Shetty M. Imaging and Differential Diagnosis of Ovarian Cancer. Semin Ultrasound CT MR (2019) 40(4):302–18. doi: 10.1053/j.sult.2019.04.002
6. Hosny A, Parmar C, Quackenbush J, Schwartz LH, Aerts HJWL. Artificial intelligence in radiology. Nat Rev Cancer (2018) 18(8):500–10. doi: 10.1038/s41568-018-0016-5
7. Sogani J, Allen B Jr, Dreyer K, McGinty G. Artificial intelligence in radiology: the ecosystem essential to improving patient care. Clin Imaging (2020) 59(1):A3–6. doi: 10.1016/j.clinimag.2019.08.001
8. Campbell S, Gentry-Maharaj A. The role of transvaginal ultrasound in screening for ovarian cancer. Climacteric (2018) 21(3):221–6. doi: 10.1080/13697137.2018.1433656
9. Rizzo S, Del Grande M, Manganaro L, Papadia A, Del Grande F. Imaging before cytoreductive surgery in advanced ovarian cancer patients. Int J Gynecol Cancer (2020) 30(1):133–8. doi: 10.1136/ijgc-2019-000819
10. Kumar Y, Koul A, Singla R, Ijaz MF. Artificial intelligence in disease diagnosis: a systematic literature review, synthesizing framework and future research agenda [published online ahead of print, 2022 Jan 13]. J Ambient Intell Humaniz Comput (2022) 1-28. doi: 10.1007/s12652-021-03612-z
11. Bedrikovetski S, Dudi-Venkata NN, Maicas G, Kroon HM, Seow W, Carneiro G, et al. Artificial intelligence for the diagnosis of lymph node metastases in patients with abdominopelvic malignancy: A systematic review and meta-analysis. Artif Intell Med (2021) 113:102022. doi: 10.1016/j.artmed.2021.102022
12. Moher D, Liberati A, Tetzlaff J, Altman DG, PRISMA Group. Preferred reporting items for systematic reviews and meta-analyses: the PRISMA statement. PloS Med (2009) 6(7):e1000097. doi: 10.1371/journal.pmed.1000097
13. Whiting PF, Rutjes AW, Westwood ME, Mallett S, Deeks JJ, Reitsma JB, et al. QUADAS-2: a revised tool for the quality assessment of diagnostic accuracy studies. Ann Intern Med (2011) 155:529–36. doi: 10.7326/0003-4819-155-8-201110180-00009
14. Higgins JP, Thompson SG, Deeks JJ, Altman DG. Measuring inconsistency in meta-analyses. BMJ (2003) 327(7414):557–60. doi: 10.1136/bmj.327.7414.557
15. Egger M, Smith GD, Schneider M, Minder C. Bias in meta-analysis detected by a simple, graphical test. BMJ (1997) 315:629–34. doi: 10.1136/bmj.315.7109.629
16. Wang H, Liu C, Zhao Z, Zhang C, Wang X, Li H, et al. Application of deep convolutional neural networks for discriminating benign, borderline, and malignant serous ovarian tumors from ultrasound images. Front Oncol (2021) 11:770683. doi: 10.3389/fonc.2021.770683
17. Aramendía-Vidaurreta V, Cabeza R, Villanueva A, Navallas J, Alcázar JL. Ultrasound image discrimination between benign and malignant adnexal masses based on a neural network approach. Ultrasound Med Biol (2016) 42(3):742–52. doi: 10.1016/j.ultrasmedbio.2015.11.014
18. Wang R, Cai Y, Lee IK, Hu R, Purkayastha S, Pan I, et al. Evaluation of a convolutional neural network for ovarian tumor differentiation based on magnetic resonance imaging. Eur Radiol (2021) 31(7):4960–71. doi: 10.1007/s00330-020-07266-x
19. Li S, Liu J, Xiong Y, Pang P, Lei P, Zou H, et al. A radiomic s approach for automated diagnosis of ovarian neoplasm malignancy in computed tomography. Sci Rep (2021) 11(1):8730. doi: 10.1038/s41598-021-87775-x
20. Liu X, Wang T, Zhang G, Hua K, Jiang H, Duan S, et al. Two-dimensional and three-dimensional T2 weighted imaging-based radiomic signatures for the preoperative discrimination of ovarian borderline tumors and malignant tumors. J Ovarian Res (2022) 15(1):22. doi: 10.1186/s13048-022-00943-z
21. Zhuang J, Cheng M-y, Zhang L-j, Zhao X, Chen R, Zhang X-a. Differential analysis of benign and malignant ovarian tumors based on T2-Dixon hydrographic imaging model. J Med Forum (2002) 43(09):15–20. doi: 10.13437/j.cnki.jcr.2016.03.027
22. Zhang H, Mao YF, Chen XJ, Wu G, Liu X, Zhang P, et al. Magnetic resonance imaging radiomics in categorizing ovarian masses and predicting clinical outcome: a preliminary study. Eur Radiol (2019) 29(7):3358–71. doi: 10.1007/s00330-019-06124-9
23. Mimura R, Kato F, Tha KK, Kudo K, Konno Y, Oyama-Manabe, et al. Comparison between borderline ovarian tumors and carcinomas using semi-automated histogram analysis of diffusion-weighted imaging: focusing on solid components. Japanese J Radiol (2016) 34(3):229–37. doi: 10.1007/s11604-016-0518-6
24. Yu XP, Wang L, Yu HY, Zou YW, Wang C, Jiao JW, et al. MDCT-based radiomics features for the differentiation of serous borderline ovarian tumors and serous malignant ovarian tumors. Cancer Manag Res (2021) 13:329–36. doi: 10.2147/CMAR.S284220
25. Li C, Wang H, Chen Y, Fang M, Zhu C, Gao Y, et al. A nomogram combining MRI multisequence radiomics and clinical factors for predicting recurrence of high-grade serous ovarian carcinoma. J Oncol (2022) 2022:1716268. doi: 10.1155/2022/1716268
26. Gore JC. Artificial intelligence in medical imaging. Magn Reson Imaging (2020) 68:A1–4. doi: 10.1016/j.mri.2019.12.006
27. Akazawa M, Hashimoto K. Artificial intelligence in ovarian cancer diagnosis. Anticancer Res (2020) 40(8):4795–800. doi: 10.21873/anticanres.14482
28. Zhang L, Huang J, Liu L. Improved deep learning network based in combination with cost-sensitive learning for early detection of ovarian cancer in color ultrasound detecting system. J Med Syst (2019) 43(8):251. doi: 10.1007/s10916-019-1356-8
29. Sherbet GV, Woo WL, Dlay S. Application of artificial intelligence-based technology in cancer management: A commentary on the deployment of artificial neural networks. Anticancer Res (2018) 38(12):6607–13. doi: 10.21873/anticanres.13027
30. Elias KM, Guo J, Bast RC Jr. Early detection of ovarian cancer. Hematol Oncol Clin North Am (2018) 32(6):903–14. doi: 10.1016/j.hoc.2018.07.003
Keywords: ovarian cancer, AI, ultrasound, meta-analysis, systematic review
Citation: Ma L, Huang L, Chen Y, Zhang L, Nie D, He W and Qi X (2023) AI diagnostic performance based on multiple imaging modalities for ovarian tumor: A systematic review and meta-analysis. Front. Oncol. 13:1133491. doi: 10.3389/fonc.2023.1133491
Received: 29 December 2022; Accepted: 30 January 2023;
Published: 21 April 2023.
Edited by:
Paolo Scollo, Kore University of Enna, ItalyReviewed by:
Giancarlo Conoscenti, Azienda Ospedaliera per l’Emergenza Cannizzaro, ItalyCopyright © 2023 Ma, Huang, Chen, Zhang, Nie, He and Qi. This is an open-access article distributed under the terms of the Creative Commons Attribution License (CC BY). The use, distribution or reproduction in other forums is permitted, provided the original author(s) and the copyright owner(s) are credited and that the original publication in this journal is cited, in accordance with accepted academic practice. No use, distribution or reproduction is permitted which does not comply with these terms.
*Correspondence: Xiaoxue Qi, OTIwOTUwMTQzQHFxLmNvbQ==
Disclaimer: All claims expressed in this article are solely those of the authors and do not necessarily represent those of their affiliated organizations, or those of the publisher, the editors and the reviewers. Any product that may be evaluated in this article or claim that may be made by its manufacturer is not guaranteed or endorsed by the publisher.
Research integrity at Frontiers
Learn more about the work of our research integrity team to safeguard the quality of each article we publish.