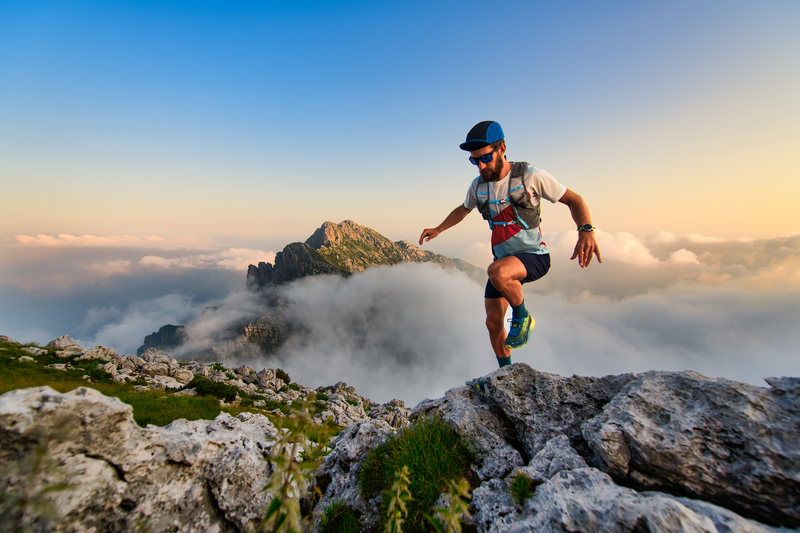
94% of researchers rate our articles as excellent or good
Learn more about the work of our research integrity team to safeguard the quality of each article we publish.
Find out more
REVIEW article
Front. Oncol. , 07 March 2023
Sec. Thoracic Oncology
Volume 13 - 2023 | https://doi.org/10.3389/fonc.2023.1133164
This article is part of the Research Topic World Lung Cancer Awareness Month 2022: Artificial Intelligence for clinical management of Lung Cancer View all 6 articles
Objectives: Lung cancer has been widely characterized through radiomics and artificial intelligence (AI). This review aims to summarize the published studies of AI based on positron emission tomography/computed tomography (PET/CT) radiomics in non-small-cell lung cancer (NSCLC).
Materials and methods: A comprehensive search of literature published between 2012 and 2022 was conducted on the PubMed database. There were no language or publication status restrictions on the search. About 127 articles in the search results were screened and gradually excluded according to the exclusion criteria. Finally, this review included 39 articles for analysis.
Results: Classification is conducted according to purposes and several studies were identified at each stage of disease:1) Cancer detection (n=8), 2) histology and stage of cancer (n=11), 3) metastases (n=6), 4) genotype (n=6), 5) treatment outcome and survival (n=8). There is a wide range of heterogeneity among studies due to differences in patient sources, evaluation criteria and workflow of radiomics. On the whole, most models show diagnostic performance comparable to or even better than experts, and the common problems are repeatability and clinical transformability.
Conclusion: AI-based PET/CT Radiomics play potential roles in NSCLC clinical management. However, there is still a long way to go before being translated into clinical application. Large-scale, multi-center, prospective research is the direction of future efforts, while we need to face the risk of repeatability of radiomics features and the limitation of access to large databases.
Lung cancer is one of the most common malignant tumors in the world and the leading cause of cancer-related death, with a five-year survival rate of 21.7% (1). Although this figure is higher than before, lung cancer is still a primary disease threatening human health. Non–small cell lung carcinoma (NSCLC) is the most common type of lung cancer, including adenocarcinoma, squamous cell carcinoma, adenosquamous carcinoma, large cell carcinoma, and sarcomatoid carcinoma (2). Tumor progression, prognosis evaluation, and determination of treatment plans are mainly dependent on the stage of the tumor and the histopathologic subtype. 18F-fluorodeoxyglucose positron emission tomography/computed tomography (18F-FDG PET/CT) is a fusion imaging technique that can reflect the anatomical structure and functional metabolic information of the lesions simultaneously. It has become one of the popular diagnostic tools in oncology due to abundant imaging information and high sensitivity it can provide. The guidelines of the National Comprehensive Cancer Network (NCCN) recommend 18F-FDG PET/CT for evaluation of patients from stage I to stage IV NSCLC and an incidentally detected lung nodule measuring more than 8 mm (3). In addition, 18F-FDG PET/CT also shows unique value in the prognosis and therapeutic response assessment (4–6).
Personalized medicine is the goal of modern cancer treatment, which aims to link genomic and clinical profiles of individual patients for more targeted therapies of tumors. Now, we recognize lung cancer as a molecularly heterogeneous disease and believe that understanding the link between underlying biology and clinical behavior is crucial for therapeutic decision-making (7). There is evidence that medical images can reflect the heterogeneity of tumors, such as cellular metabolism, necrosis, cancer-associated fibroblast, and expression of specific receptors (8, 9). Elevated glucose uptake is a hallmark of cancer. According to previous studies, tumor size, histological subtypes, and prognosis of NSCLC had influences on fluorodeoxyglucose (FDG) uptake (10–12). The standardized uptake value (SUV) is a semi-quantitative index reflecting FDG uptake in PET/CT, and the higher SUV was believed to indicate the biological aggressiveness of tumors (13). However, it does not always accurately convey tumor responses. A positive PET finding can be caused by infection or inflammation (14), while false-negative findings can result from some small nodules, low cellular density in lesions such as ground-glass opacity, or low tumor avidity of FDG (15).In the past decade, it has been recognized that medical images contain more helpful information that can not be captured by the naked eye but can be obtained by computer extraction and analysis, leading to better disease management.
The introduction of radiomics analysis provides a promising new approach to cancer assessment. Radiomics is the application of an automatic data characterization algorithm to transform image data of the region of interest (ROI) into high-dimensional feature data that can be mined (16). In radiomics analysis, the image information is quantified for analysis by the computer, which strengthens the traditional manual diagnosis. A complete radiomics analysis pipeline involves many steps (17): i) identifying the clinical question and target patient cohorts, ii) determining the imaging mode for radiomics analysis, iii)standardizing image acquisition and reconstruction, iv) segmenting tumor and defining ROI, v) extracting and selecting features, vi) building models with different machine learning algorithms, vii)training and validating the radiomics model. Figure 1 displays a workflow of radiomics analysis (18). To facilitate the clinical translation of radiomics, it is necessary to improve the robustness and repeatability of imaging markers. So far, many researchers have made efforts to this end. A set of 169 radiomics features was standardized by the Image Biomarker Standardization Initiative (IBSI) (19) so that different radiomics software can be verified and calibrated.
Figure 1 A workflow of radiomics analysis (18). (A) Image acquisition and ROI segmentation. (B) Extraction of radiomic features. (C) Model development.
The term “artificial intelligence” was coined by John McCarthy in 1955, defined as “the science and engineering of making intelligent machines” (20). Today, AI is developing rapidly in many fields, and the definitions in different kinds of literature are abundant and confusing. The AI mentioned in this review specifically refers to the virtual branch of medicine (20), that is, AI technology represented by machine learning (ML) and deep learning (DL). According to their purposes and how the underlying machine is taught, ML algorithms can be divided into three categories: supervised, unsupervised and semi-supervised (21). Currently, most ML algorithms under the background of predictive oncology are based on supervised learning. Several classical ML algorithms include random forest (RF), Support Vector Machine (SVM), Decision Trees, Naïve Bayes, and k-nearest Neighbors (22). As the state-of-the-art subcategory of ML, deep learning is essentially a variant of the artificial neural network. In deep learning, the research of the Convolution neural network(CNN) (23) has attracted much attention. CNN is a kind of multi-layer feed-forward neural network, while low-level image features are extracted by early hidden layers, and progressively higher features are learned by successive layers before classification by different classifiers. Programs have been created to automatically perform all radiomics analysis tasks from tumor segmentation to model building using AI (24–27). At present, radiomics-based ML models (or DL models) are widely developed for whole-process management of lung cancer: diagnosis, staging, treatment planning, and prognosis, so on. Some results have reported superior performance compared to traditional statistical methods or even better than radiologists.
The primary purpose of this review is to sort out and summarize the application of 18F-FDG PET/CT radiomics combined with artificial intelligence in the disease management of NSCLC, as well as opportunities and challenges.
A comprehensive search of papers published from 2012 to 2022 was conducted on the PubMed database. The following keywords and Medical Subject Heading (MeSH) words are used to generate different searches: “Carcinoma, Non-Small-Cell Lung”[Mesh], “lung neoplasm” [Mesh], “lung cancer”, “Positron Emission Tomography Computed Tomography”[Mesh], “Positron-Emission Tomography”[Mesh], “radiomic*”, “Artificial Intelligence”[Mesh], “Machine Learning”[Mesh], “Deep Learning” [Mesh]. We applied the following exclusion criteria: (1) Studies not aimed at assisting clinical management decision-making of NSCLC, such as screening, diagnosing, characterizing, predicting outcomes, or evaluating prognosis;(2)Studies not combinate PET/CT radiomics and artificial intelligence simultaneously;(3) Studies focused on methodology and technology of radiomics or AI, such as image segmentation, image denoising, comparison of feature selection methods; (4) Articles without original data, such as reviews and editorials. No study was excluded due to language and geographical location. About 127 articles in the search results were screened and gradually excluded according to the criteria in Figure 2. Then, this review included 39 articles for final analysis. We identified several articles at every step of disease management: Cancer detection(n=8), histology and stage of cancer(n=11), metastases(n=6), genotype(n=6), treatment outcome, and survival(n=8).
Lung cancer often occurs in the form of pulmonary nodules in the early stage, with a diameter of no more than 3 cm (28). Although the wide application of thin-layer CT has greatly improved the detection rate of pulmonary nodules, accurate localization and characterization is still a difficult problem for radiologists, especially when the nodules are very small. Compared with CT imaging, PET/CT shows a stronger ability to detect solitary pulmonary nodules. One large cohort study of patients with tumors reported that FDG-negative sub-centimeter pulmonary nodules are benign in 98% (29). It is recognized that integrating AI into radiomics can further reduce the false positive rate of diagnosis (30, 31). Traditional AI-assisted diagnosis is only based on CT features, so it is possible to misdiagnose vascular or non-nodular areas as solitary pulmonary nodules, resulting in a high false positive rate. Based on the ability of PET imaging to extract phenotypic and functional tumor heterogeneity information, predictive models have been established to extract quantitative features from PET/CT to distinguish benign and malignant nodules. Almost all studies achieved the best performance of the model by combining PET and CT features. Most prediction models for differentiating benign and malignant pulmonary nodules are developed based on machine learning algorithms of SVM. Support vector machine (SVM) is a generalized linear binary classifier through supervised learning, building the decision function based on a specific kernel function and the penalty parameter to avoid over-fitting. It is especially suitable for the case of a limited sample size. In a retrospective analysis, after adding delayed PET features, the SVM model shows higher diagnostic accuracy than the physician evaluation and common clinical indicators (32).
The deep learning network shows excellent performance in the prediction model. A study found that the artificial neural network showed excellent predictive value in estimating the likelihood of malignancy (AUC 0.981). The sensitivity and specificity corresponding to the optimal critical point of the ROC curve were 100% and 93.1%, respectively (33). Some studies try to realize automation from the definition of regions of interest, a high-resolution network (34) is proposed to automatically detect and classify pulmonary nodules without any stride or pooling to retain the high-resolution imaging features.
Not only lung cancer but also some benign diseases have high FDG uptake, such as granulomatous disease and inflammatory pseudotumor. Zhang et al. (35) reported that the SurfaceVolumeRatio of the CT-radiomics features and SUVpeak of the PET metabolic parameters have significance in differentiating benign and malignant lung lesions. And SurfaceVolumeRatio has a unique ability to distinguish granulomatous lesions from inflammatory pseudotumors. Sarcoidosis and lymphoma often present similar features on PET/CT, such as severely swollen lymph nodes and hypermetabolic changes involving multiple systems throughout the body. A study differentiates sarcoidosis and lymphomas with seven different feature selection approaches and four different ML classifiers. At the lesion level, they found highly accurate signatures to create models to differentiate cancer vs. sarcoidosis (AUC 0.94) and Hodgkin vs. diffuse large B-cell lymphoma (AUC 0.95) (36). In a more extensive cohort retrospective study (37), the researchers used a CNN to automatically locate and classify PET uptake patterns in lung cancer and lymphoma. It is confirmed that the addition of the atlas information (AUC 0.99) was the best feature combination, which performed better compared with the PET/CT with maximum intensity projection combination (P <0.001). We summarized the main findings on lung nodule prediction of malignancy in Table 1.
NSCLC is a group of heterogeneous diseases, of which lung adenocarcinoma and lung squamous cell carcinoma are the most common subtypes. Different subtypes of NSCLC have different phenotypic and biological characteristics, leading to significant differences in disease progression and prognosis (7). Hence, it is necessary to make different treatment plans for different subtypes.
The gold standard of histological classification is pathological evaluation. At present, many techniques can be used for tissue diagnosis and the preferred choice is image-guided needle core biopsy (38). Unfortunately, as an invasive examination, the operational risks of biopsy are inevitable, including a series of complications such as infection. Moreover, the spatio-temporal pathological heterogeneity of tumors limits the ability to capture biodiversity or disease evolution in a single biopsy (39). By contrast, the extraction of quantitative radiological features from non-invasive imaging can reflect a wealth of information about genotype, tumor microenvironment, and sensitivity to treatment (17, 40). Unlike most previous studies that explored only one model, recent studies compared the combination of different feature selection methods and machine learning algorithms to build models and evaluate the best performance (41). The RF model and SVM model performed well in many studies, and deep learning is superior to all traditional machine learning. Han et al. extracted 688 features from each ROI, and finally selected the top 50 features of each feature selection method for analysis. The best performance of subtype classification was achieved when linear discriminant analysis (AUC 0.863) and SVM (AUC 0.863) classifiers were combined with the L2,1NR feature selection method. In addition, they evaluated the VGG16 deep learning model based on transfer learning, outperforming all traditional machine learning algorithms (AUC 0.903) (42). Zhou et al. evaluated five feature selection methods and nine classification methods, the gradient boosting decision tree and the RF was considered optimal classification methods for the PET and CT datasets, respectively (43). Some studies have shown that the ability of the model to distinguish histological subtypes can be further improved by combining clinical features and tumor markers (44). Zhao et al. use the Boruta algorithm to determine an optimal subset of 13 features, including two clinical features(sex and smoking history), two laboratory indicators(CEA and SCCA), and nine PEF/CT radiomic features (38). The clinical features they included were the same as those of Ren et al (44), but there were differences in laboratory indicators. Ren et al. proposed that SCCA and CYFRA21-1 were statistically significant, while CEA levels were not statistically different between adenocarcinoma and squamous cell carcinoma patients.
The TNM staging system is a standard tool for evaluating the primary tumor, regional lymph node metastasis (LNM), and distant metastasis (45). In previous work, the CNN algorithm was developed and tested to distinguish T1-T2 from T3-T4 lung cancer on PET/CT images, achieving 69% accuracy, 70% recall and 67% specificity in the test set (46). Recently, Kasinathan et al. proposed a Cloud-based Lung Tumor Detector and Stage Classifier based on PET/CT images. The model achieved an average classification accuracy of 99.1% and an average accuracy of 98.6% (47). We summarized the main findings on histology and staging in Table 2.
NSCLC is usually accompanied by metastasis of mediastinal and hilar lymph nodes, and the identification of LNM significantly affects the staging, prognosis and treatment. Yin et al. developed a classification method based on SVM to improve the diagnostic performance of LNM. They converted the predictive model including the optimal feature SURblood into scoring rules to help clinicians make decisions (48). To solve the time-consuming problem caused by manual localization of nodules in previous studies, Wallis et al. used a U-Net to automatically identify candidate regions on PET/CT images and a 3D CNN model to classify regional lymph nodes. Through further fine-tuning the model using transfer learning, their model achieved good performance on test sets of different scanners (49).
Furthermore, there is also great clinical significance in the distinction of second primary lung cancers from pulmonary metastases because of the vastly different survival outcomes between them (50). D’Arnese et al. proposed LuCIFEx, a fully automated three-stage pipeline for the characterization of NSCLC. Similarly, LuCIFEx pipeline automatically segmented lesions on PET/CT images without manual annotation, and output the results through RF classification method after calculating the accurate radiation features. The accuracy of their model in distinguishing primary NSCLC from lung metastases is 92.3 ± 1.9% (51).
Over the past two decades, the emergence of various targeted therapies and immunotherapies has provided more individualized treatment decisions for patients with advanced NSCLC. The diagnosis of tumors at the molecular level is crucial for the accurate determination of targeted therapy. Different driver mutations have been identified in NSCLC, including oncogenic mutations in epidermal growth factor receptor (EGFR), anaplastic lymphoma kinase (ALK), BRAF, ROS1, and MET (52). If feasible, NCCN recommends that eligible patients with metastatic NSCLC be tested for molecular and biomarkers of disease-related mutations before starting treatment (1).
Early identification of EGFR mutational status is important before tyrosine kinase inhibitors treatment. There have been conflicting results that 18F-FDG uptakes may not be a reliable marker for predicting EGFR mutation status (53, 54). Present studies have validated that it is more convincing to use some PET/CT radiological features to predict EGFR mutation state (55, 56). In one large multi-center study (57), a small-residual-convolutional-network model was proposed to distinguish EGFR-mutant type from wild type, and the deep learning score (DLS) performed well with the AUC of 0.81 in the external test cohort. There was apparent heterogeneity in clinical characteristics and image acquisition because the patient cohorts came from different institutions. However, this could be regarded as an advantage, because this heterogeneity can avoid the possibility of over-fitting caused by a specific patient cohort or imaging parameters to some extent, resulting in a more robust and portable model. A similar DLS was also used to predict programmed death-ligand 1(PD-L1) status, which is the checkpoint target determined by immunohistochemistry. It was shown that the PD-L1 DLS significantly discriminated between PD-L1 positive and negative patients (AUC≥0.82 in the training, validation, and two external test cohorts) (58).
Identifying EFGR mutation subtypes is essential to guide more accurate personalized treatment. Increasing evidence has demonstrated that patients with E19 del mutation have a higher response to EGFR tyrosine kinase inhibitors (TKIs) treatment than those with E21 mis mutation, and have longer survival time (59). Liu et al. extracted and selected two sets of PET/CT radiomic features to identify E19 del mutation or E21 mis mutation, the AUC of radiomics features of the two groups is 0.77 and 0.92 respectively (60). The main clinical tools for detecting ALK rearrangement include immunohistochemistry and fluorescence in situ hybridization. Chang et al (61). firstly proposed a novel ML model to predict the ALK mutation status based on PET/CT images. They also confirmed that age and pleural effusion were independent predictors. The PET/CT-clinical combined model has a significant advantage in predicting the ALK mutation status, with the highest AUC value (0.88) in the test group. In addition, another study has successfully predicted the tumor immune microenvironment phenotype of NSCLC based on the PET/CT-clinical combined model (62). We summarized the main findings on genotype in Table 3.
One goal of radiomics is to construct models for predicting treatment response and prognosis from medical images in the context of tumor characteristics. Radiological criteria are usually used as a substitute for pathology to evaluate the response of tumor treatment, including the definitions of complete response, partial response, stable disease, and progressive disease (63). The evaluation of the efficacy of World Health Organization (WHO) criteria or Response Evaluation Criteria in Solid Tumors (RECIST) was entirely based on the anatomical size of the tumor. Since nuclear medical imaging can provide information on metabolic activity other than anatomical imaging, PET/CT is particularly valuable in evaluating tumor treatment response (64). PET Response Criteria in Solid Tumors (PERCIST 1.0) provides a systematic and structured method for assessing response to therapy in cancer patients with 18F-FDG PET (65).
In the field of radiomics, several studies have been published to evaluate the response to radiotherapy or immunotherapy based on ML algorithms, but most of them are based on CT images (66). Yoo et al. firstly constructed an RF model by extracting texture features from PET/CT images to assess the neoadjuvant concurrent chemoradiotherapy (CCRT) response of patients with stage III NSCLC. The pathological complete response after neoadjuvant CCRT is an independent prognostic factor for recurrence-free survival (RFS), and overall survival (OS) in NSCLC (67). In comparison with PET parameters and physician reporting, the ML model had a significantly higher accuracy of 93.4% (p < 0.05) (68).
Stereotactic body radiotherapy (SBRT) is now a standard of care option for patients with NSCLC, especially those not candidates for surgery. In some previous studies, the radiomics features used to build models differed significantly, which may be attributed to different patient cohorts, scanners, and segmentation/intensity discretization schemes (69). These radiomics features that were not in conformity with the IBSI standardization framework were not repetitive and comparative. It was demonstrated that the ComBat harmonization method (70) could coordinate the radiomics features derived from different acquisition and reconstruction parameters, including PET, CT and MRI imaging of NSCLC, nervous system and cervical cancer (71). Dissaux et al. applied this algorithm to a multi-center study, and by combining one feature from PET, and one from CT, the best-performing model was obtained, reaching a sensitivity of 100% and a specificity of 96% (72).
The European Society for Medical Oncology (EMSO) recommends that NSCLC patients treated with radical intent should be followed for treatment-related complications and detection of treatable relapse (73). Ahn et al. established ML models to improve clinical recurrence risk stratification, and the contrast and busyness texture features from neighborhood grey-level difference matrix were proved to be the best two predictors (74). A multi-center study compared multiple feature selection methods and ML classification algorithms, constructing a risk-stratification model for predicting recurrence, RFS and OS (75). Their model showed consistency across validation and external test sets and provide more accurate results than traditional Cox proportional-hazards models. Another multi-center study explored the relationship between tumor immune status and RFS and OS in patients receiving immunotherapy through deep learning (76). Most of the current work is based on RFS and OS, the two leading indicators to be output as the results of prediction models. Although these studies have achieved varied success, they have focused on predicting the binary results at specific time points. Huang et al. extend features extracted with the CNN to the prediction of survival as a continuous outcome by incorporating the random survival forest model, which outperformed corresponding CT-only and PET-only models (77). We summarized the main findings on treatment outcome and survival in Table 4.
Radiomics has sprung up rapidly in the past decade, and developing reliable computer-aided diagnosis systems based on AI has been recognized as an important research field in medical imaging. Although radiology has been used in many diseases, lung cancer is the most widely studied and characterized malignant tumor so far (78). This review aims to introduce and summarize the published literature on the subject of PET/CT radiomics combined with AI in the field of NSCLC, including tumor detection, histology, staging, treatment response assessment, prognosis prediction, and prediction of recurrence and metastasis. An exciting news is that most of the models developed in these works performed well, with predictive performance comparable to or even better than that of human experts, which confirms that the hope is feasible to apply AI to medical images to assist radiologists in improving diagnostic accuracy and efficiency.
However, none of them has been applied to a certain scale of clinical trials, which means there is little high-quality evidence that the work of clinicians or the disease outcomes of patients have been improved.
The process of model building, training, verification, and testing is essential before moving on a technology to a clinical trial. After training a ML or DL model, a validation set is generally used for guiding the optimization of the parameters. The cross-validation method and random sampling are most commonly used to separate validation sets. Previous studies have reported that the performance of the training set and validation set of classifiers is generally optimistically biased under a limited sample size (79). To evaluate the real performance of the model in unknown situations, an independent test set is necessary, which was not learned by the model during the training process, preferably external (80). However, most of the published studies so far only contain cross-validation results, that is, confusing the meaning of verification set and test set.
Overviewing these works, we only found that one prospective study cohort was used as an external test cohort to test the model by Mu et al. (57), while the rest of the studies are all retrospective. One of the major reasons may be the long time-taking and great difficulty of prospective study design.
A large enough training sample size is crucial for developing a robust machine algorithm, especially deep learning depends on a large number of data sets (81). However, the available clinical data is limited. Even though there are some published multi-institution cohort studies, achieving a smooth flow of data among a wide range of institutions is difficult. An effective method used by deep learning developers to solve limited samples is transfer learning, that is, fine-tuning CNN models pre-trained from natural image datasets to medical image tasks. It is reported that transfer learning was generally beneficial in improving the training convergence and robustness of the CNN model (82).
Although we emphasize the need for multi-center research, there is a repeatable risk problem for radiology. For example, different scanning protocols and image reconstruction methods may lead to limited performance or result deviation of radiomics models. Selection of reproducible and robust features is an arduous and prudent work, and there is great heterogeneity between the radiological features extracted by the current work. Many variables, including scanner type, scan time, respiratory movements, reconstruction algorithms, voxel size, etc., may affect the repeatability of radiomics (69, 83).
There are two iterative reconstruction algorithms commonly used in PET/CT images: Ordered Subset Expectation Maximization (OSEM) and Block Sequential Regularized Expectation Maximization (BSREM). It seems that BSREM shows more sensitivity towards reconstruction. According to an evaluation by Schwyzer et al., AI performed significantly better on images with BSREM than OSEM (84). Another challenge is how to remove the image noise caused by the inherent characteristics of PET/CT equipment. Veenland et al. investigated the sensitivity of four different texture feature selection methods to image noise and blur, and found that the existence of noise reduced the discrimination performance of all texture features (85, 86).
Some features depend on different segmentation methods, which also affects the reproducibility and repeatability of radiomics. The traditional method is manual segmentation, which is undoubtedly time-consuming and labor-dependent, and variability between and within observers is inevitable (87). In recent years, automatic and semi-automatic segmentation techniques have been recommended to improve efficiency and reproducibility (88). Bi et al. proposed a recurrent fusion network (RFN) for automatic tumor segmentation on PET/CT imaging, which progressively fused the intermediary segmentation results from individual phases to minimize the risk of inconsistent feature learning. This result allows tumors with uneven texture to be segmented and consistent segmentation results can be achieved on various network backbones (26).
A prior article (89) evaluated studies in this field through the Transparent Reporting of a multivariable prediction model for Individual Prognosis or Diagnosis (TRIPOD) (90). Common problems included small datasets, a lack of clinically relevant comparators, independent testing, and standards for reporting the studies. Recently, a statement provided a consensus-based reporting guideline for the Developmental and Exploratory Clinical Investigations of Decision support systems driven by Artificial Intelligence (DECIDE-AI) (91). Unlike Tripod-AI, which focuses on specific research designs, Decision-AI is a reporting guideline for the evaluation phase for early, small-scale and real-time clinical evaluation of decision support systems based on AI. As we said, there are very few AI models that have been put into clinical application in the field of PET/CT radiomics, this report emphasizes that it is important to assess actual clinical performance at a small scale after the preclinical stage and before further large-scale clinical trials. Furthermore, the report emphasized the role of human in decision-making, and experts agreed that the variability of patients and users should be considered when evaluating artificial intelligence systems.
At present, no definitive conclusion can be drawn on which approach in radiomics and AI should be preferred. Compared with machine learning, deep learning has more advantages in tumor segmentation and processing big data sets, but its black box theory lacks explanation (92). At present, there is no universally accepted method to test trust in the context of clinical artificial intelligence, which can help explain why Decision-AI does not include interpretability and trust issues in its guidelines. In the future, joint strategic management is needed to improve the clinical translatability and practicability of radiomics and AI in order to gain the trust of users (93).
The disease management pattern of NSCLC has been greatly improved in the past few decades. The introduction of screening programs for high-risk groups has significantly improved the early diagnosis and survival rate of NSCLC, unfortunately, more than half of patients have locally advanced or metastatic diseases (stage III or IV) at the time of diagnosis (94). From this starting point, clinicians still face many difficulties. First, under the trend of personalized medicine, more accurate diagnosis and subtype classification of tumors are needed. It has been proved that immunohistochemical markers, such as thyroid transcription factor -1(TTF-1), Napsin A, CK5/6, P63 and P40, can help to improve the accuracy of histological diagnosis (95). However, as we have already mentioned, a single biopsy of a small specimen cannot reflect the whole tumor. By contrast, PET/CT radiomics has been considered to reflect intra-tumor heterogeneity. This is especially important for inoperable patients and large tumors that are inconvenient for histological evaluation of the whole tumor. On the basis of radiomics, radiogenomics is gradually emerging. Radiogenomics correlates a large number of radiomics features with genomic data to reveal the potential molecular mechanism, providing a promising means of non-invasive diagnosis at the molecular level (96).
With the successful introduction of molecular markers in the clinic, targeted therapy and immunotherapy have become important treatment options, but there are still some limitations in their development. There is no evidence of targeted therapy to cure non-small cell lung cancer. Patients treated with EGFR TKIs will develop drug resistance with long-term use, leading to disease progression (97). Immunotherapy may achieve a lasting response, but only a small number of selected patients benefit from it. In addition, subsequent immune-related adverse events (IrAEs), including checkpoint inhibitor pneumonia (CIP), have been widely reported (98). In this case, radiogenomics is expected to help effective treatment options and avoid expensive treatments that do not provide survival benefits, as well as predict treatment responses. In the articles we included in the analysis, there have been studies that simultaneously associated PET/CT radiomics with clinical outcomes and genomics. Mu et al. explored the alternative markers of PD-L1 expression based on PET/CT radiomics. The results revealed the biological driving force (hypoxia) behind deep learning and provided non-invasive decision for follow-up treatment (58). This is a direction of continuing efforts in the future, that is, radiogenomics data is expected to provide accurate diagnosis, tissue classification, treatment guidance and monitoring for NSCLC patients, so as to achieve more personalized patient care.
Radiomics and artificial intelligence are considered as potential tools for the management of non-small cell lung cancer in the future, but the clinical transferability of results is an urgent problem to be solved. At present, there are several initiatives and recommendations to regulate the treatment and standardization of research reports. In the future, multi-center, prospective, larger cohort research is necessary. To overcome the technical repeatability and reproducibility defects, the establishment of a large multi-agency cooperative database covering a variety of imaging protocols and equipment, clinical environment, and patient characteristics may be the best way. Considering human factors, future developers should apply AI based on PET/CT radiomics analysis to more clinical trials to evaluate the applicability of computer-aided diagnosis systems in the local clinical environment. Furthermore, derived radiogenomics is expected to provide new insights into cancer biology, and there is still a long way to explore to achieve non-invasive decision-making under accurate medical care.
QH was responsible for writing the manuscript and supplementing materials. KL and CY were responsible for proposing amendments and revising the manuscript. YW, RH, MG, and YX were responsible for reviewing the manuscript on the technical and clinical aspects. YH and LC was the overall guide and was responsible for the whole project. All authors contributed to the article and approved the submitted version.
This study was supported by the National Natural Science Foundation of China (81960496), Yunnan Fundamental Research Projects (202101AT070050, 202201AY070001-155), Yunnan health training project of high-level talents (H-2018006, D-2017001), Xing Dian Ying Cai Support Plan, Leading Talents of Yunnan Health System (L-201206), Joint Laboratory of International Cooperation of the Ministry of Education for Regional High incidence Cancer at High altitude (K1322215), Science and Technology Innovation Team of Diagnosis and Treatment for Glucolipid Metabolic Diseases in Kunming Medical University (CXTD202106), and Yunnan Cancer Clinical Medical Center (ZX2019-05-01).
The authors declare the research was conducted in the absence of any commercial or financial relationships that could be construed as a potential conflict of interest.
All claims expressed in this article are solely those of the authors and do not necessarily represent those of their affiliated organizations, or those of the publisher, the editors and the reviewers. Any product that may be evaluated in this article, or claim that may be made by its manufacturer, is not guaranteed or endorsed by the publisher.
1. Ettinger DS, Wood DE, Aisner DL, Akerley W, Bauman JR, Bharat A, et al. Non-small cell lung cancer, version 3.2022, NCCN clinical practice guidelines in oncology. J Natl Compr Canc Netw (2022) 20(5):497–530. doi: 10.6004/jnccn.2022.0025
2. Travis WD, Brambilla E, Nicholson AG, Yatabe Y, Austin JHM, Beasley MB, et al. The 2015 world health organization classification of lung tumors: Impact of genetic, clinical and radiologic advances since the 2004 classification. J Thorac Oncol (2015) 10(9):1243–60. doi: 10.1097/JTO.0000000000000630
3. Ettinger DS, Wood DE, Aisner DL, Akerley W, Bauman J, Chirieac LR, et al. Non-small cell lung cancer, version 5.2017, NCCN clinical practice guidelines in oncology. J Natl Compr Canc Netw (2017) 15(4):504–35. doi: 10.6004/jnccn.2017.0050
4. Sheikhbahaei S, Mena E, Yanamadala A, Reddy S, Solnes LB, Wachsmann J, et al. The value of FDG PET/CT in treatment response assessment, follow-up, and surveillance of lung cancer. AJR Am J Roentgenol (2017) 208(2):420–33. doi: 10.2214/AJR.16.16532
5. Huang YE, Tsai YH, Huang YJ, Lung JH, Ho KW, Yen TC, et al. (18)F-fluorodeoxyglucose PET/CT for early prediction of outcomes in patients with advanced lung adenocarcinomas and EGFR mutations treated with first-line EGFR-TKIs. Cancers (Basel) (2022) 14(6):1507. doi: 10.3390/cancers14061507
6. Mu W, Tunali I, Gray JE, Qi J, Schabath MB, Gillies RJ. Radiomics of (18)F-FDG PET/CT images predicts clinical benefit of advanced NSCLC patients to checkpoint blockade immunotherapy. Eur J Nucl Med Mol Imaging (2020) 47(5):1168–82. doi: 10.1007/s00259-019-04625-9
7. Herbst RS, Morgensztern D, Boshoff C. The biology and management of non-small cell lung cancer. Nature (2018) 553(7689):446–54. doi: 10.1038/nature25183
8. Shangguan C, Gan G, Zhang J, Wu J, Miao Y, Zhang M, et al. Cancer-associated fibroblasts enhance tumor (18)F-FDG uptake and contribute to the intratumor heterogeneity of PET-CT. Theranostics (2018) 8(5):1376–88. doi: 10.7150/thno.22717
9. Hyun SH, Kim HS, Choi SH, Choi DW, Lee JK, Lee KH, et al. Intratumoral heterogeneity of (18)F-FDG uptake predicts survival in patients with pancreatic ductal adenocarcinoma. Eur J Nucl Med Mol Imaging (2016) 43(8):1461–8. doi: 10.1007/s00259-016-3316-6
10. Hattori A, Matsunaga T, Takamochi K, Oh S, Suzuki K. Clinical significance of positron emission tomography in subcentimeter non-small cell lung cancer. Ann Thorac Surg (2017) 103(5):1614–20. doi: 10.1016/j.athoracsur.2016.09.059
11. Zhang H, Wroblewski K, Liao S, Kampalath R, Penney BC, Zhang Y, et al. Prognostic value of metabolic tumor burden from (18)F-FDG PET in surgical patients with non-small-cell lung cancer. Acad Radiol (2013) 20(1):32–40. doi: 10.1016/j.acra.2012.07.002
12. Li M, Sun Y, Liu Y, Han A, Zhao S, Ma L, et al. Relationship between primary lesion FDG uptake and clinical stage at PET-CT for non-small cell lung cancer patients: An observation. Lung Cancer (2010) 68(3):394–7. doi: 10.1016/j.lungcan.2009.07.009
13. Berghmans T, Dusart M, Paesmans M, Hossein-Foucher C, Buvat I, Castaigne C, et al. Primary tumor standardized uptake value (SUVmax) measured on fluorodeoxyglucose positron emission tomography (FDG-PET) is of prognostic value for survival in non-small cell lung cancer (NSCLC): A systematic review and meta-analysis (MA) by the european lung cancer working party for the IASLC lung cancer staging project. J Thorac Oncol (2008) 3(1):6–12. doi: 10.1097/JTO.0b013e31815e6d6b
14. Rahman WT, Wale DJ, Viglianti BL, Townsend DM, Manganaro MS, Gross MD, et al. The impact of infection and inflammation in oncologic (18)F-FDG PET/CT imaging. BioMed Pharmacother (2019) 117:109168. doi: 10.1016/j.biopha.2019.109168
15. Kandathil A, Kay FU, Butt YM, Wachsmann JW, Subramaniam RM. Role of FDG PET/CT in the eighth edition of TNM staging of non-small cell lung cancer. Radiographics (2018) 38(7):2134–49. doi: 10.1148/rg.2018180060
16. Lambin P, Rios-Velazquez E, Leijenaar R, Carvalho S, van Stiphout RG, Granton P, et al. Radiomics: Extracting more information from medical images using advanced feature analysis. Eur J Cancer (2012) 48(4):441–6. doi: 10.1016/j.ejca.2011.11.036
17. Papanikolaou N, Matos C, Koh DM. How to develop a meaningful radiomic signature for clinical use in oncologic patients. Cancer Imaging (2020) 20(1):33. doi: 10.1186/s40644-020-00311-4
18. Piñeiro-Fiel M, Moscoso A, Pubul V, Ruibal Á, Silva-Rodríguez J, Aguiar P, et al. A systematic review of PET textural analysis and radiomics in cancer. Diagnostics (Basel) (2021) 11(2):380. doi: 10.3390/diagnostics11020380
19. Zwanenburg A, Vallières M, Abdalah MA, Hjwl A, Andrearczyk V, Apte A, et al. The image biomarker standardization initiative: Standardized quantitative radiomics for high-throughput image-based phenotyping. Radiology (2020) 295(2):328–38. doi: 10.1148/radiol.2020191145
20. Hamet P, Tremblay J. Artificial intelligence in medicine. Metabolism (2017) 69s:S36–s40. doi: 10.1016/j.metabol.2017.01.011
21. Uddin S, Khan A, Hossain ME, Moni MA. Comparing different supervised machine learning algorithms for disease prediction. BMC Med Inform Decis Mak (2019) 19(1):281. doi: 10.1186/s12911-019-1004-8
22. Bibault JE, Giraud P, Burgun A. Big data and machine learning in radiation oncology: State of the art and future prospects. Cancer Lett (2016) 382(1):110–7. doi: 10.1016/j.canlet.2016.05.033
23. Chen B, Polatkan G, Sapiro G, Blei D, Dunson D, Carin L. Deep learning with hierarchical convolutional factor analysis. IEEE Trans Pattern Anal Mach Intell (2013) 35(8):1887–901. doi: 10.1109/TPAMI.2013.19
24. Samarasinghe G, Jameson M, Vinod S, Field M, Dowling J, Sowmya A, et al. Deep learning for segmentation in radiation therapy planning: A review. J Med Imaging Radiat Oncol (2021) 65(5):578–95. doi: 10.1111/1754-9485.13286
25. Avanzo M, Wei L, Stancanello J, Vallières M, Rao A, Morin O, et al. Machine and deep learning methods for radiomics. Med Phys (2020) 47(5):e185–202. doi: 10.1002/mp.13678
26. Bi L, Fulham M, Li N, Liu Q, Song S, Dagan Feng D, et al. Recurrent feature fusion learning for multi-modality pet-ct tumor segmentation. Comput Methods Programs BioMed (2021) 203:106043. doi: 10.1016/j.cmpb.2021.106043
27. Sepehri S, Tankyevych O, Iantsen A, Visvikis D, Hatt M, Cheze Le Rest C. Accurate tumor delineation vs. rough volume of interest analysis for (18)F-FDG PET/CT radiomics-based prognostic modeling inNon-small cell lung cancer. Front Oncol (2021) 11:726865. doi: 10.3389/fonc.2021.726865
28. Hansell DM, Bankier AA, Macmahon H, McLoud TC, Müller NL, Remy J. Fleischner society: glossary of terms for thoracic imaging. Radiology (2008) 246(3):697–722. doi: 10.1148/radiol.2462070712
29. Chang ST, Nguyen DC, Raptis C, Menias CO, Zhou G, Wang-Gillam A, et al. Natural history of preoperative subcentimeter pulmonary nodules in patients with resectable pancreatic adenocarcinoma: A retrospective cohort study. Ann Surg (2015) 261(5):970–5. doi: 10.1097/SLA.0000000000000719
30. Kang F, Mu W, Gong J, Wang S, Li G, Li G, et al. Integrating manual diagnosis into radiomics for reducing the false positive rate of (18)F-FDG PET/CT diagnosis in patients with suspected lung cancer. Eur J Nucl Med Mol Imaging (2019) 46(13):2770–9. doi: 10.1007/s00259-019-04418-0
31. Zhao J, Ji G, Qiang Y, Han X, Pei B, Shi Z. A new method of detecting pulmonary nodules with PET/CT based on an improved watershed algorithm. PloS One (2015) 10(4):e0123694. doi: 10.1371/journal.pone.0123694
32. Chen S, Harmon S, Perk T, Li X, Chen M, Li Y, et al. Diagnostic classification of solitary pulmonary nodules using dual time (18)F-FDG PET/CT image texture features in granuloma-endemic regions. Sci Rep (2017) 7(1):9370. doi: 10.1038/s41598-017-08764-7
33. Scott JA, Mcdermott S, Kilcoyne A, Wang Y, Halpern EF, Ackman JB. Comparison of (18)F-FDG avidity at PET of benign and malignant pure ground-glass opacities: A paradox? part II: Artificial neural network integration of the PET/CT characteristics of ground-glass opacities to predict their likelihood of malignancy. Clin Radiol (2019) 74(9):692–6. doi: 10.1016/j.crad.2019.04.024
34. Lai YC, Wu KC, Tseng NC, Chen YJ, Chang CJ, Yen KY, et al. Differentiation between malignant and benign pulmonary nodules by using automated three-dimensional high-resolution representation learning with fluorodeoxyglucose positron emission tomography-computed tomography. Front Med (Lausanne) (2022) 9:773041. doi: 10.3389/fmed.2022.773041
35. Zhang R, Zhu L, Cai Z, Jiang W, Li J, Yang C, et al. Potential feature exploration and model development based on 18F-FDG PET/CT images for differentiating benign and malignant lung lesions. Eur J Radiol (2019) 121:108735. doi: 10.1016/j.ejrad.2019.108735
36. Lovinfosse P, Ferreira M, Withofs N, Jadoul A, Derwael C, Frix AN, et al. Distinction of lymphoma from sarcoidosis at FDG PET/CT - evaluation of radiomic-feature guided machine learning versus human reader performance. J Nucl Med (2022) 63(12):1933–40. doi: 10.2967/jnumed.121.263598
37. Sibille L, Seifert R, Avramovic N, Vehren T, Spottiswoode B, Zuehlsdorff S, et al. (18)F-FDG PET/CT uptake classification in lymphoma and lung cancer by using deep convolutional neural networks. Radiology (2020) 294(2):445–52. doi: 10.1148/radiol.2019191114
38. Zhao H, Su Y, Wang M, Lyu Z, Xu P, Jiao Y, et al. The machine learning model for distinguishing pathological subtypes of non-small cell lung cancer. Front Oncol (2022) 12:875761. doi: 10.3389/fonc.2022.875761
39. Meacham CE, Morrison SJ. Tumour heterogeneity and cancer cell plasticity. Nature (2013) 501(7467):328–37. doi: 10.1038/nature12624
40. Zanfardino M, Franzese M, Pane K, Cavaliere C, Monti S, Esposito G, et al. Bringing radiomics into a multi-omics framework for a comprehensive genotype-phenotype characterization of oncological diseases. J Transl Med (2019) 17(1):337. doi: 10.1186/s12967-019-2073-2
41. Hyun SH, Ahn MS, Koh YW, Lee SJ. A machine-learning approach using PET-based radiomics to predict the histological subtypes of lung cancer. Clin Nucl Med (2019) 44(12):956–60. doi: 10.1097/RLU.0000000000002810
42. Han Y, Ma Y, Wu Z, Zhang F, Zheng D, Liu X, et al. Histologic subtype classification of non-small cell lung cancer using PET/CT images. Eur J Nucl Med Mol Imaging (2021) 48(2):350–60. doi: 10.1007/s00259-020-04771-5
43. Zhou Y, Ma XL, Zhang T, Wang J, Zhang T, Tian R. Use of radiomics based on (18)F-FDG PET/CT and machine learning methods to aid clinical decision-making in the classification of solitary pulmonary lesions: An innovative approach. Eur J Nucl Med Mol Imaging (2021) 48(9):2904–13. doi: 10.1007/s00259-021-05220-7
44. Ren C, Zhang J, Qi M, Zhang J, Zhang Y, Song S, et al. Machine learning based on clinico-biological features integrated (18)F-FDG PET/CT radiomics for distinguishing squamous cell carcinoma from adenocarcinoma of lung. Eur J Nucl Med Mol Imaging (2021) 48(5):1538–49. doi: 10.1007/s00259-020-05065-6
45. Kay FU, Kandathil A, Batra K, Saboo SS, Abbara S, Rajiah P. Revisions to the tumor, node, metastasis staging of lung cancer (8(th) edition):Rationale, radiologic findings and clinical implications. World J Radiol (2017) 9(6):269–79. doi: 10.4329/wjr.v9.i6.269
46. Kirienko M, Sollini M, Silvestri G, Mognetti S, Voulaz E, Antunovic L, et al. Convolutional neural networks promising in lung cancer t-parameter assessment on baseline FDG-PET/CT. Contrast Media Mol Imaging (2018) 2018:1382309. doi: 10.1155/2018/1382309
47. Kasinathan G, Jayakumar S. Cloud-based lung tumor detection and stage classification using deep learning techniques. BioMed Res Int (2022) 2022:4185835. doi: 10.1155/2022/4185835
48. Yin G, Song Y, Li X, Zhu L, Su Q, Dai D, et al. Prediction of mediastinal lymph node metastasis based on (18)F-FDG PET/CT imaging using support vector machine in non-small cell lung cancer. Eur Radiol (2021) 31(6):3983–92. doi: 10.1007/s00330-020-07466-5
49. Wallis D, Soussan M, Lacroix M, Akl P, Duboucher C, Buvat I. An 18F]FDG-PET/CT deep learning method for fully automated detection of pathological mediastinal lymph nodes in lung cancer patients. Eur J Nucl Med Mol Imaging (2022) 49(3):881–8. doi: 10.1007/s00259-021-05513-x
50. Zhong F, Liu Z, An W, Wang B, Zhang H, Liu Y, et al. Radiomics study for discriminating second primary lung cancers from pulmonary metastases in pulmonary solid lesions. Front Oncol (2021) 11:801213. doi: 10.3389/fonc.2021.801213
51. D'arnese E, Donato GWD, Sozzo ED, Sollini M, Sciuto D, Santambrogio MD. On the automation of radiomics-based identification and characterization of NSCLC. IEEE J BioMed Health Inform (2022) 26(6):2670–9. doi: 10.1109/JBHI.2022.3156984
52. Kris MG, Johnson BE, Berry LD, Kwiatkowski DJ, Iafrate AJ, Wistuba II, et al. Using multiplexed assays of oncogenic drivers in lung cancers to select targeted drugs. Jama (2014) 311(19):1998–2006. doi: 10.1001/jama.2014.3741
53. Mak RH, Digumarthy SR, Muzikansky A, Engelman JA, Shepard J A, Choi NC, et al. Role of 18F-fluorodeoxyglucose positron emission tomography in predicting epidermal growth factor receptor mutations in non-small cell lung cancer. Oncologist (2011) 16(3):319–26. doi: 10.1634/theoncologist.2010-0300
54. Choi YJ, Cho BC, Jeong YH, Seo HJ, Kim HJ, Cho A, et al. Correlation between (18)f-fluorodeoxyglucose uptake and epidermal growth factor receptor mutations in advanced lung cancer. Nucl Med Mol Imaging (2012) 46(3):169–75. doi: 10.1007/s13139-012-0142-z
55. Li X, Yin G, Zhang Y, Dai D, Liu J, Chen P, et al. Predictive power of a radiomic signature based on (18)F-FDG PET/CT images for EGFR mutational status in NSCLC. Front Oncol (2019) 9:1062. doi: 10.3389/fonc.2019.01062
56. Nair JKR, Saeed UA, Mcdougall CC, Sabri A, Kovacina B, Raidu BVS, et al. Radiogenomic models using machine learning techniques to predict EGFR mutations in non-small cell lung cancer. Can Assoc Radiol J (2021) 72(1):109–19. doi: 10.1177/0846537119899526
57. Mu W, Jiang L, Zhang J, Shi Y, Gray JE, Tunali I, et al. Non-invasive decision support for NSCLC treatment using PET/CT radiomics. Nat Commun (2020) 11(1):5228. doi: 10.1038/s41467-020-19116-x
58. Mu W, Jiang L, Shi Y, Tunali I, Gray JE, Katsoulakis E, et al. Non-invasive measurement of PD-L1 status and prediction of immunotherapy response using deep learning of PET/CT images. J Immunother Cancer (2021) 9(6):e002118. doi: 10.1136/jitc-2020-002118
59. Lim SH, Lee JY, Sun JM, Ahn JS, Park K, Ahn MJ. Comparison of clinical outcomes following gefitinib and erlotinib treatment in non-small-cell lung cancer patients harboring an epidermal growth factor receptor mutation in either exon 19 or 21. J Thorac Oncol (2014) 9(4):506–11. doi: 10.1097/JTO.0000000000000095
60. Liu Q, Sun D, Li N, Kim J, Feng D, Huang G, et al. Predicting EGFR mutation subtypes in lung adenocarcinoma using (18)F-FDG PET/CT radiomic features. Transl Lung Cancer Res (2020) 9(3):549–62. doi: 10.21037/tlcr.2020.04.17
61. Chang C, Sun X, Wang G, Yu H, Zhao W, Ge Y, et al. A machine learning model based on PET/CT radiomics and clinical characteristics predicts ALK rearrangement status in lung adenocarcinoma. Front Oncol (2021) 11:603882. doi: 10.3389/fonc.2021.603882
62. Tong H, Sun J, Fang J, Zhang M, Liu H, Xia R, et al. A machine learning model based on PET/CT radiomics and clinical characteristics predicts tumor immune profiles in non-small cell lung cancer: A retrospective multicohort study. Front Immunol (2022) 13:859323. doi: 10.3389/fimmu.2022.859323
63. Chetan MR, Gleeson FV. Radiomics in predicting treatment response in non-small-cell lung cancer: Current status, challenges and future perspectives. Eur Radiol (2021) 31(2):1049–58. doi: 10.1007/s00330-020-07141-9
64. Wahl RL, Jacene H, Kasamon Y, Lodge MA. From RECIST to PERCIST: Evolving considerations for PET response criteria in solid tumors. J Nucl Med (2009) 50(Suppl 1):122s–50s. doi: 10.2967/jnumed.108.057307
65. O JH, Lodge MA, Wahl RL. Practical PERCIST: A simplified guide to PET response criteria in solid tumors 1.0. Radiology (2016) 280(2):576–84. doi: 10.1148/radiol.2016142043
66. Guo X, Li Y, Yang C, Hu Y, Zhou Y, Wang Z, et al. Deep learning-based computed tomography imaging to diagnose the lung nodule and treatment effect of radiofrequency ablation. J Healthc Eng (2021) 2021:6556266. doi: 10.1155/2021/6556266
67. Schreiner W, Gavrychenkova S, Dudek W, Rieker RJ, Lettmaier S, Fietkau R, et al. Pathologic complete response after induction therapy-the role of surgery in stage IIIA/B locally advanced non-small cell lung cancer. J Thorac Dis (2018) 10(5):2795–803. doi: 10.21037/jtd.2018.05.68
68. Yoo J, Lee J, Cheon M, Woo SK, Ahn MJ, Pyo HR, et al. Predictive value of (18)F-FDG PET/CT using machine learning for pathological response to neoadjuvant concurrent chemoradiotherapy in patients with stage III non-small cell lung cancer. Cancers (Basel) (2022) 14(8):1987. doi: 10.3390/cancers14081987
69. Galavis PE, Hollensen C, Jallow N, Paliwal B, Jeraj R. Variability of textural features in FDG PET images due to different acquisition modes and reconstruction parameters. Acta Oncol (2010) 49(7):1012–6. doi: 10.3109/0284186X.2010.498437
70. Lucia F, Visvikis D, Vallières M, Desseroit MC, Miranda O, Robin P, et al. External validation of a combined PET and MRI radiomics model for prediction of recurrence in cervical cancer patients treated with chemoradiotherapy. Eur J Nucl Med Mol Imaging (2019) 46(4):864–77. doi: 10.1007/s00259-018-4231-9
71. Orlhac F, Boughdad S, Philippe C, Stalla-Bourdillon H, Nioche C, Champion L, et al. A postreconstruction harmonization method for multicenter radiomic studies in PET. J Nucl Med (2018) 59(8):1321–8. doi: 10.2967/jnumed.117.199935
72. Dissaux G, Visvikis D, Da-Ano R, Pradier O, Chajon E, Barillot I, et al. Pretreatment (18)F-FDG PET/CT radiomics predict local recurrence in patients treated with stereotactic body radiotherapy for early-stage non-small cell lung cancer: A multicentric study. J Nucl Med (2020) 61(6):814–20. doi: 10.2967/jnumed.119.228106
73. Remon J, Soria JC, Peters S. Early and locally advanced non-small-cell lung cancer: an update of the ESMO clinical practice guidelines focusing on diagnosis, staging, systemic and local therapy. Ann Oncol (2021) 32(12):1637–42. doi: 10.1016/j.annonc.2021.08.1994
74. Ahn HK, Lee H, Kim SG, Hyun SH. Pre-treatment (18)F-FDG PET-based radiomics predict survival in resected non-small cell lung cancer. Clin Radiol (2019) 74(6):467–73. doi: 10.1016/j.crad.2019.02.008
75. Hindocha S, Charlton TG, Linton-Reid K, Hunter B, Chan C, Ahmed M, et al. A comparison of machine learning methods for predicting recurrence and death after curative-intent radiotherapy for non-small cell lung cancer: Development and validation of multivariable clinical prediction models. EBioMedicine (2022) 77:103911. doi: 10.1016/j.ebiom.2022.103911
76. Park C, Na KJ, Choi H, Ock CY, Ha S, Kim M, et al. Tumor immune profiles noninvasively estimated by FDG PET with deep learning correlate with immunotherapy response in lung adenocarcinoma. Theranostics (2020) 10(23):10838–48. doi: 10.7150/thno.50283
77. Huang B, Sollee J, Luo YH, Reddy A, Zhong Z, Wu J, et al. Prediction of lung malignancy progression and survival with machine learning based on pre-treatment FDG-PET/CT. EBioMedicine (2022) 82:104127. doi: 10.1016/j.ebiom.2022.104127
78. Avanzo M, Stancanello J, Pirrone G, Sartor G. Radiomics and deep learning in lung cancer. Strahlenther Onkol (2020) 196(10):879–87. doi: 10.1007/s00066-020-01625-9
79. Sahiner B, Chan HP, Petrick N, Wagner RF, Hadjiiski L. Feature selection and classifier performance in computer-aided diagnosis: The effect of finite sample size. Med Phys (2000) 27(7):1509–22. doi: 10.1118/1.599017
80. Shen D, Wu G, Suk HI. Deep learning in medical image analysis. Annu Rev BioMed Eng (2017) 19:221–48. doi: 10.1146/annurev-bioeng-071516-044442
81. Ardila D, Kiraly AP, Bharadwaj S, Choi B, Reicher JJ, Peng L, et al. End-to-end lung cancer screening with three-dimensional deep learning on low-dose chest computed tomography. Nat Med (2019) 25(6):954–61. doi: 10.1038/s41591-019-0447-x
82. Shin HC, Roth HR, Gao M, Lu L, Xu Z, Nogues I, et al. Deep convolutional neural networks for computer-aided detection: CNN architectures, dataset characteristics and transfer learning. IEEE Trans Med Imaging (2016) 35(5):1285–98. doi: 10.1109/TMI.2016.2528162
83. Grootjans W, Tixier F, van der Vos CS, Vriens D, Le Rest CC, Bussink J, et al. The impact of optimal respiratory gating and image noise on evaluation of intratumor heterogeneity on 18F-FDG PET imaging of lung cancer. J Nucl Med (2016) 57(11):1692–8. doi: 10.2967/jnumed.116.173112
84. Schwyzer M, Martini K, Benz DC, Burger IA, Ferraro DA, Kudura K, et al. Artificial intelligence for detecting small FDG-positive lung nodules in digital PET/CT: impact of image reconstructions on diagnostic performance. Eur Radiol (2020) 30(4):2031–40. doi: 10.1007/s00330-019-06498-w
85. Veenland JF, Grashuis JL, Gelsema ES. Texture analysis in radiographs: the influence of modulation transfer function and noise on the discriminative ability of texture features. Med Phys (1998) 25(6):922–36. doi: 10.1118/1.598271
86. Chicklore S, Goh V, Siddique M, Roy A, Marsden PK, Cook GJ. Quantifying tumour heterogeneity in 18F-FDG PET/CT imaging by texture analysis. Eur J Nucl Med Mol Imaging (2013) 40(1):133–40. doi: 10.1007/s00259-012-2247-0
87. Kothari G, Woon B, Patrick CJ, Korte J, Wee L, Hanna GG, et al. The impact of inter-observer variation in delineation on robustness of radiomics features in non-small cell lung cancer. Sci Rep (2022) 12(1):12822. doi: 10.1038/s41598-022-16520-9
88. Hatt M, Lee JA, Schmidtlein CR, Naqa IE, Caldwell C, De Bernardi E, et al. Classification and evaluation strategies of auto-segmentation approaches for PET: Report of AAPM task group no. 211. Med Phys (2017) 44(6):e1–e42. doi: 10.1002/mp.12124
89. Krarup MMK, Krokos G, Subesinghe M, Nair A, Fischer BM. Artificial intelligence for the characterization of pulmonary nodules, lung tumors and mediastinal nodes on PET/CT. Semin Nucl Med (2021) 51(2):143–56. doi: 10.1053/j.semnuclmed.2020.09.001
90. Collins GS, Reitsma JB, Altman DG, Moons KG. Transparent reporting of a multivariable prediction model for individual prognosis or diagnosis (TRIPOD): the TRIPOD statement. Bmj (2015) 350:g7594. doi: 10.1161/CIRCULATIONAHA.114.014508
91. Vasey B, Nagendran M, Campbell B, Clifton DA, Collins GS, Denaxas S, et al. Reporting guideline for the early-stage clinical evaluation of decision support systems driven by artificial intelligence: DECIDE-AI. Nat Med (2022) 28(5):924–33. doi: 10.1038/s41591-022-01772-9
92. Talukder A, Barham C, Li X, Hu H. Interpretation of deep learning in genomics and epigenomics. Brief Bioinform (2021) 22(3):bbaa177. doi: 10.1093/bib/bbaa177
93. Sollini M, Antunovic L, Chiti A, Kirienko M. Towards clinical application of image mining: A systematic review on artificial intelligence and radiomics. Eur J Nucl Med Mol Imaging (2019) 46(13):2656–72. doi: 10.1007/s00259-019-04372-x
94. Duma N, Santana-Davila R, Molina JR. Non-small cell lung cancer: Epidemiology, screening, diagnosis, and treatment. Mayo Clin Proc (2019) 94(8):1623–40. doi: 10.1016/j.mayocp.2019.01.013
95. Osmani L, Askin F, Gabrielson E, Li QK. Current WHO guidelines and the critical role of immunohistochemical markers in the subclassification of non-small cell lung carcinoma (NSCLC):Moving from targeted therapy to immunotherapy. Semin Cancer Biol (2018) 52(Pt 1):103–9. doi: 10.1016/j.semcancer.2017.11.019
97. Cooper AJ, Sequist LV, Lin JJ. Third-generation EGFR and ALK inhibitors: mechanisms of resistance and management. Nat Rev Clin Oncol (2022) 19(8):499–514. doi: 10.1038/s41571-022-00639-9
Keywords: PET/CT, NSCLC, radiomics, artificial intelligence, lung cancer
Citation: Hu Q, Li K, Yang C, Wang Y, Huang R, Gu M, Xiao Y, Huang Y and Chen L (2023) The role of artificial intelligence based on PET/CT radiomics in NSCLC: Disease management, opportunities, and challenges. Front. Oncol. 13:1133164. doi: 10.3389/fonc.2023.1133164
Received: 28 December 2022; Accepted: 20 February 2023;
Published: 07 March 2023.
Edited by:
Parul Agarwal, Jamia Hamdard University, IndiaReviewed by:
Xiaofei Hu, Army Medical University, ChinaCopyright © 2023 Hu, Li, Yang, Wang, Huang, Gu, Xiao, Huang and Chen. This is an open-access article distributed under the terms of the Creative Commons Attribution License (CC BY). The use, distribution or reproduction in other forums is permitted, provided the original author(s) and the copyright owner(s) are credited and that the original publication in this journal is cited, in accordance with accepted academic practice. No use, distribution or reproduction is permitted which does not comply with these terms.
*Correspondence: Long Chen, bG9uZWNoZW4xOTgzQGhvdG1haWwuY29t; Yunchao Huang, aHVhbmd5dW5jaDIwMTdAMTI2LmNvbQ==
†These authors have contributed equally to this work
Disclaimer: All claims expressed in this article are solely those of the authors and do not necessarily represent those of their affiliated organizations, or those of the publisher, the editors and the reviewers. Any product that may be evaluated in this article or claim that may be made by its manufacturer is not guaranteed or endorsed by the publisher.
Research integrity at Frontiers
Learn more about the work of our research integrity team to safeguard the quality of each article we publish.