- 1Department of Internal Medicine 3—Rheumatology and Immunology, Friedrich-Alexander-University Erlangen-Nürnberg (FAU) and Universitätsklinikum Erlangen, Erlangen, Germany
- 2Deutsches Zentrum für Immuntherapie (DZI), Friedrich-Alexander-University Erlangen-Nürnberg (FAU) and Universitätsklinikum Erlangen, Erlangen, Germany
- 3Division of Biochemistry, Friedrich-Alexander-Universität Erlangen-Nürnberg, Erlangen, Germany
- 4Department of Rheumatology, Renji Hospital Affiliated to Shanghai Jiao Tong University School of Medicine, Shanghai, China
Background: Human malignant melanoma is a highly aggressive, heterogeneous and drug-resistant cancer. Due to a high number of clones, harboring various mutations that affect key pathways, there is an exceptional level of phenotypic variation and intratumor heterogeneity (ITH) in melanoma. This poses a significant challenge to personalized cancer medicine. Hitherto, it remains unclear to what extent the heterogeneity of melanoma affects the immune microenvironment. Herein, we explore the interaction between the tumor heterogeneity and the host immune response in a melanoma cohort utilizing The Cancer Genome Atlas (TCGA).
Methods: Clonal Heterogeneity Analysis Tool (CHAT) was used to estimate intratumor heterogeneity, and immune cell composition was estimated using CIBERSORT. The Overall Survival (OS) among groups was analyzed using Kaplan–Meier curves with the log-rank test and multivariate cox regression. RNA-seq data were evaluated to identify differentially expressed immunomodulatory genes. The reverse phase protein array (RPPA) data platform was used to validate immune responses at protein level.
Results: Tumors with high heterogeneity were associated with decreased overall survival (p = 0.027). High CHAT tumors were correlated with less infiltration by anti-tumor CD8 T cells (p = 0.0049), T follicular cells (p = 0.00091), and M1 macrophages (p = 0.0028), whereas tumor-promoting M2 macrophages were increased (p = 0.02). High CHAT tumors correlated with a reduced expression of immunomodulatory genes, particularly Programmed Cell Death 1 (PD1) and its ligand PD-L1. In addition, high CHAT tumors exhibited lower immune Cytotoxic T lymphocytes (CTLs)-mediated toxicity pathway score (p = 2.9E−07) and cytotoxic pathway score (p = 2.9E−08). High CHAT tumors were also associated with a lower protein level of immune-regulatory kinases, such as lymphocyte-specific protein tyrosine kinase (LCK) (p = 3.4e−5) and spleen tyrosine kinase (SYK) (p = 0.0011).
Conclusions: Highly heterogeneous melanoma tumors are associated with reduced immune cell infiltration and immune response activation as well as decreased survival. Our results reveal that intratumor heterogeneity is an indicative factor for patient survival due to its impact on anti-tumor immune response.
Introduction
Melanoma is a highly heterogeneous disease with genetic and phenotypic diversity (1). The coexistence of cells with different phenotypic and molecular features within one tumor is named intratumor heterogeneity (ITH) (2). It is well known that a high number of clones, each harboring a set of various mutations, results in an exceptional level of intratumor heterogeneity in melanoma (2). Pro-tumorigenic properties, such as a tumor-promoting inflammatory milieu, and the resistance to immune destruction, at least in part, may be linked to intratumor heterogeneity (3). In melanoma, heterogeneity at the genomic and immunological level influences cancer progression and results in differential responses to therapy (4). The evaluation of intratumor heterogeneity of individual tumors as well as its impact on disease progression and therapeutic efficacy is therefore essential to overcome treatment challenges in melanoma.
It is believed that tumor initiation and progression result from dominant mutations within the original healthy cells, followed with a selection of malignant subclones (3). The Clonal Heterogeneity Analysis Tool (CHAT) is a collection of tools developed for tumor clonality analysis using high density DNA SNP array data and sequencing data (5). Using CHAT, it is possible to estimate cellular fractions for both somatic copy number alterations (sCNAs) and mutations, and to use their distributions to define the underlying clonal structure.
Tumor immune surveillance is crucial to inhibit carcinogenesis, tumor progression, and to maintain cellular homeostasis. A failure of this surveillance system is associated with poor survival (6). Nowadays, classical cancer therapies, such as chemotherapy, surgery or radiation are combined with the administration of immunomodulators, functional molecules that amplify the patient’s immune response to cancer (7). Notably, tumor infiltrating lymphocytes (TILs) are an important characteristic to define the therapeutic action and could be used to estimate the success rate of novel melanoma immunotherapeutic protocols (8). Moreover, molecular and genetic signatures of the immune cytolytic activity are related to immune-mediated cancer elimination, which critically impacts the survival of cancer patients (9). To pave the way for personalized cancer therapy, immunogenomic analysis of tumors can be used to predict the tumor immune microenvironment, clinical associations, as well as prognostic biomarkers, indicating the efficacy of cancer immunotherapy (10, 11).
Up to now, the relationship between intratumor heterogeneity and the immune microenvironment across a large cohort of melanoma patients has not been explored in depth. Thus, in the present study we aim to investigate the association of tumor heterogeneity with the composition of tumor-infiltrating immune cells, as well as immunomodulators, anti-tumor immune activity, and survival in melanoma.
Materials and Methods
Patient Cohort
By virtue of genomic data commons data portal provided officially by the Cancer Genome Atlas (TCGA) database (12) (https://cancergenome.nih.gov/), a total of 402 untreated patients with DNA single nucleotide variants (SNVs) and Copy number variation (CNV) data were included in the skin cutaneous melanoma cohort (SKCM) of TCGA Provisional cohort, of whom clinical information were available. The gene expression quantification data for TCGA cohort was downloaded through UCSC xena (13). The reverse phase protein array (RPPA) data was downloaded from The Cancer Proteome Atlas (TCPA), a user-friendly data portal developed to facilitate the access of cancer proteomics datasets to the broader research community (14).
Clonal Heterogeneity Analysis Tool (CHAT)
Tumors arise from a single mutated cell that accumulates additional mutations as it progresses. These changes give rise to tumor subpopulations, which have the ability to divide and mutate further (15). This heterogeneity may give rise to clones that have an evolutionary advantage over the others within the tumor environment, and these clones may become dominant in the tumor over time (15). CHAT estimates cellular fractions for both sCNAs and mutations, and uses their distributions to define the macroscopic, clonal architecture of the tumor and the overall intra-tumor heterogeneity, based on the estimated number of clones (5, 16). To study the correlation between heterogeneity and overall patient survival, we divided the TCGA patients into the following groups: Low ITH (clones 1, 2 and 3), High ITH (clones 4, 5 and 6).
Survival Analysis
A cohort of TCGA melanoma patients with available clinical data was subdivided based on the number of clones as calculated by the CHAT algorithm (1). Next, we calculated Kaplan–Meier survival curves for each group and tested the statistical relevance of the obtained differences between the groups by a Log-rank test. The overall survival (OS) analysis was performed by using the survival package available for R. Due to the fact that other clinical factors, such as gender and age can influence the survival outcome, it was necessary to verify, whether the differences identified in this study occur independently of these parameters. Therefore, a multivariate cox regression analysis was performed in which gender, patient age (at the time of diagnosis), and clinical stage were incorporated as additional factors.
CIBERSORT Estimation
CIBERSORT, a bioinformatics algorithm to calculate immune cell compositions based on gene expression profiles, was used to estimate the type of tumor-infiltrating immune cells (17). Immune cell fraction data were downloaded from TCIA database (https://tcia.at/home) (18). Proportions of 22 immune cells were compared between CHAT high and low tumors using the same cut-off for survival analysis in the TCGA cohort.
Single-Sample Gene Set Enrichment Analysis (ssGSEA)
Single sample GSEA (ssGSEA) calculates a gene set enrichment score for each sample as the normalized difference in empirical cumulative distribution functions of gene expression ranks inside and outside the gene set (19). The Molecular Signatures Database (MSigDB) BIOCARTA collection was scored using ssGSEA as implemented in the GSVA R package (20).
Statistical Analysis
Log-rank test and Cox proportional hazard analysis were used to compare the survival distribution between low and high groups calculated according to CHAT. Moreover, receiver operating characteristic (ROC) analysis was performed to evaluate predictive prognostic effect. Pearson correlations were calculated based on expression levels of immune cell fraction, and was plotted afterwards. Immune cell fraction comparison was analyzed using the Wilcoxon signed-rank test and gene expression comparison was analyzed using Student’s t test. In all analyses, A probability value of p <0.05 was considered statistically significant (*p <0.05; **p <0.01; ***p <0.001; ****p <0.0001). All statistical analyses and plots were generated using R version 3.6.1 (http://www.r-project.org/) and Bioconductor version 3.12 (http://bioconductor.org/).
Results
High Heterogeneity is Associated With Decreased Patient Survival in Melanoma
Patients were grouped according to the level of ITH that was defined by the number of clones. The clone number was computed based on each sample’s somatic copy number alterations and somatic mutations (Figure 1A). Patients were divided into low and high groups based on the average number of clones (CHAT high, n = 176; CHAT low, n = 226). High CHAT tumors exhibited a higher tumor purity estimation and Breslow depth, but no correlation with mutation and copy number variation (CNV) burden (Figure 1D). Patients with low ITH presented a significantly increased overall survival (p = 0.027; Figure 1B), consistent with previous observations, where patients with highly heterogeneous cancers had a reduced survival rate in pan-cancer analysis (21, 22). Receiver Operating Characteristic (ROC) analysis was performed to describe the sensitivity and specificity of survival prediction. The area under the curve (AUC) was 0.61 (Figure 1C). These observations remained unchanged after the integration of potential confounding factors, such as age, gender and tumor stage (Figure 1E and Supplementary Figure 1). Taking together, these results demonstrate that ITH of melanoma influences the patient`s survival rate.
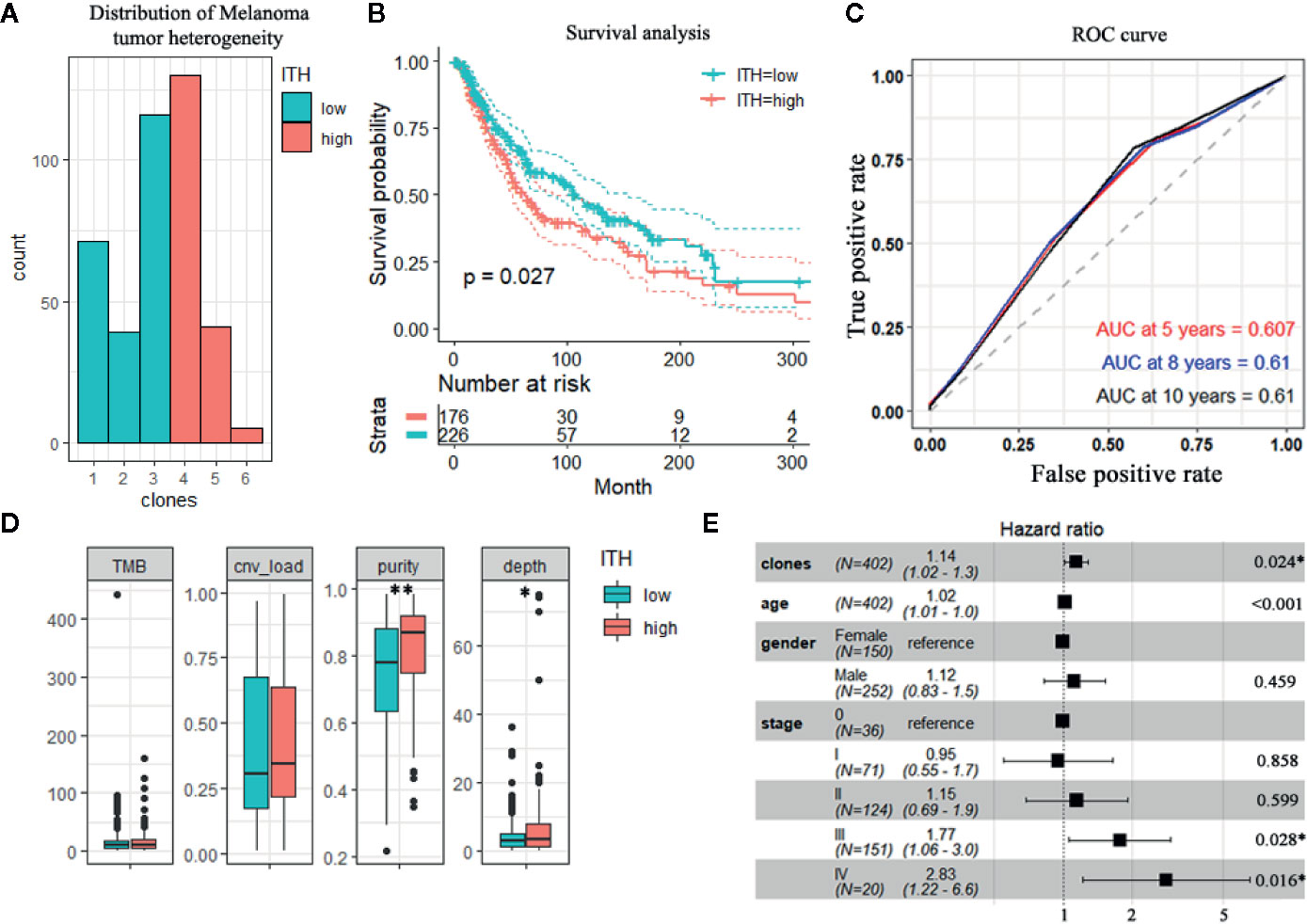
Figure 1 High heterogeneity is associated with decreased patient survival in TCGA melanoma cohort. (A) Distribution of the overall intratumor heterogeneity estimated by CHAT algorithm (n = 71, 39, 136, 130,41,9). (B) Kaplan–Meier analysis for overall survival rate of patients. Log-rank test was performed to evaluate the survival differences. (C) The prediction performance of CHAT clones for 5-, 8- and 10-year overall survival by the ROC analysis. (D) Boxplots correlating CHAT with purity and Breslow thickness depth. (E) Multivariable Cox regression analysis of the CHAT clones.
Melanoma With High Heterogeneity Are Associated With Reduced Immune Cell Infiltration
Previously, it has been shown that patients with more tumor infiltrating lymphocytes (TILs) present improved recurrence-free survival and overall survival when compared to patients with non-brisk and absent TILs (23). Therefore, we characterized the intratumoral immune landscapes within the TCGA-Melanoma cohort, and analyzed their associations with tumor heterogeneity. Each immune cell fraction calculated by CIBERSORT algorithm was compared within tumors with different CHAT clones. High CHAT tumors were associated with lower fractions of activated CD8+ T cells (p = 0.0049), follicular helper T cell (Tfh; p = 0.00091), and pro-inflammatory M1-like macrophages (p = 0.0028) as well as with higher fractions of alternatively activated M2-likemacrophages (p = 0.02; Figures 2A, B). In accordance with involvement of immune cells as playing in the multifactorial manifestation of tumor heterogeneity (24), we found that tumors with high heterogeneity not only possess less anti-tumor immune cells but also more immune suppressing cells.
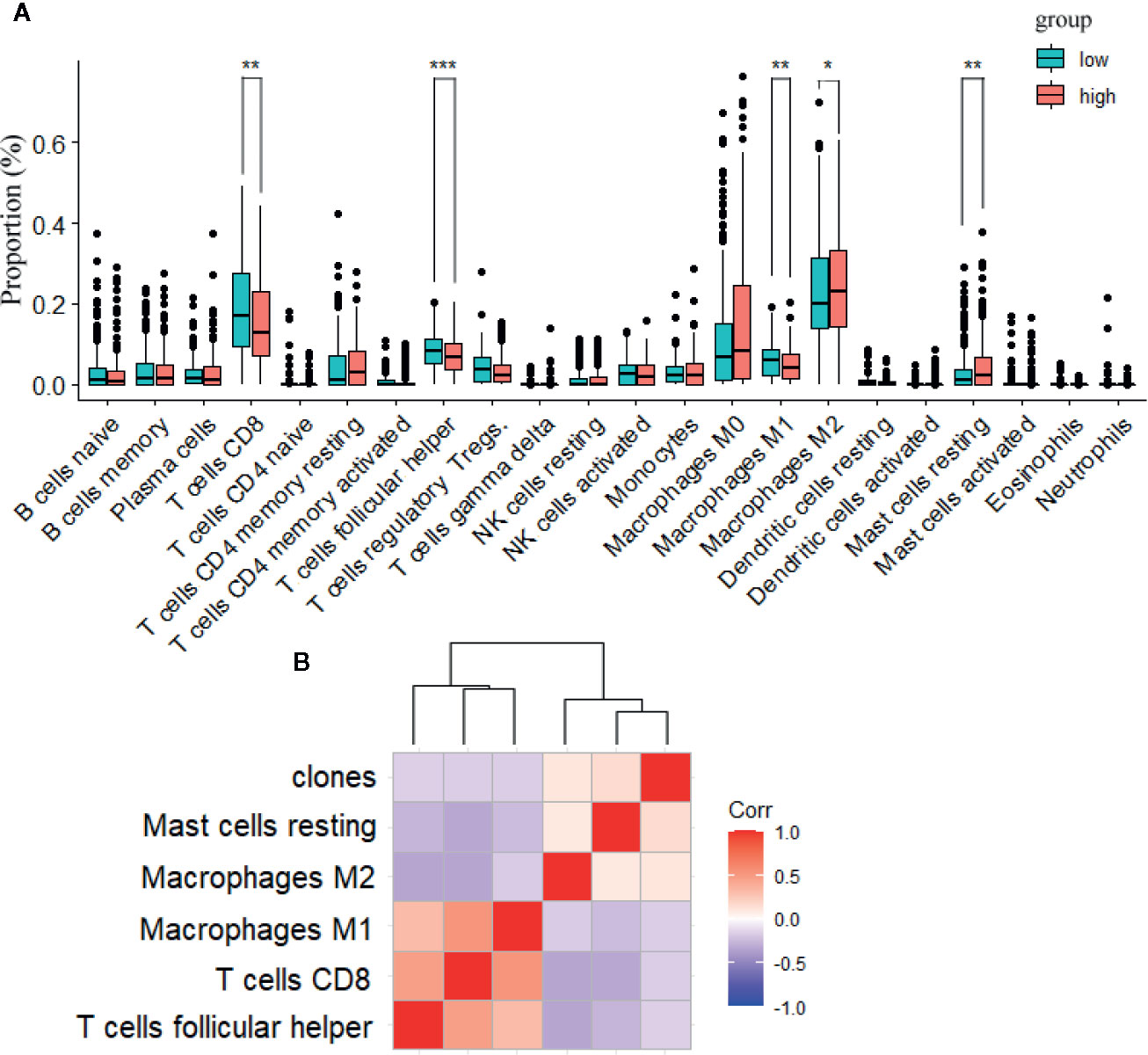
Figure 2 High heterogeneity is associated with reduced anti-tumor immune cell infiltration. (A) Cell composition fractions comparison containing 22 immune cell types between ITH high and low groups in the TCGA cohort by Wilcoxon’s test. (B) Correlation analysis among M1, M2, Tfh cell, resting Mast cell, CD8+T cell and clones by Spearman test. Clones is positively correlated with resting Mast cells, M2 and negatively correlated with M1, CD8+T cells, Tfh cell. *p < 0.05; **p < 0.01.
Tumor Heterogeneity Is Associated With Immunomodulator Gene Expression
Patients with distant metastatic diseases have a poor overall survival rate and respond less to conventional chemotherapy (25). Therefore, the use of immunomodulators is critical for cancer immunotherapy especially in non-responders. Nowadays, numerous immunomodulator agonists and antagonists are under investigation in clinical oncology (24). Still further progress is needed to fully evaluate their potential, understand their expression pattern, and mode of action in different stages of clonal diversity. Our examinations have identified a variation in the gene expression of immunomodulators across the 6 clonal subclasses, which might explain the role of intratumor heterogeneity in shaping the tumor microenvironment (Figure 3A). What’s more, the normalized enrichment score (NES) in GSEA analysis of immunomodulator gene set is −2.769(p = 0.001, Figure 3B). Blocking the interaction between the programmed cell death (PD)-1 protein and one of its ligands, PD-L1, has been reported to have impressive antitumor responses (26). We found that high CHAT tumor was associated with significantly lower mRNA expression of PD1 (p = 5.6e−06, Spearman’s rho = −0.266) and PD-L1 (p = 6.2e−08, Spearman’s rho = −0.224, Figure 3C).
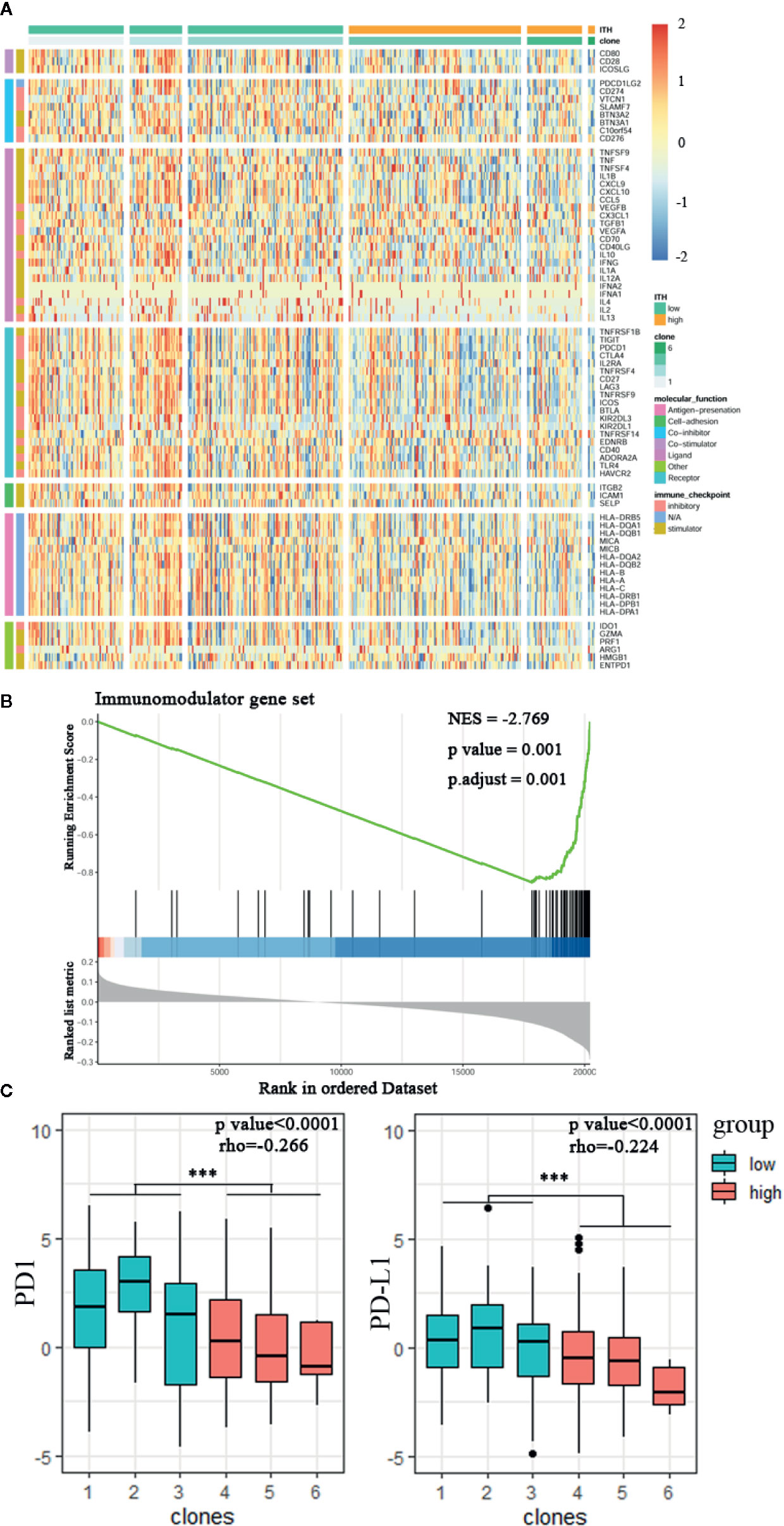
Figure 3 Tumor heterogeneity is associated with the regulation of Immunomodulator. (A) Heatmap of immunomodulator genes among the CHAT 6 clones. Data normalized by Z score transformation is used. (B) The gene set enrichment analysis (GSEA) of immunomodulator genes (NES = −2.769; p = 0.001). (C) PD1 and PD-L1 expression sectioned by clonal subclasses (Student's t test,***p < 0.001. spearman’s rho = −0.266; p <0.0001 and spearman’s rho = −0.224; p <0.0001).
Tumor Heterogeneity Is Associated With Immune Cytolytic Activity
The relation between the activation of the immune response and tumor heterogeneity was further investigated by scoring the enrichment of cytolytic immune pathways. Indeed, CTL score and T cytotoxic score were significantly lower in high CHAT tumors consistent with reduced cytolytic immune activity (p = 6.8E−07 and p = 1.2E−06; Figure 4A). Specifically, these two pathway scores were inversely correlated with the clonal diversity throughout the TCGA cohort (Spearman’s rho = −0.253, p = 2.9e−07; Spearman’s rho = −0.273, p = 2.9e−08; Figures 4B, C). This finding further supports the notion that reduced anti-tumor immune cell infiltration and diminished cytolytic activity within the tumor is inducing clonal evolution and the development of intratumor heterogeneity (27).
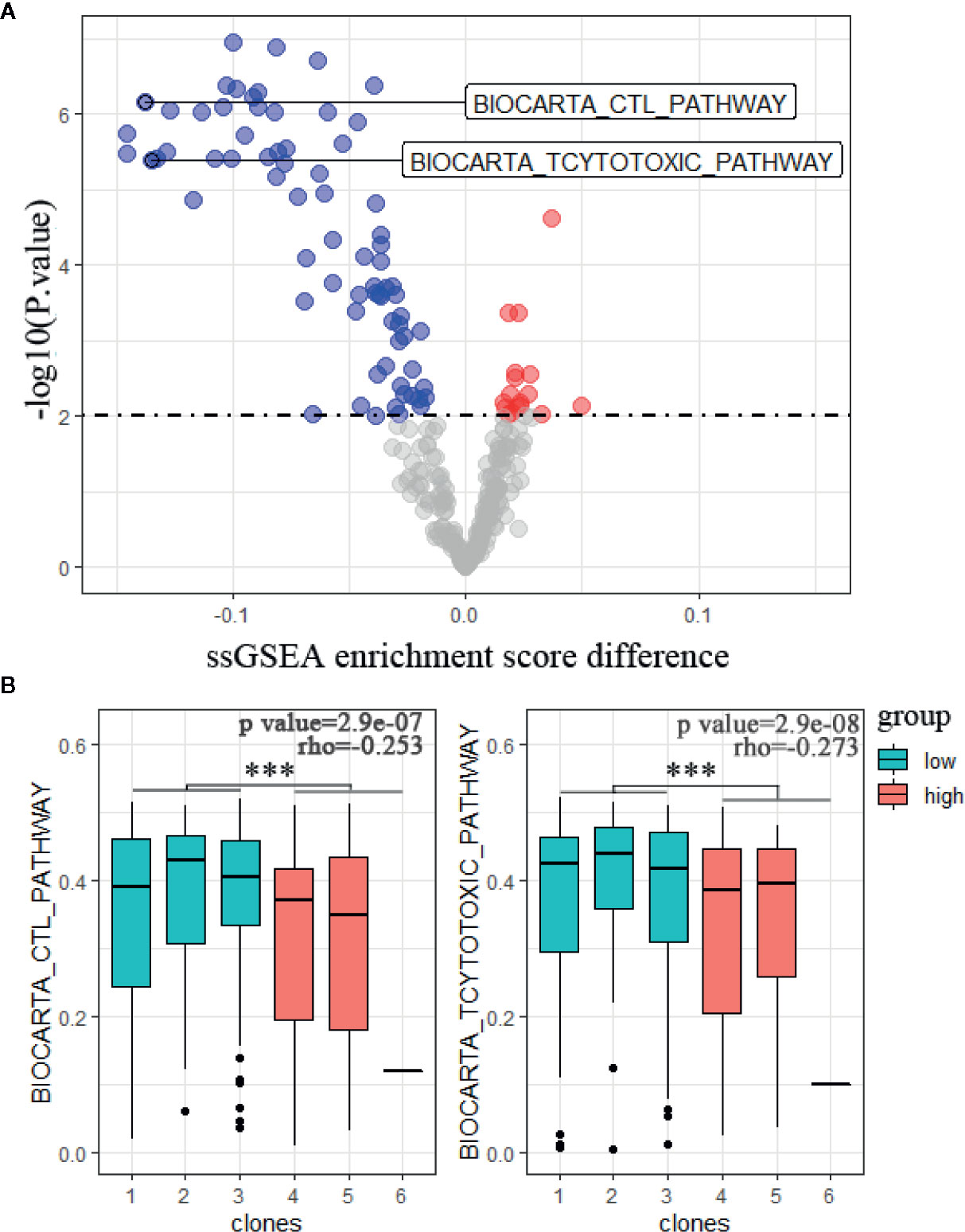
Figure 4 Tumor heterogeneity is associated with less immune cytolytic activity. (A) Volcano plot of ssGSEA enrichment score difference (x-axis) versus –log10 p value (y-axis) of BIOCARTA pathway changes in the TCGA cohort. (B) TCYTOTOXIC Pathway and CTL Pathway scores sectioned by clonal subclasses (Student's t test,***p < 0.001. spearman’s rho = −0.253; p <0.0001 and spearman’s rho = −0.273; p <0.0001).
Tumor Heterogeneity Is Associated With Immune-Related Proteins
Next, we hypothesized that the immune-related transcriptomic features, defined by the heterogeneity subclasses, are also correlated at protein levels by reverse phase protein lysate microarray (RPPA). Indeed, high ITH tumors were associated with significantly lower expression of lymphocyte-specific protein tyrosine kinase (LCK) (p = 2.3e−05, Figure 5A) and spleen tyrosine kinase (SYK) (p = 0.0023, Figure 5B) in melanoma cohort. Both proteins are non-receptor tyrosine kinases, commonly associated with anti-tumor lymphocyte signaling (28, 29). Notably, the LCK and SYK levels were inversely correlated with the degree of clone numbers throughout the TCGA cohort (Spearman’s rho = −0.235, p = 3.4e−05; Spearman’s rho = −0.187, p = 1.1e−03; Figures 5A, B).
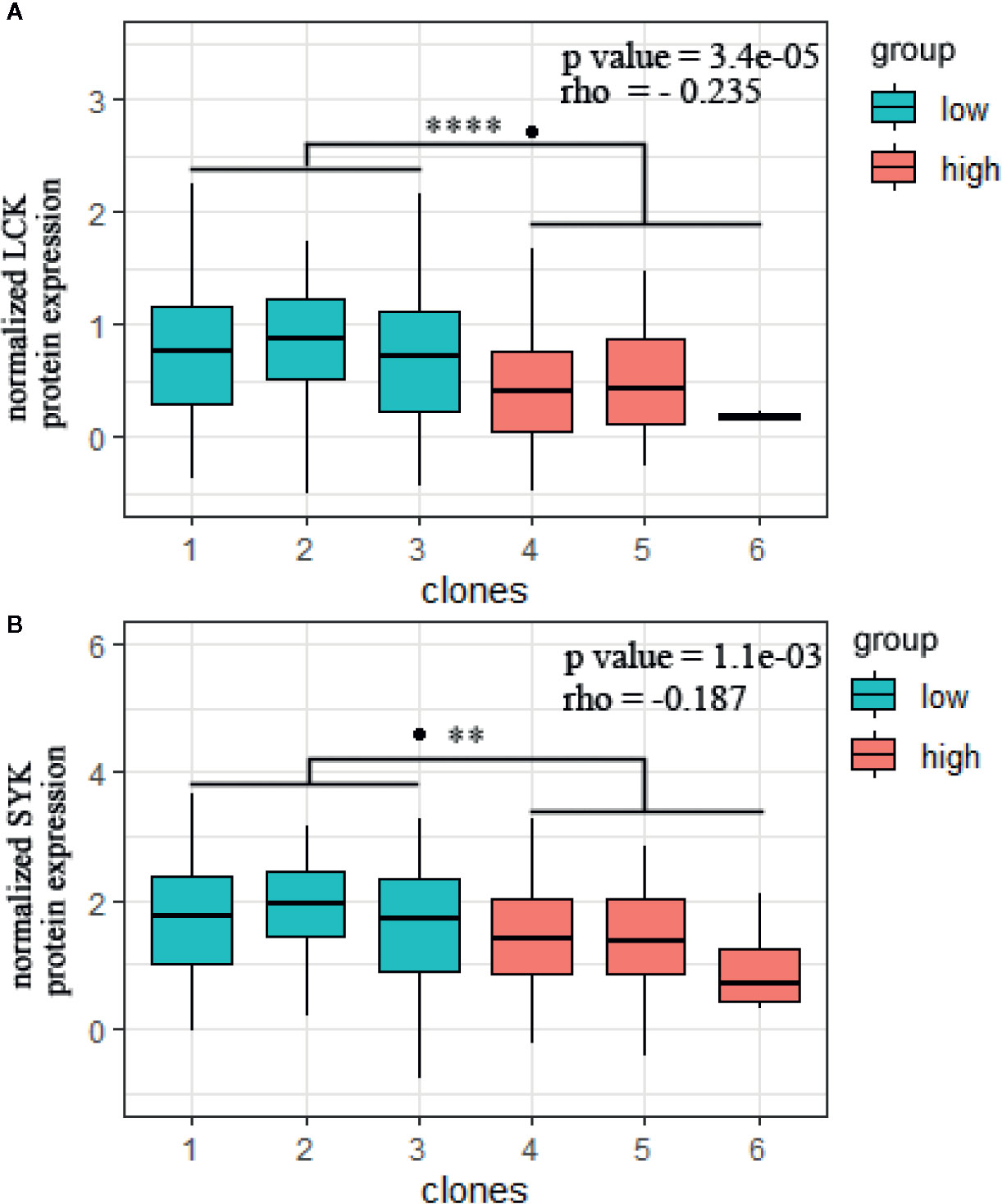
Figure 5 Tumor heterogeneity is associated with immune-related proteins. (A) LCK and (B) SYK normalized protein expression in RPPA array are subdivided by clonal subclasses (Student's t test, **p < 0.01; ****p < 0.0001. spearman’s rho = −0.235; p <0.0001 and spearman’s rho = −0.187; p = 0.0011).
Discussion
Intratumor heterogeneity accounts for differences in tumor phenotype and clinical outcome among individual patients and has wide implications for predictive or prognostic biomarker strategies (15). In addition, tumor infiltrating lymphocytes have been identified as a marker of favorable prognosis across variety of cancers, including breast, ovarian, and colon cancer (30–32). TILs could be served as a surrogate indicator for the strength of the host anti-tumor immune response. Although multiple studies have assessed the prognostic value of tumor-infiltrating lymphocytes and immune responses in primary melanoma (33–35), the relationship between intratumor heterogeneity and immune microenvironment is still unclear. Here, we could show that patients with high tumor heterogeneity possess reduced anti-tumor immune cell infiltration, immunomodulator gene expression, and low cytolytic activity, associated with a worse survival outcome.
CHAT algorithm was used to subdivide patients into low and high score groups based on a quantitative assessment of their intratumor heterogeneity. The survival analysis in this study is in line with a previous report about breast cancer, where patients with high heterogeneity showed a tendency toward worse survival rate (36, 37). High CHAT tumors demonstrated a higher tumor purity estimation and a higher Breslow thickness depth, but no correlation with mutation burden and CNV burden, which might suggest that tumors with high heterogeneity have a higher proliferation capacity. The high heterogeneity tumors were correlated with a lower proportion of anti-tumor CD8 T cells, Tfh cells, and M1-like macrophages, whereas tumor-promoting M2-like macrophages were increased. This observation is in agreement with previous reports, showing that CD8 T cells, Tfh cells and pro-inflammatory macrophages are associated with favorable prognosis in invasive melanoma and are potential targets of immune therapies (38–41).The association between the amount of infiltrating immune cells and the degree of tumor heterogeneity could be an explanation for the observed survival differences between the compared ITH groups.
The list of immunomodulator genes was collected from a literature review performed by an interdisciplinary expert group for immune-oncology from TCGA program and the immunomodulatory function is confirmed after reviewing each entry (11). Here, we report for the first time that tumors with high heterogeneity present less gene expression of immunomodulators, which might be important for the anti-tumor immune response. The recent unprecedented success of immune checkpoint blockade that target PD-1/PD-L1 axis highlights the universal power of treating the immune system across tumor types, which offers the paradigm for scientific translation from bench to bedside (42). Nevertheless, many patients are still refractory to anti-PD-1/PD-L1 therapy. Heterogeneity is one important potential factor affecting the effectiveness of PD-1/PD-L1 blockade in melanoma. These immunomodulator genes largely contribute to the clonal variation of tumors, revealing their importance in shaping the tumor microenvironment.
Tumors with high T cell-related immune activity are associated with fewer distant metastasis, and are predictive of a higher likelihood of a complete pathologic response with chemotherapy (43). Cytotoxic T cells are key players in the cellular immune response against tumors, killing degenerated cells that display foreign antigens on their surface. The CTL pathway and the cytotoxic pathway are the main paths considered in this process, as outlined in the Molecular Signatures Database (44). Our findings suggest that tumors with high intratumor heterogeneity correlate with less immune cell infiltration, which was further supported by the demonstration of less cytolytic activity in these tumors, as shown by CTL pathway and cytotoxic T cell pathway analysis.
To confirm the correlation between tumor heterogeneity and immune activity on protein levels, the RPPA data was grouped according to the clonal subclasses. We could identify a reduced expression of the kinases LCK and SYK. LCK is one of the key molecules regulating T-cell responses in cancer immunotherapy (29). SYK, another protein tyrosine kinase, has been proven to suppress malignant growth of human breast cancer cells via activation of the anti-tumor immune system (28).Their high expression in tumors with low heterogeneity likely suggests a strong immune response induced by the aggregation of immune cells within the tumor tissue.
Our study provides a novel insight into the correlation of heterogeneity with prognosis, and allows for a better understanding for improving the survival of patients with melanoma. However, the limitations should be acknowledged in our study. We have no experimental data and lack clues on the link between tumor heterogeneity and the efficiency of immunotherapy such as PD-1 blockade, adoptive T cell therapy. Experimental studies are greatly needed to provide important information to strength the mechanism behind it in melanoma.
Conclusion
Tumors with high intratumor heterogeneity demonstrate reduced infiltration with anti-tumor immune cells, lower expression of genes related to immunomodulators, lower cytolytic activity, and worse survival in melanoma. There is an urgent need to investigate the cellular, molecular and genetic changes that cause intratumor heterogeneity and it will help us to accelerate the development of prevention and treatment options, thereby improving the prognosis for melanoma patients.
Data Availability Statement
The datasets presented in this study can be found in online repositories. The names of the repository/repositories and accession number(s) can be found below: The Cancer Genome Atlas, https://portal.gdc.cancer.gov/projects/TCGA-SKCM.
Author Contributions
LZ, XM, and JW designed the study, performed the analyses. LZ, KK, SC, CX, and AB wrote the manuscript. JC provided expertise and input. XC, GS, and AB acquired funding, designed the study, and wrote the manuscript. All authors contributed to the article and approved the submitted version.
Conflict of Interest
The authors declare that the research was conducted in the absence of any commercial or financial relationships that could be construed as a potential conflict of interest.
Acknowledgements
The present work was performed in (partial) fulfillment of the requirements for obtaining the degree “Dr. med.”.
Supplementary Material
The Supplementary Material for this article can be found online at: https://www.frontiersin.org/articles/10.3389/fonc.2020.596493/full#supplementary-material
References
1. Genomic Classification of Cutaneous Melanoma. Cell (2015) 161(7):1681–96. doi: 10.1016/j.cell.2015.05.044
2. Grzywa TM, Paskal W, Wlodarski PK. Intratumor and Intertumor Heterogeneity in Melanoma. Transl Oncol (2017) 10(6):956–75. doi: 10.1016/j.tranon.2017.09.007
3. McGranahan N, Swanton C. Clonal Heterogeneity and Tumor Evolution: Past, Present, and the Future. Cell (2017) 168(4):613–28. doi: 10.1016/j.cell.2017.01.018
4. Reuben A, Spencer CN, Prieto PA, Gopalakrishnan V, Reddy SM, Miller JP, et al. Genomic and immune heterogeneity are associated with differential responses to therapy in melanoma. NPJ Genom Med (2017) 2:10. doi: 10.1038/s41525-017-0013-8
5. Li B, Li JZ. A general framework for analyzing tumor subclonality using SNP array and DNA sequencing data. Genome Biol (2014) 15(9):473. doi: 10.1186/s13059-014-0473-4
6. Kim R, Emi M, Tanabe K. Cancer immunoediting from immune surveillance to immune escape. Immunology (2007) 121(1):1–14. doi: 10.1111/j.1365-2567.2007.02587.x
7. Lavelle EC, McLachlan JB. Editorial overview: Immunomodulation: Striking the right balance: using immunomodulators to target infectious diseases, cancer, and autoimmunity. Curr Opin Pharmacol (2018) 41:vii–ix. doi: 10.1016/j.coph.2018.07.013
8. Schatton T, Scolyer RA, Thompson JF, Mihm MJ. Tumor-infiltrating lymphocytes and their significance in melanoma prognosis. Methods Mol Biol (2014) 1102:287–324. doi: 10.1007/978-1-62703-727-3_16
9. Rooney MS, Shukla SA, Wu CJ, Getz G, Hacohen N. Molecular and genetic properties of tumors associated with local immune cytolytic activity. Cell (2015) 160(1-2):48–61. doi: 10.1016/j.cell.2014.12.033
10. Li B, Severson E, Pignon JC, Zhao H, Li T, Novak J, et al. Comprehensive analyses of tumor immunity: implications for cancer immunotherapy. Genome Biol (2016) 17(1):174. doi: 10.1186/s13059-016-1028-7
11. Thorsson V, Gibbs DL, Brown SD, Wolf D, Bortone DS, Ou YT, et al. The Immune Landscape of Cancer. Immunity (2018) 48(4):812–30. doi: 10.1016/j.immuni.2018.03.023
12. Weinstein JN, Collisson EA, Mills GB, Shaw KR, Ozenberger BA, Ellrott K, et al. The Cancer Genome Atlas Pan-Cancer analysis project. Nat Genet (2013) 45(10):1113–20. doi: 10.1038/ng.2764
13. Goldman M, Craft B, Swatloski T, Cline M, Morozova O, Diekhans M, et al. The UCSC Cancer Genomics Browser: update 2015. Nucleic Acids Res (2015) 43(Database issue):D812–7. doi: 10.1093/nar/gku1073
14. Li J, Lu Y, Akbani R, Ju Z, Roebuck PL, Liu W, et al. TCPA: a resource for cancer functional proteomics data. Nat Methods (2013) 10(11):1046–7. doi: 10.1038/nmeth.2650
15. Swanton C. Intratumor heterogeneity: evolution through space and time. Cancer Res (2012) 72(19):4875–82. doi: 10.1158/0008-5472.CAN-12-2217
16. Wolf Y, Bartok O, Patkar S, Eli GB, Cohen S, Litchfield K, et al. UVB-Induced Tumor Heterogeneity Diminishes Immune Response in Melanoma. Cell (2019) 179(1):219–35. doi: 10.1016/j.cell.2019.08.032
17. Newman AM, Liu CL, Green MR, Gentles AJ, Feng W, Xu Y, et al. Robust enumeration of cell subsets from tissue expression profiles. Nat Methods (2015) 12(5):453–7. doi: 10.1038/nmeth.3337
18. Charoentong P, Finotello F, Angelova M, Mayer C, Efremova M, Rieder D, et al. Pan-cancer Immunogenomic Analyses Reveal Genotype-Immunophenotype Relationships and Predictors of Response to Checkpoint Blockade. Cell Rep (2017) 18(1):248–62. doi: 10.1016/j.celrep.2016.12.019
19. Barbie DA, Tamayo P, Boehm JS, Kim SY, Moody SE, Dunn IF, et al. Systematic RNA interference reveals that oncogenic KRAS-driven cancers require TBK1. Nature (2009) 462(7269):108–12. doi: 10.1038/nature08460
20. Hanzelmann S, Castelo R, Guinney J. GSVA: gene set variation analysis for microarray and RNA-seq data. BMC Bioinf (2013) 14:7. doi: 10.1186/1471-2105-14-7
21. Morris LG, Riaz N, Desrichard A, Senbabaoglu Y, Hakimi AA, Makarov V, et al. Pan-cancer analysis of intratumor heterogeneity as a prognostic determinant of survival. Oncotarget (2016) 7(9):10051–63. doi: 10.18632/oncotarget.7067
22. Brown SD, Warren RL, Gibb EA, Martin SD, Spinelli JJ, Nelson BH, et al. Neo-antigens predicted by tumor genome meta-analysis correlate with increased patient survival. Genome Res (2014) 24(5):743–50. doi: 10.1101/gr.165985.113
23. Weiss SA, Han SW, Lui K, Tchack J, Shapiro R, Berman R, et al. Immunologic heterogeneity of tumor-infiltrating lymphocyte composition in primary melanoma. Hum Pathol (2016) 57:116–25. doi: 10.1016/j.humpath.2016.07.008
24. Tang J, Shalabi A, Hubbard-Lucey VM. Comprehensive analysis of the clinical immuno-oncology landscape. Ann Oncol (2018) 29(1):84–91. doi: 10.1093/annonc/mdx755
25. Balch CM, Gershenwald JE, Soong SJ, Thompson JF, Atkins MB, Byrd DR, et al. Final version of 2009 AJCC melanoma staging and classification. J Clin Oncol (2009) 27(36):6199–206. doi: 10.1200/JCO.2009.23.4799
26. Munhoz RR, Postow MA. Clinical Development of PD-1 in Advanced Melanoma. Cancer J (2018) 24(1):7–14. doi: 10.1097/PPO.0000000000000299
27. Milo I, Bedora-Faure M, Garcia Z, Thibaut R, Perie L, Shakhar G, et al. The immune system profoundly restricts intratumor genetic heterogeneity. Sci Immunol (2018) 3(29):eaat1435. doi: 10.1126/sciimmunol.aat1435
28. Coopman PJ, Do MT, Barth M, Bowden ET, Hayes AJ, Basyuk E, et al. The Syk tyrosine kinase suppresses malignant growth of human breast cancer cells. Nature (2000) 406(6797):742–7. doi: 10.1038/35021086
29. Bommhardt U, Schraven B, Simeoni L. Beyond TCR Signaling: Emerging Functions of Lck in Cancer and Immunotherapy. Int J Mol Sci (2019) 20(14):3500. doi: 10.3390/ijms20143500
30. Ropponen KM, Eskelinen MJ, Lipponen PK, Alhava E, Kosma VM. Prognostic value of tumour-infiltrating lymphocytes (TILs) in colorectal cancer. J Pathol (1997) 182(3):318–24. doi: 10.1002/(SICI)1096-9896(199707)182:3<318::AID-PATH862<3.0.CO;2-6
31. Hwang WT, Adams SF, Tahirovic E, Hagemann IS, Coukos G. Prognostic significance of tumor-infiltrating T cells in ovarian cancer: a meta-analysis. Gynecol Oncol (2012) 124(2):192–8. doi: 10.1016/j.ygyno.2011.09.039
32. Adams S, Gray RJ, Demaria S, Goldstein L, Perez EA, Shulman LN, et al. Prognostic value of tumor-infiltrating lymphocytes in triple-negative breast cancers from two phase III randomized adjuvant breast cancer trials: ECOG 2197 and ECOG 1199. J Clin Oncol (2014) 32(27):2959–66. doi: 10.1200/JCO.2013.55.0491
33. Azimi F, Scolyer RA, Rumcheva P, Moncrieff M, Murali R, McCarthy SW, et al. Tumor-infiltrating lymphocyte grade is an independent predictor of sentinel lymph node status and survival in patients with cutaneous melanoma. J Clin Oncol (2012) 30(21):2678–83. doi: 10.1200/JCO.2011.37.8539
34. Clark WJ, Elder DE, Guerry DT, Braitman LE, Trock BJ, Schultz D, et al. Model predicting survival in stage I melanoma based on tumor progression. J Natl Cancer Inst (1989) 81(24):1893–904. doi: 10.1093/jnci/81.24.1893
35. Thomas NE, Busam KJ, From L, Kricker A, Armstrong BK, Anton-Culver H, et al. Tumor-infiltrating lymphocyte grade in primary melanomas is independently associated with melanoma-specific survival in the population-based genes, environment and melanoma study. J Clin Oncol (2013) 31(33):4252–9. doi: 10.1200/JCO.2013.51.3002
36. Ahmed F, Haass NK. Microenvironment-Driven Dynamic Heterogeneity and Phenotypic Plasticity as a Mechanism of Melanoma Therapy Resistance. Front Oncol (2018) 8:173:173. doi: 10.3389/fonc.2018.00173
37. McDonald KA, Kawaguchi T, Qi Q, Peng X, Asaoka M, Young J, et al. Tumor Heterogeneity Correlates with Less Immune Response and Worse Survival in Breast Cancer Patients. Ann Surg Oncol (2019) 26(7):2191–9. doi: 10.1245/s10434-019-07338-3
38. Li H, van der Leun AM, Yofe I, Lubling Y, Gelbard-Solodkin D, van Akkooi A, et al. Dysfunctional CD8 T Cells Form a Proliferative, Dynamically Regulated Compartment within Human Melanoma. Cell (2019) 176(4):775–89. doi: 10.1016/j.cell.2018.11.043
39. Qin L, Waseem TC, Sahoo A, Bieerkehazhi S, Zhou H, Galkina EV, et al. Insights Into the Molecular Mechanisms of T Follicular Helper-Mediated Immunity and Pathology. Front Immunol (2018) 9:1884:1884. doi: 10.3389/fimmu.2018.01884
40. Jensen TO, Schmidt H, Moller HJ, Hoyer M, Maniecki MB, Sjoegren P, et al. Macrophage markers in serum and tumor have prognostic impact in American Joint Committee on Cancer stage I/II melanoma. J Clin Oncol (2009) 27(20):3330–7. doi: 10.1200/JCO.2008.19.9919
41. Falleni M, Savi F, Tosi D, Agape E, Cerri A, Moneghini L, et al. M1 and M2 macrophages’ clinicopathological significance in cutaneous melanoma. Melanoma Res (2017) 27(3):200–10. doi: 10.1097/CMR.0000000000000352
42. Ai L, Xu A, Xu J. Roles of PD-1/PD-L1 Pathway: Signaling, Cancer, and Beyond. Adv Exp Med Biol (2020) 1248:33–59. doi: 10.1007/978-981-15-3266-5_3
43. Zhang J, Endres S, Kobold S. Enhancing tumor T cell infiltration to enable cancer immunotherapy. Immunotherapy (2019) 11(3):201–13. doi: 10.2217/imt-2018-0111
Keywords: immunomodulator, intratumor heterogeneity, The Cancer Genome Atlas, tumor infiltrating lymphocytes, melanoma
Citation: Lin Z, Meng X, Wen J, Corral JM, Andreev D, Kachler K, Schett G, Chen X and Bozec A (2020) Intratumor Heterogeneity Correlates With Reduced Immune Activity and Worse Survival in Melanoma Patients. Front. Oncol. 10:596493. doi: 10.3389/fonc.2020.596493
Received: 19 August 2020; Accepted: 02 November 2020;
Published: 04 December 2020.
Edited by:
Suzie Chen, Rutgers, The State University of New Jersey, United StatesReviewed by:
Chandra K. Singh, University of Wisconsin-Madison, United StatesKathleen Marie Kokolus, University at Buffalo, United States
Copyright © 2020 Lin, Meng, Wen, Corral, Andreev, Kachler, Schett, Chen and Bozec. This is an open-access article distributed under the terms of the Creative Commons Attribution License (CC BY). The use, distribution or reproduction in other forums is permitted, provided the original author(s) and the copyright owner(s) are credited and that the original publication in this journal is cited, in accordance with accepted academic practice. No use, distribution or reproduction is permitted which does not comply with these terms.
*Correspondence: Aline Bozec, YWxpbmUuYm96ZWNAdWstZXJsYW5nZW4uZGU=