- 1VA Palo Alto Health Care System, Palo Alto, CA, United States
- 2Biomedical Engineering, School of Medicine, Oregon Health & Science University, Portland, OR, United States
- 3Canary Center at Stanford, Department of Radiology, Stanford University School of Medicine, Stanford, CA, United States
- 4Department of Radiation Medicine, Oregon Health & Science University, Portland, OR, United States
- 5Division of Hematology and Medical Oncology, Oregon Health & Science University, Portland, OR, United States
- 6Cancer Early Detection & Advanced Research Center, Oregon Health & Science University, Portland, OR, United States
- 7Knight Cancer Institute, Oregon Health & Science University, Portland, OR, United States
- 8Department of Surgery, Stanford University School of Medicine, Stanford, CA, United States
- 9Department of Urology, Stanford University School of Medicine, Stanford, CA, United States
In this review, we discuss the interaction between cancer and markers of inflammation (such as levels of inflammatory cells and proteins) in the circulation, and the potential benefits of routinely monitoring these markers in peripheral blood measurement assays. Next, we discuss the prognostic value and limitations of using inflammatory markers such as neutrophil-to-lymphocyte and platelet-to-lymphocyte ratios and C-reactive protein measurements. Furthermore, the review discusses the benefits of combining multiple types of measurements and longitudinal tracking to improve staging and prognosis prediction of patients with cancer, and the ability of novel in silico frameworks to leverage this high-dimensional data.
Introduction
Cancer-associated inflammation is known to occur in the tumor microenvironment and in the systemic circulation and is correlated with disease progression and prognosis in many cancers (1). Immune mediators are involved in many phases of cancer progression including carcinogenesis, tumor growth, tumor invasion, and metastasis (2–5), and in turn, cancer cells can recruit and activate immune cells via direct contact (6) and/or through production of chemokines (7, 8) and prostaglandins (9). Numbers of circulating blood cells including neutrophils, lymphocytes, platelets, and levels of circulating proteins including C-reactive protein (CRP) and interleukins (ILs) associated with inflammatory responses, are key factors in recognition of pathways for tumorigenesis and growth (10) and could provide valuable information for improved cancer patient risk stratification and more targeted patient care.
Methods to improve early detection of cancer and gauge cancer patient prognosis are essential. Early detection can help identify cancers when they are still in a curative stage. Prognostic factors help to inform patients and physicians in end-of-life decision-making and can be utilized as inclusion/exclusion criteria for aggressive therapies or clinical trials. Overall, diagnostics and prognostics are critical to cancer therapy, and despite advances in technology and understanding of cancer cell biology, there are still many unmet needs. Currently, methods to predict tumor risk by investigating histopathological and clinical evidence such as tumor size, histological grade, histological subtype, or age are limited, and often fail to accurately stratify low- and high-risk patients (11–13). Tumor characteristics alone do not sufficiently determine patient outcomes in many malignancies likely due to heterogeneity in the patients, their innate immune response, and the genetic drivers of the disease. One way to integrate measurements of systemic immunity would be to include inflammation-associated cell enumeration, which can be easily obtained with a complete blood cell (CBC) count. The CBC count, the most frequently ordered laboratory test, consists of an automated hemogram and a five-cell automated differential count (14). The measurements in a CBC count include the white blood cell count consisting of neutrophils, lymphocytes, monocytes, eosinophils, and basophils, red blood cell count, hematocrit, hemoglobin, red blood cell indices (mean corpuscular volume, mean corpuscular hemoglobin, and mean corpuscular hemoglobin concentration), platelet count, and mean platelet volume. Select peripheral blood measurements also record information on important inflammatory markers such as CRP. Together, these components may have potential for prognosis in cancer patients. Compared to more invasive traditional diagnostic and staging tests, such as tumor size, histological grade, vascular invasion, lymph node metastases that would require surgery or expensive imaging techniques, a CBC, via a blood draw, is low cost and can be performed on a regular basis, with minimal risk to the patient.
An increasing body of evidence provides rationale for the utility of peripheral blood tests to predict cancer patient prognosis and treatment effectiveness. For example, a recent organism-wide study demonstrated that tumor eradication via immunotherapy requires peripheral immune cell activity (15), and another study showed that peripheral immune activation is predictive of recovery times following surgery in humans (16). Also notably, in various types of cancers the relative amounts of platelets and neutrophils to lymphocytes appear to be superior predictive measures as compared to assessing each component independently (17–24). Some scoring systems such as the Glasgow Prognostic Score (GPS), which measures systemic inflammation by monitoring CRP and albumin, are effective at predicting overall survival (OS) in many solid organ malignancies (25). This review will discuss studies that utilized neutrophil-to-lymphocyte ratio (NLR), platelet-to-lymphocyte ratio (PLR), and CRP to determine their value for cancer detection and prognosis.
Platelet-to-Lymphocyte Ratio
The PLR is defined as the relative number of platelets to lymphocytes, which, respectively, are hypothesized to have cancer-promoting and -fighting roles in circulation. Platelets are an integral part of hemostasis but have also been implicated to have a role in cancer progression. They can support cancer cell extravasation via the release of metalloproteases and promote tumor angiogenesis and growth at the metastatic site thought the release of growth factors, such as angiogenic factors, platelet-derived growth factor, and vascular endothelial growth factor (VEGF) (26), which enable tumor growth and metastatic spread (27–30). Platelets can also protect circulating tumor cells from killer T-cell-mediated cytolysis (31). In a symbiotic manner, cancer cells promote a platelet count increase and activation through the release of thrombopoietic cytokines and platelet agonists, respectively (9, 32, 33).
Lymphocytes are comprised of bone marrow-derived T and B cells. The B cell lineage produces antibodies that aid in the attack on invading bacteria, viruses, and toxins. T cells destroy host cells that been taken over by a virus or in select cases that have transformed to become cancerous. Lymphocytes have a crucial role in tumor defense by inducing cytotoxic death and inhibiting tumor cell proliferation and migration (5, 9).
The PLR has been shown to have predictive value in assessing the presence and progression of cancer and the response to drug therapy (34–36). In a meta-analysis of 18 studies which included 2,453 patients with ovarian cancer, PLR values were indicative of the stage of the disease and response to chemotherapy. The accuracy of predictions in these studies ranged from 55 to 80% (34). The variation in the performance was mainly attributed to the chosen cutoff for PLR. Although Polat et al. found an optimal cutoff for PLR to be 144.3 with 54% sensitivity and 59% specificity for malignancy prediction (37), Bakacak et al. found an optimal cut off to be 161.13 with 81.8% sensitivity and 50.8% specificity (38).
Platelet-to-lymphocyte has been shown to be a useful metric for determining the prognosis of a variety of cancers in patients. Increased PLR levels are a significant prognostic factor for poor prognosis in terms of OS in patients with gastric, colorectal, ovarian, hepatocellular, and lung cancers, some of which are summarized in Table 1 (18, 39–44). Raungkaewamee et al. observed that patients with a PLR of less than 200 had improved survival [progression-free survival (PFS), p = 0.003 and OS, p = 0.002] (42). However, the prognostic value of PLR remains controversial, as it failed to predict OS in several other studies (45–47). Xu et al. conducted a meta-analysis on PLR in patients with gastric cancer. Though the PLR was correlated with a higher risk of lymph node metastasis [odds ratio (OR) 1.5, 95% CI: 1.24–1.82] and increased the advanced stage cancer risk with (OR 1.99, 95% CI: 1.60–2.46), it was not a reliable predictor of OS, with an HR of 0.99 (0% CI: 0.9–1.1) (47).
Neutrophil-to-Lymphocyte Ratio
The NLR is a measurement of the relative number of neutrophils to lymphocytes in the peripheral blood and is simply derived from blood tests by dividing the absolute number of circulating neutrophils by the circulating lymphocyte population per volume. A few studies have also reported a derived NLR (d-NLR), which is the neutrophil count divided by the result of white cell count minus neutrophil count (48–51). Neutrophils play an essential role in frontline defense against pathogens by initiating and amplifying inflammatory reactions. Analogously to platelets, neutrophils can also interact with cancer cells, and produce cytokines and effector molecules such as VEGF that stimulate tumor angiogenesis, growth, and metastasis (52–56). Activated neutrophils can migrate from the venous system to tumor niches where they release large amounts of reactive oxygen species that can induce cell DNA damage and genetic instability giving rise to adenomas in the cancer microenvironment (5, 57). Interestingly, neutrophils can also have antitumor roles through direct and antibody-mediated cytotoxicity of tumor cells and activation of immune cell types such as T-cells and dendritic cells. Neutrophils can be activated by cancer cell-derived cytokines including myeloid growth factors, tumor necrosis factor (TNF) alpha, IL-10, IL-6, granulocyte colony-stimulating factors, and transforming growth factor beta (58–63). In murine models, inactivation of the tumor suppressor gene STK11/LKB1 led to significant increases in tumor-promoting cytokines and neutrophil activity; treatment of mice with an IL-6 antibody decreased the degree of tumor-associated neutrophils and improved survival as compared to control mice (64). Another insidious contribution of neutrophils is that they can encourage cancer-associated thrombosis through the generation of neutrophil extracellular traps (NETs), which are web-like structures composed of DNA, histones, and granule proteins (65). NETs have been shown to contribute to venous thrombosis in animal models (66–68). Yet these same NETs have been found to have an anti-cancer effect, as they can also serve as primers for T-cells (69).
An elevated NLR is associated with advanced stage cancer, high histological grade, and metastasis (70); moreover, an elevated NLR is also an independent prognostic factor of coronary artery disease, hypertension, chronic kidney disease, diabetes, heart failure, cerebrovascular disease, and peripheral arterial disease (71). NLR has been shown to be sensitive to atherosclerotic risk factors such as smoking, alcohol consumption, hypercholesterolemia, and metabolic syndrome (71).
According to some published works, NLR is a discriminating factor for distinguishing between malignant borderline and benign tumors (34, 37, 72, 73), particularly in ovarian cancer patients. A systematic review explored 18 studies involving 3,453 patients and found NLR values in ovarian cancer patients are significantly different from healthy controls, but the diagnostic accuracy remains limited. For example, in 63 patients who underwent laparotomy for adnexal mass evaluation, it was found that the NLR was significantly higher in malignant cases compared to benign cases (p < 0.05) and that the NLR had high sensitivity in detecting mucinous cancers (78%) (72). Another study of 275 women with ovarian masses (borderline, malignant, and benign) found that the NLR was a significant predictor (AUC = 0.604, p = 0.02) in malignant cases but not in borderline tumors (37). Cho et al. studied 192 patients with epithelial ovarian cancer, 173 with benign ovarian tumors, and 405 healthy controls and found that preoperative NLR was significantly higher (mean, 6.02) than that in benign ovarian tumor subjects (mean 2.57) and healthy controls (mean 1.98). The sensitivity and specificity of NLR in discriminating ovarian cancer was 66.1% (95% CI: 59.52–72.68%) and 82.7% (95% CI: 79.02–86.38%) (cutoff value: 2.60) (74).
Neutrophil-to-lymphocyte is also an established indicator of PFS and OS in multiple types of cancers. Clinical studies investigating NLR as a predictor of cancer patient survival are summarized in Table 2 (17, 50, 75–79) In a notable study of 12,118 patients with multiple types of cancers, reduced OS was associated with an NLR > 4 (HR—1.57, p < 0.001) or dNLR > 2 (HR—1.54, p < 0.001) prior to the diagnosis (50). NLR has also been useful in informing patient mortality following treatment. Nakamura et al. demonstrated that an NLR > 3.91 prior to chemotherapy was associated with increased mortality in the next 100 days (80). Yet there are many studies that have found no association between NLR and survival (42, 81). Disagreement in the literature could be explained by the biphasic roles of neutrophils and other inconsistencies that will be surveyed in the forthcoming “Potential Utility of Blood Measurements” section.
C-Reactive Protein
C-reactive protein is an acute phase non-specific inflammatory reactant that can be elevated due to an infection, invasive procedure, or medications (82, 83). CRP is released by the liver in response to increased levels of IL-6 released by activated macrophages, though it has also been found that IL-1 and TNF can also stimulate CRP synthesis (83). Additionally, tumor cells have been proposed as a potential trigger for CRP upregulation, as they secrete IL-6 and 8 to stimulate liver CRP production (5). CRP has a half-life of 19 h in the circulation and is a relatively stable marker of inflammation in comparison to select cytokines, which only have half-lives on the order of minutes (83). The GPS assigns a score of 0 to patients with a CRP < 10 mg/L and albumin ≥ 35 g/L, score of 1 to those with CRP ≥ 10 mg/L and albumin < 35 g/L and score of 2 for patients with CRP > 10 mg/L and albumin < 35 g/L. The modified Glasgow Prognostic Score (mGPS) is the same except a score of 1 is only dependent on having a CRP is greater than 10 mg/L (84–86).
GPS and mGPS descriptors have been shown to have a prognostic significance in patients with solid tumors, especially in gastrointestinal and kidney-related malignancies (87–92). Many of these clinical studies investigating the prognostic value of CRP are listed in Table 3 (93–100). It was also found that in pancreatic cancer patients, there is an association of only elevated CRP levels with poor clinical outcomes (OR: 1.6, 95% CI: 1.16–2.21) in a multivariate analysis (93). Furthermore, there is strong positive correlation between CRP and IL-6 levels found in advanced pancreatic cancer patients (101). Yet, there is not total agreement in the literature over the prognostic utility of CRP in select cancer types. In a meta-analysis, only three of six studies found low CRP levels to be associated with increased survival in pancreatic cancer. There is wide variation in cutoffs considered for these prognosis studies—around 34% used a CRP cutoff of <10 mg/L while other studies spanned from 2 to 50 mg/L CRP. The reason there may exist disparate cutoffs may be in part due to the highly variable nature of the peripheral blood-derived CRP levels. In order to reduce the amount of variability of CRP measurements, some researchers have instead quantified tumoral CRP over serum CRP for prognosis and recurrence predictions (102). There remains a need for larger studies to be conducted to define the importance of CRP as a biomarker for informing cancer staging and prognosis.
Multifactorial Analyses of CBC Parameters
Several studies have considered multiple parameters of CBC counts; some of which are summarized in Table 4 (1, 103–114). A few multifactorial analyses found just a single inflammation-related parameter that was an independent predictor of prognosis, which for the most part was NLR (51, 104, 106, 113, 115). For example, He et al. analyzed PLR and NLR in 243 patients with metastatic colorectal cancer and found that only NLR was a significant predictor for OS and disease-free survival (DFS). Similarly, Jia et al. investigated the prognostic significance of peripheral blood NLR, PLR, LMR, and individual platelet counts, lymphocytes, neutrophils, and monocytes individually for predicting DFS and OS in patients with breast cancer of different molecular subtypes. Multivariate analysis revealed that only NLR was a significant determinant of DFS and OS in all breast cancer patients (DFS, HR = 1.50 95% CI: 1.14–1.97, p = 0.004; OS, HR = 1.63, 95% CI: 1.07–2.49, p = 0.022) and in triple negative (negative for estrogen receptor, progesterone receptor, and human epidermal growth factor 2 receptor) breast cancer patients (DFS, HR = 2.58, 95% CI: 1.23–5.42, p = 0.012; OS, HR = 3.05, 95% CI: 1.08–8.61, p = 0.035) (106). Another study compared NLR, d-NLR, PLR, and LMR for predicting clinical outcome in 205 surgical colorectal cancer patients. Only elevated NLR was significantly associated with reduced PFS and OS in (RFS, HR 2.52, 95% CI: 1.65–3.83, p < 0.001; OS, HR 2.73, 95% CI: 1.74–4.29, p < 0.001) (51). A few studies found other CBC components that were useful outside of NLR. Some studies reported that mGPS but not NLR or PLR were independent prognostic factors of survival outcomes in surgically treated cancer patients (116, 117). Overall, even with the incorporation of CBC counts into multivariate analyses, mostly NLR remains significant. It remains to be determined whether the remaining insignificant covariates provide overlapping information with the significant covariate, or offer no prognostic value.
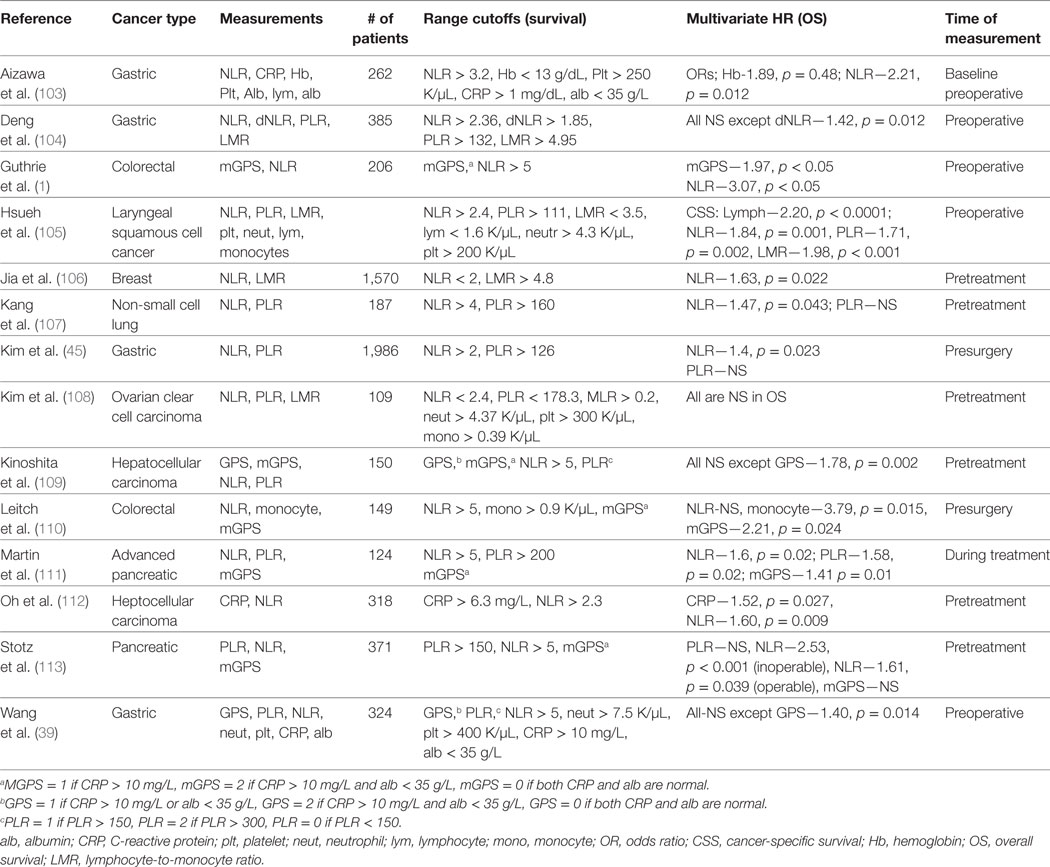
Table 4. Clinical studies investigating the role of multiple blood measurements in predicting cancer patient outcomes.
Other studies have found that multiple components of CBCs offer unique information that contribute to improved prediction of patient prognosis (105, 118, 119). Hsueh et al. investigated the prognostic value of preoperative counts of neutrophils, platelets, lymphocytes, and monocytes and ratios in 979 patients with laryngeal squamous cell cancer and measured prediction success of DFS and cancer-specific survival (CSS). They found that patients in the highest tertile of NLR (>2.4) and PLR (>111) were at higher risk of reduced DFS and CSS when compared using a multivariate analysis. The lowest tertile of lymphocytes (<1.6 × 109/L) and LMR (<3.5) were also associated with high risk of poor outcomes. Stotz et al. utilized several biomarkers such as hemoglobin, white blood cell count, NLR, d-NLR, LMR, and PLR in their prediction model for disease recurrence and survival in 149 gastrointestinal stroma tumor patients after surgical resection (19) and found significance in low hemoglobin (p = 0.029) and elevations in WBCs (p = 0.004), NLR (p = 0.015), and d-NLR (p = 0.037) being associated with poor OS in patients. Low Hb (0.049), WBC (p = 0.001), and elevated NLR (p = 0.007), d-NLR (p = 0.043), and PLR (p = 0.024) was associated with decreased RFS.
The majority of the studies mentioned up to this point have relied on single time point measurements around the time of treatment or diagnosis. In the next section, we discuss studies that have tracked inflammatory laboratory counts over multiple time points in patients and used this information to improve patient prognosis predictions.
Longitudinal Studies
Longitudinal trends of laboratory counts have primarily been accounted for by using counts prior to and after the diagnosis or treatment of a disease for prognosis prediction for patients with cancer and other malignancies. Guthrie et al. utilized longitudinal measurements of mGPS and NLR in colorectal cancer patients undergoing a curative resection. Measurements were accounted for at multiple time points prior to and following the operation. They discovered that preoperative mGPS and NLR and only the postoperative mGPS were independent predictors of prognosis (1). Derman et al. also monitored NLR in advanced non-small lung cell cancer patients before and 6 and 12 weeks after chemotherapy. The median NLR was 3.6 (range 0.1898–30.910) at baseline, 3.11 (range 0.2703–42.11) at 6 weeks and at 12 weeks it was 3.52 (range 0.2147–42.93) (120). A higher NLR at baseline, 6 and 12 weeks was associated with decreased OS.
In additional to monitoring laboratory counts surrounding a treatment, longitudinal measures could also be tracked in a trajectory analysis over various length scales and at other stages of the disease to determine if dynamic biological changes are indicative of a patient’s prognosis. One study demonstrated that the relative temporal changes of various blood cell parameters over a few days showed up in patterns that could uniquely identify different responses to infections in a mouse model (121). Additionally, it has been demonstrated that trajectories of body mass index over a lifetime are informative in improving prognosis predictions of prostate cancer patients (122). The same techniques could be applied with inflammation-related data and cancer, ideally prior to cancer treatment or diagnosis, before interventions significantly affect the lab counts.
Potential Utility of Blood Measurements
It is evident that these inflammation-related blood measurements can provide prognostic information. However, even though there have been several studies published on the prognostic significance of these factors in multiple types of cancers, they are not routinely used in a clinical setting and it remains unclear how to incorporate these data into management of patients with cancer. This could possibly be attributed to discrepancies and limitations found among previous studies. Meta-analyses of numerous studies have found inconsistent results relating to the prognostic value of blood cell parameters as predictors of survival. For example, Stevens et al. found that NLR and CRP only showed significance in two of eight articles and three of six articles, respectively (81). These inconsistencies might be due to the fact that the majority of the studies are retrospective, contain a small number of patients, and reflect heterogeneous populations. Blood cell population heterogeneity increases with patient age, nature of treatments performed, and comorbidities such as active infection, cardiovascular disease, pulmonary disease, and obesity, which are also associated with increased inflammatory markers (123–125). Additionally, the cancer cell histologic classification could greatly influence the variation reported within these studies. A meta-analysis on renal cell carcinoma (RCC) patients found heterogeneity in the types of populations recorded; two articles looked at clear-cell RCC and another article focused on non-clear cell RCC (126). It was also difficult to find consensus among studies that investigated mGPS and NLR due to heterogeneity of stages within the populations, four studies had patients with T1-T4 disease (79, 94, 127, 128), two studies with T1-T3 (129, 130), and two studies did not include T stage (131, 132). Moreover, the cutoff values that were applied for PLR-, NLR-, and CRP-related measurements were not standardized (21, 79, 126, 128). Cutoff values were instead chosen via a sensitivity analysis to maximize the receiver operating characteristic area under the curve. This method only ensures high prediction performance in a specific cohort from which the study was done and may not be generalizable to a larger population, making it less feasible for study validation or translation of these results to a clinical setting. Eventually, many of these issues could be resolved by planning and executing more carefully planned prospective studies with standardized cutoff values.
Observational studies on the blood measurements in cancer patients are subject to limitations. One concern would be that there is selection bias in the population that is mostly likely to have their laboratory counts measured. This could be especially problematic in longitudinal studies, in which cohorts are further filtered to patients that have measurements within a time period of interest. It is critical to consider that retrospective data has not been collected with the intent of creating a dataset for research. The CBC is typically ordered to diagnose a condition, screen for a condition, or monitor for preexisting conditions. Rimma et al. found that the laboratory’s test numerical value and rate of testing were correlated features, likely because when a value is outside of the normal range, additional testing is prompted until the value returns to a normal range (133). Additionally, another inherent problem is that the bias of missing data are not random; patients are seen and measured more often when they are sick, and measured less when they are healthy. Dynamics of certain lab tests vary; some are only measured in mixed patterns and biases can be disambiguated by accounting for the laboratory measurement frequency (133). One way to overcome measurements related to interventions would be algorithmically filtering laboratory values that have been taken in quick succession. Another limitation is that the models being used in these studies are not adequately equipped to deal with multiple components and time series analysis, in which there could be a substantial amount of information on the pathophysiological progression of the disease. Many of these limitations could be overcome by prospective studies as mentioned before, and employing computational methods that can handle missing data, remove noise and autocorrelation, handle larger population sizes, and extract complicated relationships among the data.
Leveraging Laboratory Data with Computational Models
Computational and mathematical models have been developed to address the complexity of high-dimensional biological data and potentially give some insight into the biological mechanisms of the disease. Two common types of computational modeling approaches include: (1) statistical and artificial intelligence (AI)based models, which reproduce a response with a given set of variables and all variables and/or parameters carry some uncertainty and (2) deterministic model which represent chemical and physical phenomenon in which there are some known relationships among the states and events (134).
A subset of methods within the field of machine learning/AI use deep learning, which is inspired by information processing in biological nervous systems. These tools can perform classification tasks similarly to an expert of a particular field and have been successful in subjects such as computer vision, speech recognition, bioinformatics, and natural language processing. These techniques are also being applied with longitudinal electronic health record (EHR) data. For example, Choi et al. used a longitudinal sequence of serum uric acid that were episodic in nature and accurately distinguished signatures of gout versus acute leukemia. Lipton et al. demonstrated that Recurrent Neural Networks, particularly those that use Long Short-Term Memory hidden units, are effective models for learning sequence data (135). These types of neural networks effectively model varying length sequences and capture long range dependencies. Even though EHR data are plagued with noisy and sparse data with irregularly timed observations, there are a variety of interpolation techniques employed in deep learning techniques to fill in missing data such as Gaussian process regression (136).
Mathematical modeling of the governing biochemical and biophysical phenomenon may also be instrumental in making cancer patient predictions. In one study, it was shown that an in silico framework based on cellular signaling interactions could predict the influence of eight different cytokines on the survival, duplication and differentiation of CD133+ hematopoietic stem and progenitor cells, which are essential for hematopoietic stem cell transplantation (137). This simulation which accounted for individual and combined effects of the cytokines was developed and validated with literature searches and in vitro experimental models. Computational models have also been instrumental in accounting for the combinatorial complexity required for melanoma drug discovery (138). These methods could be explored further when determining the best possible combination of biomarkers/cell counts to explore in a multivariate analysis.
Conclusion
In this review, we described the utility of inflammatory markers to improve cancer staging and prognosis predictions. We discussed the relationship between inflammation and cancer and described the notable blood measurements, such as platelets, neutrophils, lymphocytes, their relative ratios, and CRP. These studies are subject to limitations and inconsistencies, but with the addition of higher resolution data (multivariate and temporal), prospective studies, and advanced computational methods will have the potential to improve model predictions and yield a more thorough understanding of the pathophysiology of the disease.
Author Contributions
JS provided the initial conception, design, and writing of the article. AM and MA provided feedback, writing, and references for sections that involved mechanistic biology of platelets and immune cells, respectively. GT, JS, ST, and JL are all clinicians and each provided clinical research references, additional writing, and knowledge on current clinical care of cancer patients. PM and TW provided references, editing, and knowledge on statistics and new machine learning techniques to leverage this type of data. OM helped edit and write general sections of the article.
Conflict of Interest Statement
The authors declare that the research was conducted in the absence of any commercial or financial relationships that could be construed as a potential conflict of interest.
Funding
This work was supported by the Big Data Scientist Training Enhance Program (BD-STEP) fellowship to JS. Additionally, this study is funded by the National Institutes of Health (R01HL101972 to OM) and by the OHSU/OSU Cancer Prevention and Control Initiative. OM is an American Heart Association Established Investigator (13EIA12630000).
References
1. Guthrie GJK, Roxburgh CSD, Farhan-Alanie OM, Horgan PG, McMillan DC. Comparison of the prognostic value of longitudinal measurements of systemic inflammation in patients undergoing curative resection of colorectal cancer. Br J Cancer (2013) 109:24–8. doi:10.1038/bjc.2013.330
2. Allin KH, Bojesen SE, Nordestgaard BG. Baseline C-reactive protein is associated with incident cancer and survival in patients with cancer. J Clin Oncol (2009) 27:2217–24. doi:10.1200/JCO.2008.19.8440
3. Candido J, Hagemann T. Cancer-related inflammation. J Clin Immunol (2013) 33:79–84. doi:10.1007/s10875-012-9847-0
4. Servais C, Erez N. From sentinel cells to inflammatory culprits: cancer-associated fibroblasts in tumour-related inflammation. J Pathol (2013) 229:198–207. doi:10.1002/path.4103
6. Stoppacciaro A, Melani C, Parenza M, Mastracchio A, Bassi C, Baroni C, et al. Regression of an established tumor genetically modified to release granulocyte colony-stimulating factor requires granulocyte-T cell cooperation and T cell-produced interferon gamma. J Exp Med (1993) 178:151–61. doi:10.1084/jem.178.1.151
7. Bellocq A, Antoine M, Flahault A, Philippe C, Crestani B, Bernaudin JF, et al. Neutrophil alveolitis in bronchioloalveolar carcinoma: induction by tumor-derived interleukin-8 and relation to clinical outcome. Am J Pathol (1998) 152:83–92.
8. De Larco JE, Wuertz BRK, Furcht LT. The potential role of neutrophils in promoting the metastatic phenotype of tumors releasing interleukin-8. Clin Cancer Res (2004) 10:4895–900. doi:10.1158/1078-0432.CCR-03-0760
9. Mantovani A, Allavena P, Sica A, Balkwill F. Cancer-related inflammation. Nature (2008) 454:436–44. doi:10.1038/nature07205
10. Hanahan D, Weinberg RA. Hallmarks of cancer: the next generation. Cell (2011) 144:646–74. doi:10.1016/j.cell.2011.02.013
11. Fesinmeyer MD, Austin MA, Li CI, De Roos AJ, Bowen DJ. Differences in survival by histologic type of pancreatic cancer. Cancer Epidemiol Biomarkers Prev (2005) 14:1766–73. doi:10.1158/1055-9965.EPI-05-0120
12. Schmidt M, Böhm D, Von Törne C, Steiner E, Puhl A, Pilch H, et al. The humoral immune system has a key prognostic impact in node-negative breast cancer. Cancer Res (2008) 68:5405–13. doi:10.1158/0008-5472.CAN-07-5206
13. Matos JM, Schmidt CM, Turrini O, Agaram NP, Niedergethmann M, Saeger HD, et al. Pancreatic acinar cell carcinoma: a multi-institutional study. J Gastrointest Surg (2009) 13:1495–502. doi:10.1007/s11605-009-0938-z
14. Dixon LR. The complete blood count: physiologic basis and clinical usage. J Perinat Neonatal Nurs (1997) 11:1–18. doi:10.1097/00005237-199712000-00003
15. Spitzer MH, Carmi Y, Reticker-Flynn NE, Kwek SS, Madhireddy D, Martins MM, et al. Systemic immunity is required for effective cancer immunotherapy. Cell (2017) 168:487–502.e15. doi:10.1016/j.cell.2016.12.022
16. Gaudilliere B, Fragiadakis GK, Bruggner RV, Nicolau M, Finck R, Tingle M, et al. Coordinated surgical immune signatures contain correlates of clinical recovery. Sci Transl Med (2015) 6:255ra131. doi:10.1126/scitranslmed.3009701
17. Absenger G, Szkandera J, Pichler M, Stotz M, Arminger F, Weissmueller M, et al. A derived neutrophil to lymphocyte ratio predicts clinical outcome in stage II and III colon cancer patients. Br J Cancer (2013) 109:395–400. doi:10.1038/bjc.2013.346
18. Szkandera J, Gerger A, Liegl-Atzwanger B, Absenger G, Stotz M, Friesenbichler J, et al. The lymphocyte/monocyte ratio predicts poor clinical outcome and improves the predictive accuracy in patients with soft tissue sarcomas. Int J Cancer (2014) 135:362–70. doi:10.1002/ijc.28677
19. Stotz M, Liegl-Atzwanger B, Posch F, Mrsic E, Thalhammer M, Stojakovic T, et al. Blood-based biomarkers are associated with disease recurrence and survival in gastrointestinal stroma tumor patients after surgical resection. PLoS One (2016) 11:e0159448. doi:10.1371/journal.pone.0159448
20. Szkandera J, Pichler M, Absenger G, Stotz M, Arminger F, Weissmueller M, et al. The elevated preoperative platelet to lymphocyte ratio predicts decreased time to recurrence in colon cancer patients. Am J Surg (2014) 208:210–4. doi:10.1016/j.amjsurg.2013.10.030
21. Guthrie GJK, Charles KA, Roxburgh CSD, Horgan PG, McMillan DC, Clarke SJ. The systemic inflammation-based neutrophil-lymphocyte ratio: experience in patients with cancer. Crit Rev Oncol Hematol (2013) 88:218–30. doi:10.1016/j.critrevonc.2013.03.010
22. Huang SH, Waldron JN, Milosevic M, Shen X, Ringash J, Su J, et al. Prognostic value of pretreatment circulating neutrophils, monocytes, and lymphocytes in oropharyngeal cancer stratified by human papillomavirus status. Cancer (2015) 121:545–55. doi:10.1002/cncr.29100
23. Li J, Jiang R, Liu W-S, Liu Q, Xu M, Feng Q-S, et al. A large cohort study reveals the association of elevated peripheral blood lymphocyte-to-monocyte ratio with favorable prognosis in nasopharyngeal carcinoma. PLoS One (2013) 8:e83069. doi:10.1371/journal.pone.0083069
24. Azab B, Shah N, Radbel J, Tan P, Bhatt V, Vonfrolio S, et al. Pretreatment neutrophil/lymphocyte ratio is superior to platelet/lymphocyte ratio as a predictor of long-term mortality in breast cancer patients. Med Oncol (2013) 30:432. doi:10.1007/s12032-012-0432-4
25. McMillan DC. The systemic inflammation-based Glasgow prognostic score: a decade of experience in patients with cancer. Cancer Treat Rev (2013) 39:534–40. doi:10.1016/j.ctrv.2012.08.003
26. Bambace NM, Holmes CE. The platelet contribution to cancer progression. J Thromb Haemost (2011) 9:237–49. doi:10.1111/j.1538-7836.2010.04131.x
27. Sylman JL, Mitrugno A, Tormoen GW, Wagner TH, Mallick P, McCarty OJT. Platelet count as a predictor of metastasis and venous thromboembolism in patients with cancer. Converg Sci Phys Oncol (2017) 3:23001. doi:10.1088/2057-1739/aa6c05
28. McCarty OJ, Mousa SA, Bray PF, Konstantopoulos K. Immobilized platelets support human colon carcinoma cell tethering, rolling, and firm adhesion under dynamic flow conditions. Blood (2000) 96:1789–97. doi:10.1073/pnas.61.1.46
29. Goubran HA, Burnouf T, Radosevic M, El-Ekiaby M. The platelet–cancer loop. Eur J Intern Med (2013) 24:393–400. doi:10.1016/j.ejim.2013.01.017
30. Mitrugno A, Sylman JL, Ngo ATP, Pang J, Sears RC, Williams CD, et al. Aspirin therapy reduces the ability of platelets to promote colon and pancreatic cancer cell proliferation: implications for the oncoprotein c-MYC. Am J Physiol Cell Physiol (2017) 312:C176–89. doi:10.1152/ajpcell.00196.2016
31. Nieswandt B, Hafner M, Echtenacher B, Männel DN. Lysis of tumor cells by natural killer cells in mice is impeded by platelets. Cancer Res (1999) 59:1295–300.
32. Stone RL, Nick AM, McNeish IA, Balkwill F, Han HD, Bottsford-Miller J, et al. Paraneoplastic thrombocytosis in ovarian cancer. N Engl J Med (2012) 366:610–8. doi:10.1056/NEJMoa1110352
33. Wulaningsih W, Holmberg L, Garmo H, Malmstrom H, Lambe M, Hammar N, et al. Prediagnostic serum inflammatory markers in relation to breast cancer risk, severity at diagnosis and survival in breast cancer patients. Carcinogenesis (2015) 36:1121–8. doi:10.1093/carcin/bgv096
34. Prodromidou A, Andreakos P, Kazakos C, Vlachos DE, Perrea D, Pergialiotis V. The diagnostic efficacy of platelet-to-lymphocyte ratio and neutrophil-to-lymphocyte ratio in ovarian cancer. Inflamm Res (2017) 66:467–75. doi:10.1007/s00011-017-1026-6
35. Ozaksit G, Tokmak A, Kalkan H, Yesilyurt H. Value of the platelet to lymphocyte ratio in the diagnosis of ovarian neoplasms in adolescents. Asian Pac J Cancer Prev (2015) 16:2037–41. doi:10.7314/APJCP.2015.16.5.2037
36. Kemal Y, Demirağ G, Ekiz K, Yücel İ. Mean platelet volume could be a useful biomarker for monitoring epithelial ovarian cancer. J Obstet Gynaecol (2014) 34:515–8. doi:10.3109/01443615.2014.912620
37. Polat M, Senol T, Ozkaya E, Ogurlu Pakay G, Cikman MS, Konukcu B, et al. Neutrophil to lymphocyte and platelet to lymphocyte ratios increase in ovarian tumors in the presence of frank stromal invasion. Clin Transl Oncol (2016) 18:457–63. doi:10.1007/s12094-015-1387-7
38. Bakacak M, Serin S, Ercan Ö, Köstü B, Bostancı MS, Bakacak Z, et al. Utility of preoperative neutrophil-to-lymphocyte and platelet-to-lymphocyte ratios to distinguish malignant from benign ovarian masses. J Turk Ger Gynecol Assoc (2016) 17:21–5. doi:10.5152/jtgga.2015.0152
39. Wang Y, Xu F, Pan J, Zhu Y, Shao X, Sha J, et al. Platelet to lymphocyte ratio as an independent prognostic indicator for prostate cancer patients receiving androgen deprivation therapy. BMC Cancer (2016) 16:329. doi:10.1186/s12885-016-2363-5
40. Yu D, Liu B, Zhang L, Du K. Platelet count predicts prognosis in operable non-small cell lung cancer. Exp Ther Med (2013) 5:1351–4. doi:10.3892/etm.2013.1003
41. Krenn-Pilko S, Langsenlehner U, Thurner E-M, Stojakovic T, Pichler M, Gerger A, et al. The elevated preoperative platelet-to-lymphocyte ratio predicts poor prognosis in breast cancer patients. Br J Cancer (2014) 110:2524–30. doi:10.1038/bjc.2014.163
42. Raungkaewmanee S, Tangjitgamol S, Manusirivithaya S, Srijaipracharoen S, Thavaramara T. Platelet to lymphocyte ratio as a prognostic factor for epithelial ovarian cancer. J Gynecol Oncol (2012) 23:265–73. doi:10.3802/jgo.2012.23.4.265
43. Ozawa T, Ishihara S, Nishikawa T, Tanaka T, Tanaka J, Kiyomatsu T, et al. The preoperative platelet to lymphocyte ratio is a prognostic marker in patients with stage II colorectal cancer. Int J Colorectal Dis (2015) 30:1165–71. doi:10.1007/s00384-015-2276-9
44. Smith RA, Bosonnet L, Raraty M, Sutton R, Neoptolemos JP, Campbell F, et al. Preoperative platelet-lymphocyte ratio is an independent significant prognostic marker in resected pancreatic ductal adenocarcinoma. Am J Surg (2009) 197:466–72. doi:10.1016/j.amjsurg.2007.12.057
45. Kim EY, Lee JW, Yoo HM, Park CH, Song KY. The platelet-to-lymphocyte ratio versus neutrophil-to-lymphocyte ratio: which is better as a prognostic factor in gastric cancer? Ann Surg Oncol (2015) 22(13):4363–70. doi:10.1245/s10434-015-4518-z
46. Zhou X, Du Y, Huang Z, Xu J, Qiu T, Wang J, et al. Prognostic value of PLR in various cancers: a meta-analysis. PLoS One (2014) 9. doi:10.1371/journal.pone.0101119
47. Xu Z, Xu W, Cheng H, Shen W, Ying J, Cheng F, et al. The prognostic role of the platelet-lymphocytes ratio in gastric cancer: a meta-analysis. PLoS One (2016) 11:e0163719. doi:10.1371/journal.pone.0163719
48. Caputo D, Caricato M, Coppola A, La Vaccara V, Fiore M, Coppola R. Neutrophil to lymphocyte ratio (NLR) and derived neutrophil to lymphocyte ratio (d-NLR) predict non-responders and postoperative complications in patients undergoing radical surgery after neo-adjuvant radio-chemotherapy for rectal adenocarcinoma. Cancer Invest (2016) 34:440–51. doi:10.1080/07357907.2016.1229332
49. Dirican A, Kucukzeybek BB, Alacacioglu A, Kucukzeybek Y, Erten C, Varol U, et al. Do the derived neutrophil to lymphocyte ratio and the neutrophil to lymphocyte ratio predict prognosis in breast cancer? Int J Clin Oncol (2015) 20:70–81. doi:10.1007/s10147-014-0672-8
50. Proctor MJ, McMillan DC, Morrison DS, Fletcher CD, Horgan PG, Clarke SJ. A derived neutrophil to lymphocyte ratio predicts survival in patients with cancer. Br J Cancer (2012) 107:695–9. doi:10.1038/bjc.2012.292
51. Ying HQ, Deng QW, He BS, Pan YQ, Wang F, Sun HL, et al. The prognostic value of preoperative NLR, d-NLR, PLR and LMR for predicting clinical outcome in surgical colorectal cancer patients. Med Oncol (2014) 31:305. doi:10.1007/s12032-014-0305-0
52. Kusumanto YH, Dam WA, Hospers GAP, Meijer C, Mulder NH. Platelets and granulocytes, in particular the neutrophils, form important compartments for circulating vascular endothelial growth factor. Angiogenesis (2003) 6:283–7. doi:10.1023/B:AGEN.0000029415.62384.ba
53. Korkmaz B, Horwitz M, Jenne D, Gauthier F. Neutrophil elastase, proteinase 3, and cathepsin G as therapeutic targets in human diseases. Pharmacol Rev (2010) 62:726–59. doi:10.1124/pr.110.002733.726
54. Gastardelo TS, Cunha BR, Raposo LS, Maniglia JV, Cury PM, Lisoni FCR, et al. Inflammation and cancer: role of annexin A1 and FPR2/ALX in proliferation and metastasis in human laryngeal squamous cell carcinoma. PLoS One (2014) 9:e111317. doi:10.1371/journal.pone.0111317
55. Benevides L, Da Fonseca DM, Donate PB, Tiezzi DG, De Carvalho DD, De Andrade JM, et al. IL17 promotes mammary tumor progression by changing the behavior of tumor cells and eliciting tumorigenic neutrophils recruitment. Cancer Res (2015) 75:3788–99. doi:10.1158/0008-5472.CAN-15-0054
56. Galdiero MR, Bonavita E, Barajon I, Garlanda C, Mantovani A, Jaillon S. Tumor associated macrophages and neutrophils in cancer. Immunobiology (2013) 218:1402–10. doi:10.1016/j.imbio.2013.06.003
57. Weitzman SA, Gordon LI. Inflammation and cancer: role of phagocyte-generated oxidants in carcinogenesis. Blood (1990) 76:655–63.
58. Dumitru CA, Lang S, Brandau S. Modulation of neutrophil granulocytes in the tumor microenvironment: mechanisms and consequences for tumor progression. Semin Cancer Biol (2013) 23:141–8. doi:10.1016/j.semcancer.2013.02.005
59. Bellone G, Turletti A, Artusio E, Mareschi K, Carbone A, Tibaudi D, et al. Tumor-associated transforming growth factor-beta and interleukin-10 contribute to a systemic Th2 immune phenotype in pancreatic carcinoma patients. Am J Pathol (1999) 155:537–47. doi:10.1016/S0002-9440(10)65149-8
60. Kuper H, Adami HO, Trichopoulos D. Infections as a major preventable cause of human cancer. J Intern Med (2000) 248:171–83. doi:10.1046/j.1365-2796.2000.00742.x
61. Blaser MJ, Chyou PH, Nomura A. Age at establishment of Helicobacter pylori infection and gastric carcinoma, gastric ulcer, and duodenal ulcer risk. Cancer Res (1995) 55:562–5.
62. Scholl SM, Pallud C, Beuvon F, Hacene K, Stanley ER, Rohrschneider L, et al. Anti-colony-stimulating factor-1 antibody staining in primary breast adenocarcinomas correlates with marked inflammatory cell infiltrates and prognosis. J Natl Cancer Inst (1994) 86:120–6. doi:10.1093/jnci/86.2.120
64. Koyama S, Akbay EA, Li YY, Aref AR, Skoulidis F, Herter-Sprie GS, et al. STK11/LKB1 deficiency promotes neutrophil recruitment and proinflammatory cytokine production to suppress T-cell activity in the lung tumor microenvironment. Cancer Res (2016) 76:999–1008. doi:10.1158/0008-5472.CAN-15-1439
65. Brinkmann V, Reichard U, Goosmann C, Fauler B, Uhlemann Y, Weiss DS, et al. Neutrophil extracellular traps kill bacteria. Science (2004) 303:1532–5. doi:10.1126/science.1092385
66. Fuchs TA, Brill A, Duerschmied D, Schatzberg D, Monestier M, Myers DD, et al. Extracellular DNA traps promote thrombosis. Proc Natl Acad Sci U S A (2010) 107:15880–5. doi:10.1073/pnas.1005743107
67. Brill A, Fuchs TA, Savchenko AS, Thomas GM, Martinod K, de Meyer SF, et al. Neutrophil extracellular traps promote deep vein thrombosis in mice. J Thromb Haemost (2012) 10:136–44. doi:10.1111/j.1538-7836.2011.04544.x
68. Martinod K, Demers M, Fuchs TA, Wong SL, Brill A, Gallant M, et al. Neutrophil histone modification by peptidylarginine deiminase 4 is critical for deep vein thrombosis in mice. Proc Natl Acad Sci U S A (2013) 110:8674–9. doi:10.1073/pnas.1301059110
69. Tillack K, Breiden P, Martin R, Sospedra M. T lymphocyte priming by neutrophil extracellular traps links innate and adaptive immune responses. J Immunol (2012) 188:3150–9. doi:10.4049/jimmunol.1103414
70. Wang Y, Liu P, Xu Y, Zhang W, Tong L, Guo Z, et al. Preoperative neutrophil-to-lymphocyte ratio predicts response to first-line platinum-based chemotherapy and prognosis in serous ovarian cancer. Cancer Chemother Pharmacol (2015) 75:255–62. doi:10.1007/s00280-014-2622-6
71. Balta S, Demirkol S, Kucuk U, Sarlak H, Kurt O, Arslan Z. Neutrophil to lymphocyte ratio may predict mortality in breast cancer patients. J Breast Cancer (2013) 16:354–5. doi:10.4048/jbc.2013.16.3.354
72. Seckin KD, Karsli MF, Yucel B, Bestel M, Yildirim D, Canaz E, et al. The utility of tumor markers and neutrophil lymphocyte ratio in patients with an intraoperative diagnosis of mucinous borderline ovarian tumor. Eur J Obstet Gynecol Reprod Biol (2016) 196:60–3. doi:10.1016/j.ejogrb.2015.10.025
73. Yildirim M, Demir Cendek B, Filiz Avsar A. Differentiation between benign and malignant ovarian masses in the preoperative period using neutrophil-to-lymphocyte and platelet-to-lymphocyte ratios. Mol Clin Oncol (2015) 3:317–21. doi:10.3892/mco.2014.481
74. Cho H, Hur HW, Kim SW, Kim SH, Kim JH, Kim YT, et al. Pre-treatment neutrophil to lymphocyte ratio is elevated in epithelial ovarian cancer and predicts survival after treatment. Cancer Immunol Immunother (2009) 58:15–23. doi:10.1007/s00262-008-0516-3
75. Azab B, Bhatt VR, Phookan J, Murukutla S, Kohn N, Terjanian T, et al. Usefulness of the neutrophil-to-lymphocyte ratio in predicting short- and long-term mortality in breast cancer patients. Ann Surg Oncol (2012) 19:217–24. doi:10.1245/s10434-011-1814-0
76. Jung MR, Park YK, Jeong O, Seon JW, Ryu SY, Kim DY, et al. Elevated preoperative neutrophil to lymphocyte ratio predicts poor survival following resection in late stage gastric cancer. J Surg Oncol (2011) 104:504–10. doi:10.1002/jso.21986
77. Rachidi S, Wallace K, Wrangle JM, Day TA, Alberg AJ, Li Z. Neutrophil-to-lymphocyte ratio and overall survival in all sites of head and neck squamous cell carcinoma. Head Neck (2016) 38:E1068-74. doi:10.1002/hed.24159
78. Ubukata H, Motohashi G, Tabuchi T, Nagata H, Konishi S, Tabuchi T. Evaluations of interferon-γ/interleukin-4 ratio and neutrophil/lymphocyte ratio as prognostic indicators in gastric cancer patients. J Surg Oncol (2010) 102:742–7. doi:10.1002/jso.21725
79. Viers BR, Houston Thompson R, Boorjian SA, Lohse CM, Leibovich BC, Tollefson MK. Preoperative neutrophil-lymphocyte ratio predicts death among patients with localized clear cell renal carcinoma undergoing nephrectomy. Urol Oncol (2014) 32:1277–84. doi:10.1016/j.urolonc.2014.05.014
80. Nakamura K, Nagasaka T, Nishida T, Haruma T, Ogawa C, Kusumoto T, et al. Neutrophil to lymphocyte ratio in the pre-treatment phase of final-line chemotherapy predicts the outcome of patients with recurrent ovarian cancer. Oncol Lett (2016) 11:3975–81. doi:10.3892/ol.2016.4513
81. Stevens L, Pathak S, Nunes QM, Pandanaboyana S, Macutkiewicz C, Smart N, et al. Prognostic significance of pre-operative C-reactive protein and the neutrophil-lymphocyte ratio in resectable pancreatic cancer: a systematic review. HPB (2015) 17:285–91. doi:10.1111/hpb.12355
82. Koc M, Taysi S, Sezen O, Bakan N. Levels of some acute-phase proteins in the serum of patients with cancer during radiotherapy. Biol Pharm Bull (2003) 26:1494–7. doi:10.1248/bpb.26.1494
83. Black S, Kushner I, Samols D. C-reactive protein. J Biol Chem (2004) 279:48487–90. doi:10.1074/jbc.R400025200
84. Dutta S, Crumley ABC, Fullarton GM, Horgan PG, McMillan DC. Comparison of the prognostic value of tumour and patient related factors in patients undergoing potentially curative resection of gastric cancer. Am J Surg (2012) 204:294–9. doi:10.1016/j.amjsurg.2011.10.015
85. Ramsey S, Lamb GW, Aitchison M, McMillan DC. Prospective study of the relationship between the systemic inflammatory response, prognostic scoring systems and relapse-free and cancer-specific survival in patients undergoing potentially curative resection for renal cancer. BJU Int (2008) 101:959–63. doi:10.1111/j.1464-410X.2007.07363.x
86. Kobayashi T, Teruya M, Kishiki T, Endo D, Takenaka Y, Miki K, et al. Elevated C-reactive protein and hypoalbuminemia measured before resection of colorectal liver metastases predict postoperative survival. Dig Surg (2010) 27:285–90. doi:10.1159/000280021
87. Shrotriya S, Walsh D, Bennani-Baiti N, Thomas S, Lorton C. C-reactive protein is an important biomarker for prognosis tumor recurrence and treatment response in adult solid tumors: a systematic review. PLoS One (2015) 10:e0143080. doi:10.1371/journal.pone.0143080
88. Johnson TV, Abbasi A, Owen-Smith A, Young A, Ogan K, Pattaras J, et al. Absolute preoperative C-reactive protein predicts metastasis and mortality in the first year following potentially curative nephrectomy for clear cell renal cell carcinoma. J Urol (2010) 183:480–5. doi:10.1016/j.juro.2009.10.014
89. Kume H, Kakutani S, Yamada Y, Shinohara M, Tominaga T, Suzuki M, et al. Prognostic factors for renal cell carcinoma with bone metastasis: who are the long-term survivors? J Urol (2011) 185:1611–4. doi:10.1016/j.juro.2010.12.037
90. Steffens S, Köhler A, Rudolph R, Eggers H, Seidel C, Janssen M, et al. Validation of CRP as prognostic marker for renal cell carcinoma in a large series of patients. BMC Cancer (2012) 12:399. doi:10.1186/1471-2407-12-399
91. Koike Y, Miki C, Okugawa Y, Yokoe T, Toiyama Y, Tanaka K, et al. Preoperative C-reactive protein as a prognostic and therapeutic marker for colorectal cancer. J Surg Oncol (2008) 98:540–4. doi:10.1002/jso.21154
92. Han Y, Mao F, Wu Y, Fu X, Zhu X, Zhou S, et al. Prognostic role of C-reactive protein in breast cancer: a systematic review and meta-analysis. Int J Biol Markers (2011) 26:209–15. doi:10.5301/JBM.2011.8872
93. Szkandera J, Stotz M, Absenger G, Stojakovic T, Samonigg H, Kornprat P, et al. Validation of C-reactive protein levels as a prognostic indicator for survival in a large cohort of pancreatic cancer patients. Br J Cancer (2014) 110:183–8. doi:10.1038/bjc.2013.701
94. Lamb GWA, Aitchison M, Ramsey S, Housley SL, McMillan DC. Clinical utility of the Glasgow prognostic score in patients undergoing curative nephrectomy for renal clear cell cancer: basis of new prognostic scoring systems. Br J Cancer (2012) 106:279–83. doi:10.1038/bjc.2011.556
95. Komai Y, Saito K, Sakai K, Morimoto S. Increased preoperative serum C-reactive protein level predicts a poor prognosis in patients with localized renal cell carcinoma. BJU Int (2007) 99:77–80. doi:10.1111/j.1464-410X.2006.06497.x
96. Karakiewicz PI, Hutterer GC, Trinh QD, Jeldres C, Perrotte P, Gallina A, et al. C-reactive protein is an informative predictor of renal cell carcinoma-specific mortality: a European study of 313 patients. Cancer (2007) 110:1241–7. doi:10.1002/cncr.22896
97. Crumley ABC, Stuart RC, McKernan M, McMillan DC. Is hypoalbuminemia an independent prognostic factor in patients with gastric cancer? World J Surg (2010) 34:2393–8. doi:10.1007/s00268-010-0641-y
98. Tatokoro M, Saito K, Iimura Y, Fujii Y, Kawakami S, Kihara K. Prognostic impact of postoperative C-reactive protein level in patients with metastatic renal cell carcinoma undergoing cytoreductive nephrectomy. J Urol (2008) 180:515–9. doi:10.1016/j.juro.2008.04.025
99. Shimada H, Nabeya Y, Okazumi S-I, Matsubara H, Shiratori T, Aoki T, et al. Elevation of preoperative serum C-reactive protein level is related to poor prognosis in esophageal squamous cell carcinoma. J Surg Oncol (2003) 83:248–52. doi:10.1002/jso.10275
100. Shiu Y-C, Lin J-K, Huang C-J, Jiang J-K, Wang L-W, Huang H-C, et al. Is C-reactive protein a prognostic factor of colorectal cancer? Dis Colon Rectum (2008) 51:443–9. doi:10.1007/s10350-007-9133-z
101. Barber MD, Fearon KCH, Ross JA. Relationship of serum levels of interleukin-6, soluble interleukin-6 receptor and tumour necrosis factor receptors to the acute-phase protein response in advanced pancreatic cancer. Clin Sci (1999) 96:83–7. doi:10.1042/cs0960083
102. Nakatsu T, Motoyama S, Maruyama K, Usami S, Sato Y, Miura M, et al. Tumoral CRP expression in thoracic esophageal squamous cell cancers is associated with poor outcomes. Surg Today (2012) 42:652–8. doi:10.1007/s00595-012-0147-3
103. Aizawa M, Gotohda N, Takahashi S, Konishi M, Kinoshita T. Predictive value of baseline neutrophil/lymphocyte ratio for T4 disease in wall-penetrating gastric cancer. World J Surg (2011) 35:2717–22. doi:10.1007/s00268-011-1269-2
104. Deng Q, He B, Liu X, Yue J, Ying H, Pan Y, et al. Prognostic value of pre-operative inflammatory response biomarkers in gastric cancer patients and the construction of a predictive model. J Transl Med (2015) 13:66. doi:10.1186/s12967-015-0409-0
105. Hsueh C, Tao L, Zhang M, Cao W, Gong H, Zhou J. The prognostic value of preoperative neutrophils, platelets, lymphocytes, monocytes and calculated ratios in patients with laryngeal squamous cell cancer. Oncotarget (2017) 8:60514–27. doi:10.18632/oncotarget.16234
106. Jia W, Wu J, Jia H, Yang Y, Zhang X, Chen K, et al. The peripheral blood neutrophil-to-lymphocyte ratio is superior to the lymphocyte-to-monocyte ratio for predicting the long-term survival of triple-negative breast cancer patients. PLoS One (2015) 10(11):e0143061. doi:10.1371/journal.pone.0143061
107. Kang MH, Go S-I, Song H-N, Lee A, Kim S-H, Kang J-H, et al. The prognostic impact of the neutrophil-to-lymphocyte ratio in patients with small-cell lung cancer. Br J Cancer (2014) 111:452–60. doi:10.1038/bjc.2014.317
108. Kim HS, Choi HY, Lee M, Suh DH, Kim K, No JH, et al. Systemic inflammatory response markers and CA-125 levels in ovarian clear cell carcinoma: a two center cohort study. Cancer Res Treat (2016) 48:250–8. doi:10.4143/crt.2014.324
109. Kinoshita A, Onoda H, Imai N, Iwaku A, Oishi M, Fushiya N, et al. Comparison of the prognostic value of inflammation-based prognostic scores in patients with hepatocellular carcinoma. Br J Cancer (2012) 107:988–93. doi:10.1038/bjc.2012.354
110. Leitch EF, Chakrabarti M, Crozier JEM, McKee RF, Anderson JH, Horgan PG, et al. Comparison of the prognostic value of selected markers of the systemic inflammatory response in patients with colorectal cancer. Br J Cancer (2007) 97:1266–70. doi:10.1038/sj.bjc.6604027
111. Martin HL, Ohara K, Kiberu A, Van Hagen T, Davidson A, Khattak MA. Prognostic value of systemic inflammation-based markers in advanced pancreatic cancer. Intern Med J (2014) 44:676–82. doi:10.1111/imj.12453
112. Oh BS, Jang JW, Kwon JH, You CR, Chung KW, Kay CS, et al. Prognostic value of C-reactive protein and neutrophil-to-lymphocyte ratio in patients with hepatocellular carcinoma. BMC Cancer (2013) 13:78. doi:10.1186/1471-2407-13-78
113. Stotz M, Gerger A, Eisner F, Szkandera J, Loibner H, Ress AL, et al. Increased neutrophil-lymphocyte ratio is a poor prognostic factor in patients with primary operable and inoperable pancreatic cancer. Br J Cancer (2013) 109:416–21. doi:10.1038/bjc.2013.332
114. Wang DS, Ren C, Qiu MZ, Luo HY, Wang ZQ, Zhang DS, et al. Comparison of the prognostic value of various preoperative inflammation-based factors in patients with stage III gastric cancer. Tumour Biol (2012) 33:749–56. doi:10.1007/s13277-011-0285-z
115. He W, Yin C, Guo G, Jiang C, Wang F, Qiu H, et al. Initial neutrophil lymphocyte ratio is superior to platelet lymphocyte ratio as an adverse prognostic and predictive factor in metastatic colorectal cancer. Med Oncol (2013) 30:439. doi:10.1007/s12032-012-0439-x
116. Jamieson NB, Denley SM, Logue J, MacKenzie DJ, Foulis AK, Dickson EJ, et al. A prospective comparison of the prognostic value of tumor- and patient-related factors in patients undergoing potentially curative surgery for pancreatic ductal adenocarcinoma. Ann Surg Oncol (2011) 18:2318–28. doi:10.1245/s10434-011-1560-3
117. Pine JK, Fusai KG, Young R, Sharma D, Davidson BR, Menon KV, et al. Serum C-reactive protein concentration and the prognosis of ductal adenocarcinoma of the head of pancreas. Eur J Surg Oncol (2009) 35:605–10. doi:10.1016/j.ejso.2008.12.002
118. Huang B, Wang W, Bates M, Zhuang X. Three-dimensional {Super}-resolution {Imaging} by {Stochastic} {Optical} {Reconstruction} {Microscopy}. Science (2008) 319:810–3. doi:10.1126/science.1153529
119. Stotz M, Pichler M, Absenger G, Szkandera J, Arminger F, Schaberl-Moser R, et al. The preoperative lymphocyte to monocyte ratio predicts clinical outcome in patients with stage III colon cancer. Br J Cancer (2014) 110:435–40. doi:10.1038/bjc.2013.785
120. Derman BA, Macklis JN, Azeem MS, Sayidine S, Basu S, Batus M, et al. Relationships between longitudinal neutrophil to lymphocyte ratios, body weight changes, and overall survival in patients with non-small cell lung cancer. BMC Cancer (2017) 17(1):141. doi:10.1186/s12885-017-3122-y
121. Torres BY, Oliveira JH, Thomas Tate A, Rath P, Cumnock K, Schneider DS. Tracking resilience to infections by mapping disease space. PLoS Biol (2016) 14(4):e1002436. doi:10.1371/journal.pbio.1002436
122. Kelly SP, Graubard BI, Andreotti G, Younes N, Cleary SD, Cook MB. Prediagnostic body mass index trajectories in relation to prostate cancer incidence and mortality in the PLCO cancer screening trial. J Natl Cancer Inst (2017) 109:1–9. doi:10.1093/jnci/djw225
123. Heinrich J, Schulte H, Schonfeld R, Kohler E, Assmann G. Association of variables of coagulation, fibrinolysis and acute-phase with atherosclerosis in coronary and peripheral arteries and those arteries supplying the brain. Thromb Haemost (1995) 73:374–9.
124. Gan WQ, Man SFP, Senthilselvan A, Sin DD. Association between chronic obstructive pulmonary disease and systemic inflammation: a systematic review and a meta-analysis. Thorax (2004) 59:574–80. doi:10.1136/thx.2003.019588
125. Park HS, Park JY, Yu R. Relationship of obesity and visceral adiposity with serum concentrations of CRP, TNF-alpha and IL-6. Diabetes Res Clin Pract (2005) 69:29–35. doi:10.1016/j.diabres.2004.11.007
126. Grimes N, Tyson M, Hannan C, Mulholland C. A systematic review of the prognostic role of hematologic scoring systems in patients with renal cell carcinoma undergoing nephrectomy with curative intent. Clin Genitourin Cancer (2016) 14:271–6. doi:10.1016/j.clgc.2016.01.006
127. Qayyum T, McArdle PA, Lamb GW, Going JJ, Orange C, Seywright M, et al. Prospective study of the role of inflammation in renal cancer. Urol Int (2012) 88:277–81. doi:10.1159/000334971
128. Pichler M, Hutterer GC, Stoeckigt C, Chromecki TF, Stojakovic T, Golbeck S, et al. Validation of the pre-treatment neutrophil–lymphocyte ratio as a prognostic factor in a large European cohort of renal cell carcinoma patients. Br J Cancer (2013) 108:901–7. doi:10.1038/bjc.2013.28
129. Tai CG, Johnson TV, Abbasi A, Herrell L, Harris WB, Kucuk O, et al. External validation of the modified Glasgow prognostic score for renal cancer. Indian J Urol (2014) 30:33–7. doi:10.4103/0970-1591.124203
130. de Martino M, Pantuck AJ, Hofbauer S, Waldert M, Shariat SF, Belldegrun AS, et al. Prognostic impact of preoperative neutrophil-to-lymphocyte ratio in localized nonclear cell renal cell carcinoma. J Urol (2013) 190:1999–2004. doi:10.1016/j.juro.2013.06.082
131. Sejima T, Iwamoto H, Morizane S, Hinata N, Yao A, Isoyama T, et al. The significant immunological characteristics of peripheral blood neutrophil-to-lymphocyte ratio and Fas ligand expression incidence in nephrectomized tumor in late recurrence from renal cell carcinoma. Urol Oncol (2013) 31:1343–9. doi:10.1016/j.urolonc.2011.09.008
132. Hofbauer SL, Pantuck AJ, de Martino M, Lucca I, Haitel A, Shariat SF, et al. The preoperative prognostic nutritional index is an independent predictor of survival in patients with renal cell carcinoma. Urol Oncol (2015) 33:e1–68. doi:10.1016/j.urolonc.2014.08.005
133. Pivovarov R, Albers DJ, Sepulveda JL, Elhadad N. Identifying and mitigating biases in EHR laboratory tests. J Biomed Inform (2014) 51:24–34. doi:10.1016/j.jbi.2014.03.016
134. Motta S, Pappalardo F. Mathematical modeling of biological systems. Brief Bioinform (2013) 14:411–22. doi:10.1093/bib/bbs061
135. Lipton ZC, Kale DC, Elkan C, Wetzel R. Learning to diagnose with LSTM recurrent neural networks. (2016):1–18. doi:10.14722/ndss.2015.23268
136. Choi E, Bahadori MT, Schuetz A, Stewart WF, Sun J. Doctor AI: predicting clinical events via recurrent neural networks. JMLR Workshop Conf Proc (2016) 56:301–18.
137. Gullo F, Van Der Garde M, Russo G, Pennisi M, Motta S, Pappalardo F, et al. Computational modeling of the expansion of human cord blood CD133+ hematopoietic stem/progenitor cells with different cytokine combinations. Bioinformatics (2015) 31:2514–22. doi:10.1093/bioinformatics/btv172
Keywords: neutrophil-to-lymphocyte, platelet-to-lymphocyte, C-reactive protein, prognosis, cancer, longitudinal, biomarkers
Citation: Sylman JL, Mitrugno A, Atallah M, Tormoen GW, Shatzel JJ, Tassi Yunga S, Wagner TH, Leppert JT, Mallick P and McCarty OJT (2018) The Predictive Value of Inflammation-Related Peripheral Blood Measurements in Cancer Staging and Prognosis. Front. Oncol. 8:78. doi: 10.3389/fonc.2018.00078
Received: 04 January 2018; Accepted: 07 March 2018;
Published: 21 March 2018
Edited by:
Onder Alpdogan, Thomas Jefferson University, United StatesReviewed by:
Alessandro Isidori, AORMN Hospital, ItalyFrancesco Pappalardo, Università degli Studi di Catania, Italy
Copyright: © 2018 Sylman, Mitrugno, Atallah, Tormoen, Shatzel, Tassi Yunga, Wagner, Leppert, Mallick and McCarty. This is an open-access article distributed under the terms of the Creative Commons Attribution License (CC BY). The use, distribution or reproduction in other forums is permitted, provided the original author(s) and the copyright owner are credited and that the original publication in this journal is cited, in accordance with accepted academic practice. No use, distribution or reproduction is permitted which does not comply with these terms.
*Correspondence: Joanna L. Sylman, jsylman@gmail.com