- 1Center for Theoretical Biological Physics, Rice University, Houston, TX, United States
- 2Institute for Bioscience and Biotechnology Research, University of Maryland, Rockville, MD, United States
- 3Department of Physics, North Carolina State University, Raleigh, NC, United States
- 4Department of Chemistry and Biochemistry, University of Maryland, College Park, College Park, United States
- 5Department of Bioengineering, Rice University, Houston, TX, United States
- 6Department of Physics and Astronomy, Rice University, Houston, TX, United States
It is well known that genetic mutations can drive drug resistance and lead to tumor relapse. Here, we focus on alternate mechanisms—those without mutations, such as phenotypic plasticity and stochastic cell-to-cell variability that can also evade drug attacks by giving rise to drug-tolerant persisters. The phenomenon of persistence has been well-studied in bacteria and has also recently garnered attention in cancer. We draw a parallel between bacterial persistence and resistance against androgen deprivation therapy in prostate cancer (PCa), the primary standard care for metastatic disease. We illustrate how phenotypic plasticity and consequent mutation-independent or non-genetic heterogeneity possibly driven by protein conformational dynamics can stochastically give rise to androgen independence in PCa, and suggest that dynamic phenotypic plasticity should be considered in devising therapeutic dosing strategies designed to treat and manage PCa.
Introduction
Phenotypic plasticity, the ability of cells/organisms in a population to switch states (phenotypes) in response to environmental conditions despite identical genetic contents, can have far-reaching consequences (1). In particular, it is widely acknowledged that the stochastic differentiation of a population of genetically identical cells (in other words, a clonal population) into distinct phenotypes can offer survival advantage in unpredictable fluctuating environments (2, 3). The phenomenon of bacterial persistence—the ability of a subpopulation of a clonal bacterial population to survive exposure to high concentrations of an antibiotic—is a striking example of the advantages of phenotypic plasticity (4). The existence of persisters protects the population from extinction under sudden harsh conditions and accounts for prolonged and recurrent infections (5). Recently, the concept of phenotypic plasticity has gathered much attention in cancer biology as well. Genetically identical cancer cells can manifest diverse phenotypes during tumor progression via mechanisms, such as epithelial–mesenchymal transition (EMT) (6), mesenchymal-amoeboid transition (6, 7), and neuroendocrine differentiation (8, 9). Such phenotypic plasticity can facilitate metastasis and therapeutic resistance in cancer cells (10, 11). These examples have illustrated the dire unmet need to investigate the underlying mechanisms regulating phenotypic plasticity and consequent non-genetic heterogeneity.
Bacterial Persistence: A Hallmark of Phenotypic Plasticity
Many clonal bacterial populations respond to antibiotic drug treatment in a biphasic manner; the initial steep decrease in survival (fast killing rate) of a “normal” (drug-naïve) bacterial population is followed by a much slower decrease (slow killing rate), revealing the existence of persisters (4) (Figure 1A). These persisters, when isolated and regrown in the absence of drug, give rise to a population that is strikingly similar to the original population. When this population is exposed to the same antibiotic treatment, a similar time-kill curve is reproduced which was observed in the initial population, thereby indicating that the slower rate of killing of the persistent population is not permanent (Figure 1B). Thus, the phenomenon of persistence is different than that of resistance (defined as inherited ability of microorganisms, often due to genetic mutations, to grow at high concentrations of antibiotic irrespective of the duration of treatment) (4) (Figure 1A). Instead, bacterial persistence has been reported to act as a “phenotypic switch” where individual E. coli persisters stochastically transit into an actively growing state with their growth rate indistinguishable from the non-persisters and vice-versa (12) (Figures 1B,C). A lack of change in the persisters’ DNA sequence lends further credence to the idea that persistence is a non-genetic trait (13), i.e., the emergence of persisters need not depend on mutational or heritable changes in DNA sequence, but can result from diversity in cellular response to a repertoire of signals.
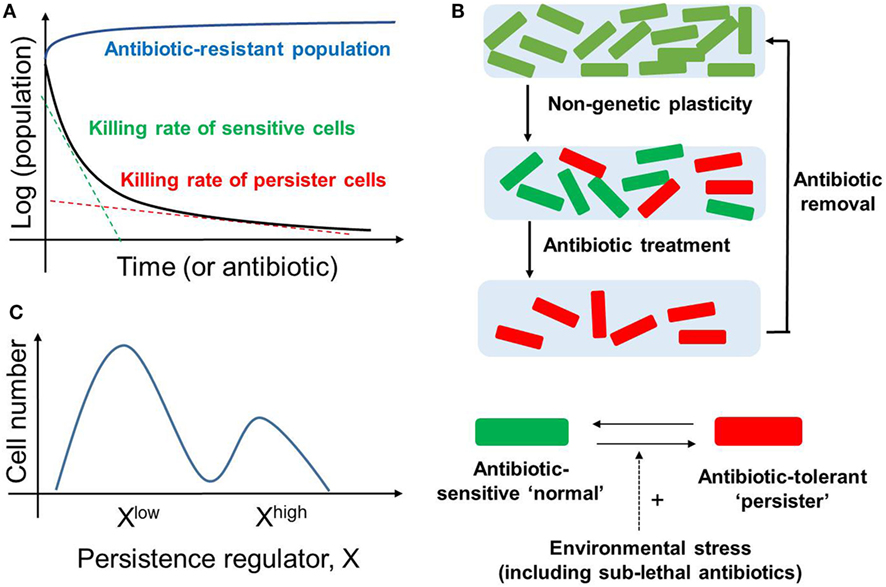
Figure 1. Bacterial Persistence. (A) Biphasic time-kill curve in bacterial populations exposed to antibiotics: faster killing rate of sensitive cell (green dotted line) followed by a slower killing rate (red dotted line) of persisters. In contrast, the antibiotic-resistant population continues to grow in presence of antibiotic (blue curve). (B) (top) An isogenic population of antibiotic sensitive cells can give rise to persisters via non-genetic/phenotypic plasticity. These slow-cycling persisters survive in the antibiotic treatment and tend to resume growth and generate a new population identical to the original population upon antibiotic removal (bottom). Persisters and non-persisters can switch among one another; the switching rate can be influenced by external stress factors. (C) Non-genetic heterogeneity of a key regulator of persistence (say X) in an isogenic population may give rise to two (or more) subpopulations that may continue switching stochastically among themselves to maintain persisters.
Direct single-cell and flow cytometry observations have suggested that persisters may arise as a subset of pre-existing dormant cells in an E. coli population (5). Specifically, some persister cells may have formed a priori even before the lethal antibiotic treatment. This pre-existing heterogeneity can be viewed as an example of “bet-hedging”—an evolutionary strategy that aims to maximize the fitness of an isogenic or a clonal population in dynamic environments through phenotypic heterogeneity, i.e., giving rise to two or more distinct subpopulations (14). Concomitant with this concept, bistability (existence of two distinct subpopulations that may reversibly transition to one another) in biochemical networks driving persistence has been proposed to give rise to persisters (15–17); this continued switching between different cell states can help to maintain a subpopulation of persisters (Figure 1C).
Another way of generating persisters is responsive diversification, where the application of sub-lethal levels of stress, including antibiotic treatment, can stimulate their formation (3, 5). Here, an initially homogeneous population can, while actively responding to the environmental change, generate stochastically different subpopulations of cells, via induced bistability in the underlying networks (18). The above-mentioned bacterial responses highlight how bacteria can deal efficiently with multiple antibiotics. Besides generating persisters, bacteria have been observed to display advanced social community skills, such as quorum sensing and developing biofilms to enhance their survival (19).
Drug-Tolerant Persisters (DTPs) and Mutation-Independent Phenotypic Switching in Cancer
More complicated and complex counterparts of the social features discussed earlier often drive adaptive tumor dynamics (20–23), for instance, cooperation among cancer cells in evading chemotherapy (24) and in successfully colonizing distant organs (25–28). “Complicatedness” refers to the number and diversity of components in a tumor microenvironment (TME) (29)—besides widespread intratumor clonal heterogeneity (30), TME contains diverse cell types, such as endothelial cells, macrophages, fibroblasts, and other immune cells (31). On the other hand, “complexity” refers to the gamut of regulatory connections among those components (29)—tumor cells communicate among themselves and with these stromal cells via multiple mechanical and/or chemical cues, and can thus alter cellular phenotypes reversibly (32–40). For example, M1 and M2 macrophages can affect epithelial–mesenchymal plasticity oppositely (34), whereas mesenchymal breast cancer cells can polarize macrophages toward M2 polarization (35). Nonlinear dynamics emerging from this multi-scale crosstalk defines the adaptive evolution of tumors and can dictate therapeutic response (41, 42). Thus, with this combination of clonal diversity and non-mutational mechanisms, such as dynamic phenotypic plasticity, the tumor, as an ecosystem, can withstand many therapeutic assaults and present clinically insuperable challenges of tumor relapse, metastasis, and therapy resistance (19).
While the implications of clonal diversity leading to therapy resistance and devising effective therapeutic strategies have been well-appreciated (43, 44), contributions of cellular plasticity driven by intrinsic (for example, the hypoxic or metabolic state of a cell) and/or extrinsic (for example, the chemokines or matrix stiffness a cell is exposed to) signals—without any essential complicity of genetic mutations (45–49)—have only recently begun to be elucidated. Here, we focus on the striking parallels between bacterial persistence and resistance of prostate cancer (PCa) cells against androgen deprivation therapy (ADT). These parallels aim to better understand how cancer, a community of heterogeneous subpopulations (19), may benefit from bet-hedging and thus evade multiple, potent-targeted therapies, and appreciate how cancer can exhibit traits of a robust, diverse, and adaptive social ecosystem.
Cancer has largely been considered a genetic disease driven by mutations (50). These primary and secondary mutations owing to clonal heterogeneity have been regarded as keystones of therapy resistance (51) (Figure 2A). However, the role of mutation-independent heterogeneity and phenotypic switching in cancer biology, such as cell-fate switching between a more dedifferentiated drug-resistant state and a well-differentiated drug-sensitive state in clonal or isogenic populations (32, 45), is gaining acceptance (46, 52). This dynamic cell-fate switching enables the emergence of multiple phenotypes from a single genotype, thus defying a precise linear genotype–phenotype mapping relationship and obfuscating the identification and targeting of mutations believed to be causal (53).
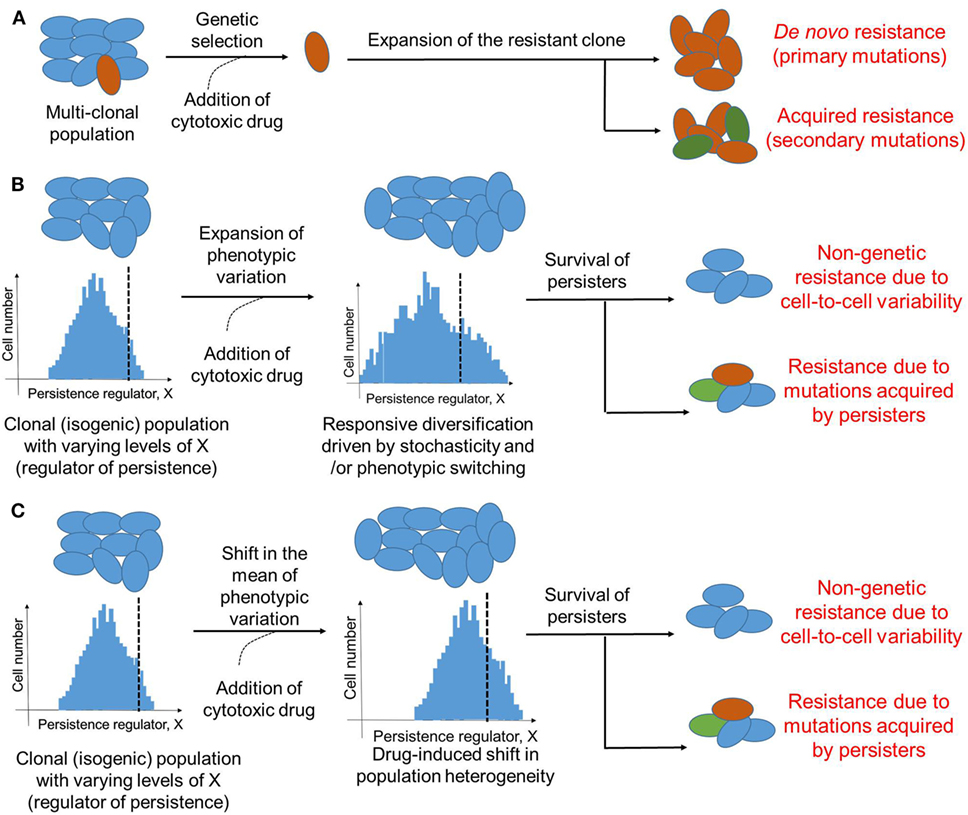
Figure 2. Modes of therapeutic resistance in cancer cells. (A) Cancer cells may resist cytotoxic drugs due to genetic mutations either pre-existing (de novo) in different clones that together constitute the cancer cell population, or those acquired during expansion of the drug-resistant clone. (B) An isogenic or a clonal population may be able to survive therapeutic assaults due to stochastic cell-to-cell variability of a key player regulating the formation of persisters. Drug treatment may enhance this non-genetic heterogeneity due to responsive diversification and/or drug-induced cellular reprogramming. This non-genetic heterogeneity can survive addition of cytotoxic drug, however, it can also lead to different acquired mutations by drug-tolerant persisters (DTPs) that can then be inherited by DTP clones. Dotted black line indicates which cells are persisters (to the right of it) versus which are not (to the left of it). (C) Same as (B), but without any change in variation of the levels of X; instead, the mean levels of X change. Cells shown in one color represent identical genetic makeup.
Striking recent observations in non-small cell lung cancer (NSCLC), melanoma, pancreatic ductal adenocarcinoma (PDAC), and breast cancer have illustrated the role of mutation-independent dynamic and adaptive phenotypic switching with implications in therapeutic design. For instance, treatment of multiple NSCLC cell lines sensitive to the epidermal growth factor receptor (EGFR) tyrosine kinase inhibition with a drug concentration 100-fold higher than the IC50 value led to the isolation of DTPs (45, 54). When propagated in drug-free media, DTPs resume growth and regain sensitivity to EGFR inhibition (45). This reversible phenomenon of persistence and the clonality of the population in which both persisters and non-persisters co-exist indicate that this phenotypic switching is mutation-independent (46). Similarly, some melanoma tumors that do not respond to B-raf proto-oncogene (BRAF) or mitogen-activated protein kinase inhibition may upregulate EGFR; this process can be reversed by discontinuing drug treatment, thereby re-sensitizing the apparently resistant cell population (54). Recent single-cell phenotyping and genome-wide transcriptomics reveal that in response to BRAF inhibition, many patient-derived BRAFV600-mutant cell lines undergo reversible cell-state transitions from a drug-naïve melanocytic state to a drug-resistant mesenchymal-like state (55). These transitions are driven not by selection of de novo genetically resistant clones, but instead result from the dynamics of underlying signaling networks (56) that can drive this adaptive transition (55). These instances of reversible and adaptive resistance against therapies are fundamentally different from de novo resistance (resistance due to “hard-wired” mechanisms, such as genetic mutations) and can help to explain clinical observations showing that some patients tend to regain sensitivity to BRAF inhibitor vemurafenib after a “drug holiday” (57). Furthermore, circulating tumor cells cultured from ER-positive/HER2-negative breast cancer patients revealed discrete HER2+ (proliferative) and HER2− (less proliferative, more drug-resistant) subpopulations that can interconvert spontaneously (58). Finally, a majority of PDAC cells were able to tolerate KRAS inhibition in both acute and sustained manner by adaptive switching through rewiring of signaling pathways (48). This switching did not invoke any significant mutational changes, underlining its non-genetic mechanism (48). These illustrative examples have motivated extensive investigations into phenotypic switching and DTPs in melanoma (47, 59, 60) and NSCLC (61), implying that drug resistance may be a reversible trait instead of a fixed modification, or that cells may dynamically enter and exit a window of drug resistance.
Similar mechanisms of phenotypic switching have been reported to regulate a dynamic equilibrium between cancer stem cells (CSCs)—a subpopulation with tumor-enhanced initiation potential and often enriched therapy resistance—and non-CSCs in breast cancer (62–64). These subpopulations have very similar, if not identical, genomic landscapes (62) and switching can be regulated by chromatin-mediated mechanisms (63), reminiscent of NSCLC studies (45), or cell–cell communication (32). Thus, similar to drug resistance, stemness need not be a static mutation-driven trait, but may be a functional reversible state that cancer cells can transiently adopt (46, 65, 66). Although the precise relation between DTPs and CSCs remains to be fully elucidated, mechanisms of drug resistance exhibited by CSCs and those by DTPs are remarkably similar (67).
Role of Stochasticity and Cell-Cell Communication in Generating DTPs
Given that persistence tends to optimize the fitness of a clonal population by distributing the limited community resources into phenotypically distinct subpopulations (5), it is not surprising that cell–cell communication may be instrumental in generating DTPs and/or CSCs via bet-hedging and/or responsive diversification mechanisms. Similar to quorum sensing in bacterial persisters (5), cell–cell communication via soluble cytokines can maintain a dynamic equilibrium of CSCs and non-CSCs (32). Similarly, DTPs isolated from multiple breast cancer cell lines (68) display enhanced Notch-Jagged signaling (69), an evolutionarily conserved cell–cell communication pathway that can contribute to multiple hallmarks of cancer (70, 71), and potentially stabilize a persister cell state (72).
Further, similar to stress-induced dynamic responsiveness in bacteria, phenotypic transitions in cancer cells can be induced by therapy (68, 73, 74). One way these transitions could happen is by enhancing the pre-existing stochastic non-genetic heterogeneity (75–77) (Figure 2B); an alternative mechanism could be by altering the mean levels of a key regulator of cell survival (Figure 2C). Stochasticity is a fundamental feature of biological systems because all biochemical reactions may contain random fluctuations given that no two cells have the exact same number of key components, such as RNA polymerase, transcription factors, etc., that can affect gene expression or activity (78). Such cell-to-cell variability has been implicated not only in modulating the probability of differentiation of embryonic stem cells into varied developmental lineages (79, 80), but also in improving population survival by diversifying cells to be able to survive stressful conditions (81, 82), i.e., by “bet-hedging”. Stochastic single-cell behavior can also play a crucial role in recreating the population heterogeneity of breast cancer cells; apparently homogeneous subpopulations of breast cancer cells exhibiting distinct phenotypes, when cultured in vitro separately, often return to equilibrium populations over time (83). This inherent cell-to-cell variability can be enhanced by drug treatment by pushing a cell population to different cell states (55). Taken together, these observations argue for taking into account the inherent noise or stochasticity while assessing and optimizing anti-cancer therapies (84).
It should be noted that although DTPs are exemplars of non-genetic heterogeneity, genetic and non-genetic aspects of surviving therapeutic assaults may be intertwined. For instance, DNA damage—a key driver of genomic instability and genetic heterogeneity—can trigger persistence in S. cerevisiae by activating stress response (85). Similarly, induction of SOS response (response to DNA damage in which cell cycle gets arrested) increases the fraction of persisters in E. coli (86). On the other hand, EGFR T790M mutations were observed in NSCLC DTPs that were T790M-negative a priori (61, 87), indicating that DTPs provide a pool of cells from which various genetic modes of resistance can evolve (87) (Figure 2B). In the EGFR-addicted NSCLC cell line PC9 that initially revealed the existence of DTPs upon EGFR tyrosine kinase inhibition (45), 17 different persister-derived erlotinib-resistant colonies (PERCs) were established from a single persister (87). These PERCs displayed different genetic mechanisms of resistance, such as T90M mutation in EGFR and MET amplification (87). These two acquired resistance mechanisms account for over half of clinically reported cases that develop resistance against EGFR inhibitors (88). Furthermore, recent studies in melanoma, where vemurafenib treatment converted a transient transcriptional state in a clonal population into stable clones exhibiting resistance against vemurafenib (47) argue that genetic and non-genetic causes of resistance are not mutually exclusive. These observations are reminiscent of bacterial persisters acquiring stable resistance against antibiotics (89), and suggest that transient effects due to drug-induced cellular reprogramming and/or cell-to-cell heterogeneity may prevent cancer cells from extinction by giving them time to acquire inheritable secondary mutations that can stably drive the progression to relapse. Furthermore, given the growth-arrested state of persisters, the mechanism by which they gain mutation(s) may be independent of cell division, for instance, genome instability driven through DNA damage and consequent repair. Thus, preventing the formation of these persisters may contribute to reduced resistance.
Non-Mutational Mechanisms of Androgen Independence in PCA
Prostate cancer is a leading cause of cancer incidence and cancer-related deaths in men. The 5-year survival rate of patients with local and regional PCa is almost 100%, but this rate drops to 28% in patients with metastasis to a distant organ (90). The primary standard of care therapy for locally advanced and metastatic PCa is ADT—surgical or chemical castration that lowers testosterone levels by stably suppressing androgen secretion (91, 92). This treatment has been in place for over 75 years, since Charles Huggins and colleagues described its efficacy in 1941 (92). While PCa patients typically respond well to ADT, most patients experience recurrence of the disease—termed as castration-resistant prostate cancer (CRPCa)—within 2–3 years of ADT (91). New treatments for CRPC, such as enzalutamide and abiretarone have been approved, but they extend median survival by merely 2–8 months (91), thus illustrating CRPC as an unmet urgent need.
Multiple mechanisms have been reported to contribute to resistance against ADT, such as increased expression of androgen receptor (AR), mutations in the ligand-binding domain of AR, and production of splice variants of AR (91) that can be upregulated in CRPC (93). Most frequently observed genetic aberrations in metastatic CRPC occur in AR, TP53, ETS family, RB1, and PTEN (94). Loss of PTEN function—often achieved by somatic mutations—has been correlated with worse survival (95) and can suppress the levels of androgen responsive genes by modulating AR activity (96). Loss of RB1 function enhances AR mRNA levels significantly and can induce resistance against ADT (97). Fusion of ETS family members, such as ERG to androgen-regulated gene TMPRSS2 can attenuate AR transcriptional activity, and thus drive selective pressure for development of PCa resistant to ADT (98). Also, inhibition of TP53 may diminish AR-mediated signaling (99). Thus, while no universal mechanism has been identified to drive evolution to CRPC, the AR pathway usually plays a key role (100, 101).
However, other non-mutational-based mechanisms similar to bacterial persistence may also contribute to this aggressive behavior. Metastatic CRPC has been reported to contain a mixture of cells displaying a range of AR expression levels (92). It is thus possible that this heterogeneity may exist a priori before the onset of ADT and/or is a product of responsive diversification, i.e., ADT induces the formation of these subpopulations from a clonal population. Recent evidence supports at least the former possibility, i.e., an isogenic population of PCa cells harbors a continuum of phenotypes with varying sensitivity to ADT, or, in other words, varying androgen-dependence. Different subclones established from a parental LNCaP cell line that is generally thought to be androgen-dependent had varying androgen sensitivity and AR activity levels that correlated with their different invasive and proliferative potential (102). Given that most of the differentially expressed genes among these clones were located on regions where no copy number variation was observed (102), the existence of these subclones possibly indicates a role of stochasticity or cell-to-cell variability in the control of AR activity levels.
Stochasticity or noise in a cell can arise due to multiple reasons. Besides the well-characterized transcriptional noise (103), there may be random fluctuations in the interaction networks themselves, especially those that comprise intrinsically disordered proteins (IDPs)—proteins that lack rigid 3D structures either along their entire length or in localized regions (104). Such promiscuity in interactions may give rise to “conformational noise” (104). IDPs have been found to be present as hub proteins in protein interaction networks from yeast to humans (105, 106), thus significantly impacting biological information transfer and propagating noise in signaling pathways. In contrast to well-defined energy landscapes of ordered proteins that determine their structure, IDPs may dynamically populate an ensemble of interconvertible structural conformations due to many local energy minima separated with low-energy barriers (107), especially when overexpressed (108). Several well-known oncogenes and tumor suppressor proteins, such as p53 (109), BRCA1 (110), PTEN (111), c-MYC (112, 113), and KRAS (114), and other key players regulating the formation of CSCs, such as LIN28, OCT4, NANOG, and SOX2 (115) have been reported to contain intrinsically disordered regions (IDRs). Further, many core modulators of EMT—a mechanism of phenotypic plasticity that shares molecular and functional overlaps with CSCs (116)—was predicted to contain IDRs (117). In the context of PCa, a striking example of an IDP is the key target of ADT itself, AR (118). Similarly, a majority of cancer/testis antigens (CTA)—a heterogeneous group of proteins that are typically expressed in testis with little or no expression in most somatic tissues, but aberrantly expressed in PCa—have been reported as IDPs (119).
Intrinsically disordered proteins may undergo a disorder-to-order transition to varying extents upon interacting with a cognate ligand, or upon specific post-translational modifications prior to ligand interaction (113, 120–122). Moreover, IDPs tend to have faster kinetics of interaction with their partners (faster binding/unbinding rates) (123), potentially amplifying promiscuity in interactions, and increasing stochasticity by allowing more flexibility in conformational switching. Considered together, these observations underscore the role of IDPs/IDPRs in phenotypic switching and thus the adaptability of biological systems in hostile environments (124, 125).
Our recent work employing multiple biophysical approaches illustrated how intrinsic disorder in a CTA named prostate-associated gene 4 (PAGE4) (126) can lead to its different conformations with implications for response to ADT (127). PAGE4 is a stress-response protein that is upregulated in response to many stress factors, such as inflammation; it is undetectable in normal adult glands, but aberrantly expressed in diseased gland and in prostatic lesions infiltrated with inflammatory cells (128). Epithelial PAGE4 correlates with and is an independent predictor of survival for patients with hormone-naïve PCa (129). PAGE4 is associated with attenuated AR signaling (129); one of the underlying mechanisms appears to involve the ability of PAGE4 to potentiate the transcription factor activator protein-1 (AP-1) (130) that can negatively regulate AR activity (131, 132). PAGE4 is phosphorylated by another component of the stress-response pathway homeodomain-interacting protein kinase 1 (HIPK1) predominantly at T51 which is critical for its ability to potentiate the transactivation of c-Jun (133), the most potent transcriptional activator of the AP-1 complex (134). PAGE4 is hyper-phosphorylated by CDC-like kinase 2 (CLK2) at many S/T residues, including T51. The interaction of PAGE4 with these two kinases leads to opposite functions. HIPK1-phosphorylated PAGE4 (HIPK1-PAGE4) potentiates c-Jun, while CLK2-phosphorylated PAGE4 (CLK2-PAGE4) attenuates c-Jun activity. This functional difference most likely arises from the different conformations of the PAGE4 ensemble, as elucidated using small-angle X-ray scattering, single-molecule fluorescence resonance energy transfer, and multidimensional NMR. HIPK1-PAGE4 exhibits a relatively compact conformational ensemble that binds AP-1, but CLK2-PAGE4 is more expanded and attains a random-coil conformation with less affinity for AP-1 (127).
As mentioned above, AP-1 can inhibit AR activity; moreover, AR can transcriptionally inhibit CLK2 (127), thereby forming a negative feedback loop in PAGE4/AR/AP-1 interactions. A recent mathematical model has predicted that this feedback loop can give rise to sustained or damped oscillations in the levels of AR activity, HIPK1-PAGE4 and CLK2-PAGE4 (Figure 3A), suggesting that androgen dependence of a cell can be a dynamic trait. Therefore, as the intracellular levels of HIPK1-PAGE4 and CLK2-PAGE4 vary dynamically, cells can go on phenotypic excursions with varying insensitivities to ADT [cells “resistant” to ADT have typically increased AR activity as an adaptive auto-regulatory mechanism (135)]. Additional interactions of these components could convert these oscillations into a multistable system. As already emphasized above, this heterogeneous population can thus potentially better evade the effects of ADT as compared to a homogeneous PCa population. This non-genetic mechanism is in contrast with the Darwinian clonal evolution model (136) which assumes the existence of mutually exclusive androgen-dependent and androgen-independent clones. Thus, in addition to genetic changes, phenotypic plasticity in PCa may be driven by underlying dynamics of the PAGE4/AP-1/AR circuit.
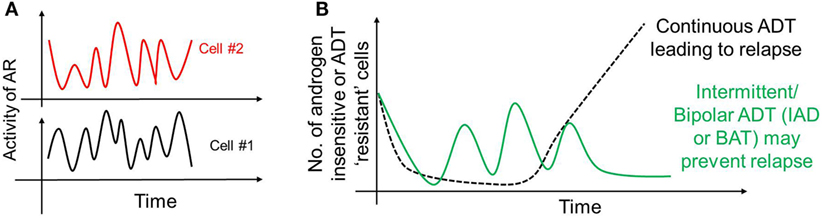
Figure 3. Non-genetic heterogeneity in prostate cancer. (A) Androgen receptor (AR)/prostate-associated gene 4 (PAGE4)/activator protein-1 (AP-1) circuit can give rise to oscillations of AR activity in a cell that can dynamically vary its dependence on androgen. These oscillations need not be synchronized across the population. (B) These oscillations, together with any other mechanisms of persistence, may survive a continuous androgen deprivation therapy and eventually regrow the entire population leading to tumor relapse (dotted black curve). However, “drug holidays,” such as intermittent androgen deprivation or bipolar androgen therapy may convert persisters to drug-sensitive cells, thus always keeping the number of androgen-independent (resistant) cells in check (solid green curve).
Another plasticity mechanism that has been recently reported to be associated with PCa relapsing from antiandrogen therapies is where PCa cells acquire morphologic features of neuroendocrine carcinoma, a cell lineage whose survival no longer depends on AR (137, 138). Similar to the observed plasticity between epithelial and mesenchymal phenotypes in breast cancer (6, 52), between proneural and mesenchymal phenotypes in glioma (139), and between neuroendocrine and mesenchymal phenotypes in small cell lung cancer (9), this more macroscopic plasticity in PCa may mediate cellular response to multiple therapies (8, 140) and serve as a hallmark for aggressive disease progression (141).
Implications of Dynamic Phenotypic Plasticity and Stochastic State Switching in Therapeutic Design
Resistance against various therapies can unquestionably result from secondary mutations (142–145) and/or pre-existing clones with specific genetic changes (43, 146–149). But, non-mutational stochastic cell-to-cell variability that can affect drug response and therapy-induced cellular reprogramming can also drive acquired resistance (45, 46, 52, 60, 61, 68, 73, 87, 150–152). Thus, similar to precision medicine attempts focusing on genomic landscape differences (153), effective therapeutic dosing strategies, and target identification calls for considering the effects of non-genetic heterogeneity and therapy-induced phenotypic plasticity that may give rise to persisters.
The existence of these persisters may offer a plausible explanation for the success of interval dosing therapeutic strategies in stalling tumor growth in many cancer types (57, 154–156). Such discontinuous treatment regimens may exploit the fitness disadvantage typically exhibited by the DTPs in the absence of drug (157), thereby leading to a regression of persister subpopulation. Particularly, in the context of PCa, treatment paradigms that involve cycles of ADT followed by no ADT [referred to as intermittent androgen deprivation (IAD)] (158) or ADT followed by supra-physiological dose of androgen [referred to as Bipolar ADT or bipolar androgen therapy (BAT)] (159) may be as good in terms of disease-free survival rates. Continuous ADT can result in a sustained pool of PCa persisters that may provide a latent reservoir of cells that can eventually acquire diverse genetic mutations accounting for stable drug-resistance, while intermittent ADT may discourage the maintenance of persisters, thus restricting phenotypic heterogeneity and resulting in higher disease-free survival rates (Figure 3B). Thus, an intermittent approach is likely to be more potent in targeting the vulnerabilities of different subpopulations at once, as compared to a continuous therapy treatment that can not only spare a set of recalcitrant population, but also stabilize a transient mechanism of drug resistance (160).
An alternative approach to intermittent or discontinuous dosing strategy is combinatorial therapy. A recent study that analyzed both human clinical trial data and the drug responses of various patient-derived xenografts (PDXs) highlighted how combinatorial therapy can be beneficial even without any synergy in drug actions, due to patient-to-patient variability (161). Combinatorial therapy has also shown initial promise in PTEN-deficient PCa, where PI3K and AR signaling inhibit each other, potentially generating multiple subpopulations (162). Inhibiting either pathway singly activates the other, enabling adaptive response. However, pharmacological inhibition of both these pathways causes almost complete regression of the disease both in PTEN-deficient PCa mouse models and in human prostate PDXs (162).
Combinatorial therapy can also help to target the vulnerabilities of DTPs. Goldman et al. (68) observed that the treatment of breast cancer cells with high concentrations of taxanes generates persisters that drive aggressive tumor formation in vivo. These persisters display activated Src family kinase/hemopoietic cell kinase pathways whose pharmacological inhibition in a temporally constrained manner led to enhanced apoptosis (68). Similarly, Deb et al. (163) identified two mutually exclusive clonal subpopulations in altered signaling states—one with upregulated pSTAT3 and the other with downregulated SMAD2/3—and targeted STAT3 and BCL6 (a transcription factor downstream of SMAD2/3) in a combinatorial manner to overcome non-genetic heterogeneity. Furthermore, dual inhibition of Wnt and Yes-associated protein (YAP) signaling can restrict the population of both epithelial-like and mesenchymal-like CSCs (164). These combinatorial therapies are reminiscent of combinations of drug pyrazinamide (that specifically targets M. tuberculosis persisters) with other canonical treatments (165). However, persisters in both bacterial and cancer cell populations can often be heterogeneous in their mechanisms and extent of drug-tolerance (4, 47, 150, 166). In fact, the very idea of defining IC50 (50% inhibitory concentration—the drug concentration where the viability of the population is half as that of the control case) implies that individual cells in a given population exhibit heterogeneous response to treat the cells with drug concentrations considered to be lethal (165). Valuable insights into the extent of heterogeneity can be gauged by other pharmacological parameters, such as the variability in maximum susceptibility of all cells in a given population to cell death, and the range of doses over which different subpopulations get killed (165).
Given that ADT has remained the primary standard of treatment for advanced/metastatic PCa for more than 75 years, we envisage that the conceptual framework outlined above can help to guide alternative treatment options. For example, clinicians may consider prescribing IAD or BAT, thus sparing the patient of the huge costs and undesirable side effects of chronic androgen deprivation. Indeed, in a recent report, albeit on just three cases, non-metastatic PCa patients were treated effectively with long-term primary IAD (167). Although IAD is not a standard therapy for patients with non-metastatic PCa, this exploratory clinical study underscores the benefits of challenging the cancer cell’s adaptive robustness due to its innate phenotypic plasticity. While the debate over the merits and controversies of administering either ADT regimen continues, and convincing data are needed to favor one over the other (157, 168), we trust the arguments presented here may inspire clinicians to reconsider treatment options and management of PCa that is currently estimated to strike one in every six men in the USA.
Phenotypic plasticity need not be a mechanism specific to PCa—it may also help normal prostate cells to cope with the significant diurnal variation (20–25%) in circulating testosterone levels in men (169). Thus, it is possible that as an adaptive evolutionary mechanism, PCa cells may be highly adept in phenotypic plasticity and persisting as a response to chronic and high fluctuations in hormonal levels and aggressive ADT—both of which represent the frequency of stressful conditions that can tune the rates of switching, and hence the frequency of persisters (170).
Conclusion
Phenotypic plasticity allows for a clone to sample many phenotypes—each with varying sensitivities—thus generating mutation-independent heterogeneity and enhancing clone survival. Therefore, phenotypic plasticity may serve as an effective bet-hedging strategy that may help overcome the varying selection pressures faced by a tumor (171). Here, we argue that besides genetically encoded resistance to ADT, PCa recurrence may also emerge from a phenomenon that bears a close resemblance to bacterial persistence—a bet-hedging strategy to face unpredictable harsh environmental fluctuations by generating non-genetic or mutation-independent phenotypic heterogeneity. Two crucial mechanisms underlying this heterogeneity—stochastic cell-to-cell variability and drug-induced cellular reprogramming—have already been implicated in forming DTPs. Here, we present one potential implementation strategy for generating cell-to-cell variability—the protein conformational dynamics of an intrinsically disordered protein PAGE4—that can generate dynamically varying AR levels in a cell, and thus give rise to different subpopulations, each with a varied sensitivity toward ADT. This “bet-hedging” may facilitate the presence of persisters—drug-tolerant reservoirs of cells from which multiple mechanisms of drug-resistance may evolve. Modulating inherent dynamic phenotypic plasticity and consequent heterogeneity may increase therapeutic efficacy.
Author Contributions
MKJ, PK, and HL conceptualized the idea. MKJ and PK wrote the first draft. JO and KW contributed to revising the manuscript.
Conflict of Interest Statement
The authors declare that the research was conducted in the absence of any commercial or financial relationships that could be construed as a potential conflict of interest.
The reviewer ML and handling editor declared their shared affiliation.
Funding
This work was supported by NSF Grant PHY-1427654 for the Center for Theoretical Biological Physics (HL), NSF DMS-1361411 (HL), NIH Grant CA 181730 (PK, JO), and NIH Grant GM 62154 (JO). MKJ received a training fellowship from the Gulf Coast Consortia on the Computational Cancer Biology Training Program (CPRIT Grant No. RP170593).
References
1. Pigliucci M, Murren CJ, Schlichting CD. Phenotypic plasticity and evolution by genetic assimilation. J Exp Biol (2006) 209:2362–7. doi:10.1242/jeb.02070
2. Hölzel M, Bovier A, Tüting T. Plasticity of tumour and immune cells: a source of heterogeneity and a cause for therapy resistance? Nat Rev Cancer (2012) 13:365–76. doi:10.1038/nrc3498
3. van Boxtel C, van Heerden JH, Nordholt N, Schmidt P, Bruggeman FJ. Taking chances and making mistakes: non-genetic phenotypic heterogeneity and its consequences for surviving in dynamic environments. J R Soc Interface (2017) 14:20170141. doi:10.1098/rsif.2017.0141
4. Brauner A, Fridman O, Gefen O, Balaban NQ. Distinguishing between resistance, tolerance and persistence to antibiotic treatment. Nat Rev Microbiol (2016) 14:320–30. doi:10.1038/nrmicro.2016.34
5. Harms A, Maisonneuve E, Gerdes K. Mechanisms of bacterial persistence during stress and antibiotic exposure. Science (2016) 354:aaf4268. doi:10.1126/science.aaf4268
6. Jolly MK, Boareto M, Huang B, Jia D, Lu M, Ben-Jacob E, et al. Implications of the hybrid epithelial/mesenchymal phenotype in metastasis. Front Oncol (2015) 5:155. doi:10.3389/fonc.2015.00155
7. Huang B, Jolly MK, Lu M, Tsarfaty I, Onuchic JN, Ben-Jacob E. Modeling the transitions between collective and solitary migration phenotypes in cancer metastasis. Sci Rep (2015) 5:17379. doi:10.1038/srep17379
8. Grigore AD, Ben-Jacob E, Farach-Carson MC. Prostate cancer and neuroendocrine differentiation: more neuronal, less endocrine? Front Oncol (2015) 5:37. doi:10.3389/fonc.2015.00037
9. Udyavar AA, Wooten DJ, Hoeksema M, Bansal M, Califano M, Estrada L, et al. Novel hybrid phenotype revealed in small cell lung cancer by a transcription factor network model that can explain tumor heterogeneity. Cancer Res (2017) 77:1063–74. doi:10.1158/0008-5472.CAN-16-1467
10. Brooks MD, Burness ML, Wicha MS. Therapeutic implications of cellular heterogeneity and plasticity in breast cancer. Cell Stem Cell (2015) 17:260–71. doi:10.1016/j.stem.2015.08.014
11. Reyes J, Lahav G. Leveraging and coping with uncertainty in the response of individual cells to therapy. Curr Opin Biotechnol (2018) 51:109–15. doi:10.1016/j.copbio.2017.12.007
12. Balaban NQ, Merrin J, Chait R, Kowalik K, Leibler S. Bacterial persistence as a phenotypic switch. Science (2004) 305:1622–5. doi:10.1126/science.1099390
13. Moyed HS, Bertrand KP. hipA, a newly recognized gene of Escherichia coli K-12 that affects frequency of persistence after inhibition of murein synthesis. J Bacteriol (1983) 155:768–75.
14. Veening J, Smits WK, Kuipers OP. Bistability, epigenetics, and bet-hedging in bacteria. Annu Rev Microbiol (2008) 62:193–212. doi:10.1146/annurev.micro.62.081307.163002
15. Feng J, Kessler DA, Ben-Jacob E, Levine H. Growth feedback as a basis for persister bistability. Proc Natl Acad Sci U S A (2013) 111:544–9. doi:10.1073/pnas.1320396110
16. Fasani RA, Savageau MA. Molecular mechanisms of multiple toxin – antitoxin systems are coordinated to govern the persister phenotype. Proc Natl Acad Sci U S A (2013) 110:E2528–37. doi:10.1073/pnas.1301023110
17. Rotem E, Loinger A, Ronin I, Levin-reisman I, Gabay C, Shoresh N, et al. Regulation of phenotypic variability by a threshold-based mechanism underlies bacterial persistence. Proc Natl Acad Sci U S A (2010) 107:12541–6. doi:10.1073/pnas.1004333107
18. Kotte O, Volkmer B, Radzikowski JL, Heinemann M. Phenotypic bistability in Escherichia coli’s central carbon metabolism. Mol Syst Biol (2014) 10:736. doi:10.15252/msb.20135022
19. Ben-Jacob E, Coffey DS, Levine H. Bacterial survival strategies suggest rethinking cancer cooperativity. Trends Microbiol (2012) 20:403–10. doi:10.1016/j.tim.2012.06.001
20. Lambert G, Estevez-Salmeron L, Oh S, Liao D, Emerson BM, Tlsty TD, et al. An analogy between the evolution of drug resistance in bacterial communities and malignant tissues. Nat Rev Cancer (2012) 11:375–82. doi:10.1038/nrc3039.An
21. Schwab ED, Pienta KJ. Cancer as a complex adaptive system. Med Hypotheses (1996) 47:235–41. doi:10.1016/S0306-9877(96)90086-9
22. Deisboeck TS, Couzin ID. Collective behavior in cancer cell populations. Bioessays (2009) 31:190–7. doi:10.1002/bies.200800084
23. Hickson J, Diane Yamada S, Berger J, Alverdy J, O’Keefe J, Bassler B, et al. Societal interactions in ovarian cancer metastasis: a quorum-sensing hypothesis. Clin Exp Metastasis (2009) 26:67–76. doi:10.1007/s10585-008-9177-z
24. Sulciner ML, Serhan CN, Gilligan MM, Mudge DK, Chang J, Gartung A, et al. Resolvins suppress tumor growth and enhance cancer therapy. J Exp Med (2017) 215(1):115–40. doi:10.1084/jem.20170681
25. Shahriari K, Shen F, Liu Q, Gong Y, Garcia FU, Fatatis A, et al. Cooperation among heterogeneous prostate cancer cells in the bone metastatic niche. Oncogene (2017) 36:2846–56. doi:10.1038/onc.2016.436
26. Aceto N, Bardia A, Miyamoto DT, Donaldson MC, Wittner BS, Spencer JA, et al. Circulating tumor cell clusters are oligoclonal precursors of breast cancer metastasis. Cell (2014) 158:1110–22. doi:10.1016/j.cell.2014.07.013
27. Grosse-Wilde A, Fouquier d’Herouei A, McIntosh E, Ertaylan G, Skupin A, Kuestner RE, et al. Stemness of the hybrid epithelial/mesenchymal state in breast cancer and its association with poor survival. PLoS One (2015) 10:e0126522. doi:10.1371/journal.pone.0126522
28. Richardson AM, Havel L, Koyen AE, Konen JM, Shupe JA, Wiles WG, et al. Vimentin is required for lung adenocarcinoma metastasis via heterotypic tumor cell-cancer-associated fibroblast interactions during collective invasion. Clin Cancer Res (2017) 24(2):420–32. doi:10.1158/1078-0432.CCR-17-1776
29. Bhat R, Pally D. Complexity: the organizing principle at the interface of biological (dis)order. J Genet (2017) 96:431–44. doi:10.1007/s12041-017-0793-8
30. Mcgranahan N, Swanton C. Clonal heterogeneity and tumor evolution: past, present, and the future. Cell (2017) 168:613–28. doi:10.1016/j.cell.2017.01.018
31. Balkwill FR, Capasso M, Hagemann T. The tumor microenvironment at a glance. J Cell Sci (2012) 125:5591–6. doi:10.1242/jcs.116392
32. Iliopoulos D, Hirsch HA, Wang G, Struhl K. Inducible formation of breast cancer stem cells and their dynamic equilibrium with non-stem cancer cells via IL6 secretion. Proc Natl Acad Sci U S A (2011) 108:1397–402. doi:10.1073/pnas.1018898108
33. Wei SC, Fattet L, Tsai JH, Guo Y, Pai VH, Majeski HE, et al. Matrix stiffness drives epithelial–mesenchymal transition and tumour metastasis through a TWIST1–G3BP2 mechanotransduction pathway. Nat Cell Biol (2015) 17:678–88. doi:10.1038/ncb3157
34. Yang M, Ma B, Shao H, Clark AM, Wells A. Macrophage phenotypic subtypes diametrically regulate epithelial-mesenchymal plasticity in breast cancer cells. BMC Cancer (2016) 16:419. doi:10.1186/s12885-016-2411-1
35. Sousa S, Brion R, Lintunen M, Kronqvist P, Sandholm J, Mönkkönen J, et al. Human breast cancer cells educate macrophages toward the M2 activation status. Breast Cancer Res (2015) 17:101. doi:10.1186/s13058-015-0621-0
36. Bocci F, Jolly MK, Tripathi SC, Aguilar M, Onuchic N, Hanash SM, et al. Numb prevents a complete epithelial – mesenchymal transition by modulating Notch signalling. J R Soc Interface (2017) 14:20170512. doi:10.1098/rsif.2017.0512
37. Tung JC, Barnes JM, Desai SR, Sistrunk C, Conklin MW, Schedin P, et al. Tumor mechanics and metabolic dysfunction. Free Radic Biol Med (2015) 79:269–80. doi:10.1016/j.freeradbiomed.2014.11.020
38. Katira P, Bonnecaze RT, Zaman MH. Modeling the mechanics of cancer: effect of changes in cellular and extra-cellular mechanical properties. Front Oncol (2013) 3:145. doi:10.3389/fonc.2013.00145
39. Webber J, Steadman R, Mason MD, Tabi Z, Clayton A. Cancer exosomes trigger fibroblast to myofibroblast differentiation. Cancer Res (2010) 70:9621–30. doi:10.1158/0008-5472.CAN-10-1722
40. Zhao H, Yang L, Baddour J, Achreja A, Bernard V, Moss T, et al. Tumor microenvironment derived exosomes pleiotropically modulate cancer cell metabolism. Elife (2016) 5:e10250. doi:10.7554/eLife.10250
41. Klemm F, Joyce JA. Microenvironmental regulation of therapeutic response in cancer. Trends Cell Biol (2015) 25:198–213. doi:10.1016/j.tcb.2014.11.006
42. Nagarsheth N, Wicha MS, Zou W. Chemokines in the cancer microenvironment and their relevance in cancer immunotherapy. Nat Rev Immunol (2017) 17:559–72. doi:10.1038/nri.2017.49
43. Burrell RA, Swanton C. Tumour heterogeneity and the evolution of polyclonal drug resistance. Mol Oncol (2014) 8:1095–111. doi:10.1016/j.molonc.2014.06.005
44. Brioli A, Melchor L, Cavo M, Morgan GJ. The impact of intra-clonal heterogeneity on the treatment of multiple myeloma. Br J Haematol (2014) 165:441–54. doi:10.1111/bjh.12805
45. Sharma SV, Lee DY, Li B, Quinlan MP, Takahashi F, Maheswaran S, et al. A chromatin-mediated reversible drug-tolerant state in cancer cell subpopulations. Cell (2010) 141:69–80. doi:10.1016/j.cell.2010.02.027
46. Pisco AO, Huang S. Non-genetic cancer cell plasticity and therapy-induced stemness in tumour relapse: “what does not kill me strengthens me”. Br J Cancer (2015) 112:1725–32. doi:10.1038/bjc.2015.146
47. Shaffer SM, Dunagin MC, Torborg SR, Torre EA, Emert B, Krepler C, et al. Rare cell variability and drug-induced reprogramming as a mode of cancer drug resistance. Nature (2017) 546:431–5. doi:10.1038/nature22794
48. Chen P-Y, Muzumdar MD, Dorans KJ, Robbins R, Bhutkar A, del Rosario A, et al. Adaptive and reversible resistance to Kras inhibition in pancreatic cancer cells. Cancer Res (2017) 78(4):985–1002. doi:10.1158/0008-5472.CAN-17-2129
49. Wooten DJ, Quaranta V. Mathematical models of cell phenotype regulation and reprogramming: make cancer cells sensitive again! Biochim Biophys Acta (2017) 1867:167–75. doi:10.1016/j.bbcan.2017.04.001
50. Stratton MR, Campbell PJ, Futreal PA. The cancer genome. Nature (2009) 458:719–24. doi:10.1038/nature07943
51. Turner NC, Reis-filho JS. Genetic heterogeneity and cancer drug resistance. Lancet Oncol (2012) 13:e178–85. doi:10.1016/S1470-2045(11)70335-7
52. Jia D, Jolly MK, Kulkarni P, Levine H. Phenotypic plasticity and cell fate decisions in cancer: insights from dynamical systems theory. Cancers (Basel) (2017) 9:70. doi:10.3390/cancers9070070
53. Brock A, Huang S. Precision oncology: between vaguely right and precisely wrong. Cancer Res (2017) 77:6473–9. doi:10.1158/0008-5472.CAN-17-0448
54. Sun C, Wang L, Huang S, Heynen GJJE, Prahallad A, Robert C, et al. Reversible and adaptive resistance to BRAF (V600E) inhibition in melanoma. Nature (2014) 508:118–22. doi:10.1038/nature13121
55. Su Y, Wei W, Robert L, Xue M, Tsoi J, Garcia-diaz A, et al. Single-cell analysis resolves the cell state transition and signaling dynamics associated with melanoma drug-induced resistance. Proc Natl Acad Sci U S A (2017) 114:13679–84. doi:10.1073/pnas.1712064115
56. Kolch W, Halasz M, Granovskaya M, Kholodenko BN. The dynamic control of signal transduction networks in cancer cells. Nat Rev Cancer (2015) 15:515–27. doi:10.1038/nrc3983
57. Seghers AC, Wilgenhof S, Lebbe C, Nyens B. Successful rechallenge in two patients with BRAF-V600-mutant melanoma who experienced previous progression during treatment with a selective BRAF inhibitor. Melanoma Res (2012) 22:466–72. doi:10.1097/CMR.0b013e3283541541
58. Jordan NV, Bardia A, Wittner BS, Benes C, Ligorio M, Zheng Y, et al. HER2 expression identifies dynamic functional states within circulating breast cancer cells. Nature (2016) 537:102–6. doi:10.1038/nature19328
59. Hoek KS, Goding CR. Cancer stem cells versus phenotype-switching in melanoma. Pigment Cell Melanoma Res (2010) 23:746–59. doi:10.1111/j.1755-148X.2010.00757.x
60. Fallahi-Sichani M, Becker V, Izar B, Baker GJ, Lin J-R, Boswell SA, et al. Adaptive resistance of melanoma cells to RAF inhibition via reversible induction of a slowly dividing de-differentiated state. Mol Syst Biol (2017) 13:905. doi:10.15252/msb
61. Hata AN, Niederst MJ, Archibald HL, Gomez-caraballo M, Siddiqui FM, Mulvey HE, et al. Tumor cells can follow distinct evolutionary paths to become resistant to epidermal growth factor receptor inhibition. Nat Med (2016) 22:262–9. doi:10.1038/nm.4040
62. Klevebring D, Rosin G, Ma R, Lindberg J, Czene K, Kere J, et al. Sequencing of breast cancer stem cell populations indicates a dynamic conversion between differentiation states in vivo. Breast Cancer Res (2014) 16:R72. doi:10.1186/bcr3687
63. Chaffer CL, Marjanovic ND, Lee T, Bell G, Kleer CG, Reinhardt F, et al. Poised chromatin at the ZEB1 promoter enables breast cancer cell plasticity and enhances tumorigenicity. Cell (2013) 154:61–74. doi:10.1016/j.cell.2013.06.005
64. Chaffer CL, Brueckmann I, Scheel C, Kaestli AJ, Wiggins PA, Rodrigues LO, et al. Normal and neoplastic nonstem cells can spontaneously convert to a stem-like state. Proc Natl Acad Sci U S A (2011) 108:7950–5. doi:10.1073/pnas.1102454108
65. Jolly MK, Jia D, Boareto M, Mani SA, Pienta KJ, Ben-Jacob E, et al. Coupling the modules of EMT and stemness: a tunable “stemness window” model. Oncotarget (2015) 6:25161–74. doi:10.18632/oncotarget.4629
66. Rooj AK, Ricklefs F, Mineo M, Nakano I, Chiocca EA, Bronisz A, et al. MicroRNA-mediated dynamic bidirectional shift between the subclasses of glioblastoma stem-like report microRNA-mediated dynamic bidirectional shift between the subclasses of glioblastoma. Cell Rep (2017) 19:2026–32. doi:10.1016/j.celrep.2017.05.040
67. Michiels JE, Van den Bergh B, Verstraeten N, Michiels J. Molecular mechanisms and clinical implications of bacterial persistence. Drug Resist Updat (2016) 29:76–89. doi:10.1016/j.drup.2016.10.002
68. Goldman A, Majumder B, Dhawan A, Ravi S, Goldman D, Kohandel M, et al. Temporally sequenced anticancer drugs overcome adaptive resistance by targeting a vulnerable chemotherapy-induced phenotypic transition. Nat Commun (2015) 6:6139. doi:10.1038/ncomms7139
69. Boareto M, Jolly MK, Goldman A, Pietila M, Mani SA, Sengupta S, et al. Notch-Jagged signaling can give rise to clusters of cells exhibiting a hybrid epithelial/mesenchymal phenotype. J R Soc Interface (2016) 13:20151106. doi:10.1098/rsif.2015.1106
70. Li D, Masiero M, Banham AH, Harris AL. The Notch ligand JAGGED1 as a target for anti-tumor therapy. Front Oncol (2014) 4:254. doi:10.3389/fonc.2014.00254
71. Boareto M, Jolly MK, Ben-Jacob E, Onuchic JN. Jagged mediates differences in normal and tumor angiogenesis by affecting tip-stalk fate decision. Proc Natl Acad Sci U S A (2015) 112:E3836–44. doi:10.1073/pnas.1511814112
72. Jolly MK, Boareto M, Lu M, Onuchic JN, Clementi C, Ben-Jacob E. Operating principles of Notch-Delta-Jagged module of cell-cell communication. New J Phys (2015) 17:55021. doi:10.1088/1367-2630/17/5/055021
73. Auffinger B, Tobias AL, Han Y, Lee G, Guo D, Dey M, et al. Conversion of differentiated cancer cells into cancer stem-like cells in a glioblastoma model after primary chemotherapy. Cell Death Dis (2014) 21:1119–31. doi:10.1038/cdd.2014.31
74. Skowron MA, Niegisch G, Fritz G, Arent T, van Roermund JG, Romano A, et al. Phenotype plasticity rather than repopulation from CD90/CK14+ cancer stem cells leads to cisplatin resistance of urothelial carcinoma cell lines. J Exp Clin Cancer Res (2015) 34:144. doi:10.1186/s13046-015-0259-x
75. Zimmer A, Amar-farkash S, Danon T, Alon U. Dynamic proteomics reveals bimodal protein dynamics of cancer cells in response to HSP90 inhibitor. BMC Syst Biol (2017) 11:33. doi:10.1186/s12918-017-0410-8
76. Cohen AA, Eden E, Issaeva I, Sigal A, Milo R, Liron Y, et al. Dynamic proteomics of individual cancer cells in response to a drug. Science (2008) 322:1511–7. doi:10.1126/science.1160165
77. El Meouche I, Siu Y, Dunlop MJ. Stochastic expression of a multiple antibiotic resistance activator confers transient resistance in single cells. Sci Rep (2016) 6:19538. doi:10.1038/srep19538
78. Kaern M, Elston TC, Blake WJ, Collins JJ. Stochasticity in gene expression: from theories to phenotypes. Nat Rev Genet (2005) 6:451–64. doi:10.1038/nrg1615
79. Abranches E, Guedes AMV, Moravec M, Maamar H, Svoboda P, Raj A, et al. Stochastic NANOG fluctuations allow mouse embryonic stem cells to explore pluripotency. Development (2014) 141:2770–9. doi:10.1242/dev.108910
80. Torres-Padilla M-E, Chambers I. Transcription factor heterogeneity in pluripotent stem cells: a stochastic advantage. Development (2014) 141:2173–81. doi:10.1242/dev.102624
81. Lidstrom ME, Konopka MC. The role of physiological heterogeneity in microbial population behavior. Nat Chem Biol (2010) 6:705–12. doi:10.1038/nchembio.436
82. Frank SA, Rosner MR. Nonheritable cellular variability accelerates the evolutionary processes of cancer. PLoS Biol (2012) 10:e1001296. doi:10.1371/journal.pbio.1001296
83. Gupta PB, Fillmore CM, Jiang G, Shapira SD, Tao K, Kuperwasser C, et al. Stochastic state transitions give rise to phenotypic equilibrium in populations of cancer cells. Cell (2011) 146:633–44. doi:10.1016/j.cell.2011.07.026
84. Sun X, Zhang J, Zhao Q, Chen X, Zhu W, Yan G, et al. Stochastic modeling suggests that noise reduces differentiation efficiency by inducing a heterogeneous drug response in glioma differentiation therapy. BMC Syst Biol (2016) 10:73. doi:10.1186/s12918-016-0316-x
85. Yaakov G, Lerner D, Bentele K, Steinberger J, Barkai N. Coupling phenotypic persistence to DNA damage increases genetic diversity in severe stress. Nat Ecol Evol (2017) 1:16. doi:10.1038/s41559-016-0016
86. Kreuzer KN. DNA damage responses in prokaryotes: regulating gene expression, modulating growth patterns, and manipulating replication forks. Cold Spring Harb Perspect Biol (2013) 5:a012674. doi:10.1101/cshperspect.a012674
87. Ramirez M, Rajaram S, Steininger RJ, Osipchuk D, Roth MA, Morinishi LS, et al. Diverse drug-resistance mechanisms can emerge from drug-tolerant cancer persister cells. Nat Commun (2016) 7:10690. doi:10.1038/ncomms10690
88. Camidge DR, Pao W, Sequist LV. Acquired resistance to TKIs in solid tumours: learning from lung cancer. Nat Rev Clin Oncol (2014) 11:473–81. doi:10.1038/nrclinonc.2014.104
89. Levin-reisman I, Ronin I, Gefen O, Braniss I, Shoresh N, Balaban NQ. Antibiotic tolerance facilitates the evolution of resistance. Science (2017) 355:826–30. doi:10.1126/science.aaj2191
90. Ziaee S, Chu GC, Huang J, Sieh S, Chung LWK. Prostate cancer metastasis: roles of recruitment and reprogramming, cell signal network and three-dimensional growth characteristics. Transl Androl Urol (2014) 4:438–54. doi:10.3978/j.issn.2223-4683.2015.04.10
91. Wadosky KM, Koochekpour S. Molecular mechanisms underlying resistance to androgen deprivation therapy in prostate cancer. Oncotarget (2016) 7:64447–70. doi:10.18632/oncotarget.10901
92. Watson PA, Arora VK, Sawyers CL. Emerging mechanisms of resistance to androgen receptor inhibitors in prostate cancer. Nat Rev Cancer (2015) 15:701–11. doi:10.1038/nrc4016
93. Hu R, Dunn TA, Wei S, Isharwal S, Veltri RW, Humphreys E, et al. Ligand-independent androgen receptor variants derived from splicing of cryptic exons signify hormone-refractory prostate cancer. Cancer Res (2009) 69:16–22. doi:10.1158/0008-5472.CAN-08-2764
94. Robinson D, Van Allen EM, Wu Y-M, Schultz N, Lonigro RJ, Mosquera J-M, et al. Integrative clinical genomics of advanced prostate resource integrative clinical genomics of advanced prostate cancer. Cell (2015) 161:1215–28. doi:10.1016/j.cell.2015.05.001
95. Ferraldeschi R, Nava D, Riisnaes R, Miranda S, Figueiredo I, Rescigno P, et al. PTEN protein loss and clinical outcome from castration-resistant prostate cancer treated with abiraterone acetate patient cohort. Eur Urol (2015) 67:795–802. doi:10.1016/j.eururo.2014.10.027
96. Mulholland DJ, Tran LM, Li Y, Cai H, Morim A, Wang S, et al. Article cell autonomous role of PTEN in regulating castration-resistant prostate cancer growth. Cancer Cell (2011) 19:792–804. doi:10.1016/j.ccr.2011.05.006
97. Sharma A, Yeow W, Ertel A, Coleman I, Clegg N, Thangavel C, et al. The retinoblastoma tumor suppressor controls androgen signaling and human prostate cancer progression. J Clin Invest (2010) 120:4478–92. doi:10.1172/JCI44239DS1
98. Yu J, Yu J, Mani R, Cao Q, Brenner CJ, Cao X, et al. An Integrated network of androgen receptor, polycomb, and TMPRSS2-ERG gene fusions in prostate cancer progression. Cancer Cell (2010) 17:443–54. doi:10.1016/j.ccr.2010.03.018
99. Cronauer MV, Schulz WA, Burchardt T, Ackermann R, Burchardt M. Inhibition of p53 function diminishes androgen receptor-mediated signaling in prostate cancer cell lines. Oncogene (2004) 23:3541–9. doi:10.1038/sj.onc.1207346
100. Ceder Y, Bjartell A, Culig Z, Rubin MA, Tomlins S, Visakorpi T, et al. The molecular evolution of castration-resistant prostate cancer. Eur Urol Focus (2016) 2:506–13. doi:10.1016/j.euf.2016.11.012
101. Obinata D, Takayama K, Takahashi S, Inoue S. Crosstalk of the androgen receptor with transcriptional collaborators: potential therapeutic targets for castration-resistant prostate cancer. Cancers (Basel) (2017) 9:9030022. doi:10.3390/cancers9030022
102. Terada N, Shiraishi T, Zeng Y, Aw-Yong KM, Mooney SM, Liu Z, et al. Correlation of Sprouty1 and Jagged1 with aggressive prostate cancer cells with different sensitivities to androgen deprivation. J Cell Biochem (2014) 115:1505–15. doi:10.1002/jcb.24805
103. Balázsi G, van Oudenaarden A, Collins JJ. Cellular decision making and biological noise: from microbes to mammals. Cell (2011) 144:910–25. doi:10.1016/j.cell.2011.01.030
104. Mahmoudabadi G, Rajagopalan K, Getzenberg RH, Hannenhalli S, Rangarajan G, Kulkarni P. Intrinsically disordered proteins and conformational noise implications in cancer. Cell Cycle (2013) 12:26–31. doi:10.4161/cc.23178
105. Haynes C, Oldfield CJ, Ji F, Klitgord N, Cusick ME, Radivojac P, et al. Intrinsic disorder is a common feature of hub proteins from four eukaryotic interactomes. PLoS Comput Biol (2006) 2:e100. doi:10.1371/journal.pcbi.0020100
106. Patil A, Kinoshita K, Nakamura H. Hub promiscuity in protein-protein interaction networks. Int J Mol Sci (2010) 11:1930–43. doi:10.3390/ijms11041930
107. Turoverov KK, Kuznetsova IM, Uversky VN. The protein kingdom extended: ordered and intrinsically disorderd proteins, their folding, supramolecular complex formation, and aggregation. Prog Biophys Mol Biol (2010) 102:73–84. doi:10.1016/j.pbiomolbio.2010.01.003.The
108. Vavouri T, Semple JI, Garcia-Verdugo R, Lehner B. Intrinsic protein disorder and interaction promiscuity are widely associated with dosage sensitivity. Cell (2009) 138:198–208. doi:10.1016/j.cell.2009.04.029
109. Wells M, Tidow H, Rutherford TJ, Markwick P, Jensen MR, Mylonas E, et al. Structure of tumor suppressor p53 and its intrinsically disordered N-terminal transactivation domain. Proc Natl Acad Sci U S A (2008) 105(15):5762–7. doi:10.1073/pnas.0801353105
110. Mark W, Liao JCC, Lu Y, Ayed A, Laister R, Szymczyna B, et al. Characterization of segments from the central region of BRCA1: an intrinsically disordered scaffold for multiple protein–protein and protein–DNA interactions? J Mol Biol (2005) 345:275–87. doi:10.1016/j.jmb.2004.10.045
111. Malaney P, Pathak RR, Xue B, Uversky VN, Dave V. Intrinsic disorder in PTEN and its interactome confers structural plasticity. Sci Rep (2013) 3:1–14. doi:10.1038/srep02035
112. Kumar D, Sharma N, Giri R. Therapeutic interventions of cancers using intrinsically disordered proteins as drug targets: c-Myc as model system. Cancer Inform (2017) 16:1–7. doi:10.1177/1176935117699408
113. Andresen C, Helander S, Lemak A, Farès C, Csizmok V, Carlsson J, et al. Transient structure and dynamics in the disordered c-Myc transactivation domain affect Bin1 binding. Nucleic Acids Res (2012) 40:6353–66. doi:10.1093/nar/gks263
114. Nussinov R, Jang H, Tsai C-J, Liao T-J, Li S, Fushman D, et al. Intrinsic protein disorder in oncogenic KRAS signaling. Cell Mol Life Sci (2017) 74:3245–61. doi:10.1007/s00018-017-2564-3
115. Xue B, Oldfield CJ, Van Y, Dunker AK, Uversky VN. Protein intrinsic disorder and induced pluripotent stem cells. Mol Biosyst (2012) 8:134–50. doi:10.1039/c1mb05163f
116. Jolly MK, Huang B, Lu M, Mani SA, Levine H, Ben-Jacob E. Towards elucidating the connection between epithelial–mesenchymal transitions and stemness. J R Soc Interface (2014) 11:20140962. doi:10.1098/rsif.2014.0962
117. Mooney SM, Jolly MK, Levine H, Kulkarni P. Phenotypic plasticity in prostate cancer: role of intrinsically disordered proteins. Asian J Androl (2016) 18:704–10. doi:10.4103/1008-682X.183570
118. McEwan IJ. Intrinsic disorder in the androgen receptor: identification, characterisation and drugability. Mol Biosyst (2012) 8:82–90. doi:10.1039/c1mb05249g
119. Rajagopalan K, Mooney SM, Parekh N, Getzenberg RH, Kulkarni P. A majority of the cancer/testis antigens are intrinsically disordered proteins. J Cell Biochem (2011) 112:3256–67. doi:10.1002/jcb.23252
120. Chakrabortee S, Meersman F, Kaminski Schierle GS, Bertoncini CW, McGee B, Kaminski CF, et al. Catalytic and chaperone-like functions in an intrinsically disordered protein associated with desiccation tolerance. Proc Natl Acad Sci U S A (2010) 107:16084–9. doi:10.1073/pnas.1006276107
121. Bah A, Vernon RM, Siddiqui Z, Krzeminski M, Muhandiram R, Zhao C, et al. Folding of an intrinsically disordered protein by phosphorylation as a regulatory switch. Nature (2015) 519:106–9. doi:10.1038/nature13999
122. He Y, Chen Y, Mooney SM, Rajagopalan K, Bhargava A, Sacho E, et al. Phosphorylation-induced conformational ensemble switching in an intrinsically disordered cancer/testis antigen. J Biol Chem (2015) 290:25090–102. doi:10.1074/jbc.M115.658583
123. Huang Y, Liu Z. Kinetic advantage of intrinsically disordered proteins in coupled folding–binding process: a critical assessment of the “fly-casting” mechanism. J Mol Biol (2009) 393:1143–59. doi:10.1016/j.jmb.2009.09.010
124. Xue B, Williams RW, Oldfield CJ, Dunker AK, Uversky VN. Archaic chaos: intrinsically disordered proteins in archaea. BMC Syst Biol (2010) 4:S1. doi:10.1186/1752-0509-4-S1-S1
125. Dunker AK, Bondos SE, Huang F, Oldfield CJ. Intrinsically disordered proteins and multicellular organisms. Semin Cell Dev Biol (2015) 37:44–55. doi:10.1016/j.semcdb.2014.09.025
126. Zeng Y, He Y, Yang F, Mooney S, Getzenberg R, Orban J, et al. The cancer/testis antigen prostate-associated gene 4 (PAGE4) is a highly intrinsically disordered protein. J Biol Chem (2011) 286:13985–94. doi:10.1074/jbc.M110.210765
127. Kulkarni P, Jolly MK, Jia D, Mooney SM, Bhargava A, Kagohara LT, et al. Phosphorylation-induced conformational dynamics in an intrinsically disordered protein and potential role in phenotypic heterogeneity. Proc Natl Acad Sci U S A (2017) 114(13):E2644–53. doi:10.1073/pnas.1700082114
128. Kulkarni P, Dunker KA, Weninger KR, Orban J. Prostate-associated gene 4 (PAGE4), an intrinsically disordered cancer/testis antigen, is a novel therapeutic target for prostate cancer. Asian J Androl (2016) 18:695–703. doi:10.4103/1008-682X.181818
129. Sampson N, Ruiz C, Zenzmaier C, Bubendorf L, Berger P. PAGE4 positivity is associated with attenuated AR signaling and predicts patient survival in hormone-naive prostate cancer. Am J Pathol (2012) 181:1443–54. doi:10.1016/j.ajpath.2012.06.040
130. Rajagopalan K, Qiu R, Mooney SM, Rao S, Shiraishi T, Sacho E, et al. The stress-response protein prostate-associated gene 4, interacts with c-Jun and potentiates its transactivation. Biochim Biophys Acta (2014) 1842:154–63. doi:10.1016/j.bbadis.2013.11.014
131. Sato M, Sadar M, Bruchovsky N, Saatcioglu F, Rennie P, Sato S, et al. Androgenic induction of prostate-specific antigen gene is repressed by protein-protein interaction between the androgen receptor and AP-1/c-Jun in the human prostate cancer cell line LNCaP. J Biol Chem (1997) 272:17485–94. doi:10.1074/jbc.272.28.17485
132. Tillman K, Oberfield JL, Shen XQ, Bubulya A, Shemshedini L. c-Fos dimerization with c-Jun represses c-Jun enhancement of androgen receptor transactivation. Endocrine (1998) 9:193–200. doi:10.1385/ENDO:9:2:193
133. Mooney SM, Qiu R, Kim JJ, Sacho EJ, Rajagopalan K, Johng D, et al. Cancer/testis antigen PAGE4, a regulator of c-Jun transactivation, is phosphorylated by homeodomain-interacting protein kinase 1, a component of the stress-response pathway. Biochemistry (2014) 53:1670–9. doi:10.1021/bi500013w
134. Shaulian E, Karin M. AP-1 as a regulator of cell life and death. Nat Cell Biol (2002) 4:131–6. doi:10.1038/ncb0502-e131
135. Isaacs JT, D’Antonio JM, Chen S, Antony L, Dalrymple SP, Ndikuyeze GH, et al. Adpative auto-regultion of androgen receptor provides a paradigm shifting rationale for bipolar androgen therapy (BAT) for castrate resistant human prostate cancer. Prostate (2012) 72:1491–505. doi:10.1002/pros.22504
136. Brock A, Chang H, Huang S. Non-genetic heterogeneity — a mutation-independent driving force for the somatic evolution of tumours. Nat Rev Genet (2009) 10:336–42. doi:10.1038/nrg2556
137. Ku SY, Rosario S, Wang Y, Mu P, Seshadri M, Goodrich ZW, et al. Rb1 and Trp53 cooperate to suppress prostate cancer lineage plasticity, metastasis, and antiandrogen resistance. Science (2017) 83:78–83. doi:10.1126/science.aah4199
138. Mu P, Zhang Z, Benelli M, Karthaus WR, Hoover E, Chen C-C, et al. SOX2 promotes lineage plasticity and antiandrogen resistance in TP53- and RB1-deficient prostate cancer. Science (2017) 355:84–8. doi:10.1126/science.aah4307
139. Segerman A, Niklasson M, Haglund C, Segerman B, Westermark B, Jarvius M, et al. Clonal variation in drug and radiation response among glioma-initiating cells is linked to proneural-mesenchymal transition article clonal variation in drug and radiation response among glioma-initiating cells is linked to proneural-mesenchymal transitio. Cell Rep (2016) 17:2994–3009. doi:10.1016/j.celrep.2016.11.056
140. Hu C-D, Choo R, Huang J. Neuroendocrine differentiation in prostate cancer: a mechanism of radioresistance and treatment failure. Front Oncol (2015) 5:90. doi:10.3389/fonc.2015.00090
141. Keener AB. Shapeshifters in cancer: how some tumor cells change phenotype to evade therapy. Nat Med (2016) 22:1194–6. doi:10.1038/nm1116-1194
142. Nazarian R, Shi H, Wang Q, Kong X, Koya RC, Lee H, et al. Melanomas acuire resistance to B-RAF (V600E) inhibition by RTK or N-RAS upregulation. Nature (2010) 468:973–7. doi:10.1038/nature09626
143. Sakai W, Swisher EM, Karlan BY, Agarwal MK, Friedman C, Villegas E, et al. Secondary mutations as a mechanism of cisplatin resistance in BRCA2-mutated cancers. Nature (2008) 451:1116–20. doi:10.1038/nature06633.Secondary
144. Gibaja V, Shen F, Harari J, Korn J, Ruddy D, Zhai H, et al. Development of secondary mutations in wild-type and mutant EZH2 alleles cooperates to confer resistance to EZH2 inhibitors. Oncogene (2016) 35:558–66. doi:10.1038/onc.2015.114
145. Suda K, Mizuuchi H, Maehara Y. Acquired resistance mechanisms to tyrosine kinase inhibitors in lung cancer with activating epidermal growth factor receptor mutation — diversity, ductility, and destiny. Cancer Metastasis Rev (2012) 31:807–14. doi:10.1007/s10555-012-9391-7
146. Turke AB, Zejnullahu K, Wu Y, Song Y, Dias-santagata D, Lifshits E, et al. Preexistence and clonal selection of MET amplification in EGFR mutant NSCLC. Cancer Cell (2010) 17:77–88. doi:10.1016/j.ccr.2009.11.022
147. Amado RG, Wolf M, Peeters M, Van Cutsem E, Siena S, Freeman DJ, et al. Wild-type KRAS is required for panitumumab efficacy in patients with metastatic colorectal cancer. J Clin Oncol (2008) 26:1626–34. doi:10.1200/JCO.2007.14.7116
148. Roche-lestienne C, Soenen-cornu V, Grardel-duflos N, Laı J, Philippe N, Facon T, et al. Several types of mutations of the Abl gene can be found in chronic myeloid leukemia patients resistant to STI571, and they can pre-exist to the onset of treatment. Blood (2002) 100:1014–9. doi:10.1182/blood.V100.3.1014
149. Van Allen EM, Wagle N, Sucker A, Treacy D, Goetz EM, Place CS, et al. The genetic landscape of clinical resistance to RAF inhibition in metastatic melanoms. Cancer Discov (2014) 4:94–109. doi:10.1158/2159-8290.CD-13-0617
150. Spencer SL, Gaudet S, Albeck JG, Burke JM, Sorger PK. Non-genetic origins of cell-to-cell variability in TRAIL-induced apoptosis. Nature (2009) 459:428–32. doi:10.1038/nature08012
151. Singh DK, Ku C, Wichaidit C, Iii RJS, Wu LF, Altschuler SJ. Patterns of basal signaling heterogeneity can distinguish cellular populations with different drug sensitivities. Mol Syst Biol (2010) 6:369. doi:10.1038/msb.2010.22
152. Knoechel B, Roderick JE, Williamson KE, Zhu J, Lohr JG, Cotton MJ, et al. An epigenetic mechanism of resistance to targeted therapy in T cell acute lymphoblastic leukemia. Nat Med (2014) 46:364–70. doi:10.1038/ng.2913
153. Chen C, He M, Zhu Y, Shi L, Wang X. Five critical elements to ensure the precision medicine. Cancer Metastasis Rev (2015) 34:313–8. doi:10.1007/s10555-015-9555-3
154. Das Thakur M, Salangsang F, Landman AS, Sellers WR, Pryer NK, Levesque MP, et al. Modelling vemurafenib resistance in melanoma reveals a strategy to forestall drug resistance. Nature (2013) 494:251–5. doi:10.1038/nature11814
155. Berry SR, Cosby R, Asmis T, Chan K, Hammad N, Krzyzanowska MK, et al. Continuous versus intermittent chemotherapy strategies in metastatic colorectal cancer: a systematic review and meta-analysis. Ann Oncol (2015) 26:477–85. doi:10.1093/annonc/mdu272
156. Amin AD, Rajan SS, Liang WS, Pongtornpipat P, Groysman MJ, Tapia EO, et al. Evidence suggesting that discontinuous dosing of ALK kinase inhibitors may prolong control of ALK þ tumors. Cancer Res (2015) 75:2916–28. doi:10.1158/0008-5472.CAN-14-3437
157. Hirata Y, Tanaka G, Bruchovsky N, Aihara K. Mathematically modelling and controlling prostate cancer under intermittent hormone therapy. Asian J Androl (2012) 14:270–7. doi:10.1038/aja.2011.155
158. Kratiras Z, Konstantinidis C, Skriapas K. A review of continuous vs intermittent androgen deprivation therapy: redefining the gold standard in the treatment of advanced prostate cancer. Myths, facts and new data on a “perpetual dispute”. Int Braz J Urol (2014) 40:3–15. doi:10.1590/S1677-5538.IBJU.2014.01.02
159. Schweizer MT, Antonarakis ES, Wang H, Ajiboye A, Spitz A, Cao H, et al. Effect of bipolar androgen therapy for asymptomatic men with castration-resistant prostate cancer: results from a pilot clinical study. Sci Transl Med (2015) 7:269ra2. doi:10.1126/scitranslmed.3010563
160. Salgia R, Kulkarni P. The genetic/non-genetic duality of drug “resistance”. Trends Cancer (2018) 4(2):110–18. doi:10.1016/j.trecan.2018.01.001
161. Palmer AC, Sorger PK. Combination cancer therapy can confer benefit via patient-to-patient variability without drug additivity or synergy. Cell (2017) 171:1678–91. doi:10.1016/j.cell.2017.11.009
162. Carver BS, Chapinski C, Wongvipat J, Hieronymus H, Chen Y, Chandarlapaty S, et al. Reciprocal feedback regulation of PI3K and androgen receptor signaling in PTEN-deficient prostate cancer. Cancer Cell (2011) 19:575–86. doi:10.1016/j.ccr.2011.04.008
163. Deb D, Rajaram S, Larsen JE, Dospoy PD, Marullo R, Li LS, et al. Combination therapy targeting BCL6 and phospho-STAT3 defeats intratumor heterogeneity in a subset of non – small cell lung cancers. Cancer Res (2017) 77:3070–82. doi:10.1158/0008-5472.CAN-15-3052
164. Sulaiman A, McGarry S, Li L, Jia D, Ooi S, Addison C, et al. (2018) Available from: http://onlinelibrary.wiley.com/doi/10.1002/1878-0261.12167/full
165. Inde Z, Dixon SJ. The impact of non-genetic heterogeneity on cancer cell death. Crit Rev Biochem Mol Biol (2017) 53:99–114. doi:10.1080/10409238.2017.1412395
166. Joers A, Kaldalu N, Tenson T. The frequency of persisters in Escherichia coli reflects the kinetics of awakening from dormancy. J Bacteriol (2010) 192:3379–84. doi:10.1128/JB.00056-10
167. Shida Y, Hakariya T, Miyata Y, Sakai H. Three cases of nonmetastatic prostate cancer treated successfully with primary intermittent androgen deprivation therapy over 10 years. Clin Case Rep (2017) 5:425–8. doi:10.1002/ccr3.854
168. Bruce JY, Lang JM, McNeel DG, Liu G. Current controversies in the management of biochemical failure in prostate cancer. Clin Adv Hematol Oncol (2012) 10:716–22.
169. Brambilla DJ, Mastumoto AM, Araujo AB, McKinlay JB. The effect of diurnal variation on clinical measurement of serum testosterone and other sexhormone levels in men. J Clin Endocrinol Metab (2009) 94:907–13. doi:10.1210/jc.2008-1902
170. Pearl S, Oppenheim A, Balaban NQ. Epigentic variability in a predator-prey system. In: Gissis S, Jablonka E, editors. Transformations of Lamarckism: From Subtle Fluids to Molecular Biology. Cambridge, MA: MIT Press.
Keywords: bet-hedging, stochasticity, androgen independence, non-genetic heterogeneity, phenotypic plasticity, intermittent androgen therapy
Citation: Jolly MK, Kulkarni P, Weninger K, Orban J and Levine H (2018) Phenotypic Plasticity, Bet-Hedging, and Androgen Independence in Prostate Cancer: Role of Non-Genetic Heterogeneity. Front. Oncol. 8:50. doi: 10.3389/fonc.2018.00050
Received: 28 October 2017; Accepted: 19 February 2018;
Published: 06 March 2018
Edited by:
Hung-Ming Lam, University of Washington, United StatesReviewed by:
Mark Labrecque, University of Washington, United StatesKouji Izumi, Kanazawa University, Japan
Daniele Baiz, Plymouth University, United Kingdom
Copyright: © 2018 Jolly, Kulkarni, Weninger, Orban and Levine. This is an open-access article distributed under the terms of the Creative Commons Attribution License (CC BY). The use, distribution or reproduction in other forums is permitted, provided the original author(s) and the copyright owner are credited and that the original publication in this journal is cited, in accordance with accepted academic practice. No use, distribution or reproduction is permitted which does not comply with these terms.
*Correspondence: Prakash Kulkarni, cGt1bGthcjRAaWJici51bWQuZWR1;
John Orban, am9yYmFuQHVtZC5lZHU=;
Herbert Levine, aGwzNEByaWNlLmVkdQ==
†Present address: Prakash Kulkarni, Department of Medical Oncology and Therapeutics Research, City of Hope National Medical Center, Duarte, CA, United States