Introduction
Polyphenols are the largest family of bioactive compounds in plant foods and represent a significant component of our diet. Evidence from large cohort studies and experimental research consistently indicates beneficial effects of polyphenol intake on cardiometabolic health (1–3). However, randomized controlled trials (RCTs) investigating polyphenol effects on cardiometabolic outcomes often yield mixed or inconclusive results, which can undermine the perceived efficacy of polyphenols in health interventions (4). A primary cause of these inconsistencies appears to be significant interindividual variability in response to polyphenols, distinguishing “responders” from “non-responders” within the same study cohort (5). For instance, a one-year flavonoid intervention study in postmenopausal women demonstrated notable variability in urinary flavonoid excretion rates, categorizing participants as “poor” or “high” excretors (6). This interindividual variability in flavonoid metabolism correlated with differences in insulin response, highlighting that variations in metabolic capacity can significantly impact physiological outcomes. However, other RCTs with different polyphenols revealed that vascular responses (e.g., blood pressure, endothelial function) varied across study populations depending on age and sex, even when bioavailability measures were similar (7–9). This heterogeneity in clinical trials poses challenges for meta-analyses, as seen when wide standard deviations and high statistical heterogeneity obscure the effects in specific populations and hamper to draw consistent conclusions about polyphenols effects (10–12). Interindividual variability in response may stem from differences in ADME processes (absorption, distribution, metabolism, excretion) or from varied responsiveness of cellular and molecular targets critical to the cardiometabolic effects of polyphenols. Key determinants likely include genetic background, age, sex, health status, and gut microbiota composition, but yet their individual contributions and interactions are poorly understood.
Efforts to understand interindividual variability in polyphenol response
The European scientific network COST POSITIVe undertook a systematic analysis to map interindividual variability in polyphenol responses and get insights into why polyphenols are effective in some individuals and less so in others (13). This analysis revealed a significant variability across polyphenol ADME capacities. For most polyphenol classes, this variation appears primarily driven by gut microbiota composition and functionality which play a central role in converting food-derived phenolics into bioactive metabolites (14, 15). POSITIVe highlighted the need for further exploration of other determinants, such as age, sex, and genetic background, where information remains limited. POSITIVe also underscored the value of metabotyping individuals for better predictive power, advocating for standardized approaches, such as using polyphenol supplements for challenge tests and advancing mass spectrometry-based metabolomic profiling (14). The POSITIVe network also conducted meta-analyses of published RCTs to examine variability in cardiometabolic responses to different polyphenol classes, employing subgroup and meta-regression analyses to identify factors (e.g., age, sex, BMI, health status) that may affect the biological response to polyphenol consumption. Although overweight individuals or those with cardiovascular risk factors appeared to respond more consistently, findings were inconsistent across polyphenol types and health outcomes, highlighting the need for further investigation (16).
Strategies for addressing interindividual variability in clinical trials
The current data on potential determinants of interindividual variability in response to polyphenols largely stems from post-hoc analyses, as few trials are designed specifically to capture this variability. Addressing interindividual variability in polyphenol research requires to use a flexible and tailored approach. Broadly, the suitable strategies can be divided into: (1) data-driven methods, which focus on the collection and analysis of rich datasets to characterize individual variability, and (2) enhanced experimental designs, which aim to control for or leverage this variability in clinical trials. Depending on the study's objectives, researchers can apply these strategies individually or in combination.The proposed approaches and their potential contributions to advancing the field are detailed below.
Data-driven methods
A thorough baseline assessment of study participants constitutes the foundation of data driven methods by providing comprehensive insights into individual characteristics such as age, sex, genetics, gut microbiota composition, health status and lifestyle factors. These data help contextualize individual responses and identify potential confounding factors. These baseline data allow for detailed correlation analyses between rich datasets and metabolic profiles or health outcomes, offering more personalized interpretations of response variability.
Building on the baseline assessment, metabotyping offers a practical way to stratify individuals into meaningful subgroups based on their metabolic capacities toward polyphenols. The metabotypes characterized by a simple dichotomy between “producer” vs. “non-producer” of specific polyphenol derived gut metabolites are quite limited (17). The most common situation is the production of all the phenolic metabolites of a catabolic pathway, but in different proportions depending on the individual (18). Accurately capturing the range of possible metabotypes requires standardized methodological workflows. Comprehensive metabolomic profiling, using techniques like mass spectrometry, enables high-resolution assessment of metabolites in biological fluids. However, the development of advanced standardized methodological and statistical tools is essential for delineating the full spectrum of metabotypes. By categorizing participants into diverse metabotypes, researchers gain a nuanced view of individual metabolic responses and can develop more precise predictions of polyphenol efficacy that are requested to move toward personalized nutrition approaches (19).
While metabotyping provides practical groupings of individuals based on metabolic profiles, the integration of omics technologies—such as genomics, epigenomics, transcriptomics, proteomics, metabolomics, and metagenomics—into clinical trials with polyphenols can comprehensively illuminate factors driving interindividual variability and allow to understand how different biological systems interact to produce varying responses to polyphenols. For example, genomics reveals genetic variations impacting polyphenol metabolism, such as polymorphisms in genes encoding conjugative enzymes (e.g., UGT1A1, SULT1A1, COMT) or cell transporters. Epigenomics explores how environmental factors modify responses to polyphenol metabolites. Transcriptomics aids in understanding the influence of polyphenols on cellular pathways, while proteomics identifies specific proteins or enzymes that are over- or under-expressed in individuals. Metabolomics differentiates metabotypes by profiling specific metabolites, while metagenomics characterizes gut bacterial communities responsible for converting polyphenols into bioactive compounds. Machine learning and big data analytics are essential for analyzing these large, complex datasets, identifying response patterns, and creating predictive models of interindividual variability.
In addition to these data-driven methods useful to characterize individual variability some experimental designs can be applied to control and manage this variability effectively in clinical trials.
Experimental designs
Stratified randomization distributes participants based on key variables likely to influence polyphenol metabolism or biological response. To that end, data from baseline assessments or metabotyping can be used to balance key factors across study arms. In particular, this approach could involve stratification by genetic and microbiome profiles, where, participants are grouped based on some genetic polymorphisms and gut microbiota composition. For instance, genetic polymorphisms in phase-2 conjugative enzymes and polyphenol transpoters could influence the profile of circulating metabolites and thereby the bioactivity of polyphenol metabolites, while gut microbiota composition could alter polyphenol metabolism and bioactive metabolite production. Stratifying participants by these characteristics prior to randomization ensures that individuals with distinct metabolic capacities are evenly distributed across study arms. The use of other phenotypic variables such as age, sex, metabolic health, and lifestyle parameters could further refine stratification, enabling more precise exploration of differential responses to polyphenols and clearer identification of responsive subgroups. Stratified randomization minimizes variability and facilitates the identification of specific factors driving interindividual differences.
Used alone or in complement to stratified randomization, crossover designs offer an additional way to reduce variability by allowing participants to serve as their own control. Indeed, a crossover design minimizes baseline discrepancies, such as differences in gut microbiota composition, and clarifies intervention-specific effects. This design is particularly effective for acute or short-term studies, as it reduces the influence of between-subject differences, thereby clarifying intervention-specific effects.
While crossover designs address group-level variability, N-of-1 trials shift the focus to capturing individual responses in a highly personalized manner. Indeed these trials which not consider average responses are valuable for assessing the effects of a specific intervention on each participant. This approach allows for response measurement over time, reducing intra- and interindividual variability as participants undergo multiple intervention and control periods. If N-of-1 trials support a personalized medicine approach, where each intervention is tailored to the individual's unique response profile, they have been yet poorly considered in the field of polyphenols. However, this method can be particularly useful in polyphenol trials as it captures unique response variations that may be masked in group-based designs. Of note, the unique N-of-1 trial conducted with polyphenols used cocoa flavanols and it revealed wide variability in blood pressure responses, with some individuals responding inconsistently or not at all, and also identified the baseline blood pressure as a major determinant of the vascular response (20). Aggregating N-of-1 data across participants with shared genetic or phenotypic characteristics can reveal response clusters and guide more personalized polyphenol interventions.
Finally, adaptive trial designs integrate insights from both data-driven methods and experimental designs, allowing for real-time adjustments to refine study protocols. Of note, adaptive trial designs are increasingly recommended methods to enhance intervention efficacy in clinical nutrition research (21). They allow protocol modifications during the study, based on interim data analyses, without compromising study validity or integrity. This flexibility enhances intervention effectiveness by dynamically adjusting factors -such as participant selection, dosage, outcome measures or study duration- based on real-time data. For instance, adaptations could involve further stratification of participants according to response profiles (responders vs. non-responders) in the study's early stages. This is particularly useful for trials focusing on interindividual variability, as it enables early identification of differing responses and refines interventions to maximize impact on responsive subgroups. Using interim analyses for protocol adjustments ensures that trials can capture the nuances of polyphenol responses and adapt the study design to meet specific research objectives.
Table 1 provides a synthesized overview of the proposed strategies, highlighting their specific contributions to addressing interindividual variability in polyphenol responses. The distinction between data-driven methods and experimental designs highlights the complementary nature of these approaches and how they can be applied flexibly depending on the study objectives and available resources. By combining these approaches as needed, researchers can advance polyphenol research and better address interindividual variability in clinical trials.
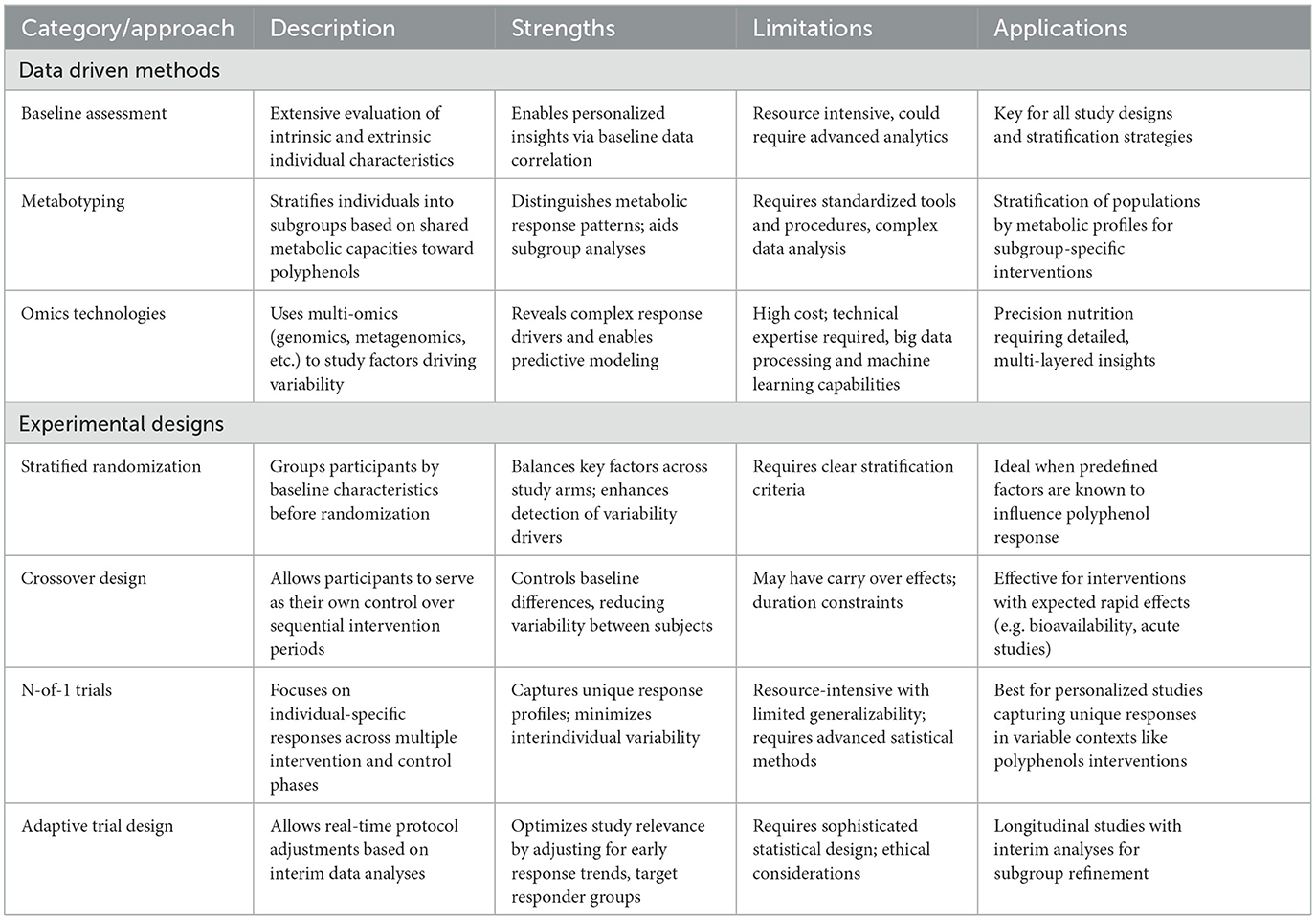
Table 1. Overview of recommended approaches to address interindividual variability in polyphenol response.
Discussion
Interindividual variability in response to polyphenols represents both a challenge and an opportunity in clinical nutrition research. The observed differences in absorption, metabolism, and physiological effects underscore the necessity of transitioning from a one-size-fits-all approach to a more personalized framework. The complementary nature of data-driven methods and experimental designs is key to addressing this variability. While data-driven methods provide the foundation for characterizing variability through baseline assessments, metabotyping, and omics technologies, enhancing experimental designs operationalize these insights to refine study protocols and outcomes. By incorporating advanced study designs, omics technologies and stratification strategies, researchers can both better understand the biological and molecular underpinnings of polyphenol efficacy and manage variability effectively. The use of these strategies will ultimately facilitate more tailored and effective nutritional interventions. Moreover, metabotyping and omics technologies offer unprecedented opportunities to uncover response patterns and stratify populations based on metabolic and biological profiles. By integrating these methods into clinical trials, researchers can identify responsive subgroups and develop tailored interventions that maximize the efficacy of polyphenols for specific populations. Also, the integration of innovative trial designs, such as adaptive and N-of-1 approaches, highlights the potential for real-time data analysis and response-driven adjustments that enhance trial relevance and impact.
Future research should prioritize collaboration across disciplines and foster data sharing, aiming to develop predictive models of polyphenol responses that accommodate individual variability. Leveraging machine learning and big data analytics will be crucial for integrating complex datasets and translating findings into actionable recommendations for public health. Embracing these innovative methodologies will not only refine our understanding of polyphenols' cardiometabolic benefits but also pave the way for the development of precise nutrition strategies that maximize health outcomes for diverse populations. The integration of these methodologies into clinical trial practices could transform the field of precision nutrition, making polyphenol-based interventions more impactful. Such advances will undoubtedly strengthen the evidence base for polyphenol efficacy in public health.
Author contributions
CM: Writing – original draft, Writing – review & editing.
Funding
The author(s) declare that no financial support was received for the research, authorship, and/or publication of this article.
Conflict of interest
The author declares that the research was conducted in the absence of any commercial or financial relationships that could be construed as a potential conflict of interest.
Generative AI statement
The author(s) declare that no Generative AI was used in the creation of this manuscript.
Publisher's note
All claims expressed in this article are solely those of the authors and do not necessarily represent those of their affiliated organizations, or those of the publisher, the editors and the reviewers. Any product that may be evaluated in this article, or claim that may be made by its manufacturer, is not guaranteed or endorsed by the publisher.
References
1. Micek A, Godos J, Del Rio D, Galvano F, Grosso G. Dietary flavonoids and cardiovascular disease: a comprehensive dose-response meta-analysis. Mol Nutr Food Res. (2021) 65:2001019. doi: 10.1002/mnfr.202001019
2. Del Rio D, Rodriguez-Mateos A, Spencer JPE, Tognolini M, Borges G, Crozier A, et al. Dietary (poly)phenolics in human health: structures, bioavailability, and evidence of protective effects against chronic diseases. Antioxid Redox Signal. (2013) 18:1818–92. doi: 10.1089/ars.2012.4581
3. Ruskovska T, Maksimova V, Milenkovic D. Polyphenols in human nutrition: from the in vitro antioxidant capacity to the beneficial effects on cardiometabolic health and related inter-individual variability – an overview and perspective. Br J Nutr. (2020) 123:241–54. doi: 10.1017/S0007114519002733
4. Manach C, Milenkovic D, Van de Wiele T, Rodriguez-Mateos A, de Roos B, Garcia-Conesa MT, et al. Addressing the inter-individual variation in response to consumption of plant food bioactives: towards a better understanding of their role in healthy aging and cardiometabolic risk reduction. Mol Nutr Food Res. (2017) 61:1600557. doi: 10.1002/mnfr.201600557
5. Milenkovic D, Morand C, Cassidy A, Konic-Ristic A, Tomas-Barberan F, Ordovas JM, et al. Interindividual variability in biomarkers of cardiometabolic health after consumption of major plant-food bioactive compounds and the determinants involved. Adv Nutr. (2017) 8:558–570. doi: 10.3945/an.116.013623
6. Cassidy A, Minihane AM. The role of metabolism (and the microbiome) in defining the clinical efficacy of dietary flavonoids. Am J Clin Nutr. (2017) 105:10–22. doi: 10.3945/ajcn.116.136051
7. Barber-Chamoux N, Milenkovic D, Verny MA, Habauzit V, Pereira B, Lambert C, et al. Substantial variability across individuals in the vascular and nutrigenomic response to an acute intake of curcumin: a randomized controlled trial. Mol Nutr Food Res. (2018) 62:1700418. doi: 10.1002/mnfr.201700418
8. Heiss C, Sansone R, Karimi H, Krabbe M, Schuler D, Rodriguez-Mateos A, et al. Impact of cocoa flavanol intake on age-dependent vascular stiffness in healthy men: a randomized, controlled, double-masked trial. Age. (2015) 37:56. doi: 10.1007/s11357-015-9794-9
9. Rodriguez-Mateos A, Cifuentes-Gomez T, Gonzalez-Salvador I, Ottaviani JI, Schroeter H, Kelm M, et al. Influence of age on the absorption, metabolism, and excretion of cocoa flavanols in healthy subjects. Mol Nutr Food Res. (2015) 59:1504–12. doi: 10.1002/mnfr.201500091
10. Yu J, Song P, Perry R, Penfold C, Cooper AR. The effectiveness of green tea or green tea extract on insulin resistance and glycemic control in type 2 diabetes mellitus: a meta-analysis. Diabetes Metab J. (2017) 41:251–62. doi: 10.4093/dmj.2017.41.4.251
11. Martini D, Chiavaroli L, González-Sarrías A, Bresciani L, Palma-Duran SA, Dall'Asta M, et al. Impact of foods and dietary supplements containing hydroxycinnamic acids on cardiometabolic biomarkers: a systematic review to explore inter-individual variability. Nutrients. (2019) 11:1805. doi: 10.3390/nu11081805
12. Li Q, Van de Wiele T. Gut microbiota as a driver of the interindividual variability of cardiometabolic effects from tea polyphenols. Crit Rev Food Sci Nutr. (2023) 63:1500–26. doi: 10.1080/10408398.2021.1965536
13. Morand C, De Roos B, Garcia-Conesa MT, Gibney ER, Landberg R, Manach C, et al. Why interindividual variation in response to consumption of plant food bioactives matters for future personalised nutrition. Proc Nutr Soc. (2020) 79:225–35. doi: 10.1017/S0029665120000014
14. Landberg R, Manach C, Kerckhof FM, Minihane AM, Saleh RNM, De Roos B, et al. Future prospects for dissecting inter-individual variability in the absorption, distribution and elimination of plant bioactives of relevance for cardiometabolic endpoints. Eur J Nutr. (2019) 58:S21–36. doi: 10.1007/s00394-019-02095-1
15. Rowland I, Gibson G, Heinken A, Scott K, Swann J, Thiele I, et al. Gut microbiota functions: metabolism of nutrients and other food components. Eur J Nutr. (2018) 57:1–24. doi: 10.1007/s00394-017-1445-8
16. Gibney ER, Milenkovic D, Combet E, Ruskovska T, Greyling A, González-Sarrías A, et al. Factors influencing the cardiometabolic response to (poly)phenols and phytosterols: a review of the COST action POSITIVe activities. Eur J Nutr. (2019). 58(Suppl 2):S37–47. doi: 10.1007/s00394-019-02066-6
17. Morand C, Tomás-Barberán FA. Interindividual variability in absorption, distribution, metabolism, and excretion of food phytochemicals should be reported. J Agric Food Chem. (2019) 67:3843–4. doi: 10.1021/acs.jafc.9b01175
18. Favari C, Rinaldi de Alvarenga JF, Sánchez-Martínez L, Tosi N, Mignogna C, Cremonini E, et al. Factors driving the inter-individual variability in the metabolism and bioavailability of (poly)phenolic metabolites: a systematic review of human studies. Redox Biol. (2024) 71:103095. doi: 10.1016/j.redox.2024.103095
19. Narduzzi L, Agullo V, Favari C, Tosi N, Mignogna C, Crozier A, et al. (Poly)phenolic compounds and gut microbiome: new opportunities for personalized nutrition. Microbiome Res Rep. (2022) 1:16. doi: 10.20517/mrr.2022.06
20. Bapir M, Campagnolo P, Rodriguez-Mateos A, Skene SS, Heiss C. Assessing variability in vascular response to cocoa with personal devices: a series of double-blind randomized crossover n-of-1 trials. Front Nutr. (2022) 9:886597. doi: 10.3389/fnut.2022.886597
Keywords: polyphenols, interindividual variability, clinical trials, ADME, (cardio)metabolic response, nutrigenomics, gut microbiota, precision nutrition
Citation: Morand C (2024) How to better consider and understand interindividual variability in response to polyphenols in clinical trials. Front. Nutr. 11:1522516. doi: 10.3389/fnut.2024.1522516
Received: 04 November 2024; Accepted: 29 November 2024;
Published: 18 December 2024.
Edited by:
Anandh Babu Pon Velayutham, The University of Utah, United StatesReviewed by:
Raffaella Canali, Council for Agricultural Research and Agricultural Economy Analysis | CREA, ItalyCopyright © 2024 Morand. This is an open-access article distributed under the terms of the Creative Commons Attribution License (CC BY). The use, distribution or reproduction in other forums is permitted, provided the original author(s) and the copyright owner(s) are credited and that the original publication in this journal is cited, in accordance with accepted academic practice. No use, distribution or reproduction is permitted which does not comply with these terms.
*Correspondence: Christine Morand, Y2hyaXN0aW5lLm1vcmFuZEBpbnJhZS5mcg==