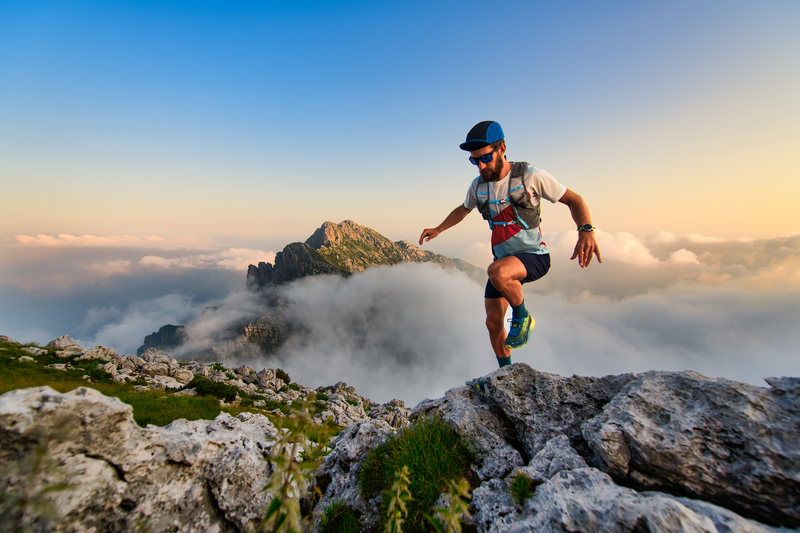
94% of researchers rate our articles as excellent or good
Learn more about the work of our research integrity team to safeguard the quality of each article we publish.
Find out more
ORIGINAL RESEARCH article
Front. Nutr. , 06 December 2024
Sec. Nutritional Epidemiology
Volume 11 - 2024 | https://doi.org/10.3389/fnut.2024.1495792
This article is part of the Research Topic The Role of Foods, Diet, and Dietary Patterns in the Prevention and Management of Diabesity View all 7 articles
Background: Cardiometabolic index (CMI) is a novel marker that can assess metabolic status. Studies have found that people with diabetes mellitus (DM) are at high risk of developing frailty. However, there is a lack of evidence between CMI and the risk of frailty in patients with DM. Therefore, the aim of this study was to investigate the association between CMI and frailty in patients with DM.
Methods: This study utilized data from the 2005-2018 National Health and Nutrition Examination Survey (NHANES). Weighted multivariate logistic regression was conducted in this study to explore the association between CMI and frailty status in patients with DM. In addition, subgroup analyses and interaction analyses were conducted to assess heterogeneity between different subgroups. Subsequently, restricted cubic spline (RCS) was also used to test for non-linear relationships.
Results: This study ultimately included 2,761 patients with DM. Weighted multivariate logistic regression showed that, after adjusting all covariates, an increase in the level of CMI was associated with an increased risk of being in a frailty status in patients with DM (OR = 1.12, 95% CI = 1.04–1.22, p = 0.005). Dividing CMI into tertiles, the risk of frailty in patients in the highest tertile (Q3) was higher than that of patients in Q1 (OR = 1.56, 95% CI = 1.18–2.07, p = 0.002). The non-linear relationship between CMI and the risk of frailty in DM patients was further confirmed by RCS analysis.
Conclusion: This study found that the higher the CMI, the higher the risk of frailty in DM patients. Maintaining a healthy low-fat dietary pattern and properly controlling blood lipid levels may reduce the risk of frailty in patients with DM.
Diabetes mellitus (DM) is a common chronic metabolic disorder, the incidence of which has increased significantly over the past few decades (1, 2). DM is one of the fastest-growing global health problems of the 21st century, imposing a heavy economic burden on society (3, 4). Recently, frailty has attracted much attention in the field of DM (5, 6). Frailty is an emerging public health problem worldwide. The prevalence of frailty in patients with DM is as high as 48%, and the probability of developing frailty is three to five times higher than that in non-DM populations (7). A large-scale meta-analysis conducted by Kong et al. (8) found that the proportions of community-dwelling elderly patients with DM who were in the frail and pre-frail states were 20.1 and 49.1%, respectively (8). Sanz’s team conducted an observational study in several hospitals in Spain to assess malnutrition in elderly patients with DM using the mini nutritional assessment (MNA), and found that the risk of malnutrition reached 39.1% and malnutrition was present in 21.2% of elderly DM patients (9). Also, the team found that malnutrition, albumin and MNA scores were strongly associated with mortality and length of hospitalization. This shows that frailty has become a non-negligible complication of DM. It not only increases the risk of adverse events such as fractures, falls, and hospitalization in patients with DM, but can also substantially increase healthcare expenditure (10). Hanlon et al. (11) suggested that frailty assessment should be incorporated into the routine management of type 2 DM (T2DM) in middle-aged and elderly people.
In 2015, Japanese scholar Wakabayashi proposed the cardiometabolic index (CMI), which is obtained by multiplying the triglycerides (TG)/high-density lipoprotein cholesterol (HDL-C) ratio by waist-to-height ratio (WHtR). CMI can assess the combined effects of body fat distribution and serum lipid levels and is now considered a new method of valuation of visceral adipose tissue (12). CMI has good diagnostic ability for various metabolic disorders including hyperuricaemia (13), liver fibrosis and non-alcoholic fatty liver disease (NAFLD) (14).
Although a non-linear association between CMI and impaired fasting plasma glucose (FPG), insulin resistance and T2DM has been demonstrated (15). However, there is still a lack of information on the association between CMI and the risk of frailty in diabetic patients. CMI is a new type of composite indicator that is convenient, easy to use and cost-effective. Active exploration of the relationship between the two may allow for more effective monitoring of whether diabetic patients are in a frailty state to facilitate effective disease management.
From 2005 to 2018, there were 70,190 participants in the NHANES dataset. Figure 1 illustrates the participant exclusion process with the following criteria: (1) participants who did not meet the diagnostic criteria for DM; (2) age under 20 years; (3) missing complete CMI variables; (4) missing frailty index (FI) variables; (5) participants with missing weight data or weights equal to 0; (6) participants with missing covariates. After excluding these factors, this study ultimately included 2,761 participants who met the criteria.
Referring to American Diabetes Association Guidelines and previous similar studies, a diagnosis of DM may be made if any of the following conditions are present (16): (1) the individual reports having been diagnosed with DM by a physician; (2) the individual is currently taking glucose-lowering medications or taking insulin injections; (3) a random blood glucose reading of 11.1 mmol/L or higher; (4) a glycated hemoglobin (HbA1c) level of 6.5% or higher; (5) a FPG level of 7.0 mmol/L or higher; or (6) a 2-h blood glucose reading of 11.1 mmol/L or higher on an oral glucose tolerance test (OGTT).
The FI was used in order to evaluate the degree of frailty. This approach was originally pioneered by Searle et al. (17). In the present study, we calculate FI following the method constructed by Hakeem et al. (18). The FI is a comprehensive assessment tool covering seven domains and 49 items, including cognition, dependence, depressive symptoms, comorbidities, physical performance and anthropometry, hospital utilization and access to care, and laboratory values. Detailed information can be found in Supplementary Table S1. The severity of each insufficiency was evaluated on a scale ranging from 0 to 1 to determine its level of severity. In this context, 0 denotes the absence of conditions, whereas 1 denotes the most severe. In order to discriminate between debilitating states, we employed a threshold of 0.21. This value refers to a large number of previous studies (19–21). Values that were more than or equal to 0.21 indicated that the individual was experiencing frailty, whereas values that were below this threshold indicated that the individual was not experiencing frailty status.
Cardiometabolic index (CMI) is a new composite index calculated using a combination of HDL-C, TG, waist circumference (WC) and height. The specific calculation is publicized as follows: CMI = [WC (cm)/height (cm)] × (TG/HDL-C) (12).
To investigate the independent association between CMI and risk of frailty in patients with DM, we considered a range of confounding factors that might influence this relationship. Among the demographic and health-related factors were age, gender, race, marital status, education level and family income to poverty ratio (PIR). We classified participants’ race into five categories: Mexican American, Non-Hispanic Black, Non-Hispanic White, other Hispanic, and other race (including multi-racial). Educational level was divided into three categories: below high school, high school, and above high school. Marital status is simply divided into married and other statuses. Smoking status was determined based on self-reported responses. The definitions are as follows: never smoker, former smoker and current smoker. Participants’ total energy intake was obtained through dietary surveys (data from 24-h dietary recall was used in this study). Laboratory test data included serum albumin (Sal).
In the NHANES research, each statistical analysis used sample weights, stratification, and clustering as components (22, 23). To do this, weights are chosen, and the official recommendations for the NHANES suggest that the first step is to determine which variable represents the smallest demographic group, and then to choose the weights that correspond to that variable.
Within this particular context, continuous variables are expressed as weighted mean ± standard error (SE), and a t-test was used to discriminate between the two groups. For the sake of comparison, categorical variables were rendered in the form of numbers and weighted percentages, and chi-square tests were used. In order to classify the CMI, it was divided into tertiles that ranged from the lowest (Q1) to the highest (Q3). In order to investigate the connection between CMI and frailty status, three different weighted logistic regression models were used. Model 1 did not adjust for any covariates. Model 2 adjusted for age, gender, and race. Model 3 was based on Model 2 and adjusted even further for other covariates, including education level, marital status, PIR, smoke, total energy intake and Sal. A trend test was carried out after CMI was transformed from a continuous variable into a tertile group. The restricted cubic spline (RCS) method was used, and possible confounding factors were taken into consideration in order to investigate the possibility of non-linear relationships between CMI and frailty status in diabetic patients. In addition, we calculated P for non-linear. Research was also carried out in the form of subgroup analyses and interaction studies. Statistical significance was determined by using p values less than 0.05.
Finally, we included 2,761 participants. The mean age of these included participants was 59.06 ± 0.36 years, and 1,440 were male (50.07%). The population was divided according to whether they were in a frailty status or not, and comparisons between groups were made, and the demographic data and laboratory test indices of the participants in each group are shown in Table 1. Notably, there were significant differences in age, gender, race, marital status, education level, BMI, PIR, smoking status, TG, WC, height, WHtR, FPG, total energy intake, Sal, CMI, FI, and the presence of hypertension in DM patients in a frailty status compared to those in a non-frailty status (p < 0.05). In the present study, it was found that DM patients in frailty status had higher CMI. They also possessed higher BMI, WC, WHtR and FI, while total energy intake and Sal were significantly lower.
In addition, the baseline characteristics of the population according to the tertiles of CMI are demonstrated in Supplementary Table S2. We found that participants in the highest tertile (Q3) had a higher FI compared to the other two groups.
To assess the association between CMI and frailty status in patients with DM, we used three weighted multivariate logistic regression models (Table 2). In the Methods section, we describe in detail the covariates to be adjusted for in each model. All three models found a positive association with frailty status when CMI was used as a continuous variable. For each unit increase in CMI from model 1 to model 3, the risk of DM patients being in a frailty status increased by 9,14, and 12%, respectively.
Table 2. The association between CMI and frailty status in patients with diabetes mellitus (weighted).
To ensure the accuracy of our results, we performed sensitivity analyses to examine the relationship between tertile-classified CMI and frailty status. We found that CMI still showed a positive association with frailty status when used as a categorical variable. Also, the risk of frailty status would increase with increasing CMI (P for trend < 0.05). In Model 3, diabetic patients in the high CMI group were more likely to be in a frailty status compared to participants in the Q1 CMI groups (OR = 1.56, 95%CI = 1.18–2.07, p = 0.002).
In addition, we conducted multivariate logistic regression analyses with FI as a continuous variable and found that CMI was positively correlated with FI in all three models as well (Supplementary Table S3).
In the RCS analysis, after adjusting for covariates, there was still a significant non-linear relationship between CMI and frailty status (P for non-linear = 0.0076) (Figure 2).
Figure 2. Restricted cubic spline (RCS) regression analyses were used to explore the relationship between CMI and frailty status in patients with DM.
In order to determine whether or not there was a correlation between CMI and frailty status in various subgroups of the population, we conducted subgroup analyses and interaction analyses (Table 3). We found that CMI maintained a positive correlation with frailty status in all subgroups. However, no interaction was observed in all subgroups (P for interaction > 0.05).
Table 3. Subgroup analysis for the association between CMI and frailty status in patients with diabetes mellitus.
It has been noted that frailty has become the third most common complication in DM patients after macrovascular and microvascular lesions (24). The health burden associated with frailty can no longer be ignored. To our knowledge, this is the first cross-sectional study to explore the association between CMI and the risk of frailty in DM patients. In this cross-sectional study, which included 2,761 patients with DM, there was a non-linear positive association between CMI and frailty status. The findings suggest a trend toward a higher risk of frailty in DM patients as CMI increases. This relationship remained unchanged after adjusting for covariates.
We reviewed and summarized previous studies on the relationship between CMI and DM. Wakabayashi et al. proposed that CMI is a new indicator that can reflect both obesity and lipids and can be used for the identification of DM (12). Their team’s study found that HbA1c was significantly higher in the highest tertile CMI group than in other lower tertiles in both male and female. In addition, a strong correlation between CMI and hyperglycaemia and DM was noted. Song et al. (15) found a non-linear positive correlation between CMI and insulin resistance, impaired fasting glucose, and DM, by analyzing data on adults in the United States. Shi et al. (25) analyzed 11,478 participants from a rural area of northeastern China, and found that an increase in CMI was associated with an increase in the chances of developing DM. CMI could be a useful and economical indicator for screening and quantifying DM in the general Chinese population. Zha et al. (26) found that CMI was positively associated with the risk of DM in the Japanese adult population. They also found that CMI interacted with gender, BMI, exercise habits, and smoking status. Using data from the China Health and Retirement Longitudinal Study (CHARLS), the risk of T2DM was shown to be considerably greater in individuals with a high CMI level in the Chinese population of middle-aged and older people than those with low CMI levels, according to the findings of Qiu et al. (27).
In addition to being a dynamic process, the development of frailty is distinguished by the fact that it may be reversed (28). Early screening of individuals who are at a high risk of developing frailty, in conjunction with early intervention, has the potential to successfully prevent the start of frailty as well as its progression. An extensive number of research teams are now engaged in the process of building risk prediction models in order to evaluate the likelihood of frailty developing in diabetic patients. By incorporating characteristics such as marital status, WC, cognitive capacity, grip strength, and social activities, Bu et al. constructed a nomogram model to predict the degree of frailty in patients with DM (29). This model was able to accurately predict the degree of frailty in these patients. There is a substantial correlation between frailty and the prognosis of diabetic patients, since frailty is a prevalent occurrence in diabetic patients. He et al. analyzed data from CHARLS and the English Longitudinal Study of Aging (ELSA) and found that frailty is associated with the development of DM in pre-DM and increases the risk of cardiovascular disease and all-cause mortality in patients with DM in the pre-DM stage and in those with DM (30). Early detection of frailty and timely implementation of targeted interventions may therefore be effective in reducing the burden associated with DM (31).
Notably, CMI is a convenient and feasible marker in clinical practice to help early screening of patients at risk of frailty. CMI is a comprehensive measure of obesity-related disease that integrates indicators of abdominal obesity and dyslipidemia, both of which are key drivers of metabolic disorders. Abdelhafiz and Sinclair (32) found that compared to non-frail patients with DM, those in the frail state tended to have a higher body weight, WC, and BMI, and to be less physically active, have elevated cholesterol elevated cholesterol levels, and also have more comorbidities. An exploratory trial conducted by Simpson et al. (33) found that the rate of aging in DM patients could be slowed and FI levels lowered through weight control. Weight loss through nutritional interventions, physical activity and even the use of medications (e.g., metformin) can improve or even reverse the debilitating state of DM patients (34). On the other hand, abnormal accumulation of lipids leads to the development of an inflammatory response (35). Numerous previous studies have shown that chronic low-grade inflammation is strongly associated with the development of DM and frailty. There is a direct link between frailty and elevated levels of inflammation, marked by elevated C-reactive protein (CRP) and interleukin (IL)-6 (36, 37). Therefore, lipid monitoring and weight management in patients with DM is helpful in reducing the risk of developing frailty.
There are several strengths of our study. To begin, it is the first study to investigate the connection between CMI levels and frailty status in patients with DM. This study offers fresh perspectives on the connection between metabolic features and nutritional status. Second, using the NHANES data, we strictly followed a complex sampling design for weighted analyses to ensure that the results are representative of the wider population. This also greatly increases the generalizability and applicability of the present results. However, in order to reduce the influence of any confounding factors on the findings of the studies, the current research included adjustments for potential variables. This allowed the researchers to shed light on the independent association that exists between CMI and frailty. Because of this, the findings of the present research have significant repercussions for public health policies that aim to recognize frailty status at an early stage and prevent it from occurring in a timely manner.
We should also acknowledge certain limitations of this study. Firstly, the sample for the research was restricted to a particular demographic region, which may restrict the extent to which the results may be generalized to other communities. Therefore, in order to increase the scope of the article’s generalizable application, data from more sources need to be added for analysis in future studies. Secondly, the present study is a cross-sectional study and it is not possible to examine whether the relationship between CMI and frailty is causal. Therefore, the results derived from this study still need to be validated by large-scale prospective studies. Furthermore, while adjusting for a number of covariates, there are still potential common factors that have not been taken into account. Overall, while our study provides valuable insights, these limitations should be taken into account when interpreting the results.
Frailty was much more likely to occur in DM patients who had higher levels of CMI. CMI can be used as a practical tool to identify DM patients who are at risk of frailty. This indicator emphasizes the importance of proper control of lipid levels and body weight in DM patients, and helps guide patients with DM to adhere to a healthy low-fat dietary pattern. At the same time, CMI could help in early screening for frailty risk, thus enabling timely interventions to slow disease progression. Future large-scale prospective studies are needed to validate the findings of this study.
Publicly available datasets were analyzed in this study. This data can be found at: NHANES (https://wwwn.cdc.gov/nchs/nhanes/default.aspx).
NHANES was approved by the Ethical Review Board of the National Centre for Health Statistics and all participants provided written informed consent. The anonymized NHANES data can be downloaded from the NHANES website and analyzed without any permission, which does not require additional ethical review board approval.
YW: Conceptualization, Data curation, Investigation, Methodology, Visualization, Writing – original draft. JY: Investigation, Resources, Supervision, Validation, Writing – review & editing.
The author(s) declare that financial support was received for the research, authorship, and/or publication of this article. This study was supported by grants from Science and Technology Support Program of Jiangsu Province (ZD202208).
The authors declare that the research was conducted in the absence of any commercial or financial relationships that could be construed as a potential conflict of interest.
All claims expressed in this article are solely those of the authors and do not necessarily represent those of their affiliated organizations, or those of the publisher, the editors and the reviewers. Any product that may be evaluated in this article, or claim that may be made by its manufacturer, is not guaranteed or endorsed by the publisher.
The Supplementary material for this article can be found online at: https://www.frontiersin.org/articles/10.3389/fnut.2024.1495792/full#supplementary-material
1. American Diabetes Association . Diagnosis and classification of diabetes mellitus. Diabetes Care. (2011) 34:S62–9. doi: 10.2337/dc11-S062
2. Iminger-Finger, I, Kargul, J, and Laurent, GJ. Diabetes: present and future. Int J Biochem Cell Biol. (2017) 88:196. doi: 10.1016/j.biocel.2017.06.003
3. Zimmet, P, Alberti, KG, Magliano, DJ, and Bennett, PH. Diabetes mellitus statistics on prevalence and mortality: facts and fallacies. Nat Rev Endocrinol. (2016) 12:616–22. doi: 10.1038/nrendo.2016.105
4. Lovic, D, Piperidou, A, Zografou, I, Grassos, H, Pittaras, A, and Manolis, A. The growing epidemic of diabetes mellitus. Curr Vasc Pharmacol. (2020) 18:104–9. doi: 10.2174/1570161117666190405165911
5. Tamura, Y, Omura, T, Toyoshima, K, and Araki, A. Nutrition Management in Older Adults with diabetes: a review on the importance of shifting prevention strategies from metabolic syndrome to frailty. Nutrients. (2020) 12:3367. doi: 10.3390/nu12113367
6. Assar, ME, Laosa, O, and Rodríguez, ML. Diabetes and frailty. Curr Opin Clin Nutr Metab Care. (2019) 22:52–7. doi: 10.1097/MCO.0000000000000535
7. Yanase, T, Yanagita, I, Muta, K, and Nawata, H. Frailty in elderly diabetes patients. Endocr J. (2018) 65:1–11. doi: 10.1507/endocrj.EJ17-0390
8. Kong, LN, Lyu, Q, Yao, HY, Yang, L, and Chen, SZ. The prevalence of frailty among community-dwelling older adults with diabetes: a meta-analysis. Int J Nurs Stud. (2021) 119:103952. doi: 10.1016/j.ijnurstu.2021.103952
9. Sanz París, A, García, JM, Gómez-Candela, C, Burgos, R, Martín, Á, and Matía, P. Malnutrition prevalence in hospitalized elderly diabetic patients. Nutr Hosp. (2013) 28:592–9. doi: 10.3305/nh.2013.28.3.6472
10. Perkisas, S, and Vandewoude, M. Where frailty meets diabetes. Diabetes Metab Res Rev. (2016) 32:261–7. doi: 10.1002/dmrr.2743
11. Hanlon, P, Jani, B, Mair, F, and McAllister, D. Multimorbidity and frailty in middle-aged adults with type 2 diabetes mellitus. Ann Fam Med. (2022) 20:2910. doi: 10.1370/afm.20.s1.2910
12. Wakabayashi, I, and Daimon, T. The “cardiometabolic index” as a new marker determined by adiposity and blood lipids for discrimination of diabetes mellitus. Clin Chim Acta. (2015) 438:274–8. doi: 10.1016/j.cca.2014.08.042
13. Li, Y, and Zeng, L. Comparison of seven anthropometric indexes to predict hypertension plus hyperuricemia among U.S. adults. Front Endocrinol. (2024) 15:1301543. doi: 10.3389/fendo.2024.1301543
14. Yan, L, Hu, X, Wu, S, Cui, C, and Zhao, S. Association between the cardiometabolic index and NAFLD and fibrosis. Sci Rep. (2024) 14:13194. doi: 10.1038/s41598-024-64034-3
15. Song, J, Li, Y, Zhu, J, Liang, J, Xue, S, and Zhu, Z. Non-linear associations of cardiometabolic index with insulin resistance, impaired fasting glucose, and type 2 diabetes among US adults: a cross-sectional study. Front Endocrinol. (2024) 15:1341828. doi: 10.3389/fendo.2024.1341828
16. American Diabetes Association . Standards of medical Care in Diabetes—2013. Diabetes Care. (2013) 36:S11–66. doi: 10.2337/dc13-S011
17. Searle, SD, Mitnitski, A, Gahbauer, EA, Gill, TM, and Rockwood, K. A standard procedure for creating a frailty index. BMC Geriatr. (2008) 8:24. doi: 10.1186/1471-2318-8-24
18. Hakeem, FF, Bernabé, E, and Sabbah, W. Association between Oral health and frailty among American older adults. J Am Med Dir Assoc. (2021) 22:559–63.e2. doi: 10.1016/j.jamda.2020.07.023
19. Zheng, G, Cheng, Y, Wang, C, Wang, B, Zou, X, Zhou, J, et al. Elucidating the causal nexus and immune mediation between frailty and chronic kidney disease: integrative multi-omics analysis. Ren Fail. (2024) 46:2367028. doi: 10.1080/0886022X.2024.2367028
20. Liu, X, Wang, Y, Shen, L, Sun, Y, Zeng, B, Zhu, B, et al. Association between frailty and chronic constipation and chronic diarrhea among American older adults: National Health and nutrition examination survey. BMC Geriatr. (2023) 23:745. doi: 10.1186/s12877-023-04438-4
21. Sun, L, Huo, X, Jia, S, and Chen, X. The association between circadian syndrome and frailty in US adults: a cross-sectional study of NHANES data from 2007 to 2018. Aging Clin Exp Res. (2024) 36:105. doi: 10.1007/s40520-024-02745-3
22. Johnson, CL, Paulose-Ram, R, Ogden, CL, Carroll, MD, Kruszon-Moran, D, Dohrmann, SM, et al. National health and nutrition examination survey: analytic guidelines, 1999-2010. Vital Health Stat. (2013) 161:1–24.
23. Chen, TC, Clark, J, Riddles, MK, Mohadjer, LK, and Fakhouri, THI. National Health and nutrition examination survey, 2015-2018: sample design and estimation procedures. Vital Health Stat 2. (2020) 184:1–35.
24. Ulley, J, and Abdelhafiz, AH. Frailty predicts adverse outcomes in older people with diabetes. Practitioner. (2017) 261:17–20.
25. Shi, WR, Wang, HY, Chen, S, Guo, XF, Li, Z, and Sun, YX. Estimate of prevalent diabetes from cardiometabolic index in general Chinese population: a community-based study. Lipids Health Dis. (2018) 17:236. doi: 10.1186/s12944-018-0886-2
26. Zha, F, Cao, C, Hong, M, Hou, H, Zhang, Q, Tang, B, et al. The nonlinear correlation between the cardiometabolic index and the risk of diabetes: a retrospective Japanese cohort study. Front Endocrinol. (2023) 14:1120277. doi: 10.3389/fendo.2023.1120277
27. Qiu, Y, Yi, Q, Li, S, Sun, W, Ren, Z, Shen, Y, et al. Transition of cardiometabolic status and the risk of type 2 diabetes mellitus among middle-aged and older Chinese: a national cohort study. J Diabetes Investig. (2022) 13:1426–37. doi: 10.1111/jdi.13805
28. Gill, TM, Gahbauer, EA, Allore, HG, and Han, L. Transitions between frailty states among community-living older persons. Arch Intern Med. (2006) 166:418–23. doi: 10.1001/archinte.166.4.418
29. Bu, F, Deng, XH, Zhan, NN, Cheng, H, Wang, ZL, Tang, L, et al. Development and validation of a risk prediction model for frailty in patients with diabetes. BMC Geriatr. (2023) 23:172. doi: 10.1186/s12877-023-03823-3
30. He, D, Li, J, Li, Y, Zhu, J, Zhou, T, Xu, Y, et al. Frailty is associated with the progression of prediabetes to diabetes and elevated risks of cardiovascular disease and all-cause mortality in individuals with prediabetes and diabetes: evidence from two prospective cohorts. Diabetes Res Clin Pract. (2022) 194:110145. doi: 10.1016/j.diabres.2022.110145
31. Cao, X, Li, X, Zhang, J, Sun, X, Yang, G, Zhao, Y, et al. Associations between frailty and the increased risk of adverse outcomes among 38,950 UK biobank participants with prediabetes: prospective cohort study. JMIR Public Health Surveill. (2023) 9:e45502. doi: 10.2196/45502
32. Abdelhafiz, AH, and Sinclair, AJ. Metabolic phenotypes explain the relationship between dysglycaemia and frailty in older people with type 2 diabetes. J Diabetes Complicat. (2022) 36:108144. doi: 10.1016/j.jdiacomp.2022.108144
33. Simpson, FR, Justice, JN, Pilla, SJ, Kritchevsky, SB, Boyko, EJ, Munshi, MN, et al. An examination of whether diabetes control and treatments are associated with change in frailty index across 8 years: an ancillary exploratory study from the action for health in diabetes (look AHEAD) trial. Diabetes Care. (2023) 46:519–25. doi: 10.2337/dc22-1728
34. Guevara, E, Simó-Servat, A, Perea, V, Quirós, C, Puig-Jové, C, Formiga, F, et al. Frailty detection in older adults with diabetes: a scoping review of assessment tools and their link to key clinical outcomes. J Clin Med. (2024) 13:5325. doi: 10.3390/jcm13175325
35. Stout, MB, Justice, JN, Nicklas, BJ, and Kirkland, JL. Physiological aging: links among adipose tissue dysfunction, diabetes, and frailty. Physiology. (2017) 32:9–19. doi: 10.1152/physiol.00012.2016
36. Soysal, P, Stubbs, B, Lucato, P, Luchini, C, Solmi, M, Peluso, R, et al. Inflammation and frailty in the elderly: a systematic review and meta-analysis. Ageing Res Rev. (2016) 31:1–8. doi: 10.1016/j.arr.2016.08.006
Keywords: diabetes mellitus, frailty, NHANES, cross-sectional study, cardiometabolic index
Citation: Wei Y and Yu J (2024) Association between cardiometabolic index and frailty among patients with diabetes mellitus: a cross-sectional study. Front. Nutr. 11:1495792. doi: 10.3389/fnut.2024.1495792
Received: 13 September 2024; Accepted: 13 November 2024;
Published: 06 December 2024.
Edited by:
Luciana Neri Nobre, Universidade Federal dos Vales do Jequitinhonha e Mucuri (UFVJM), BrazilReviewed by:
Jin Zhang, University of Mississippi Medical Center, United StatesCopyright © 2024 Wei and Yu. This is an open-access article distributed under the terms of the Creative Commons Attribution License (CC BY). The use, distribution or reproduction in other forums is permitted, provided the original author(s) and the copyright owner(s) are credited and that the original publication in this journal is cited, in accordance with accepted academic practice. No use, distribution or reproduction is permitted which does not comply with these terms.
*Correspondence: Jiangyi Yu, eWp5MjAyMTA1QG5qdWNtLmVkdS5jbg==
Disclaimer: All claims expressed in this article are solely those of the authors and do not necessarily represent those of their affiliated organizations, or those of the publisher, the editors and the reviewers. Any product that may be evaluated in this article or claim that may be made by its manufacturer is not guaranteed or endorsed by the publisher.
Research integrity at Frontiers
Learn more about the work of our research integrity team to safeguard the quality of each article we publish.