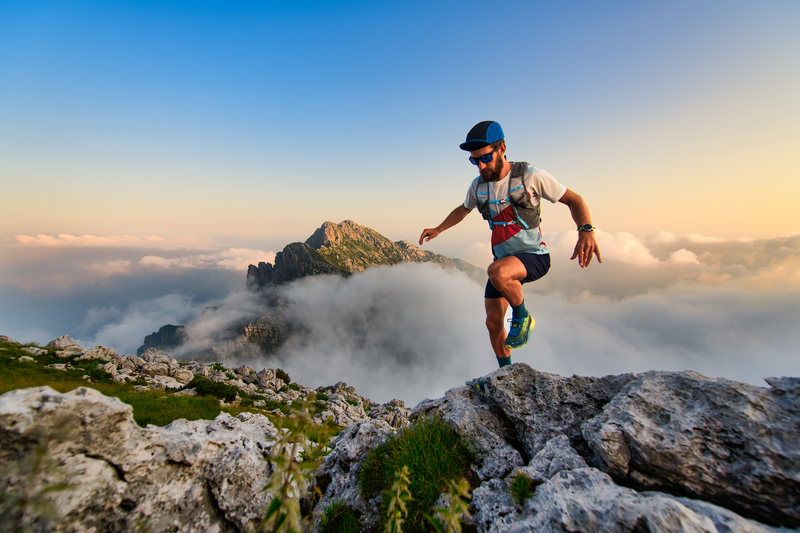
94% of researchers rate our articles as excellent or good
Learn more about the work of our research integrity team to safeguard the quality of each article we publish.
Find out more
ORIGINAL RESEARCH article
Front. Nutr. , 22 October 2024
Sec. Nutrition and Metabolism
Volume 11 - 2024 | https://doi.org/10.3389/fnut.2024.1425317
This article is part of the Research Topic Nutrition and Metabolism in Cancer: Role in Prevention and Prognosis View all 30 articles
Background: Malnutrition often occurs in patients with colorectal cancer. This study aims to develop a predictive model based on GLIM criteria for patients with colorectal cancer who underwent radical surgery.
Methods: From December 2015 to May 2021, patients with colorectal cancer who underwent radical surgery at our center were recruited for this study. We prospectively collected data on GLIM-defined malnutrition and other clinicopathological characteristics. Using Cox regeneration, we developed a novel nomogram for prognostic prediction, which was validated and compared to traditional nutritional factors for predictive accuracy.
Results: Among the 983 patients enrolled in this study, malnutrition was identified in 233 (23.70%) patients. Multivariate analysis indicated that GLIM-defined malnutrition is the independent risk factor for overall survival (HR = 1.793, 95% CI = 1.390–2.313 for moderate malnutrition and HR = 3.485, 95% CI = 2.087–5.818 for severe malnutrition). The novel nomogram based on the GLIM criteria demonstrated a better performance than existing criteria, with AUC of 0.729, 0.703, and 0.683 for 1-year, 3-year, and 5-year OS, respectively, in the validation cohort. In addition, the risk score determined by this system exhibited significantly poorer short-term and long-term clinical outcomes in high-risk groups in both malnourished and well-nourished patients.
Conclusion: Combining handgrip strength, serum albumin level, and TNM stage would help improve the predictive effect of GLIM criteria for colorectal cancer patients post-radical surgery and benefit the individual prognostic prediction of colorectal cancer.
With the third cancer incidence and second cancer-related mortality, colorectal cancer (CRC) remains the rising burden worldwide (1). Prediction and assessment of the clinical outcomes after radical colectomy are still the major concerns of surgeons. Like other severe diseases in the intestinal tract, malnutritional status is often witnessed in patients with CRC. Fasting before or after surgical treatment, hypermetabolism, symptoms of nausea or vomiting, and anemia or obstruction caused by CRC, especially in the advanced stage, are also blamed for malnutrition (2, 3). Thus, malnutrition is also regarded as an indicator of the development of CRC disease.
Malnutrition is now recognized as significantly related to poorer physical health before surgery and has an impact on both the short- and long-term prognosis of cancer patients (4, 5). Recently, the Global Leadership Initiative on Malnutrition (GLIM), consisting of three phenotypic criteria and two etiologic criteria, was published as a global consensus for the diagnosis of malnutrition (2, 6, 7), which has exhibited its advantages for prognostic assessment in gastric cancer in our previous studies (8, 9). For phenotypic criteria of GLIM, reduced muscle mass is assessed by a variety of methods, including computerized tomography (CT) scanning, bioelectrical impedance analysis (BIA), dual-energy X-ray absorptiometry (DXA), and anthropometry, which would correspond to various incidences of reduced muscle mass and GLIM-defined malnutrition (10–12). CT scanning is regarded as the standardized radiological assessment of skeletal muscle mass for its accuracy even in special conditions, such as excess adiposity or edema, and has been reported to evaluate reduced muscle mass for GLIM (10, 13). In addition, skeletal muscle function, while not a surrogate measurement of reduced muscle mass in GLIM, is an important component of sarcopenia to complete nutrition assessment after malnutrition is confirmed (11). However, with the dynamic alternation in nutritional status during the development of CRC disease, making a precise prediction on long-term prognosis based on the nutritional status of each patient remains hard and complicated.
Recent studies have introduced the combination of GLIM-defined malnutrition and other parameters, such as surgical factors, to achieve better predictive effectiveness (9, 14). In the present study, clinicopathological characteristics and data on skeletal muscle quantity and function, including CT-determined skeletal muscle mass and handgrip strength, were collected prospectively. Then, based on GLIM-defined malnutrition and other screened elements related to nutritional status and skeletal muscle function, we aimed to develop a simple but precise tool for prognostic prediction in CRC patients, which may guide nutritional support in clinical practice.
From December 2015 to May 2021, consecutive patients with stages I–III colorectal cancer were recruited in this study based on a prospective cohort. The inclusion criteria were as follows: (1) age ranging from 18 to 80 years old; (2) diagnosis of primary colorectal adenocarcinoma confirmed pathologically, having undergone radical colectomy or proctectomy; and (3) availability of data for risk screening or diagnosis of malnutrition. Exclusion criteria included: (1) confirmed evidence of distant metastasis or receipt of palliative surgery; (2) emergency surgery; and (3) inability to assess skeletal muscle mass and function. Written informed consent forms were obtained from all the patients recruited in this study, and study methodologies were approved by the Ethics Committee of the First Affiliated Hospital of Wenzhou Medical University (KY2023-R055).
The clinical data of participants were collected prospectively, which includes: (1) demographic features, such as gender, age, body mass index (BMI), American Society of Anesthesiologists (ASA) grade, Charlson Comorbidity Index (CCI), history of previous abdominal surgery, concentration of preoperative serum albumin (Alb), and hemoglobin (Hb); (2) tumor-related details, including tumor location and TNM stage; (3) surgical information, such as operative time, number of harvested lymph nodes, and stoma; (4) data for evaluating nutritional status, including weight loss, decreased food intake, and reduced muscle mass determined by skeletal muscle index (SMI) from L3 level of abdominal CT scanning based on our previous research (8) and parameters related to muscle function such as grip strength and gait speed; (5) clinical outcomes, such as postoperative complications (severe complication was defined as grade ≥ 3 according to the Calvien–Dindo scoring system), length of hospital stay, overall survival (OS), and disease-free survival (DFS).
The routine follow-up by telephone interviews or outpatient visits was carried out 1 month after discharge, every 3 months for the first 2 years, and every half year thereafter. The dates of death and recurrence were recorded to determine OS and DFS. The median follow-up time in this study was 3.53 years.
Malnutrition was diagnosed following GLIM criteria via the two-step approach, according to a previous study (9). Briefly, patients were first assessed by Nutritional Risk Screening 2002 (NRS-2002) to identify nutritional risk. Patients with a score of NRS-2002 ≥ 3 proceeded to the next step of malnutritional diagnosis, which consists of three phenotypic criteria (non-volitional weight loss, low BMI, and reduced muscle mass) and two etiologic criteria (reduced food intake or assimilation and disease burden or inflammation). In detail, patients with colorectal cancer met the disease burden based on the etiologic criteria for malnutrition. Low BMI was defined as BMI < 18.5 kg/m2, if age < 70 or BMI < 20 kg/m2, if age > 70, based on the cutoff value for the Asian population (15), and reduced muscle was determined by the SMI as reported in our previous research (9). The patients who met at least one out of the phenotypic criterion and one etiologic criterion were diagnosed with GLIM-defined malnutrition, which was graded according to the cutoff values recommended for the Asian population (BMI < 17.0 kg/m2, if age < 70 years or BMI > 17.8 kg/m2, if age < 70 years) (16).
Quantitative variables were expressed as mean ± standard deviations (SD) and analyzed using Student’s t-test if following a normal distribution, or the data were expressed as medians with interquartile ranges (IQR) and conducted using the Mann–Whitney U-test. Categorical variables were expressed as numbers with proportions (n (%)) and analyzed using the chi-squared or Fisher’s correction if necessary. Kaplan–Meier curves were conducted to describe the difference of OS and DFS between the groups. Clinical characters of the patients were screened through univariate analysis and following multivariate analysis for the factor with p < 0.05 to formulate the nomogram aiming to predict long-term survival. Receiver operating characteristic curve (AUC) and decision curve analysis (DCA) were performed to evaluate the accuracy and discriminative ability of the nomogram model and other models. All the statistical analyses were conducted using SPSS software (version 26.0) and R software (version 4.1.0), with RStudio (version 1.2.5033). A two-tailed p < 0.05 was regarded as a significant difference.
A total of 983 patients with stages I–III colorectal cancer who underwent radical surgery were recruited in this study between December 2015 and May 2021. These patients were then randomly allocated with a ratio of 2:1 to obtain the training (n = 656) and validation cohort (n = 327). The demographic characters and clinical characters are shown in Table 1. In both training and validation cohorts, patients with GLIM-defined malnutrition were significantly older (p < 0.001 and p = 0.004, respectively), had lower BMI (p < 0.001 and p < 0.001, respectively), lower preoperative serum albumin (Alb) (p < 0.001 and p < 0.001, respectively), and hemoglobin (Hb) (p < 0.001 and p < 0.001, respectively) levels. Compared to well-nourished patients, significantly lower SMI (p < 0.001 and p < 0.001, respectively), poorer performance of handgrip strength (p < 0.001 and p < 0.001, respectively), and lower gait speed (p < 0.001 and p < 0.072, respectively) were also found in the malnutrition group in both cohorts.
The percentage of patients who were malnourished and met a single GLIM criterion in each cohort is presented in Supplementary Table 1. Briefly, in the current cohort, 233 (23.70%) patients were identified as having malnutrition based on GLIM criteria, among which 24 patients were graded as having severe malnutrition. Low BMI was identified in 48 (7.32%) patients in the training cohort and 24 (7.34%) patients in the validation cohort. Reduced muscle mass was found in 160 (24.39%) and 79 (24.16%) patients in the training and validation cohort, respectively.
As shown in Table 2, postoperative complications occurred in 29.18% (68/233) of the patients with GLIM-defined malnutrition, and a significantly lower incidence of postoperative complications (22.00%) was found in the non-malnutrition group (p = 0.024). The lower incidence of severe complications (3.20 vs. 5.15%) and shorter postoperative hospital stay (11.20 ± 6.47 days vs. 13.91 ± 6.60 days) were also identified in the non-malnutrition group, but not significantly different. Similar results were also found in the training and validation cohorts. Moreover, stomas tend to be more frequently performed for patients with malnutrition (4.80 vs. 9.44%, p = 0.009). This finding supports the previous studies that malnutrition is one of the risk factors for anastomotic leak, so instead of primary anastomosis, a stoma was performed to lessen the consequences of anastomotic leak and other potential complications (17, 18).
For long-term prognosis, 238 patients died during the follow-up period. We then performed the K–M plot of these patients. The survival curve indicated that the patients with malnutrition demonstrated significantly poorer 5-year OS and 5-year DFS, which are shown in Figure 1, and the survival was even poorer for the patients with severe malnutrition (Supplementary Figure 1). Clinical factors that may affect long-term survival were analyzed using univariate and multivariate Cox regression (Table 3). In multivariate analysis, except for GLIM-defined malnutrition (p < 0.001), age (p = 0.001), TNM stage (p = 0.001), handgrip strength (p = 0.028), and serum albumin level (p = 0.042) were identified as independent prognostic factors for OS in patients who underwent radical colectomy or proctectomy.
Figure 1. Kaplan–Meier curves of 5-year OS (A) and 5-year DFS (B) for the patients with or without GLIM-defined malnutrition.
Based on the factors identified using multivariate Cox regression analysis, clinical factors, including age, serum album level, pathological TNM stage, GLIM-defined nutritional status, and grip strength, were integrated for developing the novel nomogram to predict OS (Figure 2A). A point scale was used to obtain the score of each factor and the probability of OS. The OS was defined by the total score displayed on the total score scale. The nomogram yield AUC values were 0.738 for 1-year OS, 0.681 for 3-year OS, and 0.700 for 5-year OS in the training cohort (Figure 2B), and 0.729 for 1-year OS, 0.703 for 3-year OS, and 0.683 for 5-year OS in the validation cohort, respectively (Figure 2C). The decision curve analysis (DCA) curve of the nomogram in the training and validation cohorts is presented in Figures 2D,E, respectively.
Figure 2. Establishment and validation of predictive system based on identified factors. (A) Nomogram based on age, serum album level, TNM staging, GLIM-defined malnutrition, and handgrip strength (B) AUC for survival prediction in training cohort; (C) AUC for survival prediction in validation cohort; (D) DCA for survival prediction in training cohort; (E) DCA for survival prediction in the validation cohort.
In order to further evaluate the prognostic effect of the novel nomogram system based on nutrition-related factors, we compared its AUC value with those common clinical factors used for screening malnutrition and predicting long-term prognosis for patients with CRC (19–21). Therefore, BMI, Alb, Hb, etc., served as an indicators to predict OS. As shown in Supplementary Figure 2, the AUC values of those predictors mostly ranged from 0.4 to 0.6 for 1-year, 3-year, and 5-year OS in the training or validation cohort, which were much lower than the AUC values of our novel nomogram system.
Based on this predictive nomogram, the risk score of each individual was calculated, and the patients were divided into a low-risk group (risk score < 194.90) and a high-risk group (risk score ≥ 194.90) according to the cutoff value. As demonstrated in Table 4, significantly more patients were diagnosed with malnutrition by GLIM criteria (57.01 vs. 6.48%, p < 0.001) or with nutritional risk by NRS-2002 (64.18 vs. 17.59%, p < 0.001) in the high-risk group. More patients with CCI ≥ 2 were also identified in the high-risk group (23.18 vs. 14.04%). Significantly fewer incidences of total complications (19.44 vs. 31.94%, p < 0.001) and severe complications (2.78 vs. 5.37%, p = 0.040) were found in patients with low risk, which may explain the shorter postoperative hospital stay in the low-risk group (p = 0.002). Moreover, the following analysis indicated favorable predictive effects of this scoring system for 5-year OS (p < 0.001) and 5-DFS (p < 0.001) in subgroups of patients with or without GLIM-defined malnutrition (Figures 3A–D), as well as in patients with the same TNM stage (Supplementary Figure 3). Taken together, these results indicated the favorable prognostic effect in the long-term prognosis of the novel predictive system, which is superior to a single common clinical factor related to nutritional status.
Table 4. Comparison of nutritional status and short-term outcomes of training and validation cohorts.
Figure 3. Kaplan–Meier curves for patients in the low-risk and high-risk groups of different subgroups. 5-year OS (A) and DFS (B) for patients without malnutrition; 5-year OS (C) and DFS (D) for patients without malnutrition.
Malnutrition is highly related to the postoperative complications of patients with gastrointestinal cancer who underwent radical surgery, including anastomotic stoma, and severe wound infection, which would delay postoperative recovery and affect longer-term outcomes (22–24). However, it still turned out to be difficult to predict the clinical prognosis of those patients individually according to their nutritional status (25). In our present study, we introduced a novel nomogram based on GLIM criteria and other factors related to cancer staging and nutritional status. ROC and DCA curves indicated the favorable performance of the nomogram in predicting survival for patients with CRC. In addition, further analysis also demonstrated better predictive effectiveness for prognostic prediction compared to the traditional factors, such as serum Hb level, BMI, and NRS-2002.
Malnutrition can be identified in 16.86–47.60% of the patients with gastrointestinal cancer. In advanced phrases, the incidence can be as high as 70% (4, 26–28). Malnutrition is highly related to adverse postoperative events and survival outcomes. However, single indicators such as BMI and serum prealbumin turned out to be insufficient for diagnosing malnutrition or predicting prognosis accurately under varying circumstances (29–31). In addition, assessments of nutritional status fail to achieve consistency for an individual when evaluated using different criteria (2). This may explain the diverse incidences of malnutrition in the same disease and difficulties in predicting clinical outcomes merely based on nutritional status (28). Therefore, it is vital to develop a more individual system to diagnose malnutrition for prognostic prediction by taking other disease-specific factors into account. For GLIM criteria, the capacity of mortality prediction has been recently demonstrated in patients with gastrointestinal cancer in our previous studies (9, 32). Intriguingly, however, a few researchers attempted to combine GLIM with other clinical factors such as body composition and surgical factors to achieve a more precise prediction or assessment, which indicated the potential for improvements in the GLIM criteria (4, 26). Herein, along with GLIM-defined malnutrition, we developed a novel and simple model for prediction, which also contained the significant prognostic factors selected using the Cox regression. This model demonstrated superiority over traditional criteria for assessing malnutrition in terms of prognostic prediction.
In the current study, among all the clinical factors concerning skeletal muscle quantity and quality, handgrip strength, gait speed, and SMI were the independent risk factors for the 5-year OS according to the univariate analysis, but only handgrip strength was identified using multivariate analysis to develop the predictive model. Indicators for skeletal muscle quantity (SMI), function (handgrip strength), and physical performance (gait speed) are usually used to diagnose diseases of muscular disorder, including sarcopenia and cachexia. These measures are indicative of cancer-related malnutrition due to tumor progression and consumption (33, 34). Gait speed was reported to be associated with disable-free survival in healthy older people (35) and serves as a predictor for poorer survival of patients ≥75 years of age with malignancies, such as blood cancer (36). However, as the increasing burden of early-onset CRC (first diagnosis <50 years old) has been noticed in recent years (1, 37) and the mean age of our cohort is only 63.17 years, gait speed might not be a favorable predictor for the survival of CRC patients. Handgrip strength is regarded as a reliable element to evaluate muscle function and the total skeletal muscle mass, for its simple and non-invasive method and shows a relatively high consistency with the SMI, calculated according to CT scanning to represent the muscle mass of the whole body (38). Recent studies also recommended handgrip strength to be the criterion for malnutrition-related disease in advanced cancers such as cachexia (39, 40). Consistent with these results, handgrip strength was identified by multivariate analysis, with a p-value of 0.012, to establish a predictive model in our study. Considering that GLIM contains only reduced muscle mass but without handgrip strength in phenotypic criteria, our research suggested that combining parameters of muscle function may help to improve the effect of GLIM in predicting prognosis.
For most cases of advanced cancer, cure still remains elusive; optimal nutritional status endows patients with tolerance to aggressive treatments or recurrence after the failure of long-term anticancer treatment (41). Several studies have mentioned that malnourished patients experience worse survival after surgery, which is shown in our results (Figure 1). Moreover, surprisingly, we noticed that although the long-term outcomes are highly related to the preoperative nutritional status, the AUC value of our predictive model based on GLIM decreases by time (0.729, 0.703, and 0.683, respectively, for predicting 1-year, 3-year, and 5-year OS in the validation cohort). It is reasonable to find that malnourished patients experience a higher incidence of postoperative complications (Table 3), which would affect 1-year survival (42). For the prognosis over 1 year, factors concerning postoperative recovery, including proper supportive care, intake of adequate amounts of food, and even additional oral nutritional supplements after surgery, would improve the prognosis and prolong survival (43, 44). Therefore, preoperative nutritional status demonstrated its effectiveness in predicting short- or mid-term outcomes, which would decline gradually over time in predicting long-term survival for CRC patients. Thus, we think it is necessary to evaluate the nutritional status not only before surgery but also to perform regular assessments postoperatively to provide the best supportive or integrated palliative care in time to improve the long-term survival of patients with CRC.
In conclusion, our studies demonstrated that combining handgrip strength and other factors, including serum albumin and TNM stage, would improve the capacity of GLIM in predicting the long-term survival of patients with CRC. A nomogram was also established based on GLIM-defined malnutrition and screened factors as a predictive model. The limitation of this study includes its single-center nature and exclusion of cases with distant metastasis, as only a minority of these patients received radical surgery. Although with the limitations above, the predictive system in the current study exhibited favorable effects for prognostic prediction in both malnutritional and non-malnutritional patients, respectively, which would merit the individual prediction of clinical outcomes of patients with CRC. Further studies concerning regular assessments of nutritional status after surgery should be performed to improve the prediction of CRC patients.
The datasets presented in this article are not readily available because the data used and/or analyzed during the current study are available from the corresponding author on reasonable request. Requests to access the datasets should be directed to YP, MTM1MDY2NDE1MzVAMTM5LmNvbQ==.
The studies involving humans were approved by the Ethics Committee of the First Affiliated Hospital of Wenzhou Medical University (KY2023-R055). The studies were conducted in accordance with the local legislation and institutional requirements. The participants provided their written informed consent to participate in this study.
XY: Writing – original draft, Writing – review & editing, Formal analysis. JZ: Software, Writing – review & editing. JW: Data curation, Formal analysis, Writing – review & editing. YL: Data curation, Formal analysis, Writing – original draft. XY: Investigation, Methodology, Writing – original draft, Formal analysis. XS: Data curation, Formal analysis, Writing – review & editing, Methodology. HJ: Software, Validation, Writing – original draft. ZL: Software, Writing – original draft, Validation. CH: Formal analysis, Software, Writing – review & editing. WZ: Formal analysis, Software, Writing – review & editing. QD: Supervision, Validation, Writing – original draft. WC: Writing – review & editing, Data curation, Methodology. ZY: Supervision, Writing – original draft. YP: Funding acquisition, Supervision, Writing – review & editing. DH: Funding acquisition, Investigation, Resources, Supervision, Writing – review & editing.
The author(s) declare that financial support was received for the research, authorship, and/or publication of this article. This study was funded by the National Natural Science Foundation of China (Nos. 82100951 and 81770884), the Wenzhou Municipal Science and Technology Bureau (No. Y2023487), and the Natural Science Foundation of Zhejiang Province (Nos. LQ21H070003 and LQ24H070008).
The authors declare that the research was conducted in the absence of any commercial or financial relationships that could be construed as a potential conflict of interest.
All claims expressed in this article are solely those of the authors and do not necessarily represent those of their affiliated organizations, or those of the publisher, the editors and the reviewers. Any product that may be evaluated in this article, or claim that may be made by its manufacturer, is not guaranteed or endorsed by the publisher.
The Supplementary material for this article can be found online at: https://www.frontiersin.org/articles/10.3389/fnut.2024.1425317/full#supplementary-material
1. Sung, H, Ferlay, J, Siegel, RL, Laversanne, M, Soerjomataram, I, Jemal, A, et al. Global cancer statistics 2020: GLOBOCAN estimates of incidence and mortality worldwide for 36 cancers in 185 countries. CA Cancer J Clin. (2021) 71:209–49. doi: 10.3322/caac.21660
2. Henriksen, C, Paur, I, Pedersen, A, Kvaerner, AS, Raeder, H, Henriksen, HB, et al. Agreement between GLIM and PG-SGA for diagnosis of malnutrition depends on the screening tool used in GLIM. Clin Nutr. (2022) 41:329–36. doi: 10.1016/j.clnu.2021.12.024
3. Hu, C, Zhang, Q, Jin, X, Zhang, L, Zhang, Y, Zhu, Q, et al. A paradox between preoperative overweight/obesity and change in weight during postoperative chemotherapy and its relationship to survival in stage and colorectal cancer patients. Clin Nutr. (2021) 40:2410–9. doi: 10.1016/j.clnu.2020.10.039
4. Wu, T, Xu, H, Zou, Y, Cui, J, Xu, K, Zhou, M, et al. Its clinical outcome of common cancers Inscoc, mid-arm muscle circumference or body weight-standardized hand grip strength in the GLIM superiorly predicts survival in Chinese colorectal Cancer patients. Nutrients. (2022) 14:166. doi: 10.3390/nu14235166
5. Gillis, C, Richer, L, Fenton, TR, Gramlich, L, Keller, H, Culos-Reed, SN, et al. Colorectal cancer patients with malnutrition suffer poor physical and mental health before surgery. Surgery. (2021) 170:841–7. doi: 10.1016/j.surg.2021.04.003
6. Cederholm, T, Jensen, GL, Correia, M, Gonzalez, MC, Fukushima, R, Higashiguchi, T, et al. GLIM criteria for the diagnosis of malnutrition - a consensus report from the global clinical nutrition community. Clin Nutr. (2019) 38:1–9. doi: 10.1002/jcsm.12383
7. De Groot, LM, Lee, G, Ackerie, A, and van der Meij, BS. Malnutrition screening and assessment in the Cancer care ambulatory setting: mortality predictability and validity of the patient-generated subjective global assessment short form (PG-SGA SF) and the GLIM criteria. Nutrients. (2020) 12:2287. doi: 10.3390/nu12082287
8. Chen, WZ, Yu, DY, Zhang, XZ, Zhang, FM, Zhuang, CL, Dong, QT, et al. Comparison of laparoscopic and open radical gastrectomy for gastric cancer patients with GLIM-defined malnutrition. Eur J Surg Oncol. (2023) 49:376–83. doi: 10.1016/j.ejso.2022.09.009
9. Huang, DD, Wu, GF, Luo, X, Song, HN, Wang, WB, Liu, NX, et al. Value of muscle quality, strength and gait speed in supporting the predictive power of GLIM-defined malnutrition for postoperative outcomes in overweight patients with gastric cancer. Clin Nutr. (2021) 40:4201–8. doi: 10.1016/j.clnu.2021.01.038
10. Correia, M, Tappenden, KA, Malone, A, Prado, CM, Evans, DC, Sauer, AC, et al. Utilization and validation of the global leadership initiative on malnutrition (GLIM): a scoping review. Clin Nutr. (2022) 41:687–97. doi: 10.1016/j.clnu.2022.01.018
11. Barazzoni, R, Jensen, GL, Correia, M, Gonzalez, MC, Higashiguchi, T, Shi, HP, et al. Guidance for assessment of the muscle mass phenotypic criterion for the global leadership initiative on malnutrition (GLIM) diagnosis of malnutrition. Clin Nutr. (2022) 41:1425–33. doi: 10.1016/j.clnu.2022.02.001
12. Kiss, N, Steer, B, De Van Der Schueren, M, Loeliger, J, Alizadehsani, R, Edbrooke, L, et al. Comparison of the prevalence of 21 GLIM phenotypic and etiologic criteria combinations and association with 30-day outcomes in people with cancer: a retrospective observational study. Clin Nutr. (2022) 41:1102–11. doi: 10.1016/j.clnu.2022.03.024
13. Martin, L, Gioulbasanis, I, Senesse, P, and Baracos, VE. Cancer-associated malnutrition and CT-defined sarcopenia and Myosteatosis are endemic in overweight and obese patients. JPEN J Parenter Enteral Nutr. (2020) 44:227–38. doi: 10.1002/jpen.1597
14. Zhang, Q, Zhang, KP, Zhang, X, Tang, M, Song, CH, Cong, MH, et al. Scored-GLIM as an effective tool to assess nutrition status and predict survival in patients with cancer. Clin Nutr. (2021) 40:4225–33. doi: 10.1016/j.clnu.2021.01.033
15. Skeie, E, Tangvik, RJ, Nymo, LS, Harthug, S, Lassen, K, and Viste, A. Weight loss and BMI criteria in GLIM's definition of malnutrition is associated with postoperative complications following abdominal resections - results from a National Quality Registry. Clin Nutr. (2020) 39:1593–9. doi: 10.1016/j.clnu.2019.07.003
16. Maeda, K, Ishida, Y, Nonogaki, T, and Mori, N. Reference body mass index values and the prevalence of malnutrition according to the global leadership initiative on malnutrition criteria. Clin Nutr. (2020) 39:180–4. doi: 10.1016/j.clnu.2019.01.011
17. McDermott, FD, Heeney, A, Kelly, ME, Steele, RJ, Carlson, GL, and Winter, DC. Systematic review of preoperative, intraoperative and postoperative risk factors for colorectal anastomotic leaks. Br J Surg. (2015) 102:462–79. doi: 10.1002/bjs.9697
18. Michońska, I, Polak-Szczybyło, E, Sokal, A, Jarmakiewicz-Czaja, S, Stępień, AE, and Dereń, K. Nutritional issues faced by patients with intestinal stoma: a narrative review. J Clin Med. (2023) 12:510. doi: 10.3390/jcm12020510
19. Jaspan, V, Lin, K, and Popov, V. The impact of anthropometric parameters on colorectal cancer prognosis: a systematic review and meta-analysis. Crit Rev Oncol Hematol. (2021) 159:103232. doi: 10.1016/j.critrevonc.2021.103232
20. Yamamoto, T, Kawada, K, and Obama, K. Inflammation-related biomarkers for the prediction of prognosis in colorectal Cancer patients. Int J Mol Sci. (2021) 22:8002. doi: 10.3390/ijms22158002
21. Dagmura, H, Daldal, E, and Okan, I. The efficacy of hemoglobin, albumin, lymphocytes, and platelets as a prognostic marker for survival in octogenarians and nonagenarians undergoing colorectal Cancer surgery. Cancer Biother Radiopharm. (2022) 37:955–62. doi: 10.1089/cbr.2020.4725
22. Almasaudi, AS, McSorley, ST, Dolan, RD, Edwards, CA, and McMillan, DC. The relation between malnutrition universal screening tool (MUST), computed tomography–derived body composition, systemic inflammation, and clinical outcomes in patients undergoing surgery for colorectal cancer. Am J Clin Nutr. (2019) 110:1327–34. doi: 10.1093/ajcn/nqz230
23. Martínez-Escribano, C, Arteaga Moreno, F, Pérez-López, M, Cunha-Pérez, C, Belenguer-Varea, Á, Cuesta Peredo, D, et al. Malnutrition and increased risk of adverse outcomes in elderly patients undergoing elective colorectal Cancer surgery: a case-control study nested in a cohort. Nutrients. (2022) 14:207. doi: 10.3390/nu14010207
24. McGovern, J, Grayston, A, Coates, D, Leadbitter, S, Hounat, A, Horgan, PG, et al. The relationship between the modified frailty index score (mFI-5), malnutrition, body composition, systemic inflammation and short-term clinical outcomes in patients undergoing surgery for colorectal cancer. BMC Geriatr. (2023) 23:9. doi: 10.1186/s12877-022-03703-2
25. Orell, HK, Pohju, AK, Osterlund, P, Schwab, US, Ravasco, P, and Mäkitie, A. GLIM in diagnosing malnutrition and predicting outcome in ambulatory patients with head and neck cancer. Front Nutr. (2022) 9:1030619. doi: 10.3389/fnut.2022.1030619
26. Zheng, HL, Lin, J, Shen, L-L, Yang, H-B, Xu, B-B, and Xue, Z. The GLIM criteria as an effective tool for survival prediction in gastric cancer patients. Eur J Surg Oncol. (2023) 49:964–73. doi: 10.1016/j.ejso.2023.01.009
27. Álvaro Sanz, E, Garrido Siles, M, Rey Fernández, L, Villatoro Roldán, R, Rueda Domínguez, A, and Abilés, J. Nutritional risk and malnutrition rates at diagnosis of cancer in patients treated in outpatient settings: early intervention protocol. Nutrition. (2019) 57:148–53. doi: 10.1016/j.nut.2018.05.021
28. Wimmer, E, and Glaus, A. Early identification of cancer-related malnutrition in patients with colorectal cancer before and after surgery: a literature review. Support Care Cancer. (2022) 30:8775–83. doi: 10.1007/s00520-022-07230-z
29. Caan, BJ, Cespedes Feliciano, EM, and Kroenke, CH. The importance of body composition in explaining the overweight paradox in Cancer—counterpoint. Cancer Res. (2018) 78:1906–12. doi: 10.1158/0008-5472.CAN-17-3287
30. Bibby, N, and Griffin, O. Nutritional considerations for the management of the older person with hepato-pancreatico-biliary malignancy. Eur J Surg Oncol. (2021) 47:533–8. doi: 10.1016/j.ejso.2020.04.024
31. Miller, J, Wells, L, Nwulu, U, Currow, D, Johnson, MJ, and Skipworth, RJE. Validated screening tools for the assessment of cachexia, sarcopenia, and malnutrition: a systematic review. Am J Clin Nutr. (2018) 108:1196–208. doi: 10.1093/ajcn/nqy244
32. Huang, Y, Chen, Y, Wei, L, Hu, Y, and Huang, L. Comparison of three malnutrition risk screening tools in identifying malnutrition according to global leadership initiative on malnutrition criteria in gastrointestinal cancer. Front Nutr. (2022) 9:959038. doi: 10.3389/fnut.2022.959038
33. Mardas, M, Stelmach-Mardas, M, and Madry, R. Body weight changes in patients undergoing chemotherapy for ovarian cancer influence progression-free and overall survival. Support Care Cancer. (2016) 25:795–800. doi: 10.1007/s00520-016-3462-1
34. Sánchez-Torralvo, FJ, González-Poveda, I, García-Olivares, M, Porras, N, Gonzalo-Marín, M, Tapia, MJ, et al. Poor physical performance is associated with postoperative complications and mortality in preoperative patients with colorectal Cancer. Nutrients. (2022) 14:484. doi: 10.3390/nu14071484
35. Neumann, JT, Thao, LTP, Murray, AM, Callander, E, Carr, PR, Nelson, MR, et al. Prediction of disability-free survival in healthy older people. GeroScience. (2022) 44:1641–55. doi: 10.1007/s11357-022-00547-x
36. Hantel, A, DuMontier, C, Odejide, OO, Luskin, MR, Sperling, AS, Hshieh, T, et al. Gait speed, survival, and recommended treatment intensity in older adults with blood cancer requiring treatment. Cancer. (2020) 127:875–83. doi: 10.1002/cncr.33344
37. Siegel, RL, Wagle, NS, Cercek, A, Smith, RA, and Jemal, A. Colorectal cancer statistics, 2023. CA Cancer J Clin. (2023) 73:233–54. doi: 10.3322/caac.21772
38. Asaishi, K, Matsui, M, Nishikawa, H, Goto, M, Asai, A, Ushiro, K, et al. Grip strength in patients with gastrointestinal diseases. J Clin Med. (2022) 11:2079. doi: 10.3390/jcm11082079
39. Hagens, ERC, Feenstra, ML, van Egmond, MA, van Laarhoven, HWM, Hulshof, M, Boshier, PR, et al. Influence of body composition and muscle strength on outcomes after multimodal oesophageal cancer treatment. J Cachexia Sarcopenia Muscle. (2020) 11:756–67. doi: 10.1002/jcsm.12540
40. Song, M, Zhang, Q, Tang, M, Zhang, X, Ruan, G, Zhang, X, et al. Associations of low hand grip strength with 1 year mortality of cancer cachexia: a multicentre observational study. J Cachexia Sarcopenia Muscle. (2021) 12:1489–500. doi: 10.1002/jcsm.12778
41. Arends, J . Struggling with nutrition in patients with advanced cancer: nutrition and nourishment—focusing on metabolism and supportive care. Ann Oncol. (2018) 29:ii27–34. doi: 10.1093/annonc/mdy093
42. Chok, AY, Zhao, Y, Chen, HLR, Tan, IE, Chew, DHW, Zhao, Y, et al. Elderly patients over 80 years undergoing colorectal cancer resection: development and validation of a predictive nomogram for survival. World J Gastrointest Surg. (2023) 15:892–905. doi: 10.4240/wjgs.v15.i5.892
43. Tan, S, Meng, Q, Jiang, Y, Zhuang, Q, Xi, Q, Xu, J, et al. Impact of oral nutritional supplements in post-discharge patients at nutritional risk following colorectal cancer surgery: a randomised clinical trial. Clin Nutr. (2021) 40:47–53. doi: 10.1016/j.clnu.2020.05.038
Keywords: global leadership initiative on malnutrition, clinical nutrition, colorectal cancer, nomogram, clinical outcomes
Citation: Yan X, Zhu J, Wang J, Lu Y, Ye X, Sun X, Jiang H, Li Z, He C, Zhai W, Dong Q, Chen W, Yu Z, Pan Y and Huang D (2024) Development and validation of a novel prognostic prediction system based on GLIM-defined malnutrition for colorectal cancer patients post-radical surgery. Front. Nutr. 11:1425317. doi: 10.3389/fnut.2024.1425317
Received: 29 April 2024; Accepted: 20 September 2024;
Published: 22 October 2024.
Edited by:
Lucilla Crudele, University of Bari Aldo Moro, ItalyReviewed by:
Salvatore Vaccaro, IRCCS Local Health Authority of Reggio Emilia, ItalyCopyright © 2024 Yan, Zhu, Wang, Lu, Ye, Sun, Jiang, Li, He, Zhai, Dong, Chen, Yu, Pan and Huang. This is an open-access article distributed under the terms of the Creative Commons Attribution License (CC BY). The use, distribution or reproduction in other forums is permitted, provided the original author(s) and the copyright owner(s) are credited and that the original publication in this journal is cited, in accordance with accepted academic practice. No use, distribution or reproduction is permitted which does not comply with these terms.
*Correspondence: Yifei Pan, MTM1MDY2NDE1MzVAMTM5LmNvbQ==; Dongdong Huang, aGRkMDU3N0AxNjMuY29t
†These authors have contributed equally to this work
Disclaimer: All claims expressed in this article are solely those of the authors and do not necessarily represent those of their affiliated organizations, or those of the publisher, the editors and the reviewers. Any product that may be evaluated in this article or claim that may be made by its manufacturer is not guaranteed or endorsed by the publisher.
Research integrity at Frontiers
Learn more about the work of our research integrity team to safeguard the quality of each article we publish.