- 1Department of Clinical Laboratory, Wenzhou People's Hospital, The Third Affiliated Hospital of Shanghai University, The Third Clinical Institute Affiliated to Wenzhou Medical University, Wenzhou, Zhejiang, China
- 2College of Engineering, Boston University, Boston, MA, United States
- 3Cardiac Care Unit, The First Affiliated Hospital of Wenzhou Medical University, Wenzhou, Zhejiang, China
Background: Peripheral arteriosclerosis is caused by any atherosclerosis outside the heart and brain. However, the underlying biological mechanisms are not fully understood. This study aims to explore the causal relationship between blood metabolites and peripheral arteriosclerosis.
Methods: A Mendelian randomization (MR) analysis was implemented to estimate the causality of blood metabolites on peripheral arteriosclerosis. A genome-wide association study (GWAS) of 1,400 metabolites was used as the exposure, whereas two different GWAS datasets of peripheral arteriosclerosis were the outcomes. Inverse-variance weighted (IVW) was the main analysis of causal analysis. MR-Egger, the simple mode, weighted median and weighted mode were used to increase the stability and robustness of the results. Cochran Q test, MR-Egger intercept test, the funnel plot, and MR-Pleiotropy RESidual Sum and Outlier were used for sensitivity analyses. Furthermore, metabolic pathway enrichment analysis was performed using MetaboAnalyst5.0.
Results: In this MR study, eight blood metabolites have a strong causal relationship with peripheral arteriosclerosis, including 1-myristoyl-2-arachidonoyl-GPC (14:0/20:4), 1-palmitoyl-2-arachidonoyl-gpc (16:0/20:4n6), 1-(1-enyl-stearoyl)-2-arachidonoyl-GPE, 1-palmitoyl-2-dihomo-linolenoyl-GPC, Gamma-glutamylleucine, Deoxycholic acid glucuronide and two named X- (X-24546, X-26111). In addition, five important metabolic pathways in peripheral arteriosclerosis were identified through metabolic pathway analysis.
Conclusion: This study provides evidence for the causal relationship between blood metabolites and peripheral arteriosclerosis, and these eight blood metabolites provide new perspectives for screening and prevention of peripheral arteriosclerosis in the future.
Background
Atherosclerosis, which is formed by fibrofatty lesions in the artery walls, is a systemic, progressive inflammatory disease (1). It remains a leading cause of death and morbidity worldwide. When it affects peripheral arteries, it will lead to atherosclerotic renal artery stenosis, multivessel atherosclerotic disease of mesentery, and lower extremity atherosclerosis (2, 3). In recent studies, the degree of systemic inflammation and risk of cardiovascular events in peripheral atherosclerosis are higher than those in coronary atherosclerosis, and the long-term prognosis is poor (4, 5). Age, dyslipidemia, obesity, smoking, high blood pressure and diabetes are considered risk factors for peripheral atherosclerosis (6). However, the prevention measures for peripheral atherosclerosis are still insufficient. Currently, the screening of peripheral atherosclerosis is primarily conducted using ultrasound. However, the accuracy of the results can vary significantly depending on the proficiency of the sonographer. While lower extremity arterial CTA provides more direct and clear diagnostic results, it imposes stricter requirements on the patient’s renal function. Therefore, there is a need for more straightforward indicators to aid in the screening of patients who require intervention.
In recent years, metabolomics has made active contributions to the study of the disease mechanisms. Metabolomics can elucidate biological mechanisms by identifying altered metabolites and metabolic pathways (7). Although several metabolites have been observed to be associated with peripheral arteriosclerosis in population-based cohorts, systematic studies of the causal relationship between blood metabolites and peripheral arteriosclerosis are still unclear (8). In addition, traditional observational studies face challenges in establishing causal relationships due to potential confounding factors.
Recently, Mendelian randomization (MR) analysis is proposed as a new epidemiological tool for studying the causal relationship between disease exposure and clinical outcomes (9). MR uses single nucleotide polymorphisms (SNPs) as an instrumental variable (IV). Also, MR relies on natural random classification of genetic variations during meiosis, resulting in a random distribution of genetic variations. Due to the drawbacks of high cost, long duration, and low feasibility of randomized controlled trials (RCTs), MR can be used as an alternative method to RCTs (10).
Our study aimed to use an MR analysis to fully investigate the causal effect of 1,400 blood metabolites on peripheral arteriosclerosis with pooled data from a genome-wide association study (GWAS).
Methods
Study design
An effective MR study should meet three assumptions: (1) IVs are highly correlated with interest exposure; (2) IVs are not affected by confounding factors; (3) IVs are not related to results and only affects the outcome through exposure (11). All MR Analyses were carried out using Two Sample MR in R software (4.2.1). The flowchart of this study was shown in Figure 1. STROBE-MR checklist was completed to ensure the integrity of MR Studies (Supplementary Table S1).
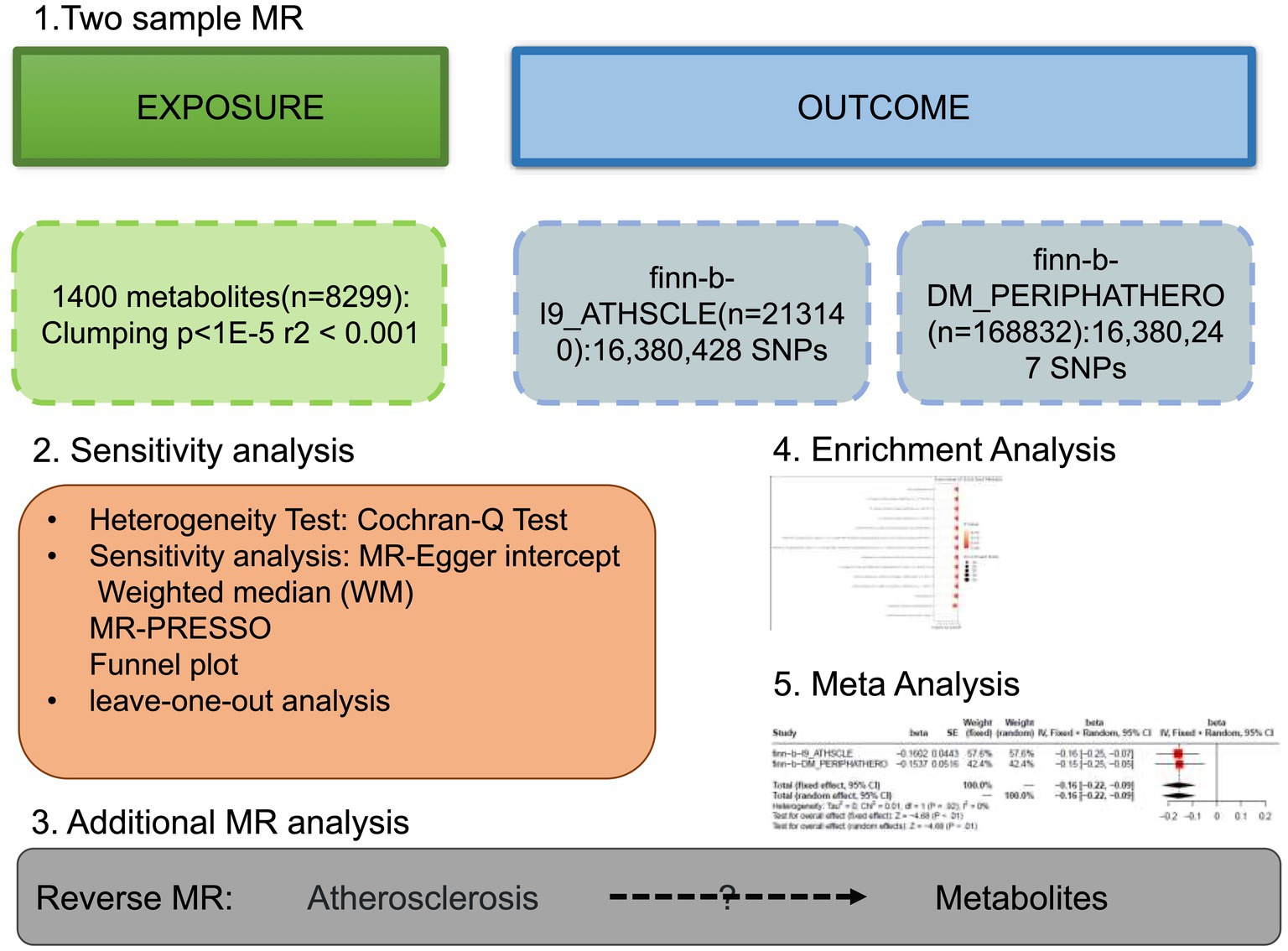
Figure 1. The study design of the study. Detailed information can be found in the main text. MR, Mendelian randomization; SNP, single nucleotide polymorphism.
GWAS data for 1,400 blood metabolites and peripheral arteriosclerosis
The genetic data of blood metabolites came from the metabonomics GWAS catalog server.1 The report was based on Chen et al.’s genome-wide association scanning with high-throughput metabolic analysis, which ultimately identified nearly 15.4 million SNPs of 1,400 metabolites associated with human genetic variation (12, 13). This study included 8,299 unrelated European subjects from the Canadian Longitudinal Study of Aging (CLSA) cohort. The levels of metabolites from plasma samples were quantified using the Ultrahigh Performance Liquid Chromatography-Tandem Mass Spectroscopy (UPLC-MS/MS) platform. After quality control and batch normalization, the quantification of metabolites was used in further research. The detailed names of 1,400 metabolites were shown in Supplementary Table S2, of which 220 were named X-because their chemical properties were not well defined.
GWAS summary data for peripheral arteriosclerosis were obtained from IEU open GWAS project2 with 16,380,428 SNPs which registration number was the finn-b-I9_ATHSCLE, containing 213,140 participants (6,599 cases and 206,541 controls). The finn-b-I9_ATHSCLE dataset, published in 2021, consists of data from the Finnish population. This dataset primarily represents a cohort excluding individuals with lower extremity and cardiovascular atherosclerosis. The replication cohort was also from IEU open GWAS project, with 16,380,247 SNPs. The registration number of the replication cohort was the finn-b-DM_PERIPHATHERO, containing 168,832 participants (6,631 cases and 162,201 controls). The finn-b-DM_PERIPHATHERO dataset, published in 2021 and focuses on a cohort with lower limb atherosclerosis. The above GWAS data for peripheral arteriosclerosis were used for preliminary analysis.
IVs selection
A series of standards were developed to screen for IV related to blood metabolites. Firstly, SNPs were extracted with significance threshold p < 1 × 10−5. Then, identification of independent variants was used R software clustering program. We clumped SNPs with clump_kb set to 10,000 and clump_r2 set to 0.001. Finally, the recommended threshold for further MR analysis was F > 10.
Statistical analysis and sensitivity analysis
The causal associations between blood metabolites and arteriosclerosis were mainly evaluated using inverse variance weighting (IVW) method by combining all Wald ratios of IVs (14). Therefore, we initially used IVW-based estimates (p < 0.05) to screen for blood metabolites that are causally associated with atherosclerosis. Meanwhile, four methods, including MR-Egger, the simple mode (SM), weighted median (WM) and weighted mode (WMO) were also used to evaluate MR estimates of potential blood metabolites. MR-Egger can detect violations of IVs assumptions, and provide estimates of effects that are not affected by these violations (15). WM is used to combine data from multiple genetic variants into a single causal estimator and gives an accurate estimate based on the assumption that at least 50% of IVs are valid (16). SM provides robustness for pleiotropy and WMO is sensitive to the difficult bandwidth selection for mode estimation (17, 18). At least three MR methods had consistent directions and magnitude, these metabolites were considered candidate metabolite markers.
Sevel sensitivity analyses were performed to assess any deviations from the MR assumptions. Cochran Q test, MR-Egger intercept test, the funnel plot, and MR-Pleiotropy RESidual Sum and Outlier (MR-PRESSO) were used to detect and correct heterogeneity and pleiotropy (15, 19). Cochran Q test-derived p < 0.05 was considered to be the heterogeneity of the results (19). MR-Egger was used to identify potential pleiotropy and to assess the impact of pleiotropy on intercept test risk estimates (15). There considered no pleiotropy when p > 0.05. MR - PRESSO was used to check again for the presence of heterogeneous SNPs (20). We used the run_mr_presso() function from the TwoSampleMR R package. The results are evaluated based on two criteria: there should be no outliers detected, and the Global Test p - value must be greater than 0.05. If these criteria are met, it indicates the absence of horizontal pleiotropy. Leave-one-out (LOO) analysis was performed to detect whether the MR estimates were influenced by individual SNPs.
Replication and meta-analysis
To comprehensively assess the robustness of candidate blood metabolites, we replicated the IVW analysis in another independent arteriosclerosis cohort, which registration number was the finn-b-DM_PERIPHATHERO. The replication analysis was using the same methods and sensitivity applied in primary analysis. Then, a meta-analysis of two MR analyses with registration number finn-b-I9_ATHSCLE and finn-b-DM_PERIPHATHERO was conducted to determine the final candidates. Meta-analysis is achieved by metafor R package.
Reverse MR
The following possible scenarios can be used to explain the genetically predicted association between the two phenotypes: (1) a valid causal relationship from phenotype 1 to phenotype 2; (2) a reverse causal relationship from phenotype 2 to phenotype 1; (3) The two phenotypes might share a common genetic structure; (4) There is a linkage disequilibrium (LD) between the major SNPs of the two phenotypes. We performed reverse MR analysis to rule out scenario 2 to assess the causal effect of the arteriosclerosis phenotype on candidate metabolites.
Enrichment analysis
The corresponding ids of potential metabolites were searched and identified in the Human Metabolome Database. To explore the potential metabolite groups or pathways that may be associated with the biological processes of arteriosclerosis, metabolic pathway enrichment analysis was conducted using MetaboAnalyst5.0’s pathway enrichment function modules3 and the p-value is not processed.
Ethical statement
The study used publicly available data. Each study in the GWAS is approved by the appropriate institutional review board, with informed consent provided by the participants or their authorized representatives.
Results
Preliminary analysis
In the GWAS data which the registration number was finn-b-I9_ATHSCLE, 233 metabolites were preliminarily identified (Figure 2A). The criteria to narrow down metabolites are as follows: (1) The directionality must be consistent across all Mendelian randomization methods tested. (2) The p - values for both MR - PRESSO and pleiotropy must be greater than 0.05. (3) The IVW p - value must be less than 0.05. After sensitivity analysis, only 10 of them met the criteria for candidate blood metabolites involved in the development of peripheral atherosclerosis, including 1-arachidonylglycerol (20:4) (OR = 1.171, 95% confidence interval (CI) = 1.074–1.277, p < 0.001), 1-palmitoyl-2-dihomo-linolenoyl-GPC (16:0/20:3n3 or 6) (OR = 0.852, 95% CI = 0.781–0.929, p < 0.001), 1-(1-enyl-stearoyl)-2-arachidonoyl-GPE (p-18:0/20:4) (OR = 1.175, 95% CI = 1.079–1.280, p < 0.001), 1-myristoyl-2-arachidonoyl-GPC (14:0/20:4) (OR = 1.176, 95% CI = 1.088–1.271, p < 0.001), Gamma-glutamylleucine (OR = 0.809, 95% CI = 0.722–0.907, p < 0.001), X-21845 (OR = 0.872, 95% CI = 0.803–0.948, p = 0.001), X-24546 (OR = 0.850, 95% CI = 0.785–0.919, p < 0.001), X-26111 (OR = 0.843, 95% CI = 0.768–0.925, p < 0.001), 1-palmitoyl-2-arachidonoyl-gpc (16:0/20:4n6) (OR = 1.129, 95% CI = 1.072–1.190, p < 0.001), Deoxycholic acid glucuronide (OR = 0.882, 95% CI = 0.816–0.952, p = 0.001) (Table 1). In summary, the estimates derived from the IVW were significant (p < 0.05), while the IVW, WM and WMO estimates showed consistent direction and magnitude, which supported the robustness of causality (Figure 3A). With the results of negative heterogeneous SNPs in MR - PRESSO, our results suggested that no outliers and pleiotropy exist in the previous analysis, indicating that the metabolite used in the next step of research will not cause bias in further analysis and is more robust. MR - PRESSO results suggested there were no heterogeneous SNPs. Besides, the intercept term of MR-Egger shows a lower risk of horizontal pleiotropy and no heterogeneity was detected by Cochran Q test. Furthermore, the LOO analysis results indicated that the results were not affected by a single SNP.
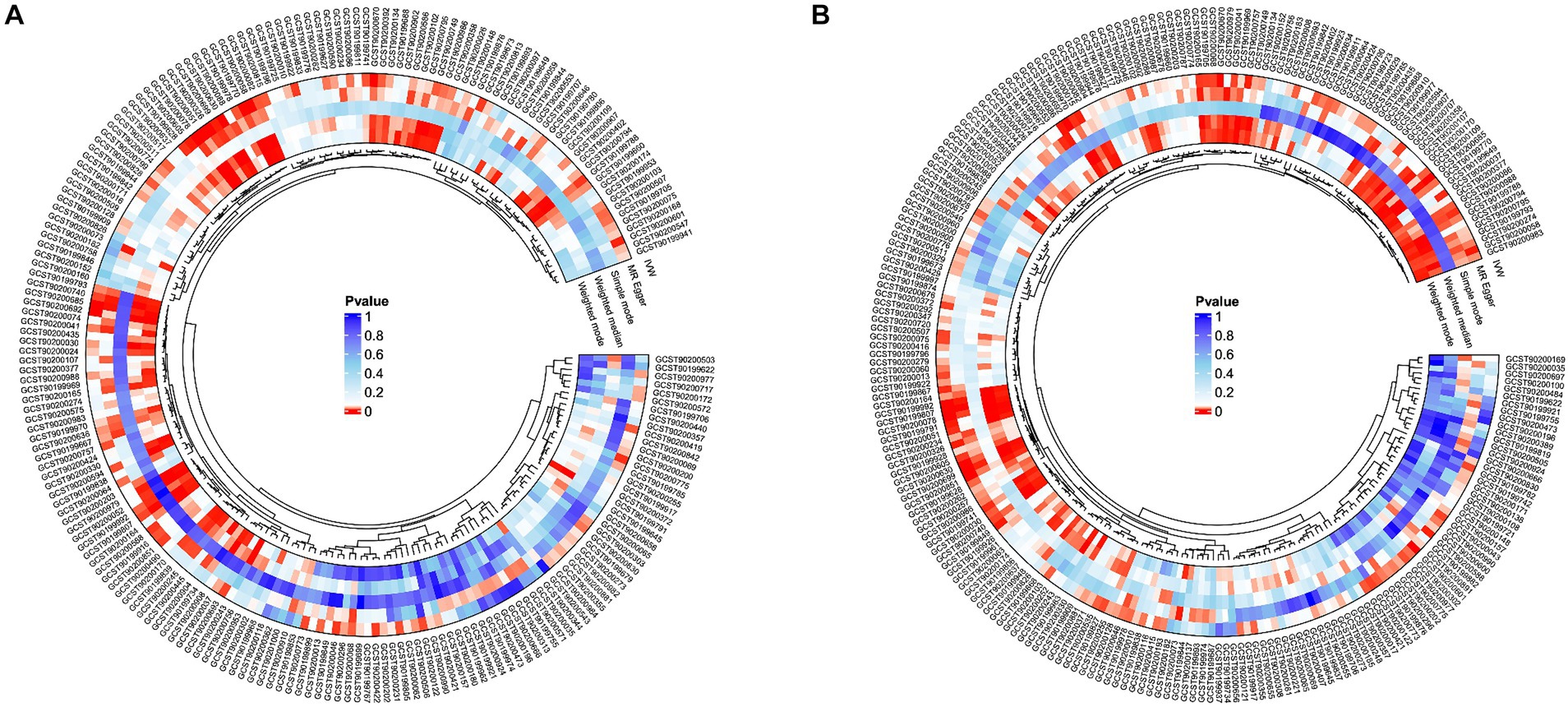
Figure 2. Potential causal metabolites (IVW: p < 0.05) for peripheral arteriosclerosis in the GWAS data. (A) finn-b-I9_ATHSCLE; (B) finn-b-DM_PERIPHATHERO; IVW, inverse variance weighting.
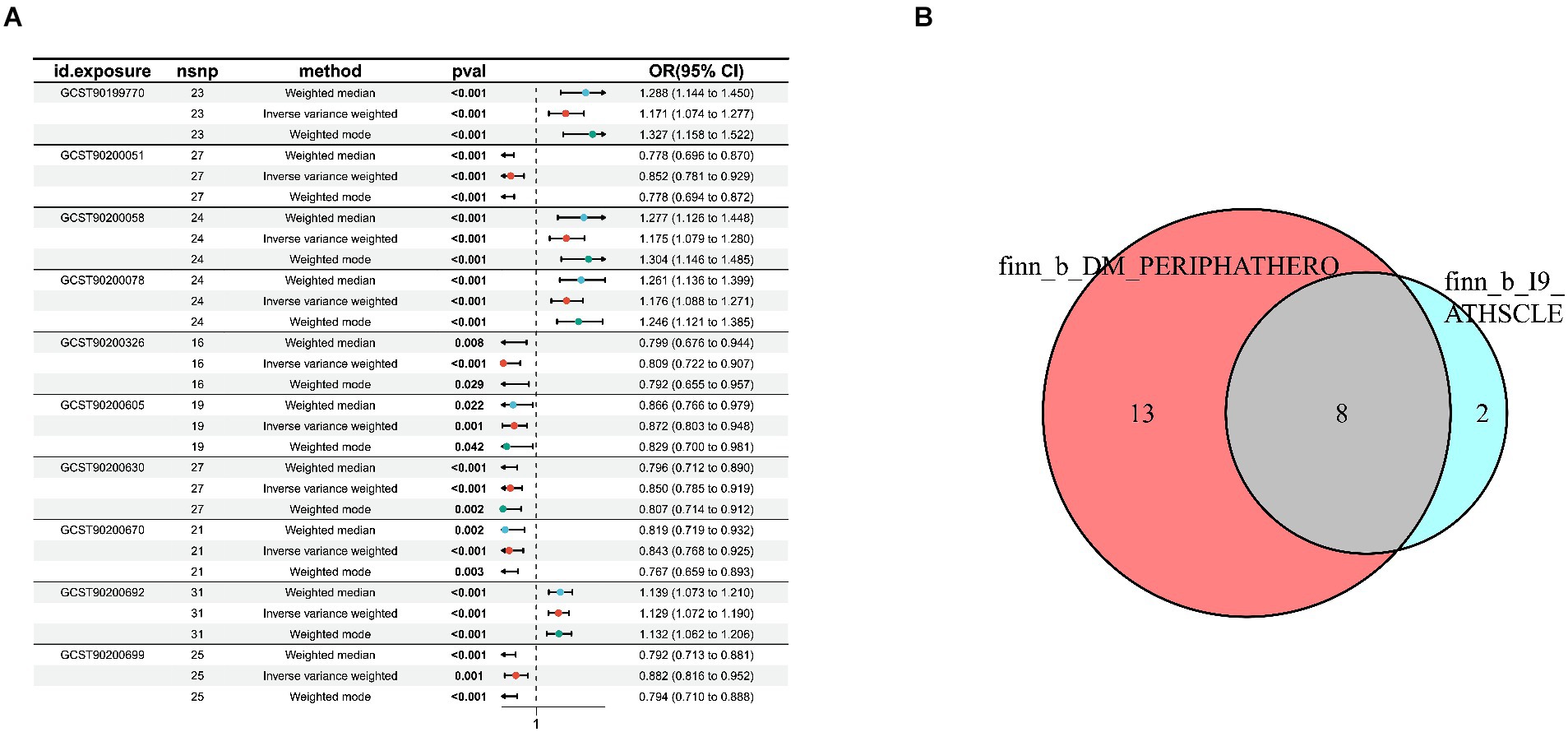
Figure 3. Sensitivity analysis for candidate blood metabolites on peripheral atherosclerosis GWAS databases. (A) finn-b-I9_ATHSCLE; (B) combined analysis of finn-b-I9_ATHSCLE database and finn-b-DM_PERIPHATHERO database.
Replication and meta-analysis
To further enhance the persuasiveness of the estimation, another GWAS data was used for repeated analysis, which registration number finn-b-DM_PERIPHATHERO. There were 206 metabolites significantly associated with peripheral arteriosclerosis (Figure 2B). Through the same sensitivity analysis, there were 21 candidate metabolites that met the criteria (Table 2; Supplementary Figure S1). Combined analysis of the two GWAS datasets, we identified eight candidate metabolites associated with peripheral arteriosclerosis (Figure 3B). Through meta-analysis, we further identified eight candidate blood metabolites (including six known and two unknown) that could influence peripheral arteriosclerosis. In detail, an increase of genetic predisposition of 1-myristoyl-2-arachidonoyl-GPC (14:0/20:4) (OR = 0.16, 95% CI = 0.11–0.22, p < 0.01), 1-palmitoyl-2-arachidonoyl-gpc (16:0/20:4n6) (OR = 0.12, 95% CI = 0.08–0.16, p < 0.01) and 1-(1-enyl-stearoyl)-2-arachidonoyl-GPE (OR = 0.16, 95% CI = 0.09–0.22, p < 0.05) would lead to an increased risk of peripheral arteriosclerosis, while gene susceptibility for higher levels of 1-palmitoyl-2-dihomo-linolenoyl-GPC (OR = −0.16, 95% CI = −0.22– −0.09, p < 0.01), X-24546 (OR = −0.17, 95% CI = −0.23- -0.11, p < 0.01), Gamma-glutamylleucine (OR = −0.22, 95% CI = −0.29- -0.12, p < 0.01), X-26111 (OR = −0.17, 95% CI = - 0.24- -0.11, p < 0.01) and Deoxycholic acid glucuronide (OR = −0.15, 95% CI = −0.20- -0.09, p < 0.01) predicted a lower risk of peripheral arteriosclerosis (Figure 4).
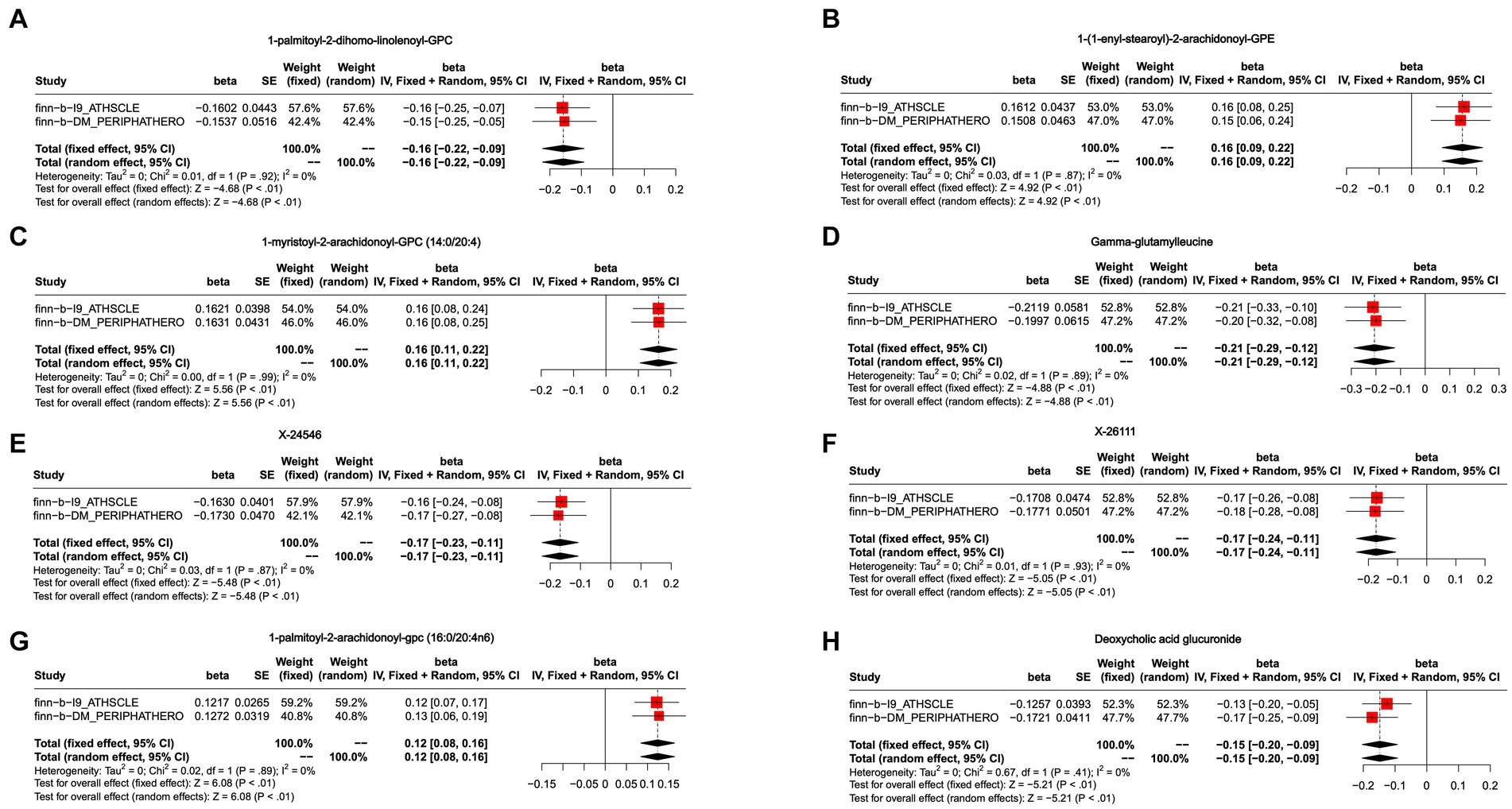
Figure 4. Meta-analysis of the causal associations between blood metabolites and peripheral arteriosclerosis. (A) 1-palmitoyl-2-dihomo-linolenoyl-GPC; (B) 1-(1-enyl-stearoyl)-2-arachidonoyl-GPE; (C) 1-myristoyl-2-arachidonoyl-GPC (14:0/20:4); (D) Gamma-glutamylleucine; (E) X-24546; (F) X-26111; (G) 1-palmitoyl-2-arachidonoyl-gpc (16:0/20:4n6); (H) Deoxycholic acid glucuronide.
Reverse causation between metabolites and peripheral arteriosclerosis
The reverse causal effect of the peripheral arteriosclerosis phenotype on the identified candidate metabolites was also obtained by MR analysis. In the IVW model, none of the peripheral arteriosclerosis phenotypes had a reverse effect on these candidate blood metabolites at the IV threshold of 5e-08.
Metabolic pathway analysis
The metabolic pathway analysis identified five metabolic pathways which may be associated with peripheral arteriosclerosis including Glycerophospholipid metabolism, Linoleic acid metabolism, alpha-Linolenic acid metabolism, Glycosylphosphatidylinositol (GPI)-anchor biosynthesis, Pentose and glucuronate interconversions (p < 0.05, Figure 5). The metabolic mechanism of metabolite formation may be involved in the occurrence and development of peripheral arteriosclerosis.
Discussion
In the present study, the causal relationship between 1,400 blood metabolites and peripheral arteriosclerosis were investigated based on the two large-scale GWAS data and rigorous MR designs. We finally determined that genetically predisposed high levels of 1-myristoyl-2-arachidonoyl-GPC, 1-palmitoyl-2-arachidonoyl-gpc (16:0/20:4n6) and 1-(1-enyl-stearoyl)-2-arachidonoyl-GPE increased risk of peripheral arteriosclerosis, while gene susceptibility for higher levels of 1-palmitoyl-2-dihomo-linolenoyl-GPC, Deoxycholic acid glucuronide, Gamma-glutamylleucine, X-26111 and X-24546 associated with lower risk of peripheral arteriosclerosis. No reverse MR effects of peripheral arteriosclerosis were found on the identified candidate metabolites. In addition, pathway enrichment analysis identified five significant metabolic pathways involved in peripheral arteriosclerosis. As far as we know, this is the first MR study to elucidate the causal relationship between blood metabolites and peripheral arteriosclerosis and incorporate metabolic pathways.
With the aging of the population, the prevalence of peripheral arteriosclerosis will be on the rise (21). Age, high blood pressure, hypercholesterolemia, diabetes, and smoking are recognized risk factors for peripheral arteriosclerosis, but the disease has been developing silently for decades (22). Therefore, one of the major challenges facing clinicians is the early diagnosis of peripheral arteriosclerosis. Although many candidate biomarkers have been proposed, the results have been limited (23, 24). The emergence of new metabolomics techniques provides new ideas for understanding peripheral arteriosclerosis. However, systematic and comprehensive studies on blood metabolomics and peripheral arteriosclerosis are still lacking. Therefore, a critical MR study was conducted to systematically evaluate the causal role of human blood metabolites in the peripheral arteriosclerosis and incorporate metabolic pathways, providing directions for screening and treatment of peripheral arteriosclerosis.
In this study, five blood metabolites were identified to have a protective effect on peripheral arteriosclerosis, including 1-palmitoyl-2-dihomo-linolenoyl-GPC, Gamma-glutamylleucine, Deoxycholic acid glucuronide and two named X- (X-24546, X-26111). However, there are few reports about the related effects of 1-palmitoyl-2-dihomo-linolenoyl-GPC, and the relationship between 1-palmitoyl-2-dihomo-linolenoyl-GPC and peripheral arteriosclerosis deserves further investigation. Glutamylleucine is a dipeptide, a by-product of the synthesis of reduced glutathione catalyzed by gamma-glutamylcysteine synthase (25). Gamma-glutamylleucine has been linked to the risk of cardiometabolic diseases such as obesity, metabolic syndrome, and type 2 diabetes. Meanwhile, the elevated levels of Gamma-glutamylleucine are associated with increased mortality of prostate cancer and play a role in the development of liver-related diseases (26, 27). Gamma-glutamyl dipeptides are involved in a variety of biological activities by activating calcium-sensitive receptors in different organs, including inflammatory activity, oxidative stress, and glucose metabolism. Calcium-sensitive receptors act as G protein-coupled receptors that regulate a variety of cellular processes related to cardiovascular health such as regulating insulin secretion, up-regulating apoptosis and releasing nitric oxide (26). Thus, Gamma-glutamylleucine may have a protective effect in peripheral arteriosclerosis, and its specific biological mechanism remains to be supplemented.
Deoxycholic acid glucuronide binds to the host and participates in the secondary bile acid cycle. Secondary bile acids play an immunomodulatory role by directly affecting the proliferation of immune cells or the production of secondary cytokines (28). Current studies have shown that secondary bile acids are metabolites of gut microbes and are related to cardiovascular health (29). In Diener et al.’s study, Deoxycholic acid glucuronide was shown to be closely associated with the flora, and bile acids can directly drive changes in the gut microbiome (30). This suggests that Deoxycholic acid glucuronide may play a role in peripheral arteriosclerosis by influencing gut microbiome. Recent studies also have found that Deoxycholic acid glucuronide, as a carbohydrate metabolite, is related to the treatment of peripheral artery disease. As a selective serotonin 5-HT2A receptor antagonist, sarpogrelate is excreted mainly in the form of glucuronide conjugate and can be used in the treatment of patients with peripheral artery disease (31). This suggested that Deoxycholic acid glucuronide has great potential in the prevention and treatment of peripheral arteriosclerotic diseases.
We also found that a genetic predisposition to higher levels of 1-myristoyl-2-arachidonoyl-GPC, 1-palmitoyl-2-arachidonoyl-gpc (16:0/20:4n6) and 1-(1-enyl-stearoyl)-2-arachidonoyl-GPE was detrimental to peripheral arteriosclerosis. To date, no association between 1-myristoyl-2-arachidonoyl-GPC and peripheral arteriosclerosis has been reported, with only one study showing that the plasma metabolite 1-myristoyl-2-arachidonoyl-GPC from the microbiota is associated with retained residual beta cell function (32). There are no studies on the relationship between 1-palmitoyl-2-arachidonoyl-gpc (16:0/20:4n6) and peripheral arteriosclerosis. For 1-(1-enyl-stearoyl)-2-arachidonoyl-GPE, Rebholz et al. showed that 1-(1-enyl-stearoyl)-2-arachidonoyl-GPE was significantly correlated with dietary protein intake in patients with chronic kidney disease, which was involved in the metabolism of glycerophospholipid (33). Glycerophospholipid metabolism is the main pathway involved in general systemic immunity and low inflammatory state, indicating that phospholipids are potential inflammatory mediators (34).
In this study, five metabolic pathways were identified that have a causal relationship with peripheral arteriosclerosis, including Glycerophospholipid metabolism, Linoleic acid metabolism, alpha-Linolenic acid metabolism, Glycosylphosphatidylinositol (GPI)-anchor biosynthesis, Pentose and glucuronate interconversions. Glycerophospholipid metabolism has been shown to play a key role in arteriosclerosis (34). Markers of lipid peroxidation damage have been shown to be elevated in patients with cardiovascular disease risk factors. Linoleic acid (LA) is a major dietary omega-6 unsaturated fatty acid, and its metabolite is arachidonic acid (AA). In 30 prospective observational studies in 13 countries, Matti et al. (35) found that the high LA levels were associated with an overall reduced risk of cardiovascular disease, cardiovascular mortality, and ischemic attacks. In randomized controlled feeding trials, dietary polyunsaturated fatty acids (primarily LA) substitutions for carbohydrates or saturated fats reduced low density lipoprotein (LDL) - cholesterol, triglycerides, and ApoB levels, and increased high density lipoprotein (HDL) - cholesterol (36, 37). High blood cholesterol concentration is the main cause of atherosclerosis, and linoleic acid can esterify cholesterol and play a role in reducing serum, liver and blood cholesterol in the body. Meanwhile, conjugated linoleic acid (CLA) is an isomer of linoleic acid. There is evidence that CLA can significantly alter HDL metabolism in vivo and mediate the protective effect of atherosclerosis (38, 39). Pentose and glucuronate interconversions are also disrupted in patients with acute coronary syndromes with anxiety disorders (40).
This study has several advantages. First, the present study covered a large scale of genetic variables to analyze the relationship between blood metabolites and peripheral atherosclerosis. Specifically, 1,400 metabolites were covered. Secondly, another set of GWAS data was used for repeated and isolated analysis, which increased the reliability of the results. Third, reverse MR is adopted to avoid reverse causality. Therefore, the causal relationship between metabolites and peripheral arteriosclerosis in this study is considered reliable.
There are still some limitations in the current study. First, metabolomics profiling was conducted using non-fasting plasma samples. Although the metabolomics measurements were adjusted by accounting for the number of hours since last meal or drink, some additional variability may not have been fully captured. Second, the metabolite data are mainly from individuals of European ancestry, and the generality of our findings to other populations deserves further exploration and verification. Third, the efficacy of IV in MR analysis is heavily reliant on the sample size of GWAS. Therefore, larger datasets are needed to enhance the accuracy of the peripheral arteriosclerosis estimates. Fourth, the accuracy of MR analysis depends on the correct interpretation of exposure by instrumental variables, highlighting the need for larger sample sizes to more precisely assess the genetic influence on metabolites. Finally, although this study identified several metabolites associated with the risk of peripheral arteriosclerosis, further research and experimental validation are necessary to confirm these findings.
Conclusion
In summary, this MR Study identified eight blood metabolites that have a causal effect on peripheral atherosclerosis and identified five metabolic pathways that may be involved in the development of peripheral atherosclerosis. The discovery of these metabolites could be considered as biomarkers for screening and prevention of peripheral arteriosclerosis in the future. The novel contributions of this manuscript include, but are not limited to, our study comprehensively exploring the causal relationship between metabolites and peripheral arteriosclerosis using metabolomic and GWAS data, providing further directions in the development of indicators and a clinical diagnostic panel to aid in the screening of patients who require intervention. We also plan to build a prospective cohort of peripheral arteriosclerosis patients and collect serum samples to clarify the clinical utility of identified metabolites in prediction or diagnosis.
Data availability statement
The data presented in the study were obtained from IEU open GWAS project (https://gwas.mrcieu.ac.uk/) which registration number were the finn-b-I9_ATHSCLE and finn-b- DM_PERIPHATHERO.
Author contributions
QY: Writing – original draft, Writing – review & editing. YZ: Data curation, Writing – original draft. KX: Writing – original draft. ZJ: Writing – original draft, Writing – review & editing.
Funding
The author(s) declare that financial support was received for the research, authorship, and/or publication of this article. This work was supported by Wenzhou Municipal Science and Technology Bureau (No.Y20210004).
Conflict of interest
The authors declare that the research was conducted in the absence of any commercial or financial relationships that could be construed as a potential conflict of interest.
Publisher’s note
All claims expressed in this article are solely those of the authors and do not necessarily represent those of their affiliated organizations, or those of the publisher, the editors and the reviewers. Any product that may be evaluated in this article, or claim that may be made by its manufacturer, is not guaranteed or endorsed by the publisher.
Supplementary material
The Supplementary material for this article can be found online at: https://www.frontiersin.org/articles/10.3389/fnut.2024.1421531/full#supplementary-material
Supplementary Figure S1 | Sensitivity analysis for candidate blood metabolites on peripheral atherosclerosis GWAS databases of finn-b-DM_PERIPHATHER.
Supplementary Table S1 | MR-STROBE checklist.
Supplementary Table S2 | The detailed names of 1400 blood metabolite.
Abbreviations
GWAS, Genome-wide association study; IV, Instrumental variable; IVW, Inverse-variance weighted; LD, Linkage disequilibrium; LOO, Leave-one-out; MR, Mendelian randomization; MR-PRESSO, MR-Pleiotropy RESidual Sum and Outlier; RCTs, Randomized controlled trials; SM, Simple mode; WM, Weighted median; WMO, Weighted mode
Footnotes
References
1. Kowara, M, and Cudnoch-Jedrzejewska, A. Pathophysiology of atherosclerotic plaque development-contemporary experience and new directions in research. Int J Mol Sci. (2021) 22:3513. doi: 10.3390/ijms22073513
2. Libby, P, Buring, JE, Badimon, L, Hansson, GK, Deanfield, J, Bittencourt, MS, et al. Atherosclerosis. Nat Rev Dis Prim. (2019) 5:56. doi: 10.1038/s41572-019-0106-z
3. Nordanstig, J, Behrendt, CA, Bradbury, AW, de Borst, GJ, Fowkes, F, Golledge, J, et al. Peripheral arterial disease (pad) - a challenging manifestation of atherosclerosis. Prev Med. (2023) 171:107489. doi: 10.1016/j.ypmed.2023.107489
4. Cattaneo, M, Wyttenbach, R, Corti, R, Staub, D, and Gallino, A. The growing field of imaging of atherosclerosis in peripheral arteries. Angiology. (2019) 70:20–34. doi: 10.1177/0003319718776122
5. Leiherer, A, Mündlein, A, Brandtner, EM, Säly, CH, Ramadani, H, Vonbank, A, et al. Lipid profiles of patients with manifest coronary versus peripheral atherosclerosis - is there a difference? J Intern Med. (2021) 290:1249–63. doi: 10.1111/joim.13368
6. Libby, P. The changing landscape of atherosclerosis. Nature. (2021) 592:524–33. doi: 10.1038/s41586-021-03392-8
7. Johnson, CH, Ivanisevic, J, and Siuzdak, G. Metabolomics: beyond biomarkers and towards mechanisms. Nat Rev Mol Cell Biol. (2016) 17:451–9. doi: 10.1038/nrm.2016.25
8. Yang, R, Dong, J, Zhao, H, Li, H, Guo, H, Wang, S, et al. Association of Branched-Chain Amino Acids with carotid intima-media thickness and coronary artery disease risk factors. PLoS One. (2014) 9:e99598. doi: 10.1371/journal.pone.0099598
9. Zheng, J, Baird, D, Borges, MC, Bowden, J, Hemani, G, Haycock, P, et al. Recent developments in Mendelian randomization studies. Curr Epidemiol Rep. (2017) 4:330–45. doi: 10.1007/s40471-017-0128-6
10. Richmond, RC, and Davey, SG. Mendelian randomization: concepts and scope. Cold Spring Harb Perspect Med. (2022) 12:a040501. doi: 10.1101/cshperspect.a040501
11. Boef, AG, Dekkers, OM, and le Cessie, S. Mendelian randomization studies: a review of the approaches used and the quality of reporting. Int J Epidemiol. (2015) 44:496–511. doi: 10.1093/ije/dyv071
12. Chen, Y, Lu, T, Pettersson-Kymmer, U, Stewart, ID, Butler-Laporte, G, Nakanishi, T, et al. Genomic atlas of the plasma metabolome prioritizes metabolites implicated in human diseases. Nat Genet. (2023) 55:44–53. doi: 10.1038/s41588-022-01270-1
13. Raina, P, Wolfson, C, Kirkland, S, Griffith, LE, Balion, C, Cossette, B, et al. Cohort profile: the Canadian longitudinal study on aging (Clsa). Int J Epidemiol. (2019) 48:1752–1753j. doi: 10.1093/ije/dyz173
14. Pierce, BL, and Burgess, S. Efficient Design for Mendelian Randomization Studies: subsample and 2-sample instrumental variable estimators. Am J Epidemiol. (2013) 178:1177–84. doi: 10.1093/aje/kwt084
15. Bowden, J, Davey Smith, G, and Burgess, S. Mendelian randomization with invalid instruments: effect estimation and Bias detection through egger regression. Int J Epidemiol. (2015) 44:512–25. doi: 10.1093/ije/dyv080
16. Bowden, J, Davey Smith, G, Haycock, PC, and Burgess, S. Consistent estimation in Mendelian randomization with some invalid instruments using a weighted median estimator. Genet Epidemiol. (2016) 40:304–14. doi: 10.1002/gepi.21965
17. Milne, RL, Kuchenbaecker, KB, Michailidou, K, Beesley, J, Kar, S, Lindström, S, et al. Identification of ten variants associated with risk of estrogen-receptor-negative breast cancer. Nat Genet. (2017) 49:1767–78. doi: 10.1038/ng.3785
18. Hartwig, FP, Davey Smith, G, and Bowden, J. Robust inference in summary data Mendelian randomization via the zero modal pleiotropy assumption. Int J Epidemiol. (2017) 46:1985–98. doi: 10.1093/ije/dyx102
19. Cohen, JF, Chalumeau, M, Cohen, R, Korevaar, DA, Khoshnood, B, and Bossuyt, PM. Cochran's Q test was useful to assess heterogeneity in likelihood ratios in studies of diagnostic accuracy. J Clin Epidemiol. (2015) 68:299–306. doi: 10.1016/j.jclinepi.2014.09.005
20. Verbanck, M, Chen, CY, Neale, B, and Do, R. Detection of widespread horizontal pleiotropy in causal relationships inferred from Mendelian randomization between complex traits and diseases. Nat Genet. (2018) 50:693–8. doi: 10.1038/s41588-018-0099-7
21. Song, P, Rudan, D, Zhu, Y, Fowkes, FJI, Rahimi, K, Fowkes, FGR, et al. Global, regional, and National Prevalence and risk factors for peripheral artery disease in 2015: an updated systematic review and analysis. Lancet Glob Health. (2019) 7:e1020–30. doi: 10.1016/s2214-109x(19)30255-4
22. Hernández-Aguilera, A, Fernández-Arroyo, S, Cabre, N, Luciano-Mateo, F, Baiges-Gaya, G, Fibla, M, et al. Plasma energy-balance metabolites discriminate asymptomatic patients with peripheral artery disease. Mediat Inflamm. (2018) 2018:2760272. doi: 10.1155/2018/2760272
23. Hazarika, S, and Annex, BH. Biomarkers and genetics in peripheral artery disease. Clin Chem. (2017) 63:236–44. doi: 10.1373/clinchem.2016.263798
24. Kim, ES, Wattanakit, K, and Gornik, HL. Using the ankle-brachial index to diagnose peripheral artery disease and assess cardiovascular risk. Cleve Clin J Med. (2012) 79:651–61. doi: 10.3949/ccjm.79a.11154
25. Li, J, Li, J, Wang, H, Qi, LW, Zhu, Y, and Lai, M. Tyrosine and glutamine-leucine are metabolic markers of early-stage colorectal cancers. Gastroenterology. (2019) 157:257–9.e5. doi: 10.1053/j.gastro.2019.03.020
26. Wu, Q, Li, J, Zhu, J, Sun, X, He, D, Li, J, et al. Gamma-Glutamyl-leucine Levels are causally associated with elevated cardio-metabolic risks. Front Nutr. (2022) 9:936220. doi: 10.3389/fnut.2022.936220
27. Huang, J, Zhao, B, Weinstein, SJ, Albanes, D, and Mondul, AM. Metabolomic profile of prostate Cancer-specific survival among 1812 Finnish men. BMC Med. (2022) 20:362. doi: 10.1186/s12916-022-02561-4
28. Zhou, W, Cheng, Y, Zhu, P, Nasser, MI, Zhang, X, and Zhao, M. Implication of gut microbiota in cardiovascular diseases. Oxidative Med Cell Longev. (2020) 2020:5394096. doi: 10.1155/2020/5394096
29. Chiang, JY. Bile acid metabolism and signaling. Compr Physiol. (2013) 3:1191–212. doi: 10.1002/cphy.c120023
30. Diener, C, Dai, CL, Wilmanski, T, Baloni, P, Smith, B, Rappaport, N, et al. Genome-microbiome interplay provides insight into the determinants of the human blood metabolome. Nat Metab. (2022) 4:1560–72. doi: 10.1038/s42255-022-00670-1
31. Kim, HJ, Jeong, ES, Seo, KA, Shin, KJ, Choi, YJ, Lee, SJ, et al. Glucuronidation of a Sarpogrelate active metabolite is mediated by Udp-glucuronosyltransferases 1a4, 1a9, and 2b4. Drug Metab Dispos. (2013) 41:1529–37. doi: 10.1124/dmd.113.051862
32. de Groot, P, Nikolic, T, Pellegrini, S, Sordi, V, Imangaliyev, S, Rampanelli, E, et al. Faecal microbiota transplantation halts progression of human new-onset type 1 diabetes in a randomised controlled trial. Gut. (2021) 70:92–105. doi: 10.1136/gutjnl-2020-322630
33. Rebholz, CM, Zheng, Z, Grams, ME, Appel, LJ, Sarnak, MJ, Inker, LA, et al. Serum metabolites associated with dietary protein intake: results from the modification of diet in renal disease (Mdrd) randomized clinical trial. Am J Clin Nutr. (2019) 109:517–25. doi: 10.1093/ajcn/nqy202
34. Zhu, Q, Wu, Y, Mai, J, Guo, G, Meng, J, Fang, X, et al. Comprehensive metabolic profiling of inflammation indicated key roles of Glycerophospholipid and arginine metabolism in coronary artery disease. Front Immunol. (2022) 13:829425. doi: 10.3389/fimmu.2022.829425
35. Marklund, M, Wu, JHY, Imamura, F, Del Gobbo, LC, Fretts, A, de Goede, J, et al. Biomarkers of dietary Omega-6 fatty acids and incident cardiovascular disease and mortality. Circulation. (2019) 139:2422–36. doi: 10.1161/circulationaha.118.038908
36. Bjermo, H, Iggman, D, Kullberg, J, Dahlman, I, Johansson, L, Persson, L, et al. Effects of N-6 Pufas compared with Sfas on liver fat, lipoproteins, and inflammation in abdominal obesity: a randomized controlled trial. Am J Clin Nutr. (2012) 95:1003–12. doi: 10.3945/ajcn.111.030114
37. Katan, MB, Zock, PL, and Mensink, RP. Effects of fats and fatty acids on blood lipids in humans: an overview. Am J Clin Nutr. (1994) 60:1017s–22s. doi: 10.1093/ajcn/60.6.1017S
38. den Hartigh, LJ. Conjugated linoleic acid effects on Cancer, obesity, and atherosclerosis: a review of pre-clinical and human trials with current perspectives. Nutrients. (2019) 11:370. doi: 10.3390/nu11020370
39. Bruen, R, Curley, S, Kajani, S, Lynch, G, O'Reilly, ME, Dillon, ET, et al. Different monocyte phenotypes result in Proresolving macrophages in conjugated linoleic acid-induced attenuated progression and regression of atherosclerosis. FASEB J. (2019) 33:11006–20. doi: 10.1096/fj.201900922R
Keywords: peripheral arteriosclerosis, Mendelian randomization, blood metabolites, GWAS, causality
Citation: Ye Q, Zhou Y, Xu K and Jiang Z (2024) Causality of blood metabolites and metabolic pathways on peripheral arteriosclerosis: a Mendelian randomization study. Front. Nutr. 11:1421531. doi: 10.3389/fnut.2024.1421531
Edited by:
Anca Pantea Stoian, Carol Davila University of Medicine and Pharmacy, RomaniaReviewed by:
Frances Wang, Johns Hopkins University, United StatesChenguang Zhang, University of Texas Health Science Center at Houston, United States
Copyright © 2024 Ye, Zhou, Xu and Jiang. This is an open-access article distributed under the terms of the Creative Commons Attribution License (CC BY). The use, distribution or reproduction in other forums is permitted, provided the original author(s) and the copyright owner(s) are credited and that the original publication in this journal is cited, in accordance with accepted academic practice. No use, distribution or reproduction is permitted which does not comply with these terms.
*Correspondence: Zhili Jiang, zhilijiangwmu@163.com
†These authors have contributed equally to this work