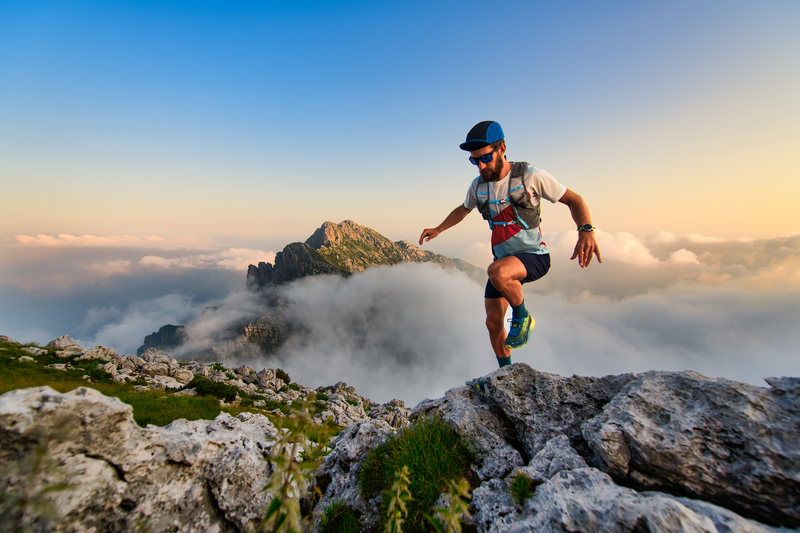
94% of researchers rate our articles as excellent or good
Learn more about the work of our research integrity team to safeguard the quality of each article we publish.
Find out more
REVIEW article
Front. Nutr. , 21 March 2024
Sec. Nutritional Epidemiology
Volume 11 - 2024 | https://doi.org/10.3389/fnut.2024.1379531
The impact of dietary intake on cognitive outcomes and dementia prevention is a topic of increasing interest. Meta-analyses of observational studies, mostly conducted within US and European populations, have reported benefits of healthy diet patterns on cognitive performance, but results from individual studies have been inconsistent. These inconsistencies are likely due to the diverse methodology used in studies, including different diet and cognitive function assessment instruments, follow-up periods, and analytical methods, which make drawing conclusions relevant to dietary guidance challenging. The objective of this project is to describe a protocol to conduct a retrospective harmonization study on dietary intake and cognitive health using data from European and US studies. The recommendations resulting from the project can be used to support evidence-based synthesis for future iterations of the Dietary Guidelines for Americans or other population-based dietary guidance. Additionally, this study will serve as a harmonization guide for future research on the relationship between diet patterns and cognition. The approach outlined ultimately aims to optimize resources and expedite research efforts for dementia prevention.
Over the past few decades, there has been increasing interest in exploring the impact of dietary intake on cognitive outcomes and dementia prevention (1). Numerous high-quality systematic reviews and meta-analyses of observational studies, mostly conducted within US and European populations, have evaluated associations between several diet patterns and cognitive functioning or dementia prevention. Most of these diets emphasize the consumption of vegetables, fruits, and whole grains (2, 3). However, the results were inconsistent for individual studies of cognitive function and diet patterns, including the Mediterranean diet (4, 5), the Dietary Approach to Stop Hypertension (DASH) diet (6, 7), the Mediterranean-DASH diet Intervention for Neurodegenerative Delay (MIND) diet (8, 9), or the anti-inflammatory diet pattern (10).
Despite promising preliminary results of meta-analyzing healthy diet patterns relative to cognitive outcomes, drawing conclusions relevant to dietary guidance remains challenging. The complexity arises from the inherent difficulties in combining and evaluating existing evidence given the heterogeneity in the study populations and the cognitive and dietary assessment methods employed, the different diet patterns and cognitive outcomes examined, and the differential length of the follow-up or timing of outcome assessment across studies (11). Thus far, there has been a lack of standardizing dietary intake and cognitive test data prior to data analysis (12–14). This hinders the interpretation of the study results and, consequently, limits the effectiveness of systematic reviews and meta-analyses in guiding dietary recommendations (15).
These challenges were highlighted by members of the 2020–2025 Dietary Guidelines Advisory Committee (DGAC) comprised of nutrition and public health experts (11). The DGAC assessed the current body of nutrition science, offering independent, science-based counsel to the US Departments of Health and Human Services (HHS) and Agriculture (USDA) during the formulation of the 2020–2025 Dietary Guidelines for Americans (11). However, the DGAC characterized the available evidence about diet patterns and risk of age-related cognitive impairment and/or dementia as limited and were unable to generate a recommendation (11). Recognizing that the issue of methodological disharmony is likely to persist in the 2025–2030 DGAC’s and future such reviews, one potential resolution involves developing a systematic approach to address this longstanding challenge within the literature.
One such approach is to pool individual participant data (IPD) collected in multiple large-scale observational cohort studies and clinical trials (16). IPD meta-analysis is considered the gold standard approach to evidence synthesis (17). Unlike most systematic reviews, it does not rely on aggregate data extracted from journal publications. Rather, the original data on each individual participant are sought from each eligible study. With larger sample sizes resulting from the pooled analysis it might be possible to enhance the statistical power to detect associations not evident in small cohorts. Moreover, analyses are standardized across studies, including variable definitions, statistical methods, and covariates, which reduces variation and potential bias in estimates. However, data cannot be pooled without careful harmonization. IPD harmonization allows integrating information from different studies while ensuring compatibility and inferential equivalence (comparability) across them.
Under the guidance of an Expert Group participating in this project and aligned with previously described guidelines (18–21), we propose to document the procedures for conducting a retrospective harmonization study about dietary intake and cognitive health using data from several European and US studies. Here, we describe our study protocol, including criteria for the selection of studies to answer our study question, define study exposures and outcomes, and describe the process we will take to harmonize these data. In addition, we briefly describe the IPD meta-analytical approach to assess the association of diet pattern intake with cognitive performance using the harmonized datasets.
For the purpose of this paper, we use the following study question to provide direction for harmonizing and meta-analyzing data from appropriate studies: What are the associations of diet pattern intake with cognitive decline or incidence of mild cognitive impairment (MCI)?
IPD harmonization necessitates a rigorous evaluation and documentation of studies participating in the exercise and a meticulous process to harmonize and integrate study-specific data under a common format. The research questions and objectives guide the harmonization procedures (18), which will be conducted as specified in Figure 1.
Figure 1. Schematic study protocol to assess the association between diet patterns and cognitive decline.
Selection of studies to address the study question will be those that meet the following criteria:
1. Data should be accessible, such as datasets available from the BioLINCC data repository that is managed by the National Institutes of Health/National Heart, Lung and Blood Institute in the US and datasets from well-established European repositories (e.g., Dementia Prevention UK data portal). Data should also be representative of different diets and populations across Europe and US.
2. The study design may be a prospective cohort study and/or clinical trial with at least 2 follow-up examinations that include at least one assessment of usual dietary intake at baseline, and more than one measure of cognitive performance collected through validated questionnaires. The reason behind these criteria is that at least two measures of cognitive performance at different points in time are needed to be able to assess the potential impact of a diet pattern on cognitive changes and incidence of MCI. If participants underwent multiple diet assessments at different time points, the dietary data from those assessments will be averaged to generate a single dietary profile for each participant. This averaging process aims to mitigate the effects of random variability and measurement error, providing a more stable representation of participants’ dietary intake. The choice of which assessments to average will be based on various factors, including the timing of assessments, consistency of responses, and validity of measures. Sensitivity analyses will be conducted to assess the robustness of results to different combinations of assessments.
3. The study population includes cognitively healthy adults living in the community (not assisted living or a nursing home) and at least 35 years old at baseline. Study participants with a diagnosis of MCI or dementia at baseline will be excluded. The interaction effect of several comorbidities or lifestyle factors (e.g., cardiovascular function, obesity, depression, diabetes, or physical activity) in the association between diet patterns and cognitive change will be assessed, including sensitivity analyses with these groups. Several models will be developed, including minimally to fully adjusted models, for example, model 1: adjusting for demographic characteristics and energy intake; model 2 adjusting for model 1 plus lifestyle factors; and model 3 adjusting for model 2 plus comorbidities, if the interaction tests were not significant. All the covariates will be harmonized.
4. The study has collected data to harmonize and derive the following variables:
a. The exposure variable will be diet patterns, such as alignment with the Healthy Eating Index (HEI) (22), the EAT-Lancet pattern (23), Mediterranean diet pattern (24)–adapted score for European and US studies (25), and/or the ‘a posteriori’ diet pattern derived by principal components analysis (26). The scores will be derived from daily intakes of food group and nutrient data assessed via food frequency questionnaire (FFQ), Diet History (DH), or multiple 24-h recalls that were interviewer-administered (self-reported dietary intake constitutes an exclusion criteria). The diet assessment should be conducted prior to or simultaneously with the first cognition assessment.
b. The outcome variables include assessments of cognitive performance at a minimum of two time points to measure cognitive change and incidence of MCI as per cut-offs of cognitive screening tests available in the studies (i.e., Mini-mental state examination (MMSE) or Montreal Cognitive Assessment (MoCA). Assessment of cognitive function may include a list of instruments measuring (i) verbal memory, (ii) verbal fluency, and (iii) executive function as specific cognitive domains, which could vary between studies. All cognitive outcomes (MCI, verbal memory, verbal fluency, and executive function) will be assessed separately.
c. Confounding factors known to be related to cognition and/or dietary intake will be included (11, 19, 27). In statistical models, variables considered by the DGAC will be included: age, ethnicity, education, socioeconomic status, smoking, physical activity, anthropometry, and alcohol intake (27). Moreover, an a priori larger list of confounders reported in the literature will be considered, such as blood pressure, lipids, glucose, medication use, and others (28). The final confounder list will include, at the very minimum, the DGAC confounder list.
d. All analyses will be stratified by sex.
Our final exposure variables will be diet patterns. To create them, the dietary data will be harmonized into (i) comparable food and beverage groups, such as fruit, vegetables, dairy, meat, sugar sweetened beverages, candy, etc., (ii) comparable units of measure, including servings/day and/or grams/day (29, 30). As a third and last step, diet patterns will be created with the harmonized data. These steps are explained in more detail below.
In order to harmonize dietary information into comparable food and beverage groups, information from DH, multiple 24-h recalls and FFQ will be used. Food subgroups obtained from a DH and multiple 24-h recalls are typically similar and more flexible to combine into a larger food subgroup than food categories listed on a FFQ. Therefore, the DH and 24-h recall food groups will be tailored to those of the FFQ. Some food categories listed on a FFQ include mixed dishes, such as pizza, fast food sandwiches, chicken, vegetable, and rice mixed dish (or casserole). These foods must be disassembled into the component parts. For example, a cheese pizza consists of refined grain crust, tomato sauce, and cheese. The component parts will be assigned to the respective food subgroup. Another example is where cheese, meat, and vegetable pizza are included in one food category; therefore, components for cheese, meat, and vegetables will be included in one recipe and components assigned to the respective food subgroup. Finally, major food groups will also be created by summing similar food subgroups.
The nutrients reported in diet studies are usually similar, however, units may differ. For example, energy intake may be reported as kilocalories or kilojoules; thus, it is necessary to convert one unit to the other. In addition, the output data from a diet assessment include servings or grams from each food group or food category consumed as well as nutrient intake. In the US, serving sizes for foods and beverages are standard, being based on USDA information (31). In Europe, portion sizes are reported in grams/day for each major food group.
Finally, diet patterns will be created using the harmonized dietary data. The final exposure variables for our study question are healthy diet patterns; for example, the HEI-2020, a score that represents diet quality (22). Food groups in servings/day as well as added sugars (grams/day) are components required for this score. Mediterranean diet pattern will also be assessed, and validated scores will be selected depending on the origin of the included studies (25). Another potential diet pattern of interest is the EAT-Lancet pattern, which was developed by experts from various fields, including health, agriculture, environmental sustainability, and political science, to examine the links between diet, human health, and the environment (32). This pattern requires grams/day of food group consumption, not servings/day of food intake, as well as daily intakes of added fats and added sugars (32). Also, some patterns include nutrients in the algorithm, e.g., added sugar, saturated fat, polyunsaturated fat, and monounsaturated fat, in addition to the food and beverage groups.
Cognitive measures across studies will be restricted to instruments that indicate a potential diagnosis of MCI, or that measure verbal memory, verbal fluency, and executive function. Decline in these cognitive domains often serves as the initial clinical indication of cognitive impairment and dementia due to Alzheimer’s disease (33, 34), and are commonly measured in neuropsychological evaluations.
To harmonize cognitive data a pre-statistical harmonization aimed at ensuring accurate and consistent inferences about cognitive health across studies (e.g., qualitative process involving a review of cohort characteristics and cognitive instruments) (21) will be carried out by categorizing cognitive tests measured in each study into cognitive abilities of interest, and assessing heterogeneity among common tests to determine comparability of tests across cohorts.
In order to determine cognitive abilities measured by cognitive instruments in each study, we will categorize each instrument according to the Cattell-Horn-Carroll (CHC) model (35), which is the most psychometrically established model of cognitive ability. Instruments measuring MCI, verbal memory, verbal fluency, and executive function according to the CHC model will be selected. As a rule of thumb, when investigating the measurement equivalence of overlapping measures within and across cohorts, we will prioritize cognitive tests that are identical. If identical tests are not available, we will focus on conceptually similar tests, and process these data to place them on comparable metrics (36).
Regarding the evaluation of potential sources of heterogeneity among common instruments, we will look at instrument version, instrument adaptation, administration procedures and component items. Data augmentation strategies will be applied if necessary to adjust for differences uncovered during the pre-statistical harmonization process, including alignment of coding procedures, winsorization, and equipercentile equating (21). To that end, we will conduct detailed exploratory analyses of test scores (e.g., dot plots and histograms, stem and leaf plots, tables of minima, maxima, medians, and means) by cohort for every test item presumed to be comparable across cohorts. Harmonization of cognitive measures will adhere to the guidelines provided by the Agency for Healthcare Research and Quality (19). First, we will apply simple monotonic linear transformations to place raw scores on comparable metrics (36). This approach is different from standardization (e.g., use of Z scores), as it only changes the absolute metric of a test, thus it allows the comparison of mean levels of a given variable across cohorts or assessment waves, and relies on comparability of the domain(s) measured by the harmonized tests (37). Then, a latent variable modeling approach will be used to estimate the quality of harmonized variables. We will test measurement equivalence of tests across assessment waves and cohorts. Each test will be conceptualized as an observable indicator of an unobserved (e.g., latent) general cognitive variable (e.g., verbal memory, verbal fluency and executive function) (19, 36).
Measurement equivalence or invariance will be tested by confirmatory factor analysis (CFA), by comparing a series of increasingly strict parsimonious models. More parsimonious or strict models allow a lesser number of parameters to vary over time for the same latent construct. Such parameters are factor loadings (representativeness of each item, labeled as λ), intercepts (mean levels of each item, labeled as τ) and residual variances (unexplained influences predicting item responses, labeled as ɛ). Levels of invariance range from configural that allows λ, τ and ɛ to vary across time, followed by metric invariance that constraints λ, scalar invariance that also constraints τ, and residual invariance that additionally constraints ɛ. If, after fitting equality constraints across cohorts/waves, we do not observe a worsening of absolute model fit (38), then the said level of measurement is judged to hold, and the parameters in question can be considered equivalent. Those tests/cohorts that may harm the assumption of measurement equivalence will be excluded from the analysis.
Definition of potential confounding factors will be made based on a selection of commonly measured confounding factors which are known to influence cognitive function and/or diet (19), at the very minimum the ones selected by the DGAC in their recent protocol for the systematic review of the evidence on dietary patterns and risk of cognitive decline will be included: age, ethnicity, education, socioeconomic status, smoking, physical activity, anthropometry, and alcohol intake (11, 27).
Harmonization of potential confounders will include (i) pre-statistical harmonization using the Maelstrom Research approach (18, 39). For this purpose, we will first define a set of variables targeted for harmonization in a DataSchema and resolved on an a priori description of each variable in order to decide if information collected on each study can be combined in a pooled analysis. And (ii) pairing process to determine compatibility of each study’s data and each variable in the DataSchema on a three-level scale of matching quality: “complete,” “partial,” or ‘impossible” match which will be reported as part of the harmonization process. However, all variables of interest that are a “complete” or “partial” match to the DataSchema will be included in the final data analysis (19).
Associations between diet patterns and cognitive performance will be analyzed using random effects IPD meta-analysis (40). Mixed models are widely used to analyze longitudinal data and are recommended to address missing data as well as to reduce non-random attrition bias. First, linear mixed models will be used to examine the associations of diet patterns and baseline cognitive performance, as well as cognitive decline (per year) on each outcome, separately for each cohort (40). Second, random effects IPD meta-analysis will be used to pool the cohort-wise linear mixed model results to obtain pooled estimates of effect sizes for each of the model terms. All analyses will be stratified by sex.
Around the world, continuing gains in life expectancy coupled with declining fertility rates are producing deep changes in demographic profiles. Today, the world’s population is more than three times larger than it was in 1950 and, by the year 2050, it is estimated to increase by nearly 2 billion people (41). With advancing age, cognitive decline becomes evident, significantly affecting independent living and serving as a hallmark of Alzheimer’s disease and related, largely untreatable dementias. Identifying interventions with the potential to prevent or delay cognitive decline is therefore a critical global public health priority.
As diet has been identified as a modifiable risk factor for the prevention of dementia (42), institutions responsible for developing guidelines and dietary recommendations for the general population point to the need for high quality evidence to answer the long-standing question: What is the relationship between diet patterns and risk of cognitive decline? (27). As highlighted above, numerous attempts have been made to shed light on this question, but different study designs and approaches to measuring both diet patterns and cognitive function have hampered the ability to draw robust conclusions for making specific dietary recommendations. As a result, cognitive endpoints are increasingly being used as primary and secondary outcomes in nutrition research. Unfortunately, there is a lack of consistency in the cognitive tasks used across studies, as well as a lack of standardization in the way cognitive test data are analyzed and reported, which adds to the difficulty of interpreting cognition itself (13).
This study protocol aims to establish the necessary criteria and analysis plan to address the lack of high-quality evidence by harmonizing the existing evidence by a multidisciplinary team of experts in the field of nutrition and cognition. To conduct an IPD meta-analysis from numerous prospective studies, all variables, including the exposure, outcome, and confounding factors, should be comparable across studies. Thus, the main objective for our study protocol was to describe the harmonization process, including the description of the instruments used to assess dietary intake and cognitive function as well as the confounding factors, but also the output. Another important consideration for obtaining robust findings is timing of assessment (43). While in prospective studies of diet and cognition there are at least one baseline and one follow-up assessment of cognitive outcomes, diet assessment (exposure) is typically done at baseline, even when follow-up spans 20 years. This is a critical limitation of many cohort studies. In nutritional epidemiology, repeated measurements of exposure are highly informative because they best represent long-term diet and minimize within-subject variation (44). For this reason, selection of studies with several diet measurements during follow-up will be prioritized.
IPD meta-analysis increases the validity of results and also aligns with the efficient use of resources, as accessible or public data is generally ready to be used and understood by the research community. However, it also poses with limitations as data has not been collected for the purpose of the study.
This protocol will be followed by a results paper with detailed information on all the pitfalls encountered, from the initial stages and data request to the harmonization process and interpretation of the results. Most importantly, the results will help to develop dietary recommendations for the prevention of cognitive decline in middle-aged and older adults that might be considered by future Dietary Guidelines for Americans Advisory Committees in their deliberations. It will also serve as a harmonization guideline for future studies investigating the relationship between diet patterns and cognition, and it is expected to enhance information dissemination on existing studies, crucial for optimizing resources and accelerating dementia prevention research.
AA-G: Methodology, Writing – original draft, Writing – review & editing. NS-D: Methodology, Writing – original draft, Writing – review & editing. S-YY: Writing – original draft, Writing – review & editing. RT: Conceptualization, Funding acquisition, Project administration, Writing – original draft, Writing – review & editing. LS: Conceptualization, Funding acquisition, Project administration, Writing – original draft, Writing – review & editing.
Courtney K. Blackwell, PhD, Northwestern University, Y2tibGFja3dlbGxAbm9ydGh3ZXN0ZXJuLmVkdQ==; Christina Khoo, PhD, Ocean Spray Cranberries, Y2tob29Ab2NlYW5zcHJheS5jb20=; Maxwell Armand Mansolf, PhD, Northwestern University, bWF4d2VsbC5tYW5zb2xmQG5vcnRod2VzdGVybi5lZHU=; Emilio Ros, MD, PhD, IDIBAPS, Barcelona, Spain, ZW1pbGlyb3NyYWhvbGFAZ21haWwuY29t; Linda Snetselaar, PhD, RD, University of Iowa, bGluZGEtc25ldHNlbGFhckB1aW93YS5lZHU=; Tina Wey, PhD, Maelstrom Research, McGill University Health Centre, dHdleUBtYWVsc3Ryb20tcmVzZWFyY2gub3Jn.
The author(s) declare that financial support was received for the research, authorship, and/or publication of this article. This work was supported by the Institute for the Advancement of Food and Nutrition Sciences (IAFNS) via grants IAFNS-IMIM-20230509 and IAFNS-UMINNESOTA-20230419. IAFNS is a nonprofit science organization that pools funding from industry and advances science through the in-kind and financial contributions from private and public sector members.
The authors declare that the research was conducted in the absence of any commercial or financial relationships that could be construed as a potential conflict of interest.
All claims expressed in this article are solely those of the authors and do not necessarily represent those of their affiliated organizations, or those of the publisher, the editors and the reviewers. Any product that may be evaluated in this article, or claim that may be made by its manufacturer, is not guaranteed or endorsed by the publisher.
1. Yassine, HN, Samieri, C, Livingston, G, Glass, K, Wagner, M, Tangney, C, et al. Nutrition state of science and dementia prevention: recommendations of the nutrition for dementia prevention working group. Lancet Healthy Longevity. (2022) 3:e501–12. doi: 10.1016/S2666-7568(22)00120-9
2. Chen, X, Maguire, B, Brodaty, H, and O'Leary, F. Dietary patterns and cognitive health in older adults: A systematic review. J Alzheimers Dis. (2019) 67:583–619. doi: 10.3233/JAD-180468
3. Liu, YH, Gao, X, Na, M, Kris-Etherton, PM, Mitchell, DC, and Jensen, GL. Dietary pattern, diet quality, and dementia: A systematic review and Meta-analysis of prospective cohort studies. J Alzheimers Dis. (2020) 78:151–68. doi: 10.3233/JAD-200499
4. Wengreen, H, Munger, RG, Cutler, A, Quach, A, Bowles, A, Corcoran, C, et al. Prospective study of dietary approaches to stop hypertension-and Mediterranean-style dietary patterns and age-related cognitive change: the Cache County study on memory, health and aging. Am J Clin Nutr. (2013) 98:1263–71. doi: 10.3945/ajcn.112.051276
5. Charisis, S, Ntanasi, E, Yannakoulia, M, Anastasiou, CA, Kosmidis, MH, Dardiotis, E, et al. Mediterranean diet and risk for dementia and cognitive decline in a Mediterranean population. J Am Geriatr Soc. (2021) 69:1548–59. doi: 10.1111/jgs.17072
6. Haring, B, Wu, C, Mossavar-Rahmani, Y, Snetselaar, L, Brunner, R, Wallace, RB, et al. No association between dietary patterns and risk for cognitive decline in older women with 9-year follow-up: data from the Women’s health initiative memory study. J Acad Nutr Diet. (2016) 116:921–30.e1. doi: 10.1016/j.jand.2015.12.017
7. Berendsen, AAM, Kang, JH, van de Rest, O, Feskens, EJM, de Groot, LCPG, and Grodstein, F. The dietary approaches to stop hypertension diet, cognitive function, and cognitive decline in American older women. J Am Med Dir Assoc. (2017) 18:427–32. doi: 10.1016/j.jamda.2016.11.026
8. Berendsen, AM, Kang, JH, Feskens, EJM, de Groot, CPGM, Grodstein, F, and van de Rest, O. Association of Long-Term Adherence to the MIND diet with cognitive function and cognitive decline in American women. J Nutr Health Aging. (2018) 22:222–9. doi: 10.1007/s12603-017-0909-0
9. Barnes, LL, Dhana, K, Liu, X, Carey, VJ, Ventrelle, J, Johnson, K, et al. Trial of the MIND diet for prevention of cognitive decline in older persons. N Engl J Med. (2023) 389:602–11. doi: 10.1056/NEJMoa2302368
10. Hayden, KM, Beavers, DP, Steck, SE, Hebert, JR, Tabung, FK, Shivappa, N, et al. The association between an inflammatory diet and global cognitive function and incident dementia in older women: the Women's Health Initiative memory study. Alzheimers Dement. (2017) 13:1187–96. doi: 10.1016/j.jalz.2017.04.004
11. Dietary Guidelines Advisory Committee. Scientific report of the 2020 dietary guidelines advisory Committee: Advisory report to the secretary of agriculture and the secretary of health and human services. Washington, DC: U.S. Department of Agriculture, Agricultural Research Service (2020).
12. Shim, J-S, Oh, K, and Kim, HC. Dietary assessment methods in epidemiologic studies. Epidemiol Health. (2014) 36:e2014009. doi: 10.4178/epih/e2014009
13. Pase, MP, and Stough, C. An evidence-based method for examining and reporting cognitive processes in nutrition research. Nutr Res Rev. (2014) 27:232–41. doi: 10.1017/S0954422414000158
14. Gurugubelli, VS, Fang, H, Shikany, JM, Balkus, SV, Rumbut, J, Ngo, H, et al. A review of harmonization methods for studying dietary patterns. Smart Health (Amst). (2022) 23:100263. doi: 10.1016/j.smhl.2021.100263
15. Romijn, AR, Latulippe, ME, Snetselaar, L, Willatts, P, Melanson, L, Gershon, R, et al. Perspective: advancing dietary guidance for cognitive health-focus on solutions to harmonize test selection, implementation, and evaluation. Adv Nutr. (2023) 14:366–78. doi: 10.1016/j.advnut.2023.03.010
16. Shaaban, CE, Tudorascu, DL, Glymour, MM, Cohen, AD, Thurston, RC, Snyder, HM, et al. A guide for researchers seeking training in retrospective data harmonization for population neuroscience studies of Alzheimer's disease and related dementias. Front Neuroimag. (2022) 1:1. doi: 10.3389/fnimg.2022.978350
17. Stewart, LA, Clarke, M, Rovers, M, Riley, RD, Simmonds, M, Stewart, G, et al. Preferred reporting items for systematic review and Meta-analyses of individual participant data: the PRISMA-IPD statement. JAMA. (2015) 313:1657–65. doi: 10.1001/jama.2015.3656
18. Fortier, I, Raina, P, Van Den Heuvel, ER, Griffith, LE, Craig, C, Saliba, M, et al. Maelstrom research guidelines for rigorous retrospective data harmonization. Int J Epidemiol. (2016) 46:dyw075–105. doi: 10.1093/ije/dyw075
19. Griffith, L, van den Heuvel, E, Fortier, I, Hofer, S, Raina, P, Sohel, N, et al. Harmonization of cognitive measures in individual participant data and aggregate data meta-analysis. Rockville, MD: Agency for Healthcare Research and Quality (2013).
20. Griffith, LE, Van Den Heuvel, E, Fortier, I, Sohel, N, Hofer, SM, Payette, H, et al. Statistical approaches to harmonize data on cognitive measures in systematic reviews are rarely reported. J Clin Epidemiol. (2015) 68:154–62. doi: 10.1016/j.jclinepi.2014.09.003
21. Briceño, EM, Gross, AL, Giordani, BJ, Manly, JJ, Gottesman, RF, Elkind, MSV, et al. Pre-statistical considerations for harmonization of cognitive instruments: harmonization of ARIC, CARDIA, CHS, FHS, MESA, and NOMAS. J Alzheimers Dis. (2021) 83:1803–13. doi: 10.3233/JAD-210459
22. Shams-White, MM, Pannucci, TE, Lerman, JL, Herrick, KA, Zimmer, M, Meyers Mathieu, K, et al. Healthy eating Index-2020: review and update process to reflect the dietary guidelines for Americans, 2020-2025. J Acad Nutr Diet. (2023) 123:1280–8. doi: 10.1016/j.jand.2023.05.015
23. Dalile, B, Kim, C, Challinor, A, Geurts, L, Gibney, ER, Galdos, MV, et al. The EAT–lancet reference diet and cognitive function across the life course. Lancet Planetary Health. (2022) 6:e749–59. doi: 10.1016/S2542-5196(22)00123-1
24. Schröder, H, Fitó, M, Estruch, R, Martínez-González, MA, Corella, D, Salas-Salvadó, J, et al. A short screener is valid for assessing Mediterranean diet adherence among older Spanish men and women. J Nutr. (2011) 141:1140–5. doi: 10.3945/jn.110.135566
25. Hutchins-Wiese, HL, Bales, CW, and Porter Starr, KN. Mediterranean diet scoring systems: understanding the evolution and applications for Mediterranean and non-Mediterranean countries. Br J Nutr. (2022) 128:1371–92. doi: 10.1017/S0007114521002476
26. Hu, FB. Dietary pattern analysis: a new direction in nutritional epidemiology. Curr Opin Lipidol. (2002) 13:3–9. doi: 10.1097/00041433-200202000-00002
27. Hoelscher, DM, Anderson, C, Booth, S, Deierlein, A, Fung, T, Gardner, C, et al. Dietary patterns and risk of cognitive decline: A systematic review protocol. May 2023. U.S. Department of Agriculture, Food and Nutrition Service, Center for Nutrition Policy and Promotion, Nutrition Evidence Systematic Review. (2023). Available at: https://nesr.usda.gov/protocols
28. Dearborn-Tomazos, JL, Wu, A, Steffen, LM, Anderson, CAM, Hu, EA, Knopman, D, et al. Association of Dietary Patterns in midlife and cognitive function in later life in US adults without dementia. JAMA Netw Open. (2019) 2:e1916641. doi: 10.1001/jamanetworkopen.2019.16641
29. Blackstone, NT, and Conrad, Z. Comparing the recommended eating patterns of the EAT-lancet commission and dietary guidelines for Americans: implications for sustainable nutrition. Curr Dev Nutr. (2020) 4:nzaa015. doi: 10.1093/cdn/nzaa015
30. Nöthlings, U, Murphy, SP, Sharma, S, Hankin, JH, and Kolonel, LN. A comparison of two methods of measuring food group intake: grams vs servings. J Am Diet Assoc. (2006) 106:737–9. doi: 10.1016/j.jada.2006.02.006
31. U.S. Department of Agriculture and U.S. (2020). Department of Health and Human Services. Dietary guidelines for Americans, 2020–2025. 9th edition. Available at: DietaryGuidelines.gov
32. Willett, W, Rockström, J, Loken, B, Springmann, M, Lang, T, Vermeulen, S, et al. Food in the Anthropocene: the EAT–lancet commission on healthy diets from sustainable food systems. Lancet. (2019) 393:447–92. doi: 10.1016/S0140-6736(18)31788-4
33. Albert, MS. Changes in cognition. Neurobiol Aging. (2011) 32:S58–63. doi: 10.1016/j.neurobiolaging.2011.09.010
34. Wilson, RS, Leurgans, SE, Boyle, PA, and Bennett, DA. Cognitive decline in prodromal Alzheimer disease and mild cognitive impairment. Arch Neurol. (2011) 68:351–6. doi: 10.1001/archneurol.2011.31
35. Schneider, J, and McGrew, K. The Cattell-horn-Carroll (CHC) model of intelligence v2.2: A visual tour and summary (2012). Available at: http://www.iapsych.com/chcv2.pdf
36. McElroy, E, Richards, M, Fitzsimons, E, Conti, G, Ploubidis, G, Sullivan, A, et al. Feasibility of retrospectively harmonising cognitive measures in five British birth cohort studies. London: CLOSER (2021).
37. Moeller, J. A word on standardization in longitudinal studies: don't. Front Psychol. (2015) 6:1389. doi: 10.3389/fpsyg.2015.01389
38. Hu, L-t, and Bentler, PM. Cutoff criteria for fit indexes in covariance structure analysis: conventional criteria versus new alternatives. Struct Equ Model. (2009) 6:1–55. doi: 10.1080/10705519909540118
39. Fortier, I, Burton, PR, Robson, PJ, Ferretti, V, Little, J, L'Heureux, F, et al. Quality, quantity and harmony: the DataSHaPER approach to integrating data across bioclinical studies. Int J Epidemiol. (2010) 39:1383–93. doi: 10.1093/ije/dyq139
40. Lipnicki, DM, Makkar, SR, Crawford, JD, Thalamuthu, A, Kochan, NA, Lima-Costa, MF, et al. Determinants of cognitive performance and decline in 20 diverse ethno-regional groups: A COSMIC collaboration cohort study. PLoS Med. (2019) 16:e1002853. doi: 10.1371/journal.pmed.1002853
41. United Nations Department of Economic and Social Affairs, Population Division. World Population Prospects 2022: Summary of Results. (2022).
42. Chowdhary, N, Barbui, C, Anstey, KJ, Kivipelto, M, Barbera, M, Peters, R, et al. Reducing the risk of cognitive decline and dementia: WHO recommendations. Front Neurol. (2022) 12:765584. doi: 10.3389/fneur.2021.765584
43. Stürmer, T, and Brookhart, MA. Study design considerations In: PDN Velentgas and P Nourjah, editors. Developing a protocol for observational comparative effectiveness research: A User's guide. Rockville, MD: Agency for Healthcare Research and Quality (US) (2013)
Keywords: diet, cognition, harmonization, meta-analysis, protocol, longitudinal data
Citation: Ayala-Garcia A, Soldevila-Domenech N, Yi S-Y, de la Torre R and Steffen LM (2024) Diet patterns associated with cognitive decline: methods to harmonize data from European and US cohort studies. Front. Nutr. 11:1379531. doi: 10.3389/fnut.2024.1379531
Received: 31 January 2024; Accepted: 11 March 2024;
Published: 21 March 2024.
Edited by:
Roberta Masella, National Institute of Health (ISS), ItalyReviewed by:
Rosaria Varì, National Institute of Health (ISS), ItalyCopyright © 2024 Ayala-Garcia, Soldevila-Domenech, Yi, de la Torre and Steffen. This is an open-access article distributed under the terms of the Creative Commons Attribution License (CC BY). The use, distribution or reproduction in other forums is permitted, provided the original author(s) and the copyright owner(s) are credited and that the original publication in this journal is cited, in accordance with accepted academic practice. No use, distribution or reproduction is permitted which does not comply with these terms.
*Correspondence: Lyn M. Steffen, c3RlZmZlbkB1bW4uZWR1; Rafael de la Torre, cnRvcnJlMkByZXNlYXJjaG1hci5uZXQ=
†These authors have contributed equally to this work and share first authorship
‡These authors have contributed equally to this work and share last authorship
§ORCID: Rafael de la Torre, https://orcid.org/0000-0002-6765-1866
Lyn M. Steffen, https://orcid.org/0000-0002-4053-6729
Disclaimer: All claims expressed in this article are solely those of the authors and do not necessarily represent those of their affiliated organizations, or those of the publisher, the editors and the reviewers. Any product that may be evaluated in this article or claim that may be made by its manufacturer is not guaranteed or endorsed by the publisher.
Research integrity at Frontiers
Learn more about the work of our research integrity team to safeguard the quality of each article we publish.