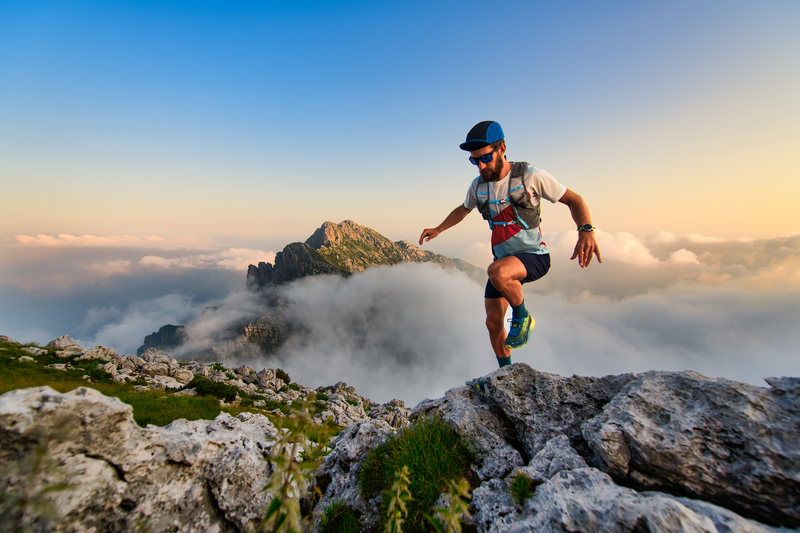
94% of researchers rate our articles as excellent or good
Learn more about the work of our research integrity team to safeguard the quality of each article we publish.
Find out more
ORIGINAL RESEARCH article
Front. Nutr. , 03 May 2024
Sec. Nutrition and Metabolism
Volume 11 - 2024 | https://doi.org/10.3389/fnut.2024.1364841
This article is part of the Research Topic Causal Associations Between Nutrition, Health, and Genes: An Evidence-based Approach View all 9 articles
Background: Nephritis is a pivotal catalyst in chronic kidney disease (CKD) progression. Although epidemiological studies have explored the impact of plasma circulating metabolites and drugs on nephritis, few have harnessed genetic methodologies to establish causal relationships.
Methods: Through Mendelian randomization (MR) in two substantial cohorts, spanning large sample sizes, we evaluated over 100 plasma circulating metabolites and 263 drugs to discern their causal effects on nephritis risk. The primary analytical tool was the inverse variance weighted (IVW) analysis. Our bioinformatic scrutiny of GSE115857 (IgA nephropathy, 86 samples) and GSE72326 (lupus nephritis, 238 samples) unveiled anomalies in lipid metabolism and immunological characteristics in nephritis. Thorough sensitivity analyses (MR-Egger, MR-PRESSO, leave-one-out analysis) were undertaken to verify the instrumental variables’ (IVs) assumptions.
Results: Unique lipoprotein-related molecules established causal links with diverse nephritis subtypes. Notably, docosahexaenoic acid (DHA) emerged as a protective factor for acute tubulointerstitial nephritis (ATIN) (OR1 = 0.84, [95% CI 0.78–0.90], p1 = 0.013; OR2 = 0.89, [95% CI 0.82–0.97], p2 = 0.007). Conversely, multivitamin supplementation minus minerals notably increased the risk of ATIN (OR = 31.25, [95% CI 9.23–105.85], p = 0.004). Reduced α-linolenic acid (ALA) levels due to lipid-lowering drugs were linked to both ATIN (OR = 4.88, [95% CI 3.52–6.77], p < 0.001) and tubulointerstitial nephritis (TIN) (OR = 7.52, [95% CI 2.78–20.30], p = 0.042). While the non-renal drug indivina showed promise for TIN treatment, the use of digoxin, hydroxocobalamin, and liothyronine elevated the risk of chronic tubulointerstitial nephritis (CTIN). Transcriptome analysis affirmed that anomalous lipid metabolism and immune infiltration are characteristic of IgA nephropathy and lupus nephritis. The robustness of these causal links was reinforced by sensitivity analyses and leave-one-out tests, indicating no signs of pleiotropy.
Conclusion: Dyslipidemia significantly contributes to nephritis development. Strategies aimed at reducing plasma low-density lipoprotein levels or ALA supplementation may enhance the efficacy of existing lipid-lowering drug regimens for nephritis treatment. Renal functional status should also be judiciously considered with regard to the use of nonrenal medications.
Nephritis, a prevalent renal disorder, is pathologically categorized into glomerulonephritis and tubulointerstitial nephritis, among other types (1, 2). It is further classified by etiology, including drug-induced nephritis, IgA nephropathy, lupus nephritis, and more (3–5). With the global prevalence of chronic kidney disease (CKD) due to nephritis exceeding 10% (6), this condition poses a substantial and growing public health concern worldwide. Thus, there’s an escalating need for early identification and mitigation of risk factors within high-risk populations, essential for alleviating the impact on global public health.
In recent years, research has unveiled abnormal plasma metabolite levels in nephritis patients, notably associated with lipoprotein metabolism (7). Disruptions in lipid metabolism are recognized as a contributing factor in nephritis and its progression to chronic kidney disease (CKD) (8, 9). Specifically, variants in the apolipoprotein L1 (APOL1) gene have been linked to APOL1 nephropathy (10). Notably, drugs tested in randomized controlled trials for nephritis primarily target immune and inflammatory pathways rather than lipid metabolism (11–14), often due to trial complexities, expenses, and the prolonged nature of nephritis (15–17). The causative link between plasma metabolites and nephritis risk, alongside the effectiveness of lipid-lowering drugs in treatment, remains uncertain.
Genome-wide association studies (GWAS) and Mendelian randomization (MR) stand as formidable tools in drug development and assessing disease risk (18, 19). GWAS offers cost-effective genetic data, and MR harnesses this information for causal inferences, mitigating environmental confounding to unveil genetic-disease associations (20, 21). Often called “nature’s randomized controlled trials,” MR investigates whether carriers of risk alleles exhibit distinct disease risks, delving into causal connections between risk factors, drug targets, and eventual outcomes (22–24).
In this investigation, we employed Mendelian randomization (MR) analysis to examine potential causal links between plasma metabolites, particularly lipoproteins, lipid-lowering medications, and nephritis. Utilizing data from an extensive genome-wide association study (GWAS) encompassing hundreds of thousands of samples, we uncovered connections between circulating metabolites and the risk of nephritis. Remarkably, diverse types of nephritis displayed unique associations with specific circulating metabolites. Moreover, across two distinct European cohorts, we identified DHA as a risk factor for acute tubulointerstitial nephritis (ATIN). Our analysis also delved into the influence of lipid-lowering drugs on nephritis outcomes and probed the viability of drugs commonly used in other conditions for potential nephritis treatment. Further validation using transcriptome data underscored the pivotal role of lipid metabolism in nephritis pathogenesis.
The directed acyclic graph (DAG) of the MR part of this study is shown in Figure 1. Summary data for all cohorts used for Mendelian randomization (MR) analysis can be obtained by the “TwoSampleMR” R package, using the keyword “Treatment/medication code” to search for drug treatment cohorts. The GWAS summary statistics used in this two-sample MR survey are the largest to date, and the outcome cohort was extracted from the Finnish cohort in the summary data using the keyword “Nephritis.” The exposure sample cohort uses data from a different source than the outcome GWAS (Finnish cohort) to minimize sample overlap to ensure robustness. Specifically, exposure cohorts of 123 plasma circulating metabolites, plasma proteins, medications, and Omega-3 polyunsaturated fatty acid levels were derived from independent studies (25–30). The drug treatment cohorts of Simvastatin, Atorvastatin, and Multivitamins +/− minerals and the validation cohort of plasma metabolites are also from the British Biobank, which is different from the Finnish database. Detailed descriptions of the cohorts are in Table 1. All these datasets were subjected to Mendelian randomization analysis following the procedures described in Figure 2. Furthermore, kidney-related datasets GSE115857 (IgA nephropathy, 86 samples) and GSE72326 (lupus nephritis, 238 samples) were retrieved from the GEO database to further investigate the lipid metabolism and immunological features of nephritis.
The Inverse variance weighting (IVW) method was employed as the primary decision-making approach in all Mendelian randomization (MR) analyses conducted in this study, although a total of five MR methods were used, including Weighted median regression, IVW, Mendelian randomization-Egger (MR Egger), Simple mode, and Weighted mode (31). Because the method can indeed satisfy the causal estimation of instruments with instrumental variable assumptions reported elsewhere (21). Additionally, MR-Egger regression and MR-PRESSO were utilized to assess the potential presence of horizontal pleiotropy among instrumental variables.
Subsequently, all exposure instrumental variables (IVs) used in the sensitivity analysis showed significant correlation with the exposure in Mendelian randomization (MR) (p < 5E-8), ensuring the relevance assumption in MR. To maintain the independence assumption in MR, we selectively chose IVs with linkage disequilibrium (LD) < 0.01 after clustering in 10,000 kb windows. Subsequently, downstream sensitivity analysis was conducted on these IVs that met the first two MR assumptions. The sensitivity analysis employed a penalization approach, implementing a systematic leave-one-out method to assess potential pleiotropy for each single nucleotide polymorphism (SNP) and to test adherence to the exclusion restriction assumption. The random-effect inverse-variance weighted (IVW) method was regarded as the primary analysis (31), and MR-Egger, weighted median, weighted mode, simple mode, and MR-pleiotropy residual sum and outlier (MR-PRESSO) were further employed as complementary methods (32). Additionally, heterogeneity among instrumental variables was assessed using the Q-statistic (p < 0.05). MR-Egger regression, along with Mendelian randomization pleiotropy residuals and outliers (MR-PRESSO), was utilized to evaluate horizontal pleiotropy. Evidence of horizontal pleiotropy was based on MR-Egger intercept values significantly deviating from zero (p < 0.05). The pleiotropy test assessed the presence of pleiotropic effects, with p-values > 0.05 considered indicative of no pleiotropy. Because this article is an “exploratory study,” which means discovering as many potentially positive things as possible, multiple testing correction is not performed. All analyses were performed using the “TwoSampleMR” and “MR-PRESSO” packages in R 4.2.3.
The “limma” package was used to identify differentially expressed genes between pathological and normal samples (FDR < 0.05, fold change >1.5). Subsequently, lipid metabolism-related genes were obtained from previous studies, which were used to obtain differentially expressed lipid metabolism-related genes. Furthermore, the DAVID tool1 (33) was employed for Gene Ontology (GO) and Kyoto Encyclopedia of Genes and Genomes (KEGG) enrichment analysis, and terms with high count values were visualized using the “ggplot2” package to generate bubble plots.
Three immune cell infiltration calculation methods, Cibersort2 (34), QuantiSEQ3 (35), EPIC4 (36), and one immune score cell calculation method, ESTIMATE5 (37), were employed to evaluate the immunological characteristics of IgA nephropathy and lupus nephritis. The Kruskal-Wallis test was used to identify differences in immune cell infiltration and immune scores between pathological and control samples (p < 0.05).
In their genome-wide association study, Kettunen et al. investigated 123 circulating metabolites in 24,925 individuals (25), enabling the use of Mendelian randomization (MR) to unveil the widespread causal impact of circulating metabolites on various diseases. Employing the inverse variance weighted (IVW) method as the primary approach in MR analysis, a total of 26 genetically predicted circulating metabolites were found to be associated with the risk of nephritis (Figure 3). Notably, various forms of high-density lipoprotein (HDL), including M.HDL.C (odds ratio (OR): 2.091, 95% confidence interval (CI): 1.204, 3.631), M.HDL.FC (OR: 2.165, 95% CI: 1.211, 3.870), HDL.C (OR: 1.549, 95% CI: 1.062, 2.259), and M.HDL.L (OR: 2.073, 95% CI: 1.032, 4.163), were identified as risk factors for chronic tubulointerstitial nephritis (CTIN). Additionally, phenylalanine (Phe) (OR: 1.906, 95% CI: 1.100, 3.303) was found to be a risk factor for tubulo-interstitial nephritis, not specified as acute or chronic (TIN), while Creatinine (Cre) was identified as a protective factor for CTIN (OR: 0.493, 95% CI: 0.244, 0.996) and a high-risk factor for glomerulonephritis (GN) (OR: 1.378, 95% CI: 1.063, 1.834). It is worth noting that different subtypes of nephritis seemed to correspond to different types of lipoproteins, except for acute tubulointerstitial nephritis (ATIN), where the risk factors were related to fatty acid moieties and proportions (Supplementary Figure S1A). For instance, HDL was identified as a risk factor for CTIN (Supplementary Figure S1B), while LDL and IDL were risk factors for GN (Supplementary Figure S2A). Among the 123 circulating metabolites, only phenylalanine was found to be a risk factor for TIN (Supplementary Figure S2B).
The MR-PRESSO global heterogeneity test showed no horizontal pleiotropy for all instrumental variables (P Global Test >0.05) (Supplementary Table S1). Cochran Q statistics indicated no heterogeneity in the associations between the 26 circulating metabolic traits and the disease (Supplementary Table S2) (Q pval >0.05). However, using the MR-Egger intercept, all risk factors representing GN, except for Crea, exhibited horizontal pleiotropic effects (Supplementary Table S3).
Single MR analysis revealed CH2.in.FA as the most highly correlated independent risk factor for ATIN (Supplementary Figure S3A). In CTIN, M.HDL.L, M.HDL.FC, M.HDL.C, and Crea showed correlation coefficients exceeding 0.7 (Supplementary Figure S3D). Crea was also the most highly correlated independent risk factor for GN (Supplementary Figure S3B), and Phe exhibited a high independent correlation within TIN (Supplementary Figure S3C).
Leave-one-out test results indicated that prominent risk factors did not have any SNP independently driving the corresponding MR results (Supplementary Figure S4). No evidence of directional pleiotropy was observed in the funnel plots (Supplementary Figure S5). Numerous SNPs exhibited the highest independent correlation with GN risk, including rs2731672, rs4939884, rs6507939, rs6507939, rs9987289, rs1800961, rs4939883, rs9987289, rs1461729, rs2126259, and rs2126259 (B > 1, p < 0.05), among others. Additionally, the B coefficient for the rs10265221 instrumental variable representing Crea reached 0.86 (Supplementary Table S4).
We conducted Mendelian randomization studies on a cohort of 115,078 samples from another MR Base. Consistent with the previous results, different types of nephritis appeared to correspond to different types of lipoproteins. Specifically, VLDL, LDL, and IDL were the primary risk factors for GN, while VLDL was the main risk factor for ATIN. Notably, polyunsaturated fatty acids (PUFA) and Docosahexaenic Acid (DHA) were newly discovered protective factors for ATIN. For TIN without specific subtyping, HDL emerged as a more reliable risk factor for TIN compared to LDL (Figure 4).
Figure 4. Mendelian randomization results of inverse variance weighted (IVW) estimates of associations between 249 plasma circulating metabolites and nephritis.
Based on the study by Deming et al., we also examined the impact of 56 proteins in the plasma of 818 individuals on the outcome of nephritis (27) (Supplementary Table S5). The results revealed only one risk factor, APOH, for TIN (OR = 1.239985889, LOWER1.139454911, UPPER1.34938644). This may be attributed to the limitations of the sample size (818 individuals) and the depth of proteomic sequencing (56 proteins). Overall, these findings collectively suggest that the content of lipoproteins in the plasma is a risk factor for developing nephritis.
In independent cohorts, docosahexaenoic acid (DHA), an Omega-3 polyunsaturated fatty acid, consistently emerged as a risk factor for acute tubulointerstitial nephritis (ATIN). Investigating relevant medications within the OMEGA-3 category in the MRbase database becomes essential. Our analysis indicates that reducing lipoprotein-associated phospholipase A2 (Lp-PLA2) via statin therapy can prevent ATIN, while an increase in Lp-PLA2 protects against tubulointerstitial nephritis (TIN). Lowering low-density lipoprotein cholesterol (LDL-C) with statins was deemed preventive against glomerulonephritis (GN) (Supplementary Figure S6A). However, fenofibrate, the second most commonly used lipid-lowering drug, showed no nephritis risk association (Supplementary Table S6).
Excessive niacin and B-complex vitamin supplementation raised nephritis risk. High vitamin B12 levels in plasma increased the likelihood of Chronic Tubulointerstitial Nephritis (CTIN), while elevated vitamin B levels post-ischemic stroke showed the same trend. Interestingly, multivitamin supplementation without appropriate mineral intake increased ATIN risk (Supplementary Figure S6B). Alterations in plasma Omega-3 polyunsaturated fatty acid levels were assessed. Decreased α-Linolenic acid (ALA) percentages in plasma contributed to ATIN, GN, and TIN. Dysregulation of Eicosapentaenoic Acid (EPA) correlated negatively with ATIN risk. Importantly, increased DHA content served as a protective factor for TIN but a risk factor for CTIN (Supplementary Figures S6C–E).
In summary, among the various factors analyzed, Multivitamins+/minerals-plasma levels emerged as the highest risk factor for ATIN, followed by decreased ALA percentage, also a top risk factor for TIN. Reduction in LDL-C emerged as a protective factor against GN, reducing the significance of other exposure factors (Figure 5).
Figure 5. Inverse variance weighting (IVW) estimated risk associations between lipid-lowering medications and changes in plasma omega-3 polyunsaturated fatty acid levels and nephritis.
To explore the possibility that other commonly used drugs in clinical practice could trigger nephritis, we extracted genome-wide association data for 263 drugs from the MR base database using the keyword “Treatment/medication code.” Using the IVW method, we assessed the causal impact of these medications on nephritis outcomes. Most of the drug use was associated with an increased likelihood of developing nephritis, with exceptions observed for Co-amilofruse, Seretide 50 evohaler, Paracetamol, and Indivina 1 mg/2.5 mg tablet (Figure 6). Drugs that are causally linked to the risk of nephritis are often used to treat other conditions, such as Thyroid, Diabetes, Respiratory and Vitamin deficiency. Specifically, Methotrexate and Insulin products emerged as potential high-risk factors for various forms of nephritis. Furthermore, the usage of Digoxin was identified as a high-risk factor for CTIN, followed by Hydroxocobalamin product and Liothyronine. In the case of GN, the use of Calcichew d3 tablet was associated with a high-risk profile, followed by Beclometasone and Folic acid product (Figure 6).
Figure 6. Inverse variance weighting (IVW) estimated the risk association between potential non-nephritic medications and nephritis.
Publicly available datasets from GEO (GSE115857–86 samples, GSE72326–238 samples) were analyzed for IgA nephropathy and lupus nephritis. Differential analysis identified 936 and 1,063 differentially expressed genes for IgA nephropathy and lupus nephritis, respectively. (Supplementary Figures S7A,D).
We assessed 28 and 47 differentially expressed genes related to lipid metabolism in IgA nephropathy and lupus nephritis, respectively, based on our previous research involving 776 lipid metabolism-related genes. Functional enrichment analysis (DAVID 6.8) showed that these genes predominantly localized to the endoplasmic reticulum membrane and were associated with functions like heme binding, iron ion binding, and monooxygenase activity. They played roles in fatty acid metabolic processes, long-chain fatty acid metabolic processes, Metabolic pathways, the PPAR signaling pathway, and insulin resistance (Supplementary Figure S7G).
Abnormal lipid metabolism pathways in lupus nephritis mirrored those in IgA nephropathy. Differentially expressed molecules were primarily located at the endoplasmic reticulum membrane, engaged in lipid metabolic processes, and exhibited oxidoreductase activity (involving the CH-CH group of donors). These genes were linked to Metabolic pathways, Glycerophospholipid metabolism, and Fatty acid metabolism pathways (Supplementary Figure S7H).
In recent years, immune checkpoint inhibitors (ICIs) have been effective in cancer treatment and managing kidney diseases, including nephritis induced by immune deposits (38, 39). New algorithms and “next-generation” sequencing enable computational assessment of immune infiltration differences between nephritis and healthy kidney tissue (34–37). Using CIBERSORT, EPIC, QUANTISEQ, and ESTIMATE methods, immune infiltration disparities in IgA nephropathy and lupus nephritis were revealed. Both conditions showed abnormal B cell and CD4-positive T cell infiltration (Supplementary Figures S8A,B), consistently observed with other algorithms (Supplementary Figures S8C–F). IgA nephropathy exhibited abnormal infiltration of NK cells, monocytes, and activated dendritic cells (Supplementary Figures S8A,C,E), while lupus nephritis displayed abnormal Treg cells, M0 macrophages, and M1 macrophages infiltration (Supplementary Figures S8B,D,F).
In this study, we utilized a two-sample Mendelian randomization (MR) approach to investigate causal connections between plasma metabolites and nephritis. Bioinformatics analysis of transcriptomics data revealed distinct lipid metabolism and immune traits in IgA nephropathy and lupus nephritis.
The MR analysis of plasma circulating metabolites in two cohorts, Kettunen (24,925 samples) and Borges CM (115,078 samples), demonstrated that different types of lipoproteins are causally associated with distinct types of nephritis. Specifically, HDL-related circulating metabolites showed a tendency to be causally associated with CTIN, consistent with previous reports (40, 41). While randomized controlled trials did not show evident causal relationships between LDL and IDL in GN, reports indicated a negative correlation between LDL-C and renal function as well as kidney disease (42, 43). Unfortunately, current evidence does not support a causal relationship between VLDL-related circulating metabolites and ATIN. Lp-PLA2 is one of the isoforms in the phospholipase superfamily and is secreted into plasma by macrophages, T cells and mast cells (44). Lp-PLA is currently a recognized marker of cardiovascular disease, but it plays different roles in inflammation and atherosclerosis depending on the lipoprotein bound in plasma (45). The results of this study show that the decrease in Lp-PLA2 caused by statins is a protective factor for ATIN, and the increase in Lp-PLA2 caused by statins is also a protective factor for TIN. This may be caused by intra-group differences in TIN (Tubulo-interstitial nephritis, not specified as acute or chronic), because the summary data we obtained failed to distinguish ATIN and TIN in the TIN group. In addition, the clinical manifestations of TIN with different causes will be different (46–49), and different types of nephritis will also have different pathological characteristics and clinical outcomes (50–53). Therefore, compared with TIN, the conclusion that decreased Lp-PLA2 is a protective factor for ATIN is more reliable, while the conclusion that increased Lp-PLA2 is a protective factor for TIN needs to be treated with caution.
In the mentioned cohorts, an intriguing negative link between DHA and ATIN led to deeper exploration of Omega-3 fatty acid drugs (e.g., statins, niacin, fenofibrate) in nephritis. Surprisingly, multivitamin supplementation without minerals was tied to higher ATIN risk, warranting further investigation. Statins, commonly employed in CKD treatment for reducing cardiovascular disease risk (54), showed interesting associations in our study. Specifically, statin-induced ALA reduction was found to increase the risk of ATIN and GN, while statin-induced LDL reduction exhibited a protective effect against GN. Previous studies have identified ALA as a predictive biomarker for chronic kidney disease (CKD) (55), although its ability to slow the progression of IgA nephropathy remains inconclusive (56). In contrast, LDL has been suggested to play a role in CKD progression in certain observational and mechanistic studies (42, 57), and some reports even propose lower LDL levels to enhance the therapeutic benefits of statins in treating patients with chronic kidney disease (58). Based on these findings, we recommend cautious ALA supplementation with statins in CKD for optimized efficacy.
In recent years, numerous drugs have been discovered to possess potential for treating other diseases (59, 60), a phenomenon termed “new uses of old drugs” (61). Therefore, driven by curiosity about whether drugs used for other diseases are beneficial in treating nephritis, we further explored the causal relationship between 263 other drugs and nephritis. Among the 26 positive results, digoxin, hydroxocobalamin, and liothyronine were identified as the only three high-risk factors for CTIN. Among these, Digoxin is commonly believed to reduce the risk of CKD through its treatment of cardiovascular diseases (62, 63). However, our research results provide support for the notion that excessive use of Digoxin is, in fact, one of the high-risk factors for CKD (64). Additionally, thyroid hormones regulated by liothyronine and thyroid diseases are often closely related to CKD (65–67). In an MR study concerning drugs, concluding without considering hidden co-founders and pathway analysis is risky. Therefore, the findings of these drugs must be treated with caution. They can only serve as a basis for subsequent research and cannot be directly used for clinical recommendations and guidance.
The aforementioned findings indicate that lipoproteins and fatty acids in circulating metabolites are potential risk factors for nephritis, a conclusion supported by proteomic data analysis (68, 69). For instance, Chen et al. conducted quantitative proteomic analysis on 59 IgA nephropathy cases and 19 normal controls, identifying novel molecular subtypes (70). They highlighted specific alterations in fatty acid β-oxidation, the TCA cycle, glycolysis, and oxidative phosphorylation among these subtypes, findings consistent with those of Dong et al. (71). The gut microbiome, proteomic and metabolomic landscape of renal diseases offers insights into pathogenesis, treatment, and prevention, yet transcriptomic studies remain scarce (50, 72–75). Scientists are also promoting the discovery of new treatments and targets for various types of nephritis (76–79). Indeed, integrating transcriptomics with other omics can further advance the discovery of novel mechanisms and biomarkers for renal diseases (80). Therefore, we leveraged transcriptomic data from IgA nephropathy and lupus nephritis to elucidate their lipid metabolism and immune features, as dysregulation of immune regulatory molecules or immune function is also considered a contributing factor to nephritis onset and progression (38). Dysregulation of lipid oxidation, uptake, and biosynthesis is deemed a predisposing factor for CKD, a notion supported by an early pioneering study (9, 81). Additionally, IgA nephropathy and lupus nephritis exhibit aberrant B-cell and CD4 T-cell infiltration. Mechanistic and observational studies suggest that modulation through B cells and CD4 T cells can effectively suppress the development of IgA nephropathy and lupus nephritis, findings consistent with our study results (82–87).
Compared to other Mendelian randomization studies on kidney diseases, the strength of our research lies in the direct use of clinically diagnosed outcomes as the outcome variables, including Acute tubulo-interstitial nephritis, Chronic tubulo-interstitial nephritis, Glomerulonephritis, and Tubulo-interstitial nephritis. Instead of relying on traditional measures of kidney function such as glomerular filtration rate or creatinine clearance, we have utilized these clinically relevant diagnostic outcomes, enabling a clearer delineation of the causal relationships between exposure factors and diseases. Moreover, the robust sensitivity analysis ensures the reliability of the instrumental variables, as described in the Methods section.
This study used summary statistics data from MR base, limiting our ability to explore U-shaped relationships between exposure factors and nephritis. The availability of larger sample sizes was restricted, affecting statistical power for some instrumental variables. In addition, in order to explore more potential positive exposure factors, this study did not correct the results, which may lead to false positives. Not only that, when analyzing the causal association between exposure factors (Omega-3 polyunsaturated fatty acid) and nephritis outcomes, a cohort of European ancestry was not available, and the different ancestry of the exposure and outcome samples may lead to biased results. Although this result meets the criteria for a test of heterogeneity, this result needs to be interpreted with caution. Moreover, due to the inability to obtain complete original data, bidirectional causal MR analysis was not performed, and it was also impossible to further clarify the pathological stratification of outcome variables to obtain more valuable clinical significance. Nevertheless, the study identified the studied exposure factors as causal factors for nephritis.
Our MR study supports a causal link between plasma metabolites, especially DHA, and nephritis. Lipid-lowering drugs offer potential as nephritis treatments, possibly in combination with other medications for enhanced outcomes. Non-nephritic drugs like Indivina and co-amilofruse show promise for TIN treatment. However, caution is needed with drugs like Digoxin, hydroxocobalamin, and liothyronine, depending on the patient’s renal function. Additionally, transcriptomics data analysis confirms abnormal lipid metabolism and immune features in nephritis, advancing our understanding of its pathogenesis and potential for targeted therapies.
The data presented in the study are deposited in the GEO repository, accession number GSE115857 (https://www.ncbi.nlm.nih.gov/geo/query/acc.cgi?acc=GSE115857) and GSE72326 (https://www.ncbi.nlm.nih.gov/geo/query/acc.cgi?acc=GSE72326).
Ethical approval was not required for the study involving humans in accordance with the local legislation and institutional requirements. Written informed consent to participate in this study was not required from the participants or the participants’ legal guardians/next of kin in accordance with the national legislation and the institutional requirements.
FS: Conceptualization, Data curation, Formal analysis, Methodology, Writing – original draft. YY: Conceptualization, Data curation, Investigation, Software, Writing – original draft. DW: Supervision, Validation, Writing – original draft. RW: Methodology, Supervision, Writing – original draft. RL: Validation, Writing – original draft. YZ: Methodology, Writing – original draft. EL: Data curation, Writing – original draft. MW: Writing – original draft. YT: Resources, Supervision, Writing – review & editing. YD: Investigation, Resources, Supervision, Writing – review & editing. YX: Funding acquisition, Project administration, Supervision, Writing – review & editing.
The author(s) declare financial support was received for the research, authorship, and/or publication of this article. This work was supported by the National Natural Science Foundation of China [32270834 (to YX)]; and the Basic Science and Frontier Technology Research Program of Chongqing Science and Technology Commission [grant number cstc2021jcyj-msxmX0312 (to YX)].
We thank all those who contributed to the GWAS study cohort in Table 1, and those who contributed to the two transcriptomics cohorts GSE115857 and GSE72326.
The authors declare that the research was conducted in the absence of any commercial or financial relationships that could be construed as a potential conflict of interest.
All claims expressed in this article are solely those of the authors and do not necessarily represent those of their affiliated organizations, or those of the publisher, the editors and the reviewers. Any product that may be evaluated in this article, or claim that may be made by its manufacturer, is not guaranteed or endorsed by the publisher.
The Supplementary material for this article can be found online at: https://www.frontiersin.org/articles/10.3389/fnut.2024.1364841/full#supplementary-material
1. ^https://david.ncifcrf.gov/tools.jsp
2. ^https://cibersortx.stanford.edu/
3. ^https://icbi.i-med.ac.at/software/quantiseq/doc/
1. Floege, J, and Amann, K. Primary glomerulonephritides. Lancet. (2016) 387:2036–48. doi: 10.1016/S0140-6736(16)00272-5
2. Perazella, MA, and Markowitz, GS. Drug-induced acute interstitial nephritis. Nat Rev Nephrol. (2010) 6:461–70. doi: 10.1038/nrneph.2010.71
3. Satoskar, AA, Parikh, SV, and Nadasdy, T. Epidemiology, pathogenesis, treatment and outcomes of infection-associated glomerulonephritis. Nat Rev Nephrol. (2020) 16:32–50. doi: 10.1038/s41581-019-0178-8
4. Lai, KN, Tang, SC, Schena, FP, Novak, J, Tomino, Y, Fogo, AB, et al. IgA nephropathy. Nat Rev Dis Primers. (2016) 2:16001. doi: 10.1038/nrdp.2016.1
5. Anders, HJ, Saxena, R, Zhao, MH, Parodis, I, Salmon, JE, and Mohan, C. Lupus nephritis. Nat Rev Dis Primers. (2020) 6:7. doi: 10.1038/s41572-019-0141-9
6. Jha, V, Garcia-Garcia, G, Iseki, K, Li, Z, Naicker, S, Plattner, B, et al. Chronic kidney disease: global dimension and perspectives. Lancet. (2013) 382:260–72. doi: 10.1016/S0140-6736(13)60687-X
7. Dong, L, Li, YQ, Guo, SM, Xu, G, Wei, W, and Han, M. Hypercholesterolemia correlates with glomerular phospholipase A2 receptor deposit and serum anti-phospholipase A2 receptor antibody and predicts proteinuria outcome in idiopathic membranous nephropathy. Front Immunol. (2022) 13:905930. doi: 10.3389/fimmu.2022.905930
8. Brennan, E, Kantharidis, P, Cooper, ME, and Godson, C. Pro-resolving lipid mediators: regulators of inflammation, metabolism and kidney function. Nat Rev Nephrol. (2021) 17:725–39. doi: 10.1038/s41581-021-00454-y
9. Baek, J, He, C, Afshinnia, F, Michailidis, G, and Pennathur, S. Lipidomic approaches to dissect dysregulated lipid metabolism in kidney disease. Nat Rev Nephrol. (2022) 18:38–55. doi: 10.1038/s41581-021-00488-2
10. Genovese, G, Friedman, DJ, Ross, MD, Lecordier, L, Uzureau, P, Freedman, BI, et al. Association of trypanolytic ApoL1 variants with kidney disease in African Americans. Science. (2010) 329:841–5. doi: 10.1126/science.1193032
11. Lv, J, Wong, MG, Hladunewich, MA, Jha, V, Hooi, LS, Monaghan, H, et al. Effect of Oral methylprednisolone on decline in kidney function or kidney failure in patients with IgA nephropathy: the TESTING randomized clinical trial. JAMA. (2022) 327:1888–98. doi: 10.1001/jama.2022.5368
12. Kaye, KS, Bhowmick, T, Metallidis, S, Bleasdale, SC, Sagan, OS, Stus, V, et al. Effect of Meropenem-Vaborbactam vs piperacillin-Tazobactam on clinical cure or improvement and microbial eradication in complicated urinary tract infection: the TANGO I randomized clinical trial. JAMA. (2018) 319:788–99. doi: 10.1001/jama.2018.0438
13. Furie, RA, Aroca, G, Cascino, MD, Garg, JP, Rovin, BH, Alvarez, A, et al. B-cell depletion with obinutuzumab for the treatment of proliferative lupus nephritis: a randomised, double-blind, placebo-controlled trial. Ann Rheum Dis. (2022) 81:100–7. doi: 10.1136/annrheumdis-2021-220920
14. He, J, Zhang, R, Shao, M, Zhao, X, Miao, M, Chen, J, et al. Efficacy and safety of low-dose IL-2 in the treatment of systemic lupus erythematosus: a randomised, double-blind, placebo-controlled trial. Ann Rheum Dis. (2020) 79:141–9. doi: 10.1136/annrheumdis-2019-215396
15. Fanouriakis, A, Tziolos, N, Bertsias, G, and Boumpas, DT. Update omicronn the diagnosis and management of systemic lupus erythematosus. Ann Rheum Dis. (2021) 80:14–25. doi: 10.1136/annrheumdis-2020-218272
16. Alsharhan, L, and Beck, LH Jr. Membranous nephropathy: Core curriculum 2021. Am J Kidney Dis. (2021) 77:440–53. doi: 10.1053/j.ajkd.2020.10.009
17. Pattrapornpisut, P, Avila-Casado, C, and Reich, HN. IgA nephropathy: Core curriculum 2021. Am J Kidney Dis. (2021) 78:429–41. doi: 10.1053/j.ajkd.2021.01.024
18. Carss, KJ, Deaton, AM, Del Rio-Espinola, A, Diogo, D, Fielden, M, Kulkarni, DA, et al. Using human genetics to improve safety assessment of therapeutics. Nat Rev Drug Discov. (2023) 22:145–62. doi: 10.1038/s41573-022-00561-w
19. Przybyla, L, and Gilbert, LA. A new era in functional genomics screens. Nat Rev Genet. (2022) 23:89–103. doi: 10.1038/s41576-021-00409-w
20. Heerspink, HJL, Radhakrishnan, J, Alpers, CE, Barratt, J, Bieler, S, Diva, U, et al. Sparsentan in patients with IgA nephropathy: a prespecified interim analysis from a randomised, double-blind, active-controlled clinical trial. Lancet. (2023) 401:1584–94. doi: 10.1016/S0140-6736(23)00569-X
21. Davey Smith, G, and Hemani, G. Mendelian randomization: genetic anchors for causal inference in epidemiological studies. Hum Mol Genet. (2014) 23:R89–98. doi: 10.1093/hmg/ddu328
22. Ference, BA, Majeed, F, Penumetcha, R, Flack, JM, and Brook, RD. Effect of naturally random allocation to lower low-density lipoprotein cholesterol on the risk of coronary heart disease mediated by polymorphisms in NPC1L1, HMGCR, or both: a 2 x 2 factorial Mendelian randomization study. J Am Coll Cardiol. (2015) 65:1552–61. doi: 10.1016/j.jacc.2015.02.020
23. Li, Y, Sundquist, K, Zhang, N, Wang, X, Sundquist, J, and Memon, AA. Mitochondrial related genome-wide Mendelian randomization identifies putatively causal genes for multiple cancer types. EBioMedicine. (2023) 88:104432. doi: 10.1016/j.ebiom.2022.104432
24. Li, Z, Zhang, B, Liu, Q, Tao, Z, Ding, L, Guo, B, et al. Genetic association of lipids and lipid-lowering drug target genes with non-alcoholic fatty liver disease. EBioMedicine. (2023) 90:104543. doi: 10.1016/j.ebiom.2023.104543
25. Kettunen, J, Demirkan, A, Wurtz, P, Draisma, HH, Haller, T, Rawal, R, et al. Genome-wide study for circulating metabolites identifies 62 loci and reveals novel systemic effects of LPA. Nat Commun. (2016) 7:11122. doi: 10.1038/ncomms11122
26. Borges, MC, Haycock, P, Zheng, J, Hemani, G, Howe, LJ, Schmidt, AF, et al. The impact of fatty acids biosynthesis on the risk of cardiovascular diseases in Europeans and east Asians: a Mendelian randomization study. Hum Mol Genet. (2022) 31:4034–54. doi: 10.1093/hmg/ddac153
27. Deming, Y, Xia, J, Cai, Y, Lord, J, Del-Aguila, JL, Fernandez, MV, et al. Genetic studies of plasma analytes identify novel potential biomarkers for several complex traits. Sci Rep. (2016) 6:18092. doi: 10.1038/srep18092
28. Aslibekyan, S, An, P, Frazier-Wood, AC, Kabagambe, EK, Irvin, MR, Straka, RJ, et al. Preliminary evidence of genetic determinants of adiponectin response to fenofibrate in the genetics of lipid lowering drugs and diet network. Nutr Metab Cardiovasc Dis. (2013) 23:987–94. doi: 10.1016/j.numecd.2012.07.010
29. Moy, KA, Mondul, AM, Zhang, H, Weinstein, SJ, Wheeler, W, Chung, CC, et al. Genome-wide association study of circulating vitamin D-binding protein. Am J Clin Nutr. (2014) 99:1424–31. doi: 10.3945/ajcn.113.080309
30. Dorajoo, R, Sun, Y, Han, Y, Ke, T, Burger, A, Chang, X, et al. A genome-wide association study of n-3 and n-6 plasma fatty acids in a Singaporean Chinese population. Genes Nutr. (2015) 10:53. doi: 10.1007/s12263-015-0502-2
31. Burgess, S, Davey Smith, G, Davies, NM, Dudbridge, F, Gill, D, Glymour, MM, et al. Guidelines for performing Mendelian randomization investigations: update for summer 2023. Wellcome Open Res. (2019) 4:186. doi: 10.12688/wellcomeopenres.15555.1
32. Zhang, N, Liao, Y, Zhao, H, Chen, T, Jia, F, Yu, Y, et al. Polycystic ovary syndrome and 25-hydroxyvitamin D: a bidirectional two-sample Mendelian randomization study. Front Endocrinol (Lausanne). (2023) 14:1110341. doi: 10.3389/fendo.2023.1110341
33. Sherman, BT, Hao, M, Qiu, J, Jiao, X, Baseler, MW, Lane, HC, et al. DAVID: a web server for functional enrichment analysis and functional annotation of gene lists (2021 update). Nucleic Acids Res. (2022) 50:W216–21. doi: 10.1093/nar/gkac194
34. Chen, B, Khodadoust, MS, Liu, CL, Newman, AM, and Alizadeh, AA. Profiling tumor infiltrating immune cells with CIBERSORT. Methods Mol Biol. (2018) 1711:243–59. doi: 10.1007/978-1-4939-7493-1_12
35. Plattner, C, Finotello, F, and Rieder, D. Deconvoluting tumor-infiltrating immune cells from RNA-seq data using quanTIseq. Methods Enzymol. (2020) 636:261–85. doi: 10.1016/bs.mie.2019.05.056
36. Racle, J, and Gfeller, D. EPIC: a tool to Estimate the proportions of different cell types from bulk gene expression data. Methods Mol Biol. (2020) 2120:233–48. doi: 10.1007/978-1-0716-0327-7_17
37. Yoshihara, K, Shahmoradgoli, M, Martinez, E, Vegesna, R, Kim, H, Torres-Garcia, W, et al. Inferring tumour purity and stromal and immune cell admixture from expression data. Nat Commun. (2013) 4:2612. doi: 10.1038/ncomms3612
38. Anders, HJ, Kitching, AR, Leung, N, and Romagnani, P. Glomerulonephritis: immunopathogenesis and immunotherapy. Nat Rev Immunol. (2023) 23:453–71. doi: 10.1038/s41577-022-00816-y
39. Sprangers, B, Leaf, DE, Porta, C, Soler, MJ, and Perazella, MA. Diagnosis and management of immune checkpoint inhibitor-associated acute kidney injury. Nat Rev Nephrol. (2022) 18:794–805. doi: 10.1038/s41581-022-00630-8
40. Lanktree, MB, Theriault, S, Walsh, M, and Pare, G. HDL Cholesterol, LDL Cholesterol, and triglycerides as risk factors for CKD: a Mendelian randomization study. Am J Kidney Dis. (2018) 71:166–72. doi: 10.1053/j.ajkd.2017.06.011
41. Rasheed, H, Zheng, J, Rees, J, Sanderson, E, Thomas, L, Richardson, TG, et al. The causal effects of serum lipids and apolipoproteins on kidney function: multivariable and bidirectional Mendelian-randomization analyses. Int J Epidemiol. (2021) 50:1569–79. doi: 10.1093/ije/dyab014
42. Rahman, M, Yang, W, Akkina, S, Alper, A, Anderson, AH, Appel, LJ, et al. Relation of serum lipids and lipoproteins with progression of CKD: the CRIC study. Clin J Am Soc Nephrol. (2014) 9:1190–8. doi: 10.2215/CJN.09320913
43. Kuma, A, Uchino, B, Ochiai, Y, Kawashima, M, Enta, K, Tamura, M, et al. Impact of low-density lipoprotein cholesterol on decline in estimated glomerular filtration rate in apparently healthy young to middle-aged working men. Clin Exp Nephrol. (2018) 22:15–27. doi: 10.1007/s10157-017-1407-8
44. Peng, Z, Chang, Y, Fan, J, Ji, W, and Su, C. Phospholipase A2 superfamily in cancer. Cancer Lett. (2021) 497:165–77. doi: 10.1016/j.canlet.2020.10.021
45. Tselepis, AD. Oxidized phospholipids and lipoprotein-associated phospholipase a(2) as important determinants of Lp(a) functionality and pathophysiological role. J Biomed Res. (2018) 31:13–22. doi: 10.7555/JBR.31.20160009
46. From the American Association of Neurological Surgeons (AANS), American Society of Neuroradiology (ASNR), Cardiovascular and Interventional Radiology Society of Europe (CIRSE), Canadian Interventional Radiology Association (CIRA), Congress of Neurological Surgeons (CNS), European Society of Minimally Invasive Neurological Therapy (ESMINT), European Society of Neuroradiology (ESNR), European Stroke Organization (ESO), Society for Cardiovascular Angiography and Interventions (SCAI), Society of Interventional Radiology (SIR), Society of NeuroInterventional Surgery (SNIS), and World Stroke Organization (WSO)Sacks, D, Baxter, B, et al. Multisociety consensus quality improvement revised consensus statement for endovascular therapy of acute ischemic stroke. Int J Stroke. (2018) 13:612–32. doi: 10.1177/1747493018778713
47. Mohd, R, Chin, SF, Shaharir, SS, and Cham, QS. Involvement of gut microbiota in SLE and lupus nephritis. Biomedicines. (2023) 11:653. doi: 10.3390/biomedicines11030653
48. Hu, Q, Chen, Y, Deng, X, Li, Y, Ma, X, Zeng, J, et al. Diabetic nephropathy: focusing on pathological signals, clinical treatment, and dietary regulation. Biomed Pharmacother. (2023) 159:114252. doi: 10.1016/j.biopha.2023.114252
49. Muhammad, A, Zhang, Y, Huang, L, Yuan, Q, Wang, W, Pu, J, et al. The diagnosis of acute interstitial nephritis caused by infection versus antibiotic-induced interstitial nephritis: a narrative review. Clin Kidney J. (2024) 17:sfae054. doi: 10.1093/ckj/sfae054
50. Jiang, Y, Wang, T, Yu, W, Wu, F, Guo, R, Li, H, et al. Combination of the gut microbiota and clinical indicators as a potential index for differentiating idiopathic membranous nephropathy and minimal change disease. Ren Fail. (2023) 45:2209392. doi: 10.1080/0886022X.2023.2209392
51. Mok, CC, Teng, YKO, Saxena, R, and Tanaka, Y. Treatment of lupus nephritis: consensus, evidence and perspectives. Nat Rev Rheumatol. (2023) 19:227–38. doi: 10.1038/s41584-023-00925-5
52. Shi, J, Xu, S, Chen, J, and Wu, H. Clinical manifestations and outcomes in tubulointerstitial nephritis and uveitis syndrome: a case report and a systematic review in China. Int Urol Nephrol. (2024) 56:1415–27. doi: 10.1007/s11255-023-03797-6
53. Southgate, G, Clarke, P, and Harmer, MJ. Renal outcomes in tubulointerstitial nephritis and uveitis (TINU) syndrome: a systematic review and meta-analysis. J Nephrol. (2023) 36:507–19. doi: 10.1007/s40620-022-01478-8
54. Chen, TK, Knicely, DH, and Grams, ME. Chronic kidney disease diagnosis and management: a review. JAMA. (2019) 322:1294–304. doi: 10.1001/jama.2019.14745
55. Han, KH, Kim, B, Ji, SC, Kang, HG, Cheong, HI, Cho, JY, et al. Mechanism of chronic kidney disease progression and novel biomarkers: a Metabolomic analysis of experimental glomerulonephritis. Meta. (2020) 10:169. doi: 10.3390/metabo10040169
56. Jia, Q, Shi, Y, Bennink, MB, and Pestka, JJ. Docosahexaenoic acid and eicosapentaenoic acid, but not alpha-linolenic acid, suppress deoxynivalenol-induced experimental IgA nephropathy in mice. J Nutr. (2004) 134:1353–61. doi: 10.1093/jn/134.6.1353
57. Kerforne, T, Favreau, F, Khalifeh, T, Maiga, S, Allain, G, Thierry, A, et al. Hypercholesterolemia-induced increase in plasma oxidized LDL abrogated pro angiogenic response in kidney grafts. J Transl Med. (2019) 17:26. doi: 10.1186/s12967-018-1764-4
58. Cholesterol Treatment Trialists, C, Herrington, WG, Emberson, J, Mihaylova, B, Blackwell, L, Reith, C, et al. Impact of renal function on the effects of LDL cholesterol lowering with statin-based regimens: a meta-analysis of individual participant data from 28 randomised trials. Lancet Diabetes Endocrinol. (2016) 4:829–39. doi: 10.1016/S2213-8587(16)30156-5
59. Yang, H, Chen, X, Li, K, Cheaito, H, Yang, Q, Wu, G, et al. Repurposing old drugs as new inhibitors of the ubiquitin-proteasome pathway for cancer treatment. Semin Cancer Biol. (2021) 68:105–22. doi: 10.1016/j.semcancer.2019.12.013
60. Kale, VP, Habib, H, Chitren, R, Patel, M, Pramanik, KC, Jonnalagadda, SC, et al. Old drugs, new uses: drug repurposing in hematological malignancies. Semin Cancer Biol. (2021) 68:242–8. doi: 10.1016/j.semcancer.2020.03.005
61. Godoy, DA, Badenes, R, Pelosi, P, and Robba, C. Ketamine in acute phase of severe traumatic brain injury "an old drug for new uses?". Crit Care. (2021) 25:19. doi: 10.1186/s13054-020-03452-x
62. Beldhuis, IE, Lam, CSP, Testani, JM, Voors, AA, Van Spall, HGC, Ter Maaten, JM, et al. Evidence-based medical therapy in patients with heart failure with reduced ejection fraction and chronic kidney disease. Circulation. (2022) 145:693–712. doi: 10.1161/CIRCULATIONAHA.121.052792
63. Lunney, M, Ruospo, M, Natale, P, Quinn, RR, Ronksley, PE, Konstantinidis, I, et al. Pharmacological interventions for heart failure in people with chronic kidney disease. Cochrane Database Syst Rev. (2020) 2020:CD012466. doi: 10.1002/14651858.CD012466.pub2
64. Muanda, FT, Weir, MA, Ahmadi, F, McArthur, E, Sontrop, JM, Kim, RB, et al. Digoxin dosing and the risk of toxicity in older adults with CKD. Am J Kidney Dis. (2022) 79:912–7. doi: 10.1053/j.ajkd.2021.09.024
65. Uchiyama-Matsuoka, N, Tsuji, K, Uchida, HA, Kitamura, S, Itoh, Y, Nishiyama, Y, et al. Masked CKD in hyperthyroidism and reversible CKD status in hypothyroidism. Front Endocrinol (Lausanne). (2022) 13:1048863. doi: 10.3389/fendo.2022.1048863
66. Schultheiss, UT, Steinbrenner, I, Nauck, M, Schneider, MP, Kotsis, F, Baid-Agrawal, S, et al. Thyroid function, renal events and mortality in chronic kidney disease patients: the German chronic kidney disease study. Clin Kidney J. (2021) 14:959–68. doi: 10.1093/ckj/sfaa052
67. Zoccali, C, Tripepi, G, Cutrupi, S, Pizzini, P, and Mallamaci, F. Low triiodothyronine: a new facet of inflammation in end-stage renal disease. J Am Soc Nephrol. (2005) 16:2789–95. doi: 10.1681/ASN.2005040356
68. Kobayashi, H, Looker, HC, Satake, E, Saulnier, PJ, Md Dom, ZI, O'Neil, K, et al. Results of untargeted analysis using the SOMAscan proteomics platform indicates novel associations of circulating proteins with risk of progression to kidney failure in diabetes. Kidney Int. (2022) 102:370–81. doi: 10.1016/j.kint.2022.04.022
69. Dubin, RF, Deo, R, Ren, Y, Wang, J, Zheng, Z, Shou, H, et al. Proteomics of CKD progression in the chronic renal insufficiency cohort. Nat Commun. (2023) 14:6340. doi: 10.1038/s41467-023-41642-7
70. Chen, X, Li, M, Zhu, S, Lu, Y, Duan, S, Wang, X, et al. Proteomic profiling of IgA nephropathy reveals distinct molecular prognostic subtypes. iScience. (2023) 26:105961. doi: 10.1016/j.isci.2023.105961
71. Dong, J, Zheng, F, Liu, F, He, J, Li, S, Pu, W, et al. Global-feature of autoimmune glomerulonephritis using proteomic analysis of laser capture microdissected glomeruli. Front Immunol. (2023) 14:1131164. doi: 10.3389/fimmu.2023.1131164
72. Rinschen, MM, and Saez-Rodriguez, J. The tissue proteome in the multi-omic landscape of kidney disease. Nat Rev Nephrol. (2021) 17:205–19. doi: 10.1038/s41581-020-00348-5
73. Dubin, RF, and Rhee, EP. Proteomics and metabolomics in kidney disease, including insights into etiology, Treatment, and prevention. Clin J Am Soc Nephrol. (2020) 15:404–11. doi: 10.2215/CJN.07420619
74. Barone, FP, Lourenço, SV, Azevedo, LS, de Souza, MN, Coutinho-Camillo, CM, and Florezi, GP. Metabolomics and biomarkers for lupus nephritis – a systematic review. Surg Exp Pathol. (2023) 6:11. doi: 10.1186/s42047-023-00136-y
75. Hu, L, Peng, Z, Bai, G, Fu, H, Tan, DJ, Wang, J, et al. Lipidomic profiles in serum and urine in children with steroid sensitive nephrotic syndrome. Clin Chim Acta. (2024) 555:117804. doi: 10.1016/j.cca.2024.117804
76. Akhmatova, YA, Akhmatov, A, Yuldashev, BA, and Ruzikulov, NY. Chronic tubulointerstitial nephritis in children: new approaches to diagnosis and treatment. E3S Web Conf. (2023) 413:03031. doi: 10.1051/e3sconf/202341303031
77. Li, HY, Huang, LF, Huang, XR, Wu, D, Chen, XC, Tang, JX, et al. Endoplasmic reticulum stress in systemic lupus erythematosus and lupus nephritis: potential therapeutic target. J Immunol Res. (2023) 2023:7625817. doi: 10.1155/2023/7625817
78. Sestan, M, and Jelusic, M. Diagnostic and management strategies of IgA Vasculitis nephritis/Henoch-Schonlein Purpura nephritis in pediatric patients: current perspectives. Pediatric Health Med Ther. (2023) 14:89–98. doi: 10.2147/PHMT.S379862
79. Zhao, H, Yang, CE, Liu, T, Zhang, MX, Niu, Y, Wang, M, et al. The roles of gut microbiota and its metabolites in diabetic nephropathy. Front Microbiol. (2023) 14:1207132. doi: 10.3389/fmicb.2023.1207132
80. Wen, Y, Su, E, Xu, L, Menez, S, Moledina, DG, Obeid, W, et al. Analysis of the human kidney transcriptome and plasma proteome identifies markers of proximal tubule maladaptation to injury. Sci Transl Med. (2023) 15:eade7287. doi: 10.1126/scitranslmed.ade7287
81. Kang, HM, Ahn, SH, Choi, P, Ko, YA, Han, SH, Chinga, F, et al. Defective fatty acid oxidation in renal tubular epithelial cells has a key role in kidney fibrosis development. Nat Med. (2015) 21:37–46. doi: 10.1038/nm.3762
82. Yang, M, Long, D, Hu, L, Zhao, Z, Li, Q, Guo, Y, et al. AIM2 deficiency in B cells ameliorates systemic lupus erythematosus by regulating Blimp-1-Bcl-6 axis-mediated B-cell differentiation. Signal Transduct Target Ther. (2021) 6:341. doi: 10.1038/s41392-021-00725-x
83. Wang, YM, Shaw, K, Zhang, GY, Chung, EYM, Hu, M, Cao, Q, et al. Interleukin-33 exacerbates IgA glomerulonephritis in transgenic mice overexpressing B cell activating factor. J Am Soc Nephrol. (2022) 33:966–84. doi: 10.1681/ASN.2021081145
84. Sallustio, F, Serino, G, Cox, SN, Dalla Gassa, A, Curci, C, De Palma, G, et al. Aberrantly methylated DNA regions lead to low activation of CD4+ T-cells in IgA nephropathy. Clin Sci (Lond). (2016) 130:733–46. doi: 10.1042/CS20150711
85. Bai, L, Li, H, Li, J, Song, J, Zhou, Y, Liu, B, et al. Immunosuppressive effect of artemisinin and hydroxychloroquine combination therapy on IgA nephropathy via regulating the differentiation of CD4+ T cell subsets in rats. Int Immunopharmacol. (2019) 70:313–23. doi: 10.1016/j.intimp.2019.02.056
86. Caielli, S, Veiga, DT, Balasubramanian, P, Athale, S, Domic, B, Murat, E, et al. A CD4(+) T cell population expanded in lupus blood provides B cell help through interleukin-10 and succinate. Nat Med. (2019) 25:75–81. doi: 10.1038/s41591-018-0254-9
87. Rupanagudi, KV, Kulkarni, OP, Lichtnekert, J, Darisipudi, MN, Mulay, SR, Schott, B, et al. Cathepsin S inhibition suppresses systemic lupus erythematosus and lupus nephritis because cathepsin S is essential for MHC class II-mediated CD4 T cell and B cell priming. Ann Rheum Dis. (2015) 74:452–63. doi: 10.1136/annrheumdis-2013-203717
Keywords: plasma circulating metabolites, nephritis, two-sample Mendelian randomization, therapeutic agents, bioinformatics
Citation: Shao F, Yao Y, Weng D, Wang R, Liu R, Zhang Y, Li E, Wang M, Tang Y, Ding Y and Xie Y (2024) Causal association of plasma circulating metabolites with nephritis: a Mendelian randomization study. Front. Nutr. 11:1364841. doi: 10.3389/fnut.2024.1364841
Received: 03 January 2024; Accepted: 16 April 2024;
Published: 03 May 2024.
Edited by:
Giuseppe Murdaca, University of Genoa, ItalyReviewed by:
Mariia Ivanova, IEO, European Institute of Oncology IRCCS, ItalyCopyright © 2024 Shao, Yao, Weng, Wang, Liu, Zhang, Li, Wang, Tang, Ding and Xie. This is an open-access article distributed under the terms of the Creative Commons Attribution License (CC BY). The use, distribution or reproduction in other forums is permitted, provided the original author(s) and the copyright owner(s) are credited and that the original publication in this journal is cited, in accordance with accepted academic practice. No use, distribution or reproduction is permitted which does not comply with these terms.
*Correspondence: Yuewu Tang, MTM4OTYyNjA2MzZAMTYzLmNvbQ==; Yubin Ding, ZGluZ3liQGNxbXUuZWR1LmNu; Yajun Xie, eWp4aWVAY3FtdS5lZHUuY24=
†These authors have contributed equally to this work and share first authorship
Disclaimer: All claims expressed in this article are solely those of the authors and do not necessarily represent those of their affiliated organizations, or those of the publisher, the editors and the reviewers. Any product that may be evaluated in this article or claim that may be made by its manufacturer is not guaranteed or endorsed by the publisher.
Research integrity at Frontiers
Learn more about the work of our research integrity team to safeguard the quality of each article we publish.