- Department of Laboratory Medicine, The First Hospital of Qiqihar, Qiqihar, Heilongjiang, China
Background: The Dietary Inflammation Index (DII) is a tool for evaluating the potential for dietary inflammation, and inflammation is a major cause of exacerbation in chronic kidney disease. Our study aimed to investigate the relationship between DII and albuminuria.
Methods: Data were obtained from the 2005–2018 National Health and Nutrition Examination Survey (NHANES) after excluding pregnant, minors, and missing data of urinary albumin-creatinine ratio (ACR), estimated glomerular filtration rate (eGFR), and DII were enrolled in our study. Albuminuria was defined as ACR > 30 mg/g. DII was calculated and divided into tertiles. After fully adjusted, multivariate logistic regression analysis and subgroup analysis were performed to investigate the association between DII and albuminuria.
Results: A total of 22,607 participants including 2,803 (12.40%) with and 19,804 (87.60%) without albuminuria were enrolled in our study. The albuminuria increased with the increasing DII tertiles (Tertile 1: 10.81%; Tertile 2: 12.41%; Tertile 3:13.97%, P < 0.001). After fully adjusting for covariates, multivariate logistic regression showed that the higher the DII, the greater the odds of albuminuria (OR = 1.19; 95% CI, 1.00–1.41, P < 0.001). Subgroup analysis and interaction test of participants found that the positive correlation between DII and albuminuria was not significantly related to gender, age, BMI, hypertension, diabetes, and eGFR (P for interaction >0.05).
Conclusion: Elevated DII is associated with high odds of albuminuria. Further large-scale prospective studies are still needed to analyze the role of DII in albuminuria.
Introduction
Increased urinary albumin excretion is the most important clinical manifestation of early nephropathy (1). The urinary albumin creatinine ratio (ACR) is a reliable indicator to assess glomerular injury and albuminuria. ACR can be used in the diagnosis of early chronic kidney disease (CKD), diabetic nephropathy, and hypertensive nephropathy (2). Albuminuria was defined as ACR>30 mg/g. Albuminuria is not only an independent predictor of CKD progression but also a target of treatment in CKD therapy (3–5). Studies have found that moderately increased albuminuria (ACR = 30–300mg/g) occurs in 5%-19% of the general population (6). Matsushita et al. (7) found that albuminuria was significantly associated with all-cause mortality and cardiovascular mortality in the general population. Therefore, the public health interventions can be implemented to attenuate CKD by studying the risk factors of albuminuria.
The Dietary Inflammation Index (DII) is used as a standardized scoring tool to assess the impact of diet on inflammation. This index was first calculated by Cavicchia et al. and further updated by Shivappa et al. (8, 9). DII correlates with a variety of inflammatory factors including C-reactive protein (CRP), tumor necrosis factor (TNF)-α, interleukin (IL)-6, IL−1β, IL−4, and IL−10, etc. Therefore, a higher DII represents a pro-inflammatory diet while a negative DII represents an anti-inflammatory diet. DII has been demonstrated to be associated with CKD, rheumatoid arthritis, kidney stones, diabetes, and hyperuricemia (10–14). DII is also associated with lipid metabolism, thyroid function, and sex hormone secretion (15–17). Dyslipidemia due to decreased lipoprotein catabolism in patients with nephrotic syndrome. Parathyroid hormone is elevated in patients with renal insufficiency due to decreased renal excretion of calcium. The reticular band of the adrenal cortex secretes adrenal sex hormones. Thus, DII may affect renal function by regulating lipid metabolism as well as the endocrine system. DII is an independent risk factor for all-cause and cardiovascular mortality (18).
Numerous studies have shown that inflammation leads to a decline in kidney function. CRP is a risk factor for acute kidney injury (AKI) and CKD by leading to the decline of the estimated glomerular filtration rate (eGFR) (19). TNF-α directly affects kidney function by regulating kidney hemodynamics and excretory functions (20). Serum IL-1β levels were significantly higher in patients with albuminuria than without-albuminuria in patients with diabetes, and the concentration and ACR showed a significant negative correlation (21). However, the association between DII and albuminuria has not been clearly articulated. Therefore, our purpose was to explore whether elevated DII is associated with an increased odds of albuminuria by investigating the participants in the National Health and Nutrition Examination Survey (NHANES).
Materials and methods
Survey description
All cross-sectional data for this study were obtained from NHANES, an ongoing nationally representative sample recruited every 2 years by the National Center for Health Statistics (NCHS) through multistratified, multistage probability sampling across the United States to monitor the nutritional status and health risk factors of the civilian population in the non-institutionalized United States (22). Demographic data, dietary data, and health condition data were obtained through in-home interviews; physical and laboratory examinations were obtained through mobile examination centers (MECs) with participants. We retrieved data of demographics data, dietary data, examination data, laboratory data, and questionnaire data. The protocol for the National Health and Nutrition Examination Survey study was approved by the National Center for Health Statistics Research Ethics Review Board (NHANES 2005–2006, NHANES 2007–2008, NHANES 2009–2010: Protocol #2005–06; NHANES 2011–2012, NHANES 2013–2014, NHANES 2015–2016: Protocol #2011–17; NHANES 2017–2018): Protocol #2011–17 (Effective through October 26, 2017); Protocol #2018–01 (Effective beginning October 26, 2017). The valid informed consent was obtained from all participants for NHANES. All the protocols, detailed experimental design, and data are available at: https://www.cdc.gov/nchs/nhanes/. The findings and conclusions in this paper are those of the authors and do not necessarily represent the views of the Research Data Center, the National Center for Health Statistics, or the Centers for Disease Control and Prevention. Data collection for National Center for Health Statistics (NCHS) was approved by the NCHS Research Ethics Review Board. Analysis of de-identified data from the survey is exempt from the federal regulations for the protection of human research participants. Analysis of restricted data through the NCHS Research Data Center is also approved by the NCHS ERB.
We recruited 7 NHANES cycles from 2005 to 2018 to assess the association between DII and albuminuria. A total of 70,190 participants were enrolled in the study at first. We included 22,607 eligible participants in the final study based on exclusion criteria: age < 20 years (n = 30,441); pregnancy was defined by self-report pregnant now (n = 708); missing data ACR (n = 2,506), eGFR was calculated from the data about gender, age, and SCr (n = 1,970), and DII was calculated from 24 h dietary history interview (n = 11,958) (Figure 1).
Sampling weights are usually used to ensure the data enrolled are representative and unbiased when the data from complex investigations. However, adjusting the variables used to calculate sampling weights in regression analyses may lead to excessive adjustment bias and reduce the accuracy of the analysis. Therefore, we determined to demonstrate our results without the sampling weights.
Definition of dietary inflammation index
The DII score was developed by Shivappa et al. (9) to assess the potential level of inflammation from dietary components through dietary intake recorded by participants' 24-h dietary history interviews (data from USDA's Food and Nutrient Database for Dietary Studies). In our study, DII was calculated from the intake of 28 dietary components and nutrients, including protein, carbohydrates, cholesterol, total fat, saturated fat, monounsaturated fatty acids (MUFAs), n-3 fatty acids, polyunsaturated fatty acids (PUFAs), n-6 fatty acids, vitamin A/B12/C/D/E, thiamine (vitamin B1), riboflavin (vitamin B2), folic acid, zinc, selenium, beta-carotene, alcohol, fiber, Fe, Mg, caffeine, niacin, and energy (23). First, the average value of dietary components and nutrients consumed by each participant during the day (midnight to midnight) was subtracted from the global average per capita daily intake of dietary components and nutrients. The resulting value was divided by the standard deviation of the global average per capita daily dietary component or nutrient intake and multiplied by the associated inflammatory effect index. The above calculation results in a Z-score, which is used to express the “standardized global average.” Individual DII scores were obtained by multiplying the total inflammation score for each dietary component or nutrient after converting the Z-scores into percentiles (to reduce the effect of right skew). An elevated DII score indicates an increased pro-inflammatory effect of the diet, while a negative score indicates an anti-inflammatory effect of the diet.
Definition of albuminuria
Data for urinary albumin and urinary creatinine were obtained from the laboratory data of the 2005–2018 NHANES participants tested at MECs. Urinary albumin was measured by a solid-phase fluorescence immunoassay; urinary creatinine was measured by a modified Jaffe kinetic method. All methodological information was obtained from the Laboratory Procedure Manual of NHANES. ACR was calculated by dividing the urine albumin concentration by the urine creatinine concentration. The diagnosis of albuminuria is defined as an ACR >30 mg/g (24). In our study, albuminuria was defined as an outcome variable.
Covariates
We also included covariates that may have influenced the association between DII and albuminuria in participants in the study, including age (years); gender (male/female); race/ethnicity (Mexican America/Other Hispanic/Non-Hispanic White /Non-Hispanic Black/Other); education (less than high school/high school or equivalent/college or above/not recorded); systolic blood pressure (SBP; mmHg); diastolic blood pressure (DBP; mmHg); body mass index was calculated as weight (kilograms) divided by height (m) squared and divided (BMI; kg/m2); waist circumference (cm); hypertension was defined as having been diagnosed by a physician or taking hypertension medication (yes/no); diabetes were defined as self-reported doctor diagnosis of diabetes, HbA1c ≥6.5%, FPG ≥7.0 mmol/L, postprandial 2-h plasma glucose≥11.1 mmol/L from an oral glucose tolerance test or use of insulin or oral hypoglycemic medication (yes/no); smoking status (current/former/never/not recorded); serum albumin (ALB; g/L); serum Blood Urea Nitrogen (BUN; mmol/L); serum Uric Acid (UA; μmol/L); alanine transaminase (ALT; U/L); aspartate transaminase (AST; U/L); total cholesterol (mmol/L); high-density cholesterol (HDL-C; mmol/L); and eGFR (ml/min/1.73 m2). The estimated glomerular filtration rate (eGFR; ml/min/1.73 m2) was calculated based on the formula: eGFR = 142*min (standardized Scr/K, 1)α *max (standardized Scr/K, 1)−1.200 *0.9938Age *1.012 [if female] (K = 0.7 (females) or 0.9 (males); α = −0.241 (females) or −0.302 (males)) according to the CKD Epidemiology Collaboration (CKD-EPI) creatinine equation (25). Serum creatinine (SCr; mg/dl) was determined by the Jaffe rate method and calibrated by standardized isotope dilution mass spectrometry (6).
Statistical analysis
Statistical analysis was performed using R version 3.4.3 (http://www.R-project.org, The R Foundation) and Empower software (www.empowerstats.com; X&Y Solutions, Inc., Boston, MA). The enrolled participants were stratified into three groups based on DII tertiles (T1-T3) in the sensitivity analysis. Analysis of variation in baseline characteristics across participants grouped by DII tertiles was performed using linear regression models for continuous variables and the chi-square test for the categorical variables. Continuous variables in the study are presented as mean ± standardized values. Categorical variables are presented as frequencies and percentages. P-values for continuous variables, obtained by Kruskal Wallis test, and if the count variable theoretical number < 10, P-values obtained by Fisher's exact probability test. The P < 0.05 was determined statistically significant. The baseline characteristics across participants to test the relationship between critical variables and albuminuria to target special populations.
To investigate the relationship between DII and albuminuria. We used multifactorial logistic regression to test for an association between DII and albuminuria in three different models. Model 1 was unadjusted for covariates, Model 2 was adjusted for age, gender, race/ethnicity, and Model 3 was further adjusted for education, SBP, DBP, BMI, waist circumference, hypertension, diabetes, smoking status, ALB, BUN, UA, ALT, AST, total cholesterol, HDL-C, and eGFR. We conducted the monofactor analysis to perform the association between each covariate and albuminuria in the fully adjusted models. The OR of albuminuria was each unit increase in continuous variables and compared with the reference group for categorical variables. The smoothed fitted curves to assess the linear relationship between DII and albuminuria in Model 3.eGFR was categorized as < 60, 60–89.9, and ≥90 ml/min/1.73m2, and BMI was categorized as < 25 (normal weight), 25–29.9 (overweight), and ≥30 kg/m2 (obese) in subgroup analysis. By stratification factors: sex (male/female); age (< 60/≥60 years); BMI (< 25/25–29.9/≥30 kg/m2); hypertension (yes/no); diabetes (yes/no); eGFR (< 60/60–89.9/≥90 ml/min/1.73 m2) were subjected to subgroup analysis and interaction tests with stratification factors as potential effect modifiers to test the heterogeneity of associations between subgroups.
Results
Baseline characteristics of participants by DII tertile
Based on inclusion criteria, a total of 22,607 participants from NHANES 2005 to 2018 were enrolled, of whom with an average age of 50.26 ± 17.68 years. The 49.78% participants were male and 50.22% were female. A total 12.40% of the participants were categorized as albuminuria overall. The percentage of albuminuria increased with the DII tertiles (Tertile 1: 10.81%; Tertile 2: 12.41%; Tertile 3: 13.97%; P < 0.001). There were statistically significant differences in age, gender, race, education, SBP, DBP, BMI, waist circumference, hypertension, diabetes, smoking status, serum Albumin, serum Blood Urea Nitrogen, serum Uric Acid, eGFR, ALT, AST, ACR among the DII tertiles (-5.2811–0.8623, 0.8628–2.63771, 2.6378–5.79464). All baseline characteristics were summarized in Table 1.
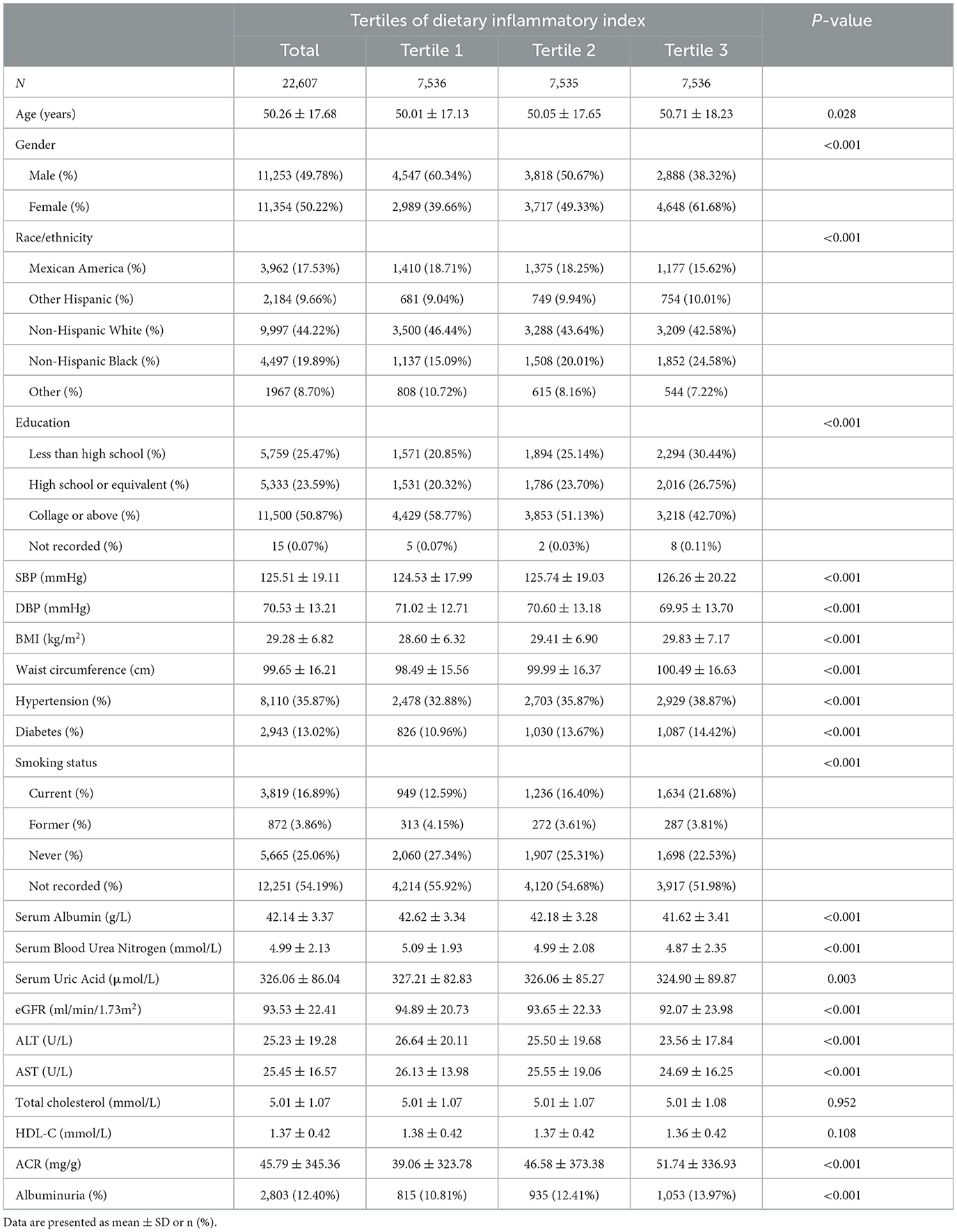
Table 1. Baseline characteristics of study population according to dietary inflammatory index tertiles.
Association of albuminuria with DII
Our results indicated that higher DII was significantly associated with an elevated probability of albuminuria in the crude model (OR = 1.08; 95% CI, 1.05–1.10, P < 0.001), minimally adjusted model (OR = 1.07; 95% CI, 1.05–1.09, P < 0.001), and fully adjusted model (OR = 1.05; 95% CI, 1.02–1.10, P < 0.01). In fully adjusted model, each unit increase in DII was associated with 5% increased odds of albuminuria. We further converted DII from a continuous variable to a categorical variable by tertiles. After full adjustment, the risk of albuminuria in participants was 19% higher and statistically significant in the highest DII tertile compared to the lowest DII tertile (OR = 1.19; 95% CI, 1.00–1.41, P < 0.05). The participants in the medium DII tertile also demonstrated higher odds of albuminuria compared to the lowest DII tertile; however, this correlation was not statistically significant (OR = 1.12; 95% CI, 0.95–1.33, P > 0.05) (Table 2).
In the fully adjusted model, age, race, education, SBP, DBP, BMI, waist circumference, hypertension, diabetes, smoking status, Serum Albumin, Serum Blood Urea Nitrogen, Serum Uric Acid, eGFR, AST, HDL-C associated with the increased odds of albuminuria significantly. The odds of albuminuria increased by 4% for each year of age (P < 0.001); non-Hispanic whites were 12% less likely to have albuminuria compared to Mexican Americans (P < 0.05); the odds of albuminuria decreased by 29% for those with a high school or equivalent compared to those with less than a high school education (P < 0.001), and decreased by 43% for those with a college degree or above (P < 0.001); the odds of albuminuria for the non-hypertension and non-diabetes compared to hypertension and diabetes were 68% (P < 0.001) and 79% (P < 0.001); the odds of albuminuria increased by 2% for each unit increase in BMI and waist circumference (P < 0.001), and increased by 3% for each unit increase in SBP (P < 0.001), decreased by 1% for each unit increase in DBP (P < 0.001). Increased serum albumin, eGFR, and HDL-C reduced the risk of albuminuria, and increased serum blood urea nitrogen, serum uric acid, and AST escalated the odds of albuminuria (Table 3).
Moreover, the smooth curve fitting showed no non-linear relationship between DII and albuminuria in participants (Figure 2).
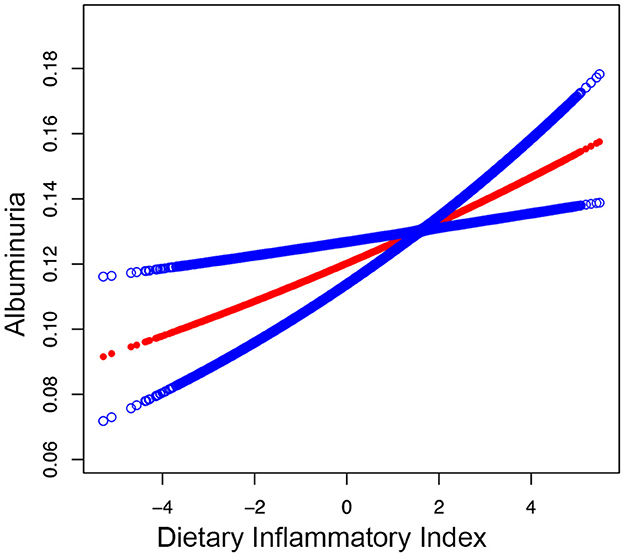
Figure 2. Smooth curve fitting for DII and albuminuria. A linear relationship between DII and albuminuria was detected by the generalized model.
Subgroup analysis
To assess whether the relationship between DII and albuminuria is stable across diverse demographics, we performed a subgroup analysis. The results showed that DII was positively associated with albuminuria across subgroups and was more significant in participants who were male, age ≥ 60, overweight, non-hypertension, and non-diabetes (P < 0.01), however, there was no statistically significant interaction effect between DII and albuminuria across a range of subgroups stratified by gender, age, BMI, diabetes, hypertension, and eGFR (P for interaction > 0.05) (Figure 3).
Discussion
Our study found an association between the higher DII and the increased probability of albuminuria after adjusting for various potential confounders in participants through a cross-sectional study of 70,190 participants from NHANES. The odds of albuminuria had a 5% increase with each unit increase in DII. Subgroup analysis and interaction tests confirmed that the findings are consistent and reliable across the different populations. This is the first study to examine the association between DII and albuminuria and identified the positive correlation between DII and albuminuria. Reducing the degree of dietary inflammation may become an intervention to prevent and alleviate abnormal excretion of albuminuria.
The stable positive correlation between DII and albuminuria was confirmed in a fully adjusted model. In a multifactorial logistic regression model, the effects of BMI and waist circumference were notable. This finding is consistent with the study by Qin et al. (26) on weight-adjusted waist circumference index and increased urinary albumin excretion. Hypertension and diabetes also had significant effect in the fully adjusted model. As Guo et al. (27) found that the relationship between DII and chronic kidney disease in participants with type II diabetes, the ACR was 1.41 times greater in the highest DII group than the lowest (T3 vs. T1). Several randomized controlled trials have also demonstrated a worse prognosis for kidney disease patients with diabetes or hypertension (28, 29). Therefore, kidney disease patients with diabetes or hypertension should be given higher priority. In subgroup analysis, none of the interactions were significant across gender, age, BMI, diabetes, hypertension, and eGFR subgroups, indicating that the association between DII and albuminuria in the general population.
Previous studies have reported an association between DII and a variety of renal diseases including CKD and kidney stones by examining different epidemiologic methods and participants (10, 12, 27, 30). A study based on 21,649 adult Americans reported the odds of prevalent CKD increased from 5.3% in the lowest to 9.3% in the highest DII quartile (31). Research enrolled 1,422 Western Australian women confirmed a higher risk of Kidney Related Diseases in the highest quintile of DII group (32). The all-cause mortality increased from 24.04% in the lowest to 31.23% in the highest DII tertile shown in a report based on 4,554 CKD patients (33). A study from Iowa women renal cancer patients revealed a positive association between higher DII scores and renal cancer risk, although there were no significant interactions (34). However, due to the limited inclusion of data, missing data, and substantial heterogeneity in all the above studies, more large-scale, multicenter studies are still needed in the future.
DII is a calculated value that evaluates the degree of inflammation in a participant's diet. The 28 dietary components and nutrients used to calculate DII have been shown in numerous experiments to correlate with the progression of CKD. A randomized controlled trial found that thiamine helped reduce the degree of albuminuria (35). Increased vitamin D intake has been found to slow down the progression of CKD by modulating inflammatory response (36). CKD patients on hemodialysis who were supplemented with omega-3 fatty acids had significantly lower levels of CRP, IL-6, TNF-alpha, and IL-10/IL-6 ratio, reducing cardiovascular risk (37). However, interestingly a study found that elevated serum MUFAs levels in patients during CKD progression may lead to an increased risk of cardiovascular disease, but dietary MUFAs intake had no effect on serum MUFAs levels (38). Beta-carotene inhibits inflammatory genes for protection against Ang II-induced kidney injury (39). Selenium alleviates acute kidney injury induced by ischemia-reperfusion injury (40). Low intake of fiber and potassium and high intake of sugar and cholesterol contribute to the progression of CKD (41). Based on these experimental results, we believe that it is feasible to investigate the association between DII and albuminuria. The use of DII to assess the dietary inflammation among individuals at-risk for albuminuria may identify potential modifiable dietary factors, whereby decreased DII may decrease the odds of albuminuria and CKD prevalence.
Poorly controlled diabetes can lead to decreased eGFR, hyperalbuminuria, and eventual progression to diabetic nephropathy (42). Patients with essential hypertension are more likely to develop albuminuria than the average subject (43). In our subgroup analysis, the OR values were higher in the subgroups with diabetes and hypertension (Diabetes: OR = 1.01; non-Diabetes: OR = 1.07; Hypertension: OR = 1.02; non-Hypertension OR = 1.10). This may be related to the fact that diabetes patients need to take glucose-lowering drugs, and hypertensive patients need to take antihypertensive drugs for a long period. Many commonly used hypoglycemic and antihypertensive drugs have been shown to act as anti-inflammatory agents through different mechanisms. Metformin may act as an anti-inflammatory agent by inhibiting NLRP3 inflammasome activation and IL-1β production in macrophages, as well as inflammasome-independent IL-6 secretion (44). Empagliflozin attenuates chronic inflammation associated with obesity by reducing plasma TNF-α levels (45). Perindopril prevents sepsis-induced acute kidney injury by reducing plasma TNF-α levels (46). ACEIs and ARBs reduce levels of inflammatory markers including IL-6, TNF-α, and CRP (47).
Strengths of our study included the use of a large representation of a number of specimens and the fact that we adjusted for multiple confounders. For example, eGFR is an important indicator for evaluating renal function and excludes the effects of age, gender, and so on. Therefore, we used eGFR as a covariate in our fully adjusted model, and subgrouped participants according to eGFR to make our study more accurate. Although only 28 dietary components and nutrients were included in our study's calculation of DII, previous studies have confirmed that using 28 of the dietary components and nutrients in the calculation of DII is as effective in predicting dietary validation potential as the larger 45 dietary components and nutrients (48). However, there were also some unavoidable limitations of our study, so the revelations of this study should be interpreted with caution. Firstly, the present study being a cross-sectional study, it was not possible to derive a causal relationship, and secondly, we were unable to include all the covariates affecting the outcome and could not completely exclude the influence of other factors; In addition, the presence of memory bias in the questionnaire data in the NHANES database can lead to some random error; and participants with missing DII data in the database were also prone to missing data on both BMI and waist circumference (33), the two data we included as covariates, and thus it may have caused potentially biased. Recently, related studies have found that the energy-adjusted dietary inflammation index (E-DII) mitigates bias due to differences in energy intake (49). In the future, further inclusion of E-DII and large prospective studies or randomized clinical trials are needed to confirm this result.
Conclusion
Our study demonstrated that elevated DII causing by inappropriate consumption is associated with high odds of albuminuria. While the potential causal relationship between DII and albuminuria needs more investigation. Therefore, controlling DII through dietary modifications may be a novel focus on attenuating the degree of albuminuria.
Data availability statement
The original contributions presented in the study are included in the article/supplementary material, further inquiries can be directed to the corresponding author.
Ethics statement
Ethical review and approval was not required for the study on human participants in accordance with the local legislation and institutional requirements. Written informed consent from the participants was not required to participate in this study in accordance with the national legislation and the institutional requirements.
Author contributions
LD: Validation, Visualization, Writing – original draft, Writing – review & editing, Project administration. HG: Conceptualization, Resources, Supervision, Writing – review & editing. CZ: Investigation, Methodology, Writing – review & editing. BJ: Data curation, Formal analysis, Writing – review & editing. SZ: Data curation, Investigation, Writing – review & editing. JZ: Formal analysis, Software, Writing – review & editing.
Funding
The author(s) declare that no financial support was received for the research, authorship, and/or publication of this article.
Conflict of interest
The authors declare that the research was conducted in the absence of any commercial or financial relationships that could be construed as a potential conflict of interest.
Publisher's note
All claims expressed in this article are solely those of the authors and do not necessarily represent those of their affiliated organizations, or those of the publisher, the editors and the reviewers. Any product that may be evaluated in this article, or claim that may be made by its manufacturer, is not guaranteed or endorsed by the publisher.
Abbreviations
DII, Dietary Inflammation Index; NHANES, National Health and Nutrition Examination Survey; ACR, urinary albumin-creatinine ratio; eGFR, estimated glomerular filtration rate; CKD, chronic kidney disease; CRP, C-reactive protein; TNF, tumor necrosis factor; IL, interleukin; AKI, acute kidney injury; NCHS, National Center for Health Statistics; MECs, mobile examination centers; MUFAs, monounsaturated fatty acids; PUFAs, polyunsaturated fatty acids; vitamin B1, thiamine; vitamin B2, riboflavin; SBP, systolic blood pressure; DBP, diastolic blood pressure; BMI, body mass index; ALB, albumin; BUN, Blood Urea Nitrogen; UA, Uric Acid; ALT, alanine transaminase; AST, aspartate transaminase; HDL-C, high-density cholesterol; CKD-EPI, CKD Epidemiology Collaboration; SCr, Serum creatinine; E-DII, energy-adjusted dietary inflammation index.
References
1. Molitoris BA, Sandoval RM, Yadav SPS, Wagner MC. Albumin uptake and processing by the proximal tubule: physiological, pathological, and therapeutic implications. Physiol Rev. (2022) 102:1625–67. doi: 10.1152/physrev.00014.2021
2. Inoue K, Streja E, Tsujimoto T, Kobayashi H. Urinary albumin-to-creatinine ratio within normal range and all-cause or cardiovascular mortality among US adults enrolled in the NHANES during 1999–2015. Ann Epidemiol. (2021) 55:15–23. doi: 10.1016/j.annepidem.2020.12.004
3. Iseki K, Tokashiki K, Iseki C, Kohagura K, Kinjo K, Takishita S. Proteinuria and decreased body mass index as a significant risk factor in developing end-stage renal disease. Clin Exp Nephrol. (2008) 12:363–9. doi: 10.1007/s10157-008-0061-6
4. De Zeeuw D. Albuminuria not only a cardiovascular/renal risk marker, but also a target for treatment? Kidney Int. (2004) 66:S2–6. doi: 10.1111/j.1523-1755.2004.09201.x
5. Heerspink HJL, Greene T, Tighiouart H, Gansevoort RT, Coresh J, Simon AL. Change in albuminuria as a surrogate endpoint for progression of kidney disease: a meta-analysis of treatment effects in randomised clinical trials. Lancet Diabetes Endocrinol. (2019) 7:128–39. doi: 10.1016/S2213-8587(19)30080-4
6. Qin Z, Li H, Wang L, Geng J, Yang Q, Su B, et al. Systemic Immune-Inflammation Index Is Associated With Increased Urinary Albumin Excretion: A Population-Based Study. Front Immunol. (2022) 13:863640. doi: 10.3389/fimmu.2022.863640
7. Matsushita K, van der Velde M, Astor BC, Woodward M, Levey AS, Jong PEde, et al. Association of estimated glomerular filtration rate and albuminuria with all-cause and cardiovascular mortality in general population cohorts: a collaborative meta-analysis. Lancet. (2010) 375:2073–81. doi: 10.1016/S0140-6736(10)60674-5
8. Cavicchia PP, Steck SE, Hurley TG, Hussey JR, Ma Y, Ockene IS, et al. A new dietary inflammatory index predicts interval changes in serum high-sensitivity C-reactive protein. J Nutr. (2009) 139:2365–72. doi: 10.3945/jn.109.114025
9. Shivappa N, Steck SE, Hurley TG, Hussey JR, Hebert JR. Designing and developing a literature-derived, population-based dietary inflammatory index. Public Health Nutr. (2014) 17:1689–96. doi: 10.1017/S1368980013002115
10. Rouhani MH, Najafabadi MM, Surkan PJ, Esmaillzadeh A, Feizi A, Azadbakht L. Dietary inflammatory index and its association with renal function and progression of chronic kidney disease. Clin Nutr ESPEN. (2019) 29:237–41. doi: 10.1016/j.clnesp.2018.09.001
11. King DE, Xiang J. The Dietary Inflammatory Index Is Associated With Diabetes Severity. The J Am Board Family Med. (2019) 32:801–6. doi: 10.3122/jabfm.2019.06.190092
12. Zhang C, Qiu S, Bian H, Tian B, Wang H, Tu X, et al. Association between Dietary Inflammatory Index and kidney stones in US adults: data from the National Health and Nutrition Examination Survey (NHANES) 2007-2016. Public Health Nutr. (2021) 24:6113–21. doi: 10.1017/S1368980021000793
13. Xiang S, Wang Y, Qian S, Li J, Jin Y, Ding X, et al. The association between dietary inflammation index and the risk of rheumatoid arthritis in Americans. Clin Rheumatol. (2022) 41:2647–58. doi: 10.1007/s10067-022-06217-9
14. Tomata Y, Shivappa N, Zhang S, Nurrika D, Tanji F, Sugawara Y, et al. Dietary inflammatory index and disability-free survival in community-dwelling older adults. Nutrients. (2018) 10:1896. doi: 10.3390/nu10121896
15. Ma Y, Li R, Zhan W, Huang X, Zhou Y, Sun Y, et al. Associations between dietary inflammatory index and sex hormones among 6- to 19-year-old children and adolescents in NHANES 2015-2016. Front Endocrinol. (2021) 12:792114. doi: 10.3389/fendo.2021.792114
16. Wang M, Lu X, Zheng X, Liu J. The relationship between dietary inflammatory index values and thyroid function in the US adult population: an analysis of the NHANES 2007-2012 cohort. Immun Inflamm Dis. (2023) 11:e1016. doi: 10.1002/iid3.1016
17. Chen X, Hou C, Yao L, Li J, Gui M, Wang M, et al. Dietary inflammation index is associated with dyslipidemia: evidence from national health and nutrition examination survey, 1999-2019. Lipids Health Dis. (2023) 22:149. doi: 10.1186/s12944-023-01914-z
18. Gao Y, Wang Y, Zhang D, Wu T, Li Q. The Relationship Between Dietary Inflammatory Index and All-Cause, Cardiovascular Disease-Related, and Cancer-Related Mortality. J Multidiscip Healthc. (2023) 16:2543–56. doi: 10.2147/JMDH.S423558
19. Li J, Chen J, Lan HY, Tang Y. Role of C-reactive protein in kidney diseases. Kidney Dis. (2023) 9:73–81. doi: 10.1159/000528693
20. Mehaffey E, Majid DSA. Tumor necrosis factor-α, kidney function, and hypertension. Am J Physiol Renal Physiol. (2017) 313:F1005–f1008. doi: 10.1152/ajprenal.00535.2016
21. Hutton HL, Alikhan MA, Kitching AR. Inflammasomes in the kidney. Exp Suppl. (2018) 108:177–210. doi: 10.1007/978-3-319-89390-7_8
22. Curtin LR, Mohadjer LK, Dohrmann SM, Kruszon-Moran D, Mirel LB, Carroll MD, et al. National health and nutrition examination survey: sample design, 2007-2010. Vital Health Stat. (2013) 2:1–23.
23. Liu H, Tan X, Liu Z, Ma X, Zheng Y, Zhu B, et al. Association Between Diet-Related Inflammation and COPD: findings From NHANES III. Front Nutr. (2021) 8:732099. doi: 10.3389/fnut.2021.732099
24. Cho YT, Chen CW, Chen MP, Hu JL, Su H, Shiea J, et al. Diagnosis of albuminuria by tryptic digestion and matrix-assisted laser desorption ionization/time-of-flight mass spectrometry. Clin Chim Acta. (2013) 420:76–81. doi: 10.1016/j.cca.2012.12.016
25. Levey AS, Stevens LA, Schmid CH, Zhang Y, Castro III AF, Feldman HI, et al. new equation to estimate glomerular filtration rate. Ann Intern Med. (2009) 150:604–12. doi: 10.7326/0003-4819-150-9-200905050-00006
26. Qin Z, Chang K, Yang Q, Yu Q, Liao R, Su B. The association between weight-adjusted-waist index and increased urinary albumin excretion in adults: A population-based study. Front Nutr. (2022) 9:941926. doi: 10.3389/fnut.2022.941926
27. Guo C, Lin Y, Wu S, Li H, Wu M, Wang F. Association of the dietary inflammation index (DII) with the prevalence of chronic kidney disease in patients with type-2 diabetes mellitus. Ren Fail. (2023) 45:2277828. doi: 10.1080/0886022X.2023.2277828
28. Look AHEAD Research Group. Effect of a long-term behavioural weight loss intervention on nephropathy in overweight or obese adults with type 2 diabetes: a secondary analysis of the Look AHEAD randomised clinical trial. The Lancet Diab Endocrinol. (2014) 2:801–09. doi: 10.1016/S2213-8587(14)70156-1
29. Mahfoud F, Kandzari DE, Kario K, Townsend RR, Weber MA, Schmieder RE, Böhm M. Long-term efficacy and safety of renal denervation in the presence of antihypertensive drugs (SPYRAL HTN-ON MED): a randomised, sham-controlled trial. The Lancet. (2022) 399:1401–10. doi: 10.1016/S0140-6736(22)00455-X
30. Moludi J, Fateh HL, Pasdar Y, Moradinazar M, Sheikhi L, Saber A, et al. Association of dietary inflammatory index with chronic kidney disease and kidney stones in Iranian adults: a cross-sectional study within the Ravansar non-communicable diseases cohort. Front Nutr. (2022) 9:955562. doi: 10.3389/fnut.2022.955562
31. Mazidi M, Shivappa N, Wirth MD, Hebert JR, Kengne AP. Greater dietary inflammatory index score is associated with higher likelihood of chronic kidney disease. Br J Nutr. (2018) 120:204–9. doi: 10.1017/S0007114518001071
32. Bondonno NP, Blekkenhorst LC, Bird AL, Lewis JR, Hodgson JM, Shivappa N, et al. Dietary inflammatory index and the aging kidney in older women: a 10-year prospective cohort study. Eur J Nutr. (2020) 59:3201–11. doi: 10.1007/s00394-019-02160-9
33. Yan LJ, Zhang FR, Ma CS, Zheng Y. Higher dietary inflammatory index is associated with increased all-cause mortality in adults with chronic kidney disease. Front Nutr. (2022) 9:883838. doi: 10.3389/fnut.2022.883838
34. Shivappa N, Blair CK, Prizment AE, Jacobs DR, Hébert JR. Dietary inflammatory index and risk of renal cancer in the Iowa Women's Health Study. Eur J Nutr. (2018) 57:1207–13. doi: 10.1007/s00394-017-1403-5
35. Raval AD, Thakker D, Rangoonwala AN, Gor D, Walia R. Vitamin B and its derivatives for diabetic kidney disease. Cochrane Datab Syst Rev. (2015) 1:1–14. doi: 10.1002/14651858.CD009403.pub2
36. Shroff R, Wan M, Rees L. Can vitamin D slow down the progression of chronic kidney disease?. Pediatric Nephrol. (2012) 27:2167–73. doi: 10.1007/s00467-011-2071-y
37. Valle Flores JA, Fariño Cortéz JE, Mayner Tresol GA, Perozo Romero J, Blasco Carlos M, Nestares T. Oral supplementation with omega-3 fatty acids and inflammation markers in patients with chronic kidney disease in hemodialysis. Appl Physiol Nutr Metab. (2020) 45:805–11. doi: 10.1139/apnm-2019-0729
38. Mika A, Sikorska-Wiśniewska M, Małgorzewicz S, Stepnowski P, Debska-Slizień A, Sledziński T, et al. Potential contribution of monounsaturated fatty acids to cardiovascular risk in chronic kidney disease. Pol Arch Intern Med. (2018) 128:755–63. doi: 10.20452/pamw.4376
39. Kaliappan G, Nagarajan P, Moorthy R, Kalai Gana Selvi S, Avinash Raj T, Mahesh Kumar J. Ang II induce kidney damage by recruiting inflammatory cells and up regulates PPAR gamma and Renin 1 gene: effect of β carotene on chronic renal damage. J Thromb Thrombolysis. (2013) 36:277–85. doi: 10.1007/s11239-012-0835-2
40. Wang S, Chen Y, Han S, Liu Y, Gao J, Huang Y, et al. Selenium nanoparticles alleviate ischemia reperfusion injury-induced acute kidney injury by modulating GPx-1/NLRP3/Caspase-1 pathway. Theranostics. (2022) 12:3882–95. doi: 10.7150/thno.70830
41. Kaesler N, Baid-Agrawal S, Grams S, Nadal J, Schmid M, Schneider MP, et al. Low adherence to CKD-specific dietary recommendations associates with impaired kidney function, dyslipidemia, and inflammation. Eur J Clin Nutr. (2021) 75:1389–97. doi: 10.1038/s41430-020-00849-3
42. Oshima M, Shimizu M, Yamanouchi M, Toyama T, Hara A, Furuichi K, et al. Trajectories of kidney function in diabetes: a clinicopathological update. Nat Rev Nephrol. (2021) 17:740–50. doi: 10.1038/s41581-021-00462-y
43. Ritz E, Nowicki M, Fliser D, Hörner D, Klimm HP. Proteinuria and hypertension. Kidney Int Suppl. (1994) 47:1–14.
44. Xian H, Liu Y, Rundberg Nilsson A, Gatchalian R, Crother TR, Tourtellotte WG, et al. Metformin inhibition of mitochondrial ATP and DNA synthesis abrogates NLRP3 inflammasome activation and pulmonary inflammation. Immunity. (2021) 54:1463–77.e11. doi: 10.1016/j.immuni.2021.05.004
45. Xu L, Nagata N, Nagashimada M, Zhuge F, Ni Y, Chen G, et al. SGLT2 inhibition by empagliflozin promotes fat utilization and browning and attenuates inflammation and insulin resistance by polarizing M2 macrophages in diet-induced obese mice. EBioMedicine. (2017) 20:137–49. doi: 10.1016/j.ebiom.2017.05.028
46. Kostakoglu U, Mercantepe T, Yilmaz HK, Tumkaya L, Batcik S, Pinarbas E, et al. The protective effects of perindopril against acute kidney damage caused by septic shock. Inflammation. (2021) 44:148–59. doi: 10.1007/s10753-020-01316-8
47. Silva IVG, de Figueiredo RC, Rios DRA. Effect of different classes of antihypertensive drugs on endothelial function and inflammation. Int J Mol Sci. (2019) 20:3458. doi: 10.3390/ijms20143458
48. Li A, Chen Y, Schuller AA, van der Sluis LWM, Tjakkes GE. Dietary inflammatory potential is associated with poor periodontal health: a population -based study. J Clin Periodontol. (2021) 48:907–18. doi: 10.1111/jcpe.13472
Keywords: dietary inflammation index, albuminuria, National Health and Nutrition Examination Survey (NHANES), cross-sectional study, population-based study
Citation: Ding L, Guo H, Zhang C, Jiang B, Zhang S and Zhang J (2024) Association between dietary inflammation index and albuminuria: results from the National Health and Nutrition Examination Survey. Front. Nutr. 11:1361890. doi: 10.3389/fnut.2024.1361890
Received: 27 December 2023; Accepted: 29 March 2024;
Published: 15 April 2024.
Edited by:
Mary S. McCarthy, Madigan Army Medical Center, United StatesReviewed by:
Laura Frank, Alexion Pharmaceuticals, United StatesQuanjun Lyu, The First Affliated Hosptial of Zhengzhou, China
Torfinn Hynnekleiv, Innlandet Hospital Trust, Norway
Copyright © 2024 Ding, Guo, Zhang, Jiang, Zhang and Zhang. This is an open-access article distributed under the terms of the Creative Commons Attribution License (CC BY). The use, distribution or reproduction in other forums is permitted, provided the original author(s) and the copyright owner(s) are credited and that the original publication in this journal is cited, in accordance with accepted academic practice. No use, distribution or reproduction is permitted which does not comply with these terms.
*Correspondence: Ling Ding, 18845146679@163.com