- 1Department of Gastroenterological Surgery, The Affiliated Hospital of Qingdao University, Qingdao, China
- 2Neurology Department, Central Hospital Affiliated to Shandong First Medical University, Jinan, China
- 3Department of Radiation Oncology, The Affiliated Hospital of Qingdao University, Qingdao, China
- 4Institute of Nutrition and Health, College of Public Health, Qingdao University, Qingdao, China
Background: Postoperative complications in adhesive small bowel obstruction (ASBO) significantly escalate healthcare costs and prolong hospital stays. This study endeavors to construct a nomogram that synergizes computed tomography (CT) body composition data with inflammatory-nutritional markers to forecast postoperative complications in ASBO.
Methods: The study’s internal cohort consisted of 190 ASBO patients recruited from October 2017 to November 2021, subsequently partitioned into training (n = 133) and internal validation (n = 57) groups at a 7:3 ratio. An additional external cohort comprised 52 patients. Body composition assessments were conducted at the third lumbar vertebral level utilizing CT images. Baseline characteristics alongside systemic inflammatory responses were meticulously documented. Through univariable and multivariable regression analyses, risk factors pertinent to postoperative complications were identified, culminating in the creation of a predictive nomogram. The nomogram’s precision was appraised using the concordance index (C-index) and the area under the receiver operating characteristic (ROC) curve.
Results: Postoperative complications were observed in 65 (48.87%), 26 (45.61%), and 22 (42.31%) patients across the three cohorts, respectively. Multivariate analysis revealed that nutrition risk score (NRS), intestinal strangulation, skeletal muscle index (SMI), subcutaneous fat index (SFI), neutrophil-lymphocyte ratio (NLR), and lymphocyte-monocyte ratio (LMR) were independently predictive of postoperative complications. These preoperative indicators were integral to the nomogram’s formulation. The model, amalgamating body composition and inflammatory-nutritional indices, demonstrated superior performance: the internal training set exhibited a 0.878 AUC (95% CI, 0.802–0.954), 0.755 accuracy, and 0.625 sensitivity; the internal validation set displayed a 0.831 AUC (95% CI, 0.675–0.986), 0.818 accuracy, and 0.812 sensitivity. In the external cohort, the model yielded an AUC of 0.886 (95% CI, 0.799–0.974), 0.808 accuracy, and 0.909 sensitivity. Calibration curves affirmed a strong concordance between predicted outcomes and actual events. Decision curve analysis substantiated that the model could confer benefits on patients with ASBO.
Conclusion: A rigorously developed and validated nomogram that incorporates body composition and inflammatory-nutritional indices proves to be a valuable tool for anticipating postoperative complications in ASBO patients, thus facilitating enhanced clinical decision-making.
Introduction
Adhesive small bowel obstruction (ASBO) ranks as a leading cause of emergency hospital admissions and surgeries (1). Despite advancements in surgical methods, treatment for ASBO patients may lead to extended hospital stays, increased healthcare costs, and notably high morbidity (48%) and mortality rates (5%) (2, 3). The incidence of postoperative complications significantly impacts patients’ postoperative quality of life, an essential metric in evaluating therapeutic effectiveness. Clavien et al. introduced a surgical complications classification system that aids in accurately assessing outcomes across different treatment approaches (4). While the American College of Surgeons National Surgical Quality Improvement Project (NSQIP) is a prevalent risk prediction tool, its complexity and potential inaccuracies render it less suitable for specific patient populations (5).
To date, no universally accepted risk prediction system exists for ASBO patients. Developing a model to forecast postoperative complications and identify risk factors is crucial. Growing evidence suggests that the prognosis and progression of bowel obstruction are linked not only to bowel dysfunction but also to systemic inflammatory responses (6–8). The persistent obstruction leads to digestive tract dilation, intestinal barrier compromise, microbial translocation, and infiltration of inflammatory cells like neutrophils, lymphocytes, platelets, and monocytes, indicative of inflammatory responses in clinical settings (9, 10). Recent research has explored the connection between patient outcomes and various inflammatory-nutritional scores in small bowel obstruction cases (11, 12). These studies have examined scores such as the neutrophil–lymphocyte ratio (NLR), platelet–lymphocyte ratio (PLR), monocyte–lymphocyte ratio (MLR), the albumin–alkaline phosphatase ratio (ALP), systemic immune-inflammation index (SII), and prognostic nutritional index (PNI). These calculated indicators, including NLR and PLR, have proven more sensitive than singular hematological markers like C-reactive protein (CRP) or lymphocyte count in reflecting the inflammatory response and predicting disease progression (13).
Nutritional status is a critical determinant for ASBO, influencing disease progression and patient prognosis (14, 15). Lee et al. demonstrated that nutritional data, such as body mass index (BMI) and weight loss, are correlated with an increased risk of major complications in ASBO patients (16). However, this study did not provide detailed quantitative insights into nutritional status. The widespread adoption of computed tomography (CT) has advanced body composition research, offering more granular insights than traditional metrics like BMI and weight fluctuation (17). CT-based multiple body composition parameters are usually obtained from the images at the level of third lumbar vertebra (L3), which focus on skeletal muscle and adipose tissue (18). These parameters offer superior informativeness in defining nutrition related disorders such as sarcopenia, visceral obesity and sarcopenic obesity, and are associated with adverse outcomes in several gastrointestinal diseases (19, 20).
Despite numerous studies exploring the links between individual nutritional and inflammatory markers and surgical outcomes, comprehensive research integrating laboratory and CT-derived body composition data for ASBO patients is scant. We conducted a systematic and thorough collection of body composition and systemic inflammatory markers (NLR, PLR, LMR, SII, PNI) data to explore their associations. Furthermore, a nomogram was developed to ascertain their predictive capacity for postoperative complications in ASBO patients.
Materials and methods
Patients
This study adheres to the principles of the Declaration of Helsinki. We retrospectively reviewed cases of ASBO from October 2017 to November 2021, utilizing our center’s clinicopathologic database. The inclusion criteria were: (1) diagnosis of ASBO based on clinical or radiological evidence; (2) undergoing emergent surgery due to ASBO; (3) availability of abdominal CT scans and comprehensive hematological indices during hospitalization preoperatively. Exclusion criteria included: (1) conditions that could affect peripheral blood cell counts, such as autoimmune diseases, leukemia, and other hematological malignancies; (2) small bowel obstruction due to primary tumors, hernias, or inflammatory bowel disease; (3) lack of complete clinical data; and (4) age under 18 years. The participants were subsequently divided into training (n = 133) and internal validation (n = 57) cohorts at a 7:3 ratio. A total of 52 patients were enrolled in the external validation cohort from the Central Hospital Affiliated to Shandong First Medical University between January 2022 and January 2023. Figure 1 illustrates the flowchart of patient selection.
Date collection
This retrospective study extracted basic clinical data, including age, sex, BMI, symptoms, comorbidities, nutritional risk score (NRS), American Society of Anesthesiologists (ASA) score, intraoperative findings, and related laboratory indicators from the de-identified database and electronic medical record system. Inflammatory-nutritional markers were determined as follows: NLR = N/L, PLR = P/L, LMR = L/M; SII=P × N/L; PNI = albumin (g/L) +5 × L (109/L), where N: neutrophil count, L: lymphocyte [109]/L; P: platelet count, M: monocyte count (21, 22).
Evaluation of CT-based body composition
Using the institutional PACS (Picture Archiving and Communication System), postoperative L3 CT images were obtained for each patient. Slicer O Matic software (version 5.0)1 was used for assessing body composition. The CT Hounsfield units (HU) thresholds were set at −190 to −30 for intermuscular and subcutaneous adipose tissue, −150 to −50 for visceral adipose tissue, and −29 to +150 for skeletal muscle area (23). The evaluation areas were delineated by two experienced radiologists who were blinded to the clinical characteristics of the patients. The body composition indexes (cm2/m2), including skeletal muscle index (SMI), subcutaneous fat index (SFI), visceral fat index (VFI), and intermuscular adipose tissue index (IFI), were defined as the body composition area (cm2) by height squared (m2). Figure 2 presents a schematic diagram of the study workflow.
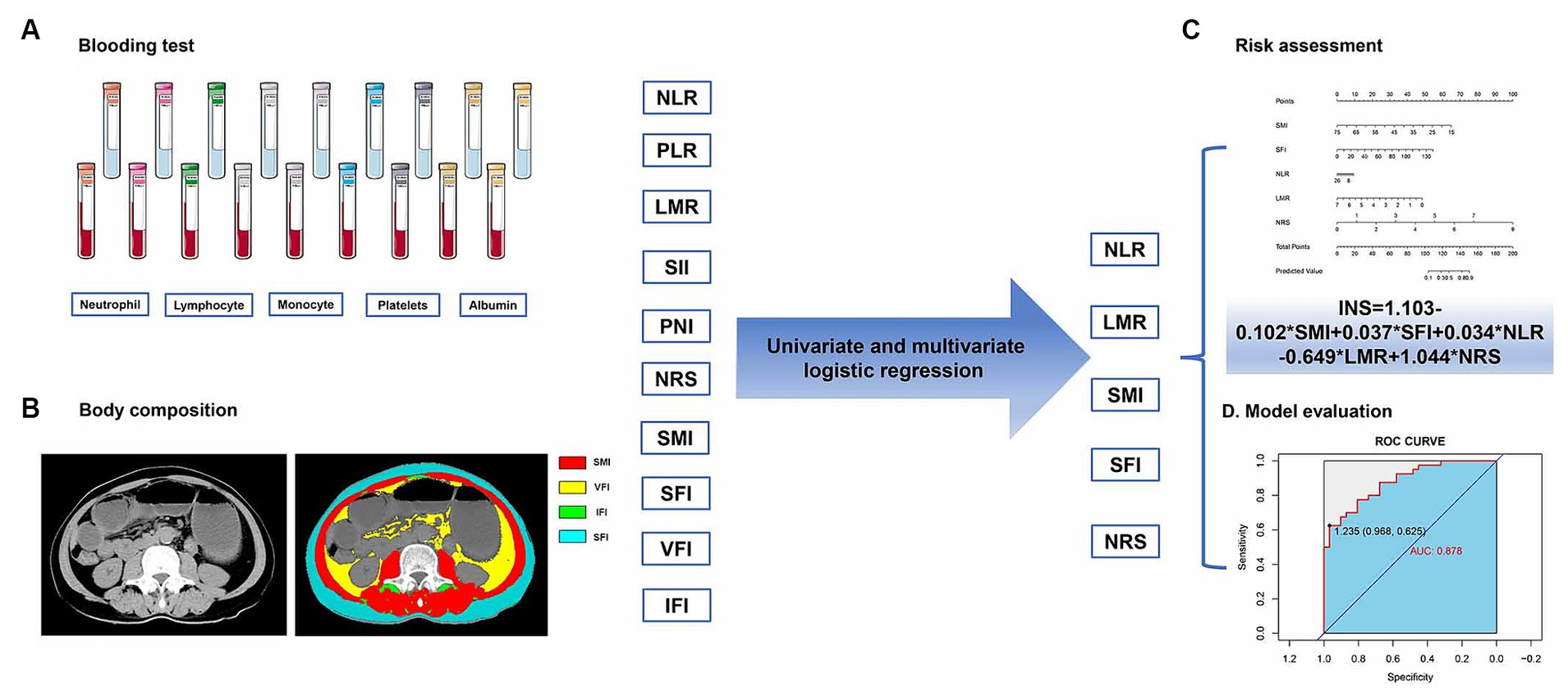
Figure 2. The process of analyzing inflammatory-nutritional markers, from data collection to model creation, involves: (A) blood test, (B) body composition, (C) risk assessment, and (D) model evaluation.
Definitions of postoperative complications
Postoperative complications were classified according to the Clavien–Dindo classification (4). Our analysis focused on complications that occurred within 1 month after the surgical procedure. In cases where a patient experienced multiple complications either simultaneously or sequentially, the most severe complication was selected as the primary outcome for this study.
Statistical analysis
Statistical analysis was conducted using R software version 3.6.32 and SPSS version 25.0. We utilized the Kolmogorov–Smirnov test to assess the normal distribution of texture features. Intergroup categorical variables were examined using Fisher’s exact tests and Chi-square tests, while independent-sample t-tests were applied for continuous variables. The “rms” R package facilitated the generation of ROC curves, areas under the curves (AUCs), a nomogram, and corresponding calibration curves (24, 25). The “rmda” package was employed for decision curve analysis (DCA) (26). A p-value of less than 0.05 was considered statistically significant.
Results
Characteristics of enrolled patients
In total, 190 patients with ASBO were included in the study (96 men and 94 women; average age 62.48 ± 13.50 years). They were randomized into training (n = 133) and internal validation (n = 57) cohorts at a 7:3 ratio. An external validation cohort comprised 52 patients from another center. Basic characteristics of the three cohorts are presented in Table 1. In the training cohort, 65 patients (48.87%; 35 men and 30 women; average age 63.20 ± 13.73 years) experienced complications, compared to 26 patients (45.61%; 15 men and 11 women; average age 67.46 ± 15.85 years) in the internal validation cohort. The external validation cohort included 22 patients (42.31%; 12 men and 10 women; average age 61.23 ± 10.70 years) with complications. Factors such as preoperative infection, ASA score, NRS, intestinal strangulation, CRP, NLR, PLR, LMR, SII, PNI, SMI, and SFI showed a significant correlation with postoperative complications in the training set (p < 0.05).
Overview of complications
The incidence of complications across different grades did not significantly differ among the three cohorts (p > 0.05) (Table 2). There were 37 (27.82%), 14 (24.56%), and 13 (25%) patients who experienced severe complications (Grade III or higher) in training, internal validation, and external validation cohorts, demonstrating comparable rates of severe complications.
Univariate and multivariate analysis of inflammatory-nutritional markers
Table 3 indicates significant differences between the two groups in preoperative infection, ASA score, NRS, intestinal strangulation, CRP, NLR, PLR, LMR, SII, PNI, SMI, and SFI, as identified by univariate regression in the training cohort (p < 0.05). These variables with p-values less than 0.05 were subsequently included in the multivariate regression analysis. Our findings reveal that NRS (OR = 21.731, p = 0.002), intestinal strangulation (OR = 401.665, p = 0.008), NLR (OR = 4.264, p = 0.029), LMR (OR = 0.183, p = 0.034), SMI (OR = 0.708, p = 0.008), and SFI (OR = 1.115, p = 0.014) are independent predictors of postoperative complications.
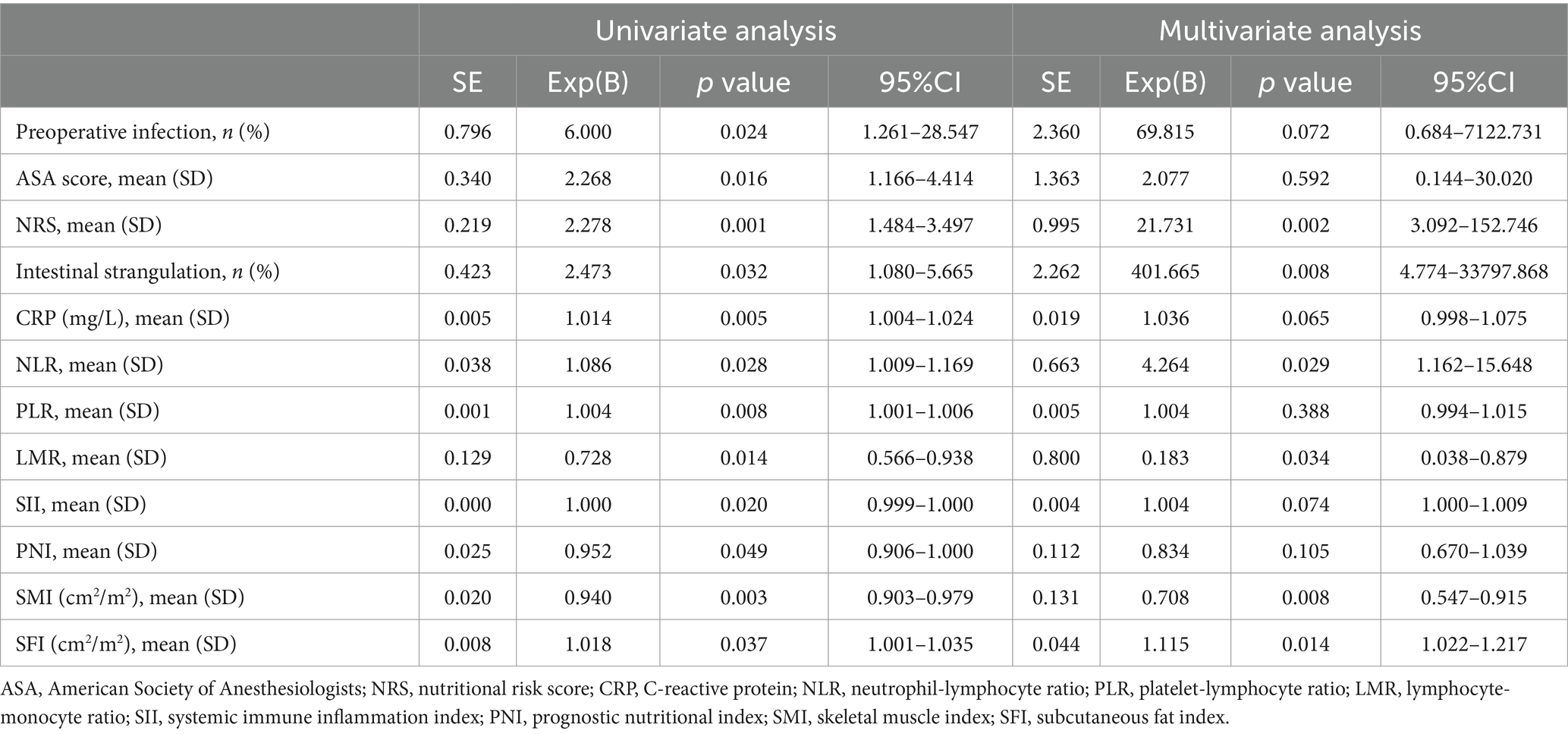
Table 3. Univariate and multivariate analysis of patients with complications versus those without in the training set.
Inflammatory-nutritional model construction and verification
Figure 3 presents a correlation matrix of inflammatory-nutritional biomarkers, with correlation coefficients ranging from −1 (red) to 1 (blue) in training and validation sets. Hemoglobin (HB) was found to be correlated with SMI in both the training [Pearson Correlation Coefficient (PCC) = 0.196, p = 0.023] and internal validation sets (PCC = 0.348, p = 0.008), as shown in Supplementary Tables S1–S3. To avoid multicollinearity, the inflammatory-nutritional model was constructed using indicators with a PCC below 0.7 (27).
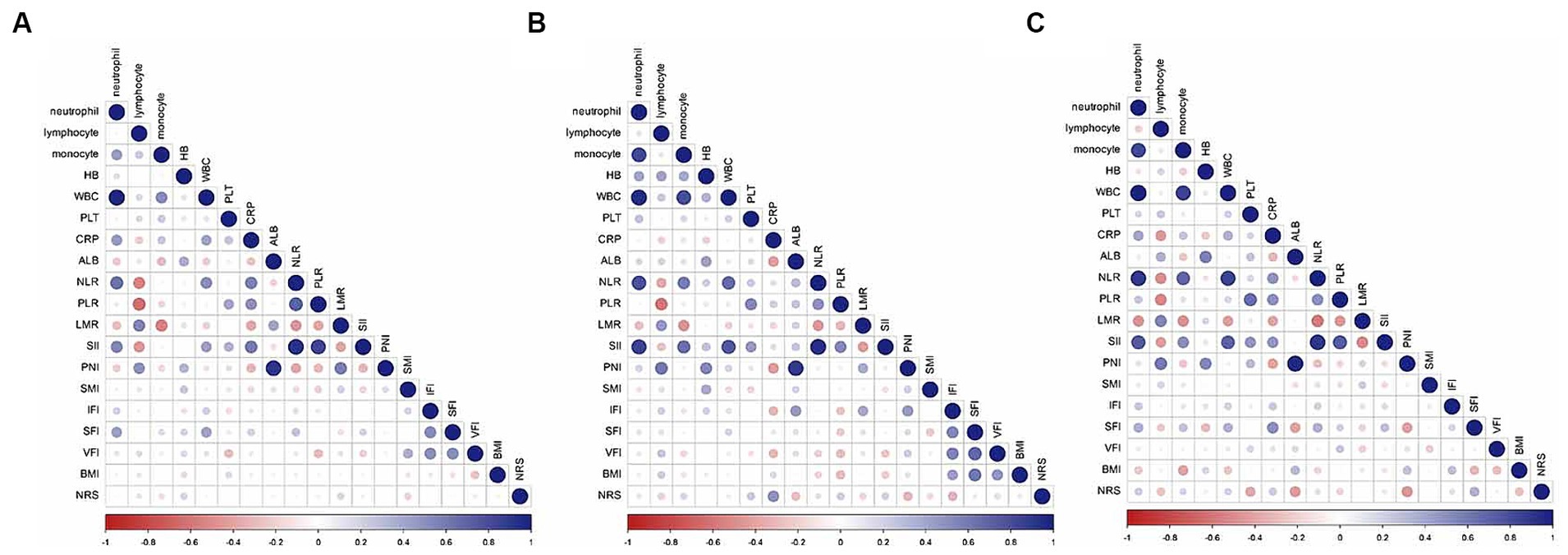
Figure 3. Correlation matrix of nutritional inflammatory biomarkers in the training (A), internal validation (B), and external validation (C) sets.
A nomogram derived from the multivariate analysis was developed, incorporating NRS, NLR, PLR, SMI, and SFI. Each patient’s total score was calculated by summing the scores of these five predictive factors, which were then used to evaluate the risk of postoperative complications. A higher total score correlated positively with an increased probability of postoperative complications (Figure 4A). The inflammatory-nutritional score (INS) was calculated as 1.103–0.102*SMI + 0.037*SFI + 0.034*NLR-0.649*LMR + 1.044*NRS. Calibration curves demonstrated good agreement in three cohorts (Figures 4B–D). Validation was conducted using the bootstrap method, and model performance was assessed over 1,000 iterations.
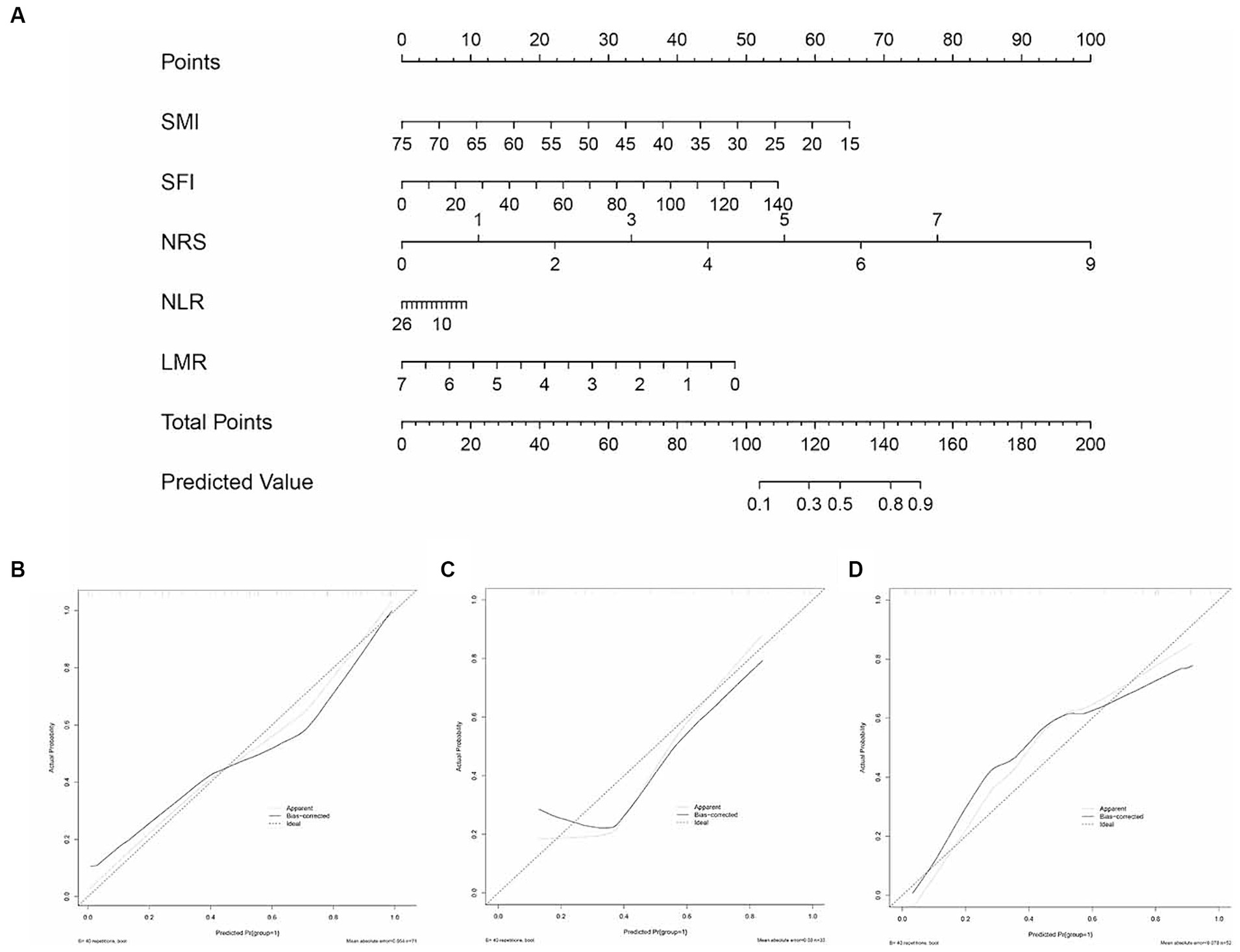
Figure 4. The inflammatory-nutrition nomogram and its calibration curves. (A) Development of the inflammatory-nutrition nomogram in the training set, incorporating SMI, SFI, NLR, PLR, and NRS. Calibration curves for the nomogram in the training (B), internal validation (C), and external validation (D) sets.
Evaluating predictive performance of the three models
Based on inflammatory-nutritional markers, we established an inflammatory model (NLR, PLR, LMR, SII, PNI) and nutritional model (SMI, IFI, SFI, VFI). ROC analysis revealed that the nomogram achieved AUCs of 0.878 (95% CI, 0.802–0.954) in the training set, 0.831 (95% CI, 0.675–0.986) in the internal validation set, and 0.886 (95% CI, 0.799–0.974) in the external validation set. These results surpassed those of the inflammatory model (0.648, 95% CI 0.554–0.742) and the nutritional model (0.674, 95% CI 0.583–0.766) in the training set, 0.655 (95% CI 0.508–0.802) and 0.766 (95% CI 0.642–0.889) in the internal validation set, and 0.814 (95% CI 0.695–0.933) and 0.811 (95% CI 0.689–0.932) respectively (Figures 5A–C) in the external validation set. Decision curve analysis (DCA) indicated that our nomogram achieved greater net benefits at optimal threshold probabilities in predicting complications in ASBO cases (Figures 5D–F). Table 4 details the predictive performance of the inflammatory model, nutritional model, and nomogram in three cohorts.
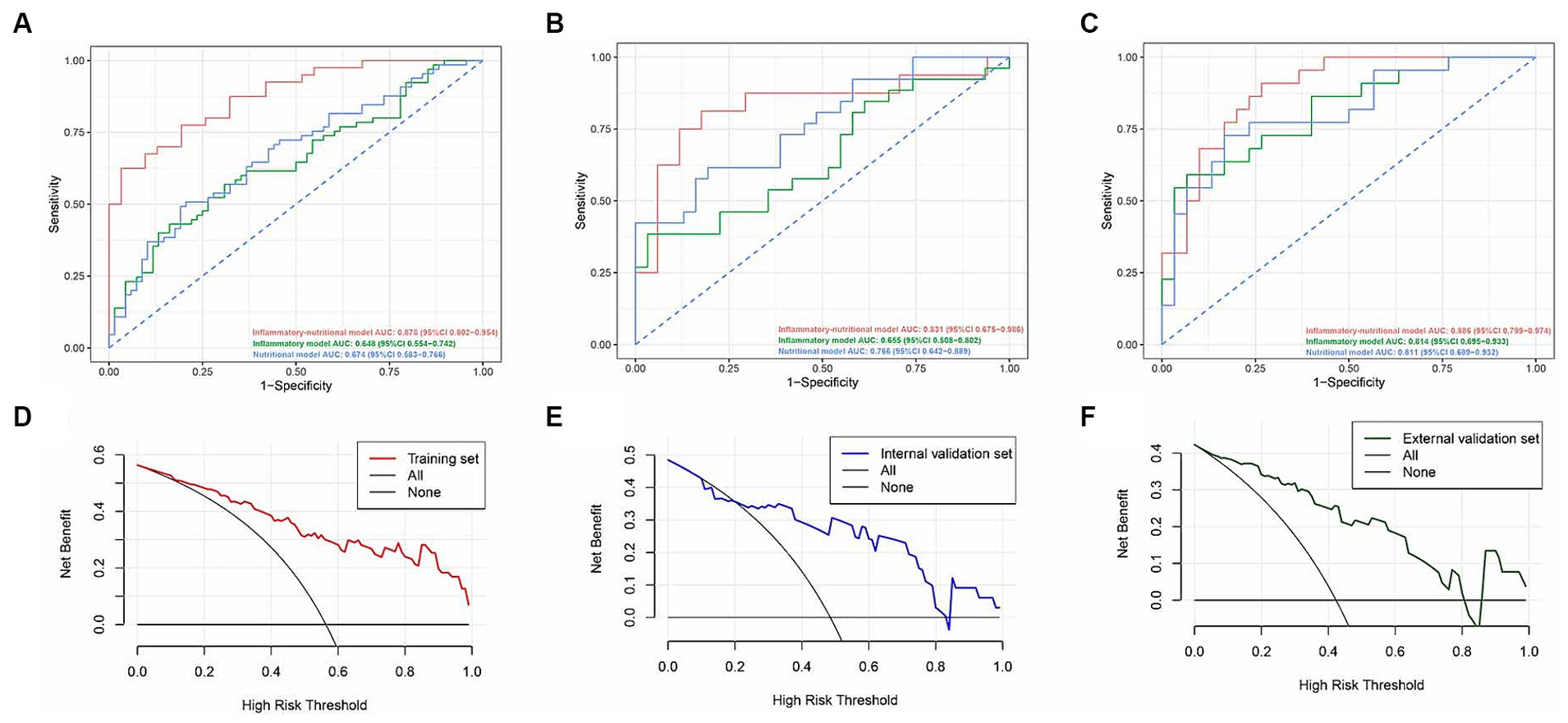
Figure 5. Receiver operating characteristic curves of the inflammatory-nutrition model, the inflammatory model, and the nutritional model in the training (A), internal validation (B), and external validation (C) sets. Decision curve analysis for the inflammatory-nutrition model in the training (D), internal validation (E), and external validation (F) sets.
Discussion
The choice between conservative management and surgical intervention for ASBO patients continues to be debated. Previous research indicated that the method of treatment correlates with postoperative complications in ASBO patients (28, 29). In our study, 113 of the 242 ASBO patients (46.69%) encountered complications (30). This significant rate of postoperative complications adversely affects ASBO patient outcomes, underscoring the necessity for a predictive model to foresee these complications and assist in clinical decision-making (31). Our current research, involving 242 ASBO patients, validated the efficacy of a nomogram that incorporates CT-based body composition and inflammatory-nutritional markers. This model, easy to compute, holds broad applicability in clinical practice.
Increasing evidence suggests that poor nutritional status is a prognostic risk factor for various gastrointestinal disorders, encompassing both malignancies and benign conditions (14, 32, 33). In ASBO cases, impaired intestinal function curtails the efficacy of standard enteral interventions in swiftly rectifying malnutrition, elevating the risk of severe malnutrition and impeding potential enhancements in nutritional status due to acute gastrointestinal failure (34). Consequently, clinicians are in pursuit of reliable indicators to accurately evaluate the nutritional status of ASBO patients (35). Although traditional nutritional assessment tools like body weight and BMI offer a general insight into an individual’s nutritional status, they do not provide specific details on body composition, such as muscle mass or regional fat distribution. Recent research has increasingly acknowledged the pivotal role of body composition in determining a patient’s nutritional state and postoperative outcomes (36, 37). Our study also showed that the SMIs of ASBO patients with complications were significantly lower than those of patients without complications (p < 0.05), and multivariable analysis confirmed this protective factor (OR = 0.708). This association indicated the importance of maintaining skeletal muscle mass quality for postoperative recovery of ASBO patients (38). Patients at a heightened risk of sarcopenia may undergo a persistent inflammatory response that disrupts normal nitrogen metabolism, increasing the likelihood of postoperative complications. This is consistent with prior findings that surgical patients with reduced skeletal muscle mass have poorer prognoses, encounter more postoperative complications, require more intensive care, and exhibit higher mortality rates (39, 40). Furthermore, our observations indicate that a high SFI correlates with postoperative complications in ASBO patients, adding to the discourse on the “obesity paradox” and supporting the notion that sarcopenic obesity is as indicative of surgical outcomes as sarcopenia alone, as several studies have previously reported (41, 42).
ASBO is often associated with acute inflammatory process, a key marker of disease progression. Mueller et al. discovered that as obstruction advances and intestinal barrier dysfunction ensues, luminal flora can penetrate the mucosal layer, triggering host immune responses (43). This uncontrolled inflammatory response is characterized by immune cells infiltration and the release of inflammatory mediators. Numerous systemic inflammatory response indicators, derived from serum biomarkers, have been developed to assess the extent of this response. In our study, systemic inflammatory response indicators, including CRP, PLR, NLR, LMR, PNI and SII, were examined. We found that patients with higher NLR and lower LMR values were more likely to experience postoperative complications, corroborating previous findings that NLR and LMR are crucial indicators for predicting disease severity and prognosis in cancer patients (12, 44, 45). NLR and LMR calculations involve neutrophils, lymphocytes, and monocytes. Changes in NLR and LMR values generally reflect disturbances in these immune cell types and their prognostic significance, linked to the effects of such cells. Neutrophil and monocyte activation, a response to infection signals, is a fundamental component of the innate immune response phase and is implicated in the pathogenesis of various diseases (46). A decrease in lymphocyte count, indicating impaired immune function, hampers the body’s ability to combat persistent infections (11, 47, 48). In recent years, the interplay between inflammation and nutrition has gained significant attention. The volume of research examining the impact of nutrition on the immune system is continuously expanding (49, 50). On correlation analysis, we also found HB and SMI were positively correlated (Supplementary Table S1) which implied the underlying mechanistic association between anemia and sarcopenia in ASBO settings. Consistent with the findings of Hirani’s study, lower HB levels might contribute to a decrease in skeletal muscle volume, through biological pathways generally involving decreased oxygenation of skeletal muscle tissues (51). Anemia caused by ASBO may impair oxygen delivery and expenditure within muscle tissues, creating hypoxia within the local microenvironment that undermines the function of skeletal muscle cells, ultimately leading to skeletal muscle loss and sarcopenia.
The findings from our multivariate and correlation analyses have paved the way for the development of a practical predictive model for postoperative complications in ASBO patients. This model aims to identify those at heightened risk for such complications. While most previous studies have focused on the predictive power of single inflammatory–nutritional scores or CT composition indices on prognosis (45, 52), these singular measures often fail to provide a comprehensive and accurate representation of a patient’s entire inflammatory-nutritional status, thereby limiting their practical accuracy (21). Recently, there has been a trend toward developing prognostic scores based on multiple inflammatory-nutrition indices. For instance, Wang et al. created a prognostic score incorporating LMR, NLR, and PLR to predict outcomes for gastrectomy patients’ post-chemotherapy, with their nomogram demonstrating superior predictive performance (C-index 0.707) compared to single-index models (53). Our previous research utilized multiple inflammatory-nutritional scores to construct a model for predicting postoperative quality of life in gastric cancer patients (22). In this study, we initially attempted to create an inflammation-based model (Inflam-model) using inflammatory scores (Inflam-scores) and a radiography-based model (Radio-model) using body composition parameters. However, both models exhibited suboptimal performance. Consequently, we explored whether combining various inflammatory factors with nutrition-related indicators could enhance the predictive accuracy for ASBO patients. We selected significant inflammatory-nutritional and radiographic indicators from the multivariable analysis to construct a combined predictive model. Our risk predictive model, integrating two Inflam-scores, two Radio-scores, and NRS, showed improved performance in both cohorts. These Radio-scores and Inflam-scores, derived from routine clinical practice, make this multiparametric model practical, particularly in preoperative settings. Identifying patients with a high inflammatory state and low nutritional status preoperatively is crucial in clinical practice. Accordingly, prognosis may be improved through prompt and effective therapeutic interventions.
This study has several limitations. Firstly, the sample size was comparatively small, indicating the necessity for subsequent multicenter studies with expanded sample sizes. Secondly, the expansion of the intestine lumen within the abdominal cavity may affect the accuracy of visceral adipose tissue detection on CT images. Relying solely on measurements of visceral adipose tissue in single CT slides may not sufficiently predict postoperative outcomes. Dynamic and comprehensive assessments of whole-body composition warrant further study. Thirdly, although the correlation between the inflammatory and nutritional factors identified in ultimate model was investigated initially, the causal relationship of these factors is unknown, which might have impact on clinical management. Some promising new statistical methods could assist in quantifying robustness of causal inferences in future research (54).
Conclusion
In summary, we have developed and validated a nomogram that incorporates CT body composition data and inflammatory–nutritional scores to predict postoperative complications in patients with ASBO. Given its usability and the positive results achieved in our initial cohort, this model demonstrates potential as an effective tool for guiding nutritional treatment and decision-making in ASBO cases in future clinical settings.
Data availability statement
The original contributions presented in the study are included in the article/Supplementary material, further inquiries can be directed to the corresponding authors.
Author contributions
ZW: Data curation, Writing – original draft. BS: Formal analysis, Methodology, Writing – original draft. YY: Formal analysis, Writing – original draft. JL: Validation, Writing – original draft. DL: Formal analysis, Writing – review & editing. YL: Writing – review & editing. RL: Funding acquisition, Writing – review & editing.
Ethics statement
The studies involving humans were approved by The Affiliated Hospital of Qingdao University. The studies were conducted in accordance with the local legislation and institutional requirements. Written informed consent for participation was not required from the participants or the participants’ legal guardians/next of kin in accordance with the national legislation and institutional requirements.
Funding
The author(s) declare financial support was received for the research, authorship, and/or publication of this article. This research was supported by the National Natural Science Foundation of China (82000482) and Beijing Xisike Clinical Oncology Research Foundation (Y-NESTLE2022QN-0230).
Conflict of interest
The authors declare that the research was conducted in the absence of any commercial or financial relationships that could be construed as a potential conflict of interest.
Publisher’s note
All claims expressed in this article are solely those of the authors and do not necessarily represent those of their affiliated organizations, or those of the publisher, the editors and the reviewers. Any product that may be evaluated in this article, or claim that may be made by its manufacturer, is not guaranteed or endorsed by the publisher.
Supplementary material
The Supplementary material for this article can be found online at: https://www.frontiersin.org/articles/10.3389/fnut.2024.1345570/full#supplementary-material
Footnotes
References
1. Krielen, P, di Saverio, S, ten Broek, R, Renzi, C, Zago, M, Popivanov, G, et al. Laparoscopic versus open approach for adhesive small bowel obstruction, a systematic review and meta-analysis of short term outcomes. J Trauma Acute Care Surg. (2020) 88:866–74. doi: 10.1097/TA.0000000000002684
2. Sakari, T, Christersson, M, and Karlbom, U. Mechanisms of adhesive small bowel obstruction and outcome of surgery; a population-based study. BMC Surg. (2020) 20:62. doi: 10.1186/s12893-020-00724-9
3. ten Broek, RPG, Krielen, P, di Saverio, S, Coccolini, F, Biffl, WL, Ansaloni, L, et al. Bologna guidelines for diagnosis and management of adhesive small bowel obstruction (ASBO): 2017 update of the evidence-based guidelines from the world society of emergency surgery ASBO working group. World J Emerg Surg. (2018) 13:24. doi: 10.1186/s13017-018-0185-2
4. Clavien, PA, Barkun, J, de Oliveira, ML, Vauthey, JN, Dindo, D, Schulick, RD, et al. The Clavien-Dindo classification of surgical complications: five-year experience. Ann Surg. (2009) 250:187–96. doi: 10.1097/SLA.0b013e3181b13ca2
5. Clark, DE, Fitzgerald, TL, and Dibbins, AW. Procedure-based postoperative risk prediction using NSQIP data. J Surg Res. (2018) 221:322–7. doi: 10.1016/j.jss.2017.09.003
6. Medzhitov, R . Origin and physiological roles of inflammation. Nature. (2008) 454:428–35. doi: 10.1038/nature07201
7. Nellgård, P, and Cassuto, J. Inflammation as a major cause of fluid losses in small-bowel obstruction. Scand J Gastroenterol. (1993) 28:1035–41. doi: 10.3109/00365529309098305
8. Tsumura, H, Ichikawa, T, Hiyama, E, Murakami, Y, and Sueda, T. Systemic inflammatory response syndrome (SIRS) as a predictor of strangulated small bowel obstruction. Hepato-Gastroenterology. (2004) 51:1393–6.
9. Kim, JH, Ha, HK, Kim, JK, Eun, HW, Park, KB, Kim, BS, et al. Usefulness of known computed tomography and clinical criteria for diagnosing strangulation in small-bowel obstruction: analysis of true and false interpretation groups in computed tomography. World J Surg. (2004) 28:63–8. doi: 10.1007/s00268-003-6899-6
10. Scaglione, M, Galluzzo, M, Santucci, D, Trinci, M, Messina, L, Laccetti, E, et al. Small bowel obstruction and intestinal ischemia: emphasizing the role of MDCT in the management decision process. Abdom Radiol (NY). (2022) 47:1541–55. doi: 10.1007/s00261-020-02800-3
11. Yoon, JB, and Lee, SH. The neutrophil-to-lymphocyte ratio has feasible predictive value for hospital mortality in patients with small bowel obstruction in the emergency department. Am J Emerg Med. (2021) 44:428–33. doi: 10.1016/j.ajem.2020.05.049
12. Taşcı, Hİ . The role of neutrophil-to-lymphocyte ratio in predicting disease progression and emergency surgery indication in benign intestinal obstructions. Ulus Travma Acil Cerrahi Derg. (2022) 28:1238–47. doi: 10.14744/tjtes.2022.46944
13. Wyllie, DH, Bowler, IC, and Peto, TE. Relation between lymphopenia and bacteraemia in UK adults with medical emergencies. J Clin Pathol. (2004) 57:950–5. doi: 10.1136/jcp.2004.017335
14. Lee, MJ, Sayers, AE, Drake, TM, Singh, P, Bradburn, M, Wilson, TR, et al. Malnutrition, nutritional interventions and clinical outcomes of patients with acute small bowel obstruction: results from a national, multicentre, prospective audit. BMJ Open. (2019) 9:e029235. doi: 10.1136/bmjopen-2019-029235
15. Lee, MJ, Sayers, AE, Drake, TM, Hollyman, M, Bradburn, M, Hind, D, et al. UK-based, multisite, prospective cohort study of small bowel obstruction in acute surgical services: National Audit of small bowel obstruction (NASBO) protocol. BMJ Open. (2017) 7:e016796. doi: 10.1136/bmjopen-2017-016796
16. Lee, MJ, Sayers, AE, Drake, TM, Marriott, PJ, Anderson, ID, Bach, SP, et al. National prospective cohort study of the burden of acute small bowel obstruction. BJS Open. (2019) 3:354–66. doi: 10.1002/bjs5.50136
17. Kwon, MR, Ko, ES, Park, MS, Jeong, WK, Hwang, NY, Kim, JH, et al. Impact of skeletal muscle loss and visceral obesity measured using serial CT on the prognosis of operable breast cancers in Asian patients. Korean J Radiol. (2022) 23:159–71. doi: 10.3348/kjr.2020.1475
18. Teigen, LM, Kuchnia, AJ, Nagel, E, Deuth, C, Vock, DM, Mulasi, U, et al. Impact of software selection and image J tutorial corrigendum on skeletal muscle measures at the third lumbar vertebra on computed tomography scans in clinical populations. JPEN J Parenter Enteral Nutr. (2018) 42:933–41. doi: 10.1002/jpen.1036
19. Bamba, S, Inatomi, O, Takahashi, K, Morita, Y, Imai, T, Ohno, M, et al. Assessment of body composition from CT images at the level of the third lumbar vertebra in inflammatory bowel disease. Inflamm Bowel Dis. (2021) 27:1435–42. doi: 10.1093/ibd/izaa306
20. Velho, S, Morão, B, Gouveia, C, Agostinho, L, Torres, J, Maio, R, et al. Body composition and Crohn's disease behavior: is adiposity the main game changer? Nutrition. (2023) 108:111959. doi: 10.1016/j.nut.2022.111959
21. Woodford, EP, Woodford, HM, Hort, AR, Pang, TC, Lam, VWT, and Nahm, CB. Neutrophil-lymphocyte ratio and platelet-lymphocyte ratio use in detecting bowel ischaemia in adhesional small bowel obstruction. ANZ J Surg. (2022) 92:2915–20. doi: 10.1111/ans.18073
22. Zhang, S, Liu, R, Zhang, M, Hu, JL, Xiang, S, Jiang, Z, et al. The predictive value of a new inflammatory-nutritional score for quality of life after laparoscopic distal gastrectomy for gastric cancer. Nutr Cancer. (2023) 75:1165–76. doi: 10.1080/01635581.2023.2181732
23. Xiang, S, Yang, YK, Wang, TY, Yang, ZT, Lu, Y, and Liu, SL. Development and validation of a nomogram to predict anastomotic leakage in colorectal cancer based on CT body composition. Front Nutr. (2022) 9:974903. doi: 10.3389/fnut.2022.974903
24. Ohori Tatsuo Gondo And Riu Hamada, M, Gondo, T, and Hamada, R. Nomogram as predictive model in clinical practice. Gan To Kagaku Ryoho. (2009) 36:901–6. Japanese
25. Hajian-Tilaki, K . Receiver operating characteristic (ROC) curve analysis for medical diagnostic test evaluation. Caspian J Intern Med. (2013) 4:627–35.
26. Van Calster, B, Wynants, L, Verbeek, JFM, Verbakel, JY, Christodoulou, E, Vickers, AJ, et al. Reporting and interpreting decision curve analysis: a guide for investigators. Eur Urol. (2018) 74:796–804. doi: 10.1016/j.eururo.2018.08.038
27. Richard, B, and Andrew, F. Regression analysis and linear models: concepts, applications, and implementation. New York, NY: The Guilford Press (2016).
28. Fung, BSC, Behman, R, Nguyen, MA, Nathens, AB, Look Hong, NJ, Pechlivanoglou, P, et al. Longer trials of non-operative management for adhesive small bowel obstruction are associated with increased complications. J Gastrointest Surg. (2020) 24:890–8. doi: 10.1007/s11605-019-04156-6
29. Hackenberg, T, Mentula, P, Leppäniemi, A, and Sallinen, V. Laparoscopic versus open surgery for acute adhesive small-bowel obstruction: a propensity score-matched analysis. Scand J Surg. (2017) 106:28–33. doi: 10.1177/1457496916641341
30. Khaikin, M, Schneidereit, N, Cera, S, Sands, D, Efron, J, Weiss, EG, et al. Laparoscopic vs. open surgery for acute adhesive small-bowel obstruction: patients' outcome and cost-effectiveness. Surg Endosc. (2007) 21:742–6. doi: 10.1007/s00464-007-9212-1
31. McGuire, HH Jr, Horsley, JS 3rd, Salter, DR, and Sobel, M. Measuring and managing quality of surgery. Statistical vs incidental approaches. Arch Surg. (1992) 127:733–7; discussion 738. doi: 10.1001/archsurg.1992.01420060113017
32. Emenaker, NJ, and Vargas, AJ. Nutrition and cancer research: resources for the nutrition and dietetics practitioner. J Acad Nutr Diet. (2018) 118:550–4. doi: 10.1016/j.jand.2017.10.011
33. Roncoroni, L, Gori, R, Elli, L, Tontini, GE, Doneda, L, Norsa, L, et al. Nutrition in patients with inflammatory bowel diseases: a narrative review. Nutrients. (2022) 14:751. doi: 10.3390/nu14040751
34. Vaizey, CJ, Maeda, Y, Barbosa, E, Bozzetti, F, Calvo, J, Irtun, Ø, et al. European Society of Coloproctology consensus on the surgical management of intestinal failure in adults. Color Dis. (2016) 18:535–48. doi: 10.1111/codi.13321
35. Veen, T, Ramanathan, P, Ramsey, L, Dort, J, and Tabello, D. Predictive factors for operative intervention and ideal length of non-operative trial in adhesive small bowel obstruction. Surg Endosc. (2023) 37:8628–35. doi: 10.1007/s00464-023-10282-9
36. Borga, M, West, J, Bell, JD, Harvey, NC, Romu, T, Heymsfield, SB, et al. Advanced body composition assessment: from body mass index to body composition profiling. J Investig Med. (2018) 66:1–9. doi: 10.1136/jim-2018-000722
37. Ahima, RS, and Lazar, MA. Physiology. The health risk of obesity--better metrics imperative. Science. (2013) 341:856–8. doi: 10.1126/science.1241244
38. Lipina, C, and Hundal, HS. Lipid modulation of skeletal muscle mass and function. J Cachexia Sarcopenia Muscle. (2017) 8:190–201. doi: 10.1002/jcsm.12144
39. Giani, M, Rezoagli, E, Grassi, A, Porta, M, Riva, L, Famularo, S, et al. Low skeletal muscle index and myosteatosis as predictors of mortality in critically ill surgical patients. Nutrition. (2022) 101:111687. doi: 10.1016/j.nut.2022.111687
40. Nishikawa, H, Nakamura, S, Miyazaki, T, Kakimoto, K, Fukunishi, S, Asai, A, et al. Inflammatory bowel disease and sarcopenia: its mechanism and clinical importance. J Clin Med. (2021) 10:4214. doi: 10.3390/jcm10184214
41. Tweed, TT, van der Veen, A, Tummers, S, van Dijk, D, Luyer, MDP, Ruurda, JP, et al. Body composition is a predictor for postoperative complications after gastrectomy for gastric cancer: a prospective side study of the LOGICA trial. J Gastrointest Surg. (2022) 26:1373–87. doi: 10.1007/s11605-022-05321-0
42. Doyle, SL, Donohoe, CL, Lysaght, J, and Reynolds, JV. Visceral obesity, metabolic syndrome, insulin resistance and cancer. Proc Nutr Soc. (2012) 71:181–9. doi: 10.1017/S002966511100320X
43. Mueller, MH, Zhao, X, Macheroux, T, Kasparek, MS, Seeliger, H, and Kreis, ME. Differential activation of afferent neuronal and inflammatory pathways during small bowel obstruction (SBO). Neurogastroenterol Motil. (2016) 28:1599–608. doi: 10.1111/nmo.12861
44. Lowsby, R, Gomes, C, Jarman, I, Lisboa, P, Nee, PA, Vardhan, M, et al. Neutrophil to lymphocyte count ratio as an early indicator of blood stream infection in the emergency department. Emerg Med J. (2015) 32:531–4. doi: 10.1136/emermed-2014-204071
45. Mandaliya, H, Jones, M, Oldmeadow, C, and Nordman, IIC. Prognostic biomarkers in stage IV non-small cell lung cancer (NSCLC): neutrophil to lymphocyte ratio (NLR), lymphocyte to monocyte ratio (LMR), platelet to lymphocyte ratio (PLR) and advanced lung cancer inflammation index (ALI). Transl Lung Cancer Res. (2019) 8:886–94. doi: 10.21037/tlcr.2019.11.16
46. Liew, PX, and Kubes, P. The Neutrophil's role during health and disease. Physiol Rev. (2019) 99:1223–48. doi: 10.1152/physrev.00012.2018
47. Toptas, M, Akkoc, İ, Savas, Y, Uzman, S, Toptas, Y, and Can, MM. Novel hematologic inflammatory parameters to predict acute mesenteric ischemia. Blood Coagul Fibrinolysis. (2016) 27:127–30. doi: 10.1097/MBC.0000000000000372
48. Tsokos, GC . Lymphocytes, cytokines, inflammation, and immune trafficking. Curr Opin Rheumatol. (1995) 7:376–83. doi: 10.1097/00002281-199509000-00003
49. Alwarawrah, Y, Kiernan, K, and Mac Iver, NJ. Changes in nutritional status impact immune cell metabolism and function. Front Immunol. (2018) 9:1055. doi: 10.3389/fimmu.2018.01055
50. Zhou, X, Du, L, Shi, R, Chen, Z, Zhou, Y, Li, Z, et al. Early-life food nutrition, microbiota maturation and immune development shape life-long health. Crit Rev Food Sci Nutr. (2019) 59:S30–8. doi: 10.1080/10408398.2018.1485628
51. Hirani, V, Naganathan, V, Blyth, F, le Couteur, DG, Seibel, MJ, Waite, LM, et al. Low hemoglobin concentrations are associated with sarcopenia, physical performance, and disability in older Australian men in cross-sectional and longitudinal analysis: the Concord health and ageing in men project. J Gerontol A Biol Sci Med Sci. (2016) 71:1667–75. doi: 10.1093/gerona/glw055
52. Kang, Y, Zhu, X, Lin, Z, Zeng, M, Shi, P, Cao, Y, et al. Compare the diagnostic and prognostic value of MLR, NLR and PLR in CRC patients. Clin Lab. (2021) 67:2003–2009. doi: 10.7754/Clin.Lab.2021.201130
53. Wang, N, Xi, W, Lu, S, Jiang, J, Wang, C, Zhu, Z, et al. A novel inflammatory-nutritional prognostic scoring system for stage III gastric cancer patients with radical gastrectomy followed by adjuvant chemotherapy. Front Oncol. (2021) 11:650562. doi: 10.3389/fonc.2021.650562
54. Zhang, ZH, Jin, P, Feng, M, Yang, J, Huang, J, Chen, L, et al. Causal inference with marginal structural modeling for longitudinal data in laparoscopic surgery: a technical note. Laparoscopic, Endoscopic and Robotic Surgery. (2022) 5:146–52. doi: 10.1016/j.lers.2022.10.002
Glossary
Keywords: body composition, inflammatory-nutritional markers, adhesive small bowel obstruction, postoperative complications, prediction
Citation: Wang Z, Sun B, Yu Y, Liu J, Li D, Lu Y and Liu R (2024) A novel nomogram integrating body composition and inflammatory-nutritional markers for predicting postoperative complications in patients with adhesive small bowel obstruction. Front. Nutr. 11:1345570. doi: 10.3389/fnut.2024.1345570
Edited by:
Gabriela Villaça Chaves, National Cancer Institute (INCA), BrazilReviewed by:
Xiaobin Gu, First Affiliated Hospital of Zhengzhou University, ChinaMingkun Zhao, Fudan University, China
Zhongheng Zhang, Sir Run Run Shaw Hospital, China
Copyright © 2024 Wang, Sun, Yu, Liu, Li, Lu and Liu. This is an open-access article distributed under the terms of the Creative Commons Attribution License (CC BY). The use, distribution or reproduction in other forums is permitted, provided the original author(s) and the copyright owner(s) are credited and that the original publication in this journal is cited, in accordance with accepted academic practice. No use, distribution or reproduction is permitted which does not comply with these terms.
*Correspondence: Yun Lu, luyun@qdu.edu.cn; Ruiqing Liu, liuruiqing@qdu.edu.cn