- Department of Health and Sport Sciences, TUM School of Medicine and Health, Technical University of Munich, Munich, Germany
Background: Accurate dietary assessment remains a challenge, particularly in free-living settings. Continuous glucose monitoring (CGM) shows promise in optimizing the assessment and monitoring of ingestive activity (IA, i.e., consumption of calorie-containing foods/beverages), and it might enable administering dietary Just-In-Time Adaptive Interventions (JITAIs).
Objective: In a scoping review, we aimed to answer the following questions: (1) Which CGM approaches to automatically detect IA in (near-)real-time have been investigated? (2) How accurate are these approaches? (3) Can they be used in the context of JITAIs?
Methods: We systematically searched four databases until October 2023 and included publications in English or German that used CGM-based approaches for human (all ages) IA detection. Eligible publications included a ground-truth method as a comparator. We synthesized the evidence qualitatively and critically appraised publication quality.
Results: Of 1,561 potentially relevant publications identified, 19 publications (17 studies, total N = 311; for 2 studies, 2 publications each were relevant) were included. Most publications included individuals with diabetes, often using meal announcements and/or insulin boluses accompanying meals. Inpatient and free-living settings were used. CGM-only approaches and CGM combined with additional inputs were deployed. A broad range of algorithms was tested. Performance varied among the reviewed methods, ranging from unsatisfactory to excellent (e.g., 21% vs. 100% sensitivity). Detection times ranged from 9.0 to 45.0 min.
Conclusion: Several CGM-based approaches are promising for automatically detecting IA. However, response times need to be faster to enable JITAIs aimed at impacting acute IA. Methodological issues and overall heterogeneity among articles prevent recommending one single approach; specific cases will dictate the most suitable approach.
1 Introduction
Nutrition has a major impact on people’s health and well-being (1–8). However, accurately assessing nutrition and dietary intake remains challenging, with the most precise tools often involving high costs, participant and staff burden, or privacy issues (9–14). Yet, valid and reliable measurement of dietary behavior is essential to accurately detect changes in research settings and guide patient counseling in clinical practice (e.g., weight loss programs). Technological advances in recent years have led to new approaches for accurately assessing dietary intake that try to overcome some of the shortcomings of traditional dietary assessment methods (9, 15–18).
An attractive technology-based option for assessing the consumption of calorie-containing foods and beverages (ingestive activity, IA) is continuous glucose monitoring (CGM). CGM involves using a sensor that measures glucose concentrations in the interstitial fluid (19, 20) as a proxy for blood glucose levels (20, 21). CGM has become an important tool in diabetes care (19, 22–25). For instance, it is an integral component of artificial pancreas (AP) systems designed to automate and improve blood glucose regulation in individuals with type 1 diabetes mellitus (T1DM) via the utilization of CGM, an insulin infusion pump, and a control algorithm (26). Beyond diabetes management, CGM is gaining popularity for use in healthy individuals and athletes (20, 27). Several CGM devices show satisfactory accuracy data (20, 28, 29).
The automatic and (near-)real-time detection of IA via CGM could offer benefits in (clinical) practice, including a reduced participant and staff burden. In addition, interventionists could monitor meal plan adherence more closely and detect deviations from intervention goals as they occur. Consequently, targeted and personalized countermeasures could be deployed proactively. One particularly useful approach would be CGM-based detection of IA in the context of Just-In-Time Adaptive Interventions (JITAIs). JITAIs aim to exploit the full potential of remote monitoring combined with delivering intervention content in the moment/context when it is most needed and the patient is likely to be (most) receptive (30). Preliminary results show promising effects of JITAIs on predicting and preventing dietary lapses (31). If detection times of the CGM-based approaches in question were extremely short (e.g., less than a few minutes), JITAIs could aim at acutely impacting IA (e.g., sending a prompt asking a person to terminate a meal). If detection times were relatively short (e.g., less than an hour), JITAIs could aim at altering subsequent IA (e.g., a dinner meal). In both cases, information on IA would be much more readily available than with traditional dietary assessment methods (e.g., 24-h recalls).
However, there may also be challenges associated with the use of CGM for the automatic monitoring of IA. On the one hand, there are system-inherent challenges. For example, postprandial rises in blood glucose vary in timing and extent depending on meal composition, meal quantity, inter-individual variability, and many other factors (32–40). Further, there is a delay between interstitial fluid and blood glucose concentrations (20, 41, 42). On the other hand, blood glucose levels are not only influenced by IA but also by other factors such as physical activity, stress, and diurnal fluctuations (20, 41, 43–55). Thus, false positive detections (e.g., erroneously flagging a meal due to glucose increases caused by stress) and false negative detections (e.g., erroneously not flagging a meal because other factors render the glucose response too flat) might occur.
In the past years, research examining the use of CGM for the automatic detection of IA has accumulated. Recent publications reviewed options for the automatic detection of IA using wearable−/sensor-based methods (15–18), but they did not specifically address CGM. The present article aims to close this research gap and answer the following guiding questions:
1. Which approaches using CGM for the automatic detection of IA in (near-)real-time have been investigated, and have these approaches relied solely on CGM or also used other data (e.g., sensors/wearables)?
2. How accurate are these approaches in detecting IA?
3. Can these approaches be used in the context of JITAIs?
2 Methods
The reporting of this review is based on the updated Preferred Reporting Items for Systematic Reviews and Meta-Analyses Extension for Scoping Reviews (PRISMA-ScR) guideline (56).
2.1 Search strategy
The primary systematic literature search was conducted on 09 September 2022, using the IEEE Xplore, PubMed, Scopus, and Web of Science databases. An identical supplementary search was conducted on 02 October 2023. The search term was developed and refined by two authors (JB, CH) to capture all relevant publications, and the search term contained: (intake OR uptake OR eating OR ingest* OR meal OR drink* OR beverage OR consum* OR oral) AND (monitor* OR assess* OR detect* OR estimat* OR measur* OR sens*) AND (“continuous glucose monitoring” OR “real time continuous glucose monitoring” OR “real-time continuous glucose monitoring” OR “flash glucose monitoring” OR “intermittently scanned continuous glucose monitoring” OR CGM OR rtCGM OR isCGM OR “artificial pancreas” OR “artificial beta cell*” OR “artificial beta-cell*” OR “artificial β-cell*” OR “artificial β cell*”) AND (algorithm OR “deep learning” OR “machine learning” OR “neural network*” OR AI OR “artificial intelligence“). Because a fully-closed-loop AP system must first detect meals to adequately manage the following increases in glucose by delivering insulin to the patient (57), the search also included AP systems.
JB conducted the database searches and removed duplicates for the primary and supplementary searches. Two authors (JB, CH) screened the titles and abstracts against the predefined eligibility. In discrepancies, a consensus was reached via discussions and ineligible publications were discarded. For the primary search, JB screened the full texts of the remaining publications for eligibility and consulted with CH, who then independently screened these full texts in cases of uncertainty. In addition, one other author (SHF) conducted independent cross-checks for a randomly selected 20% of the full texts. For the supplementary search, two authors (JB, CH) independently screened the full texts of the remaining publications. Again, in case of discrepancies, a consensus was reached via discussions, and subsequently, ineligible publications were discarded. Another author (CG) was consulted for her technical/mathematical expertise during the screening process. JB hand-searched the reference lists of eligible publications for any additional relevant literature. In several cases, the corresponding authors of articles were contacted, e.g., to receive full texts or raw data or to clarify results.
2.2 Eligibility criteria
We included publications if the following inclusion criteria were met: (1) publications were written in English or German and published until 2 October 2023; (2) publications are original articles published in peer-reviewed scientific journals or conference papers; (3) at least one performance measure of the automatic detection of IA is reported explicitly. For example, the accuracy was calculated by comparing the CGM-based (did not have to exclusively rely on CGM as input) approach against a ground-truth method (e.g., self-reported or observed IA); (4) a CGM-based approach was used to detect IA in vivo in free-living, semi free-living, or laboratory settings. This also included trials of AP systems if criterion 3 was met; (5) only the most recent publication on a specific approach by a particular research group was included if it supersedes preceding publications.
We excluded publications for the following exclusion criteria: (1) the approach was not tested in human participants (e.g., in silico studies); (2) no outcome results were reported (e.g., study protocol publications); (3) outcomes did not include an explicit performance measure describing the results of the automatic detection of IA (e.g., only figures showcasing the CGM trends over time); (4) the methodology was described without sufficient detail. We did not apply restrictions regarding publication date or participant age.
2.3 Data extraction
The following information was extracted: (1) first author and publication year; (2) a summary of the study; (3) sample size and, if available, sex and age of participants; (4) participants’ diabetes status [no diabetes, prediabetes, T1DM or type 2 diabetes mellitus (T2DM)]; (5) scope of the study (duration/number of IA events) and, if available, information on the IA events (e.g., meal composition); (6) ground-truth/criterion method(s); (7) performance measure(s); (8) details on the CGM device and if applicable other relevant devices used in the study.
JB extracted relevant information from the original publications, and in cases of uncertainty, the respective publications were double-checked by CH. Two other authors (CG, SHF) also double-checked the extracted information. One other author (CG) further extracted technical details of the tested approaches.
2.4 Data synthesis
We synthesized the evidence qualitatively, focusing on answering the three research questions outlined above. Although using an explicit cutoff (e.g., ≥80% F1-score or accuracy) is desirable for performance evaluation and has been used in a related review (16), this approach was not feasible, as only a few publications reported accuracy and/or F1-score values.
Furthermore, we appraised the included publications critically. We considered the following aspects of being of concern: (1) error-prone methods for identifying the ground truth of IA [e.g., self-reported IA (58) or retrospective identification from CGM data, whereas inpatient settings with observed IA were generally assumed to be less error-prone]; (2) a sample consisting exclusively of individuals with diabetes as this might limit generalizability to non-diabetic populations; (3) meal announcement/meal-accompanying insulin boluses, as there might be an interference with the (early) postprandial blood glucose levels that are relevant for the automatic detection of IA; (4) algorithm inputs other than CGM since ultimately a CGM-only approach would be desirable to minimize costs and effort.
3 Results
The literature search identified a total of 1,561 potentially relevant publications. Nineteen publications reporting data from 17 studies (for 2 studies, 2 publications each were relevant, see Table 1), including 311 participants, met the inclusion criteria (59–66, 68, 70, 71, 73–75, 82–85, 87). Figure 1 shows the process of the literature search, screening, and selection in a PRISMA-style flow diagram (88).
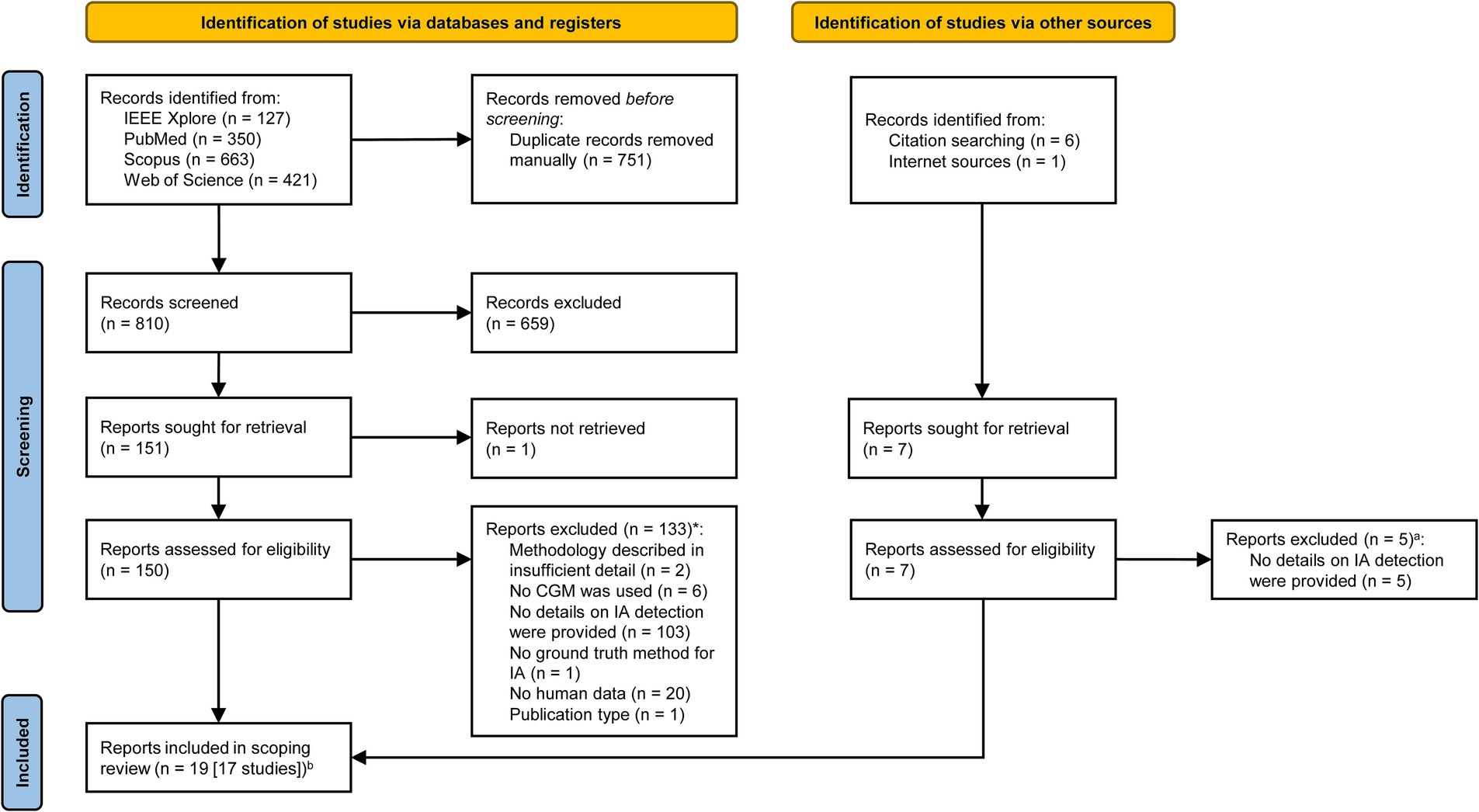
Figure 1. PRISMA Flow diagram (88). aIn some cases, more than one reason led to the exclusion of a publication; here, only the primary reason is listed for each publication. bIn two cases, two publications of the same study were included. CGM, continuous glucose monitoring; IA, ingestive activity.
Many of the screened publications were excluded from the present review because they did not include details on the detection of IA but instead focused on measures of glycemic control (e.g., time in specific glucose ranges). Further, some publications were excluded because they included graphic CGM data with IA marked as such but did not provide quantitative data on the detection of IA. Another common reason for exclusion was investigation in silico, often using virtual patients with T1DM.
We were unable to retrieve the full text of one publication despite several efforts to contact the authors directly. This publication was excluded; however, it was considered likely ineligible based on its abstract.
3.1 Study characteristics
The included publications were published between 2008 and 2023 and reported an average sample size of 18.3 (SD = 15.1) participants. Table 1 provides an overview of the included publications and the extracted information. The publications covered a wide age range, including pediatric (62, 73), adolescent (62, 64, 65), and adult (59–61, 63, 66, 68, 70, 71, 74, 75, 82–85, 87) populations. Fourteen publications included participants with T1DM (59, 62–66, 68, 70, 71, 73, 74, 84, 85, 87), one publication included a sample of participants with T1DM or T2DM (75), one publication included participants with prediabetes or moderately controlled T2DM (83) and three publications included participants without diabetes (60, 61, 82). Publications included both controlled/inpatient (59, 62–65, 68, 74, 82, 85) and free-living settings (60, 61, 66, 70, 71, 73, 75, 83, 87).
In several aspects, there was substantial heterogeneity among the included publications. First, the number and type of performance metrics reported for the tested approaches differed substantially. Commonly reported performance metrics included the number of true and/or false positives and/or negatives (including frequencies per day and rates) (64, 66, 68, 70, 73, 75, 84, 85, 87), sensitivity (60, 61, 70, 73–75, 84, 87), specificity (60, 61, 70), accuracy (61, 70), precision (60, 61, 66), F1-score (60, 61, 66), Matthew’s correlation coefficient (60, 61), Pearson’s correlations (83), detection time or time until an insulin bolus was delivered (59, 62–66, 70, 73–75, 84), change in glucose concentrations (62, 65, 84, 85), and area under the curve (82). However, even when the same metrics were reported, their definition was sometimes inconsistent across publications. For instance, the detection window for true positive detections ranged from 60 to 180 min, depending on the publication (66, 73, 75). In addition, the general study setup varied between publications, including differences in the sample composition, the use of meal announcement/meal-accompanying insulin boluses, the ground-truth method used for identifying IA, the devices used, and the scope of the data collection (Table 1).
Table 2 shows the result of the critical appraisal of all included publications. There were some concerns regarding the applied methodology for all publications; these concerns were substantial for most publications. In 16/19 (84.2%) publications, the sample consisted exclusively of individuals with (pre)diabetes (59, 62–66, 68, 70, 71, 73–75, 83–85, 87). Further, 9/19 (47.4%) publications used error-prone methods for measuring the ground truth of IA, mostly self-reported IA (60, 61, 66, 70, 71, 73, 75, 83, 87). Moreover, 7/19 (36.8%) publications used meal announcements and/or meal-accompanying insulin boluses (66, 68, 70, 71, 73, 75, 87). Finally, 8/19 (42.1%) publications utilized other inputs besides CGM, e.g., heart rate or the insulin sensitivity factor (59–61, 63, 64, 70, 74, 82). Overall, 15/19 (78.9%) publications elicited methodological concerns in two or more appraised domains (59–61, 63, 64, 66, 68, 70, 71, 73–75, 83, 84, 87), and all publications had methodological concerns in at least one of the appraised domains (59–66, 68, 70, 71, 73–75, 82–85, 87).
3.2 Overview of detection approaches
Our review identified a wide range of methods to automatically detect IA, including fuzzy logic (59, 63), model predictive control (74), support vector machine (60), random forest (60, 61, 82), (extreme) gradient boosting trees (60, 61, 82), backward difference (62), Kalman filter estimation (62), second derivative of glucose (62), Kalman filters (64, 68), switching signal generator (68), simulation-based explanation (83), classification of horizons (73), analysis of the glucose trajectory (84), pattern recognition using linear discriminant analysis (73), and threshold violation-based approaches (73). Further, adaptive model-based (64), super-twisting-based (66, 71), feedback scheme-based (70), and physiological parameter-invariant-based (87) meal detection approaches were applied.
The reviewed approaches used different inputs to automatically detect IA. As summarized in Table 2, some methods relied solely on CGM as an input (62, 65, 66, 68, 71, 73, 75, 83–85, 87). Others also included data from insulin treatment or other sensor systems (e.g., accelerometry, photoplethysmography, temperature sensors; see Table 1) (59–61, 63, 64, 70, 74, 82).
3.3 Performance of the approaches
We identified several CGM-based approaches for the automatic detection of IA that achieved high values in the respective performance metrics (Table 1). However, the substantial heterogeneity in the applied methodology and reporting of results needs to be considered.
For instance, Godoy and colleagues achieved 98.9% sensitivity, 96.7% specificity, and 97.6% accuracy with their feedback scheme-based algorithm (70). Notably, the algorithm uses certain patient-specific parameters, such as the insulin sensitivity factor derived from participants’ usual diabetes treatment (70). Similarly, the algorithm by El Fathi et al. successfully detected 12/12 meals without any false positives and a detection time of 35 min (64). In two publications using the same dataset, Bertrand et al. investigated several IA detection approaches in individuals without diabetes (60, 61). A range of performance metrics is reported in both publications. In the first publication, the highest achieved mean sensitivity was 66.8%, and the highest achieved mean specificity was 77.3%, for example (60). In the second publication, the highest achieved mean sensitivity was 66.8%, and the highest achieved mean specificity was 97.7% (61). Importantly, in both publications, the models did not exclusively rely on CGM as input (60, 61). Similarly, Palacios et al. had a sample of individuals without diabetes (82). However, their models, too, did not exclusively rely on CGM as input, but also utilized other physiological variables such as heart rate and skin temperature (82). Palacios et al. reported the area under the receiver operating characteristic curve (AUC-ROC) and the area under the precision-recall curve (AUC-PR) (82). For cold-start cases with a window size of k = 110 min, they reported an AUC-ROC of 89.1% and an AUC-PR value of 80.3% (82). For non-cold-start cases and k = 20 min, the AUC-ROC was 99.6%, and the AUC-PR was 96.4% (82).
The performance of CGM-only approaches, which hold particularly great value for practical applications, varied substantially. Sensitivities varied between 20.8% (73) and 96.8% (85). Where reported, average false positive detections ranged from 0.4 (75) to 2.8 (73) IA events per day. Selected publications further reported a false positive rate of 20.8% (84), a false discovery rate of 16.6% (74), and a median precision value of 73.0% (66). Moreover, publications reported detection times between 11.8 min (mean) (73) and 45.0 min (median) (66). Importantly, all CGM-only approaches were tested on samples consisting exclusively of individuals with (pre)diabetes, some of which also used meal announcements/insulin boluses. A detailed description of the performance metrics for each of the included publications is provided in Table 1.
3.4 Detection times
The detection time is the relevant metric to evaluate whether the identified CGM-based IA detection approaches could be used in the context of JITAIs.
A detection time measure was reported in 11/19 (57.9%) publications (59, 62–66, 70, 73–75, 84). The detection time was commonly defined as the time between the start of the IA (i.e., typically a meal) and its (automatic) detection by the CGM-based approach. Mean (59, 62, 64, 70, 73–75, 84) and median detection times (65, 66, 84) were reported, thus impeding direct comparisons. One publication reported the median time of the delivered prandial insulin boluses (63).
Overall, the reported detection times varied between 9.0 min (mean) (70) and 45.0 min (median) (66), with most values falling into the 20-to-40-min range (Table 1).
4 Discussion
The primary objective of this review was to examine whether CGM can be used to automatically detect IA in (near-)real time. In sum, there are various promising approaches that show satisfactory to excellent performance on measures such as sensitivity and specificity. However, the performance of CGM-based methods for automatically detecting IA varies. Similarly, detection times vary, but currently, they appear too long to administer JITAIs for acutely altering IA. Methodological issues and overall heterogeneity among publications make it difficult to recommend the best-performing approach.
4.1 Which approaches using CGM for the automatic detection of IA in (near-)real-time have been investigated, and have these approaches relied solely on CGM or also used other data (e.g., sensors/wearables)?
Our results indicate that both CGM-only approaches and those supplemented with other input data (e.g., accelerometry, photoplethysmography, temperature sensors) have been tested. Moreover, various algorithms have been used to detect IA. Since approaches using different sensor modalities and/or programming methods were successful at automatically detecting IA, it is evident that various solutions can be used for automated, CGM-based IA detection.
4.2 How accurate are these approaches in detecting IA?
Our review showed that the performance evaluation of any single approach depends on the respective case and priorities. For example, if the goal is to combine a CGM-based approach with smartphone prompts to enable comprehensive diet logs, the method should have high sensitivity to avoid missing a potential IA (false negative). In this case, specificity would only play a minor role as nothing is lost by sending a prompt in response to a false positive detection – the prompt can remain unanswered by the patient/participant. In contrast, when the goal is to use the CGM-based approach as a stand-alone IA assessment tool, high specificity would be critical to avoid artificial inflation of the number of daily meals, for example. Thus, a single best approach for all scenarios could not be identified. The substantial heterogeneity of the applied methods and reporting of results, including the broad range of the number and type of reported performance metrics and their varying definitions, made it difficult to compare the performance of the different approaches.
However, collectively, our results demonstrate that there are indeed several relatively well-performing CGM-based approaches for the automatic detection of IA. One example is the feedback scheme-based algorithm by Godoy et al., which achieved near-perfect sensitivity, specificity, and accuracy (70). However, this algorithm relies on several patient-specific parameters as input that are derived from participants’ usual diabetes treatment (70). Thus, it remains to be determined whether this approach could be adapted to work equally well in individuals without diabetes, for whom these data are not routinely assessed. Similarly, methodological issues further limiting studies’ internal and/or external validity pertain to using meal announcements or insulin boluses and focusing on samples with diabetes in the reviewed studies. All included publications suffered at least one such methodological limitation (Table 2).
Several reviewed articles reported solutions that relied solely on CGM as input for their IA detection algorithms. Performance among these approaches varied, but sensitivities ≥90% were achieved by several groups (73, 84, 85), and false positive occurrences < 1 per day were reported (75). This suggests that inputs other than CGM are not necessary to achieve excellent performance in automatically detecting IA.
Of note, some algorithms that incorporated inputs other than CGM might also work with CGM as their only input for the specific goal of IA detection. For example, in Bertrand et al.’s machine-learning algorithms, data from two wrist-worn activity trackers were incorporated in addition to the CGM data (61). However, the 20 most important features were derived from the CGM data (61). Hence, it is likely that an adaptation of their algorithm that relies exclusively on the CGM data as input might also achieve good – albeit likely somewhat worse – IA detection performance. Similar cases can be made for other publications in which insulin data were used as input in addition to the CGM data (59, 63, 64). These results suggest that it is possible to automatically detect IA using CGM-based and even CGM-only algorithms.
4.3 Can these approaches be used in the context of JITAIs?
Generally, to successfully administer a dietary JITAI, IA must be detected in (near-)real-time. However, precisely how short the detection would have to be depends on the specific goal, as outlined before. Detection times as fast as 9.0 min were reported (70), but most approaches needed 20 to 40 min to detect IA (Table 1). This can generate feedback on IA much faster than traditional dietary assessment methods, such as 24-h recalls, thus creating opportunities for earlier intervention. For instance, detecting deviations from a standardized study procedure (e.g., when IA is detected in a fasting window) is likely possible. Further, when deviations from a specific meal plan (e.g., low carbohydrate) are detected, the plan could be adjusted for the subsequent meals on the same day. Automated meal detection could further trigger behavioral intervention prompts regarding portion size and eating rate (i.e., reminders to eat more slowly) for future meals. However, in most cases, detection times are too long to modify/influence IA truly in the moment it occurs (e.g., a participant on a ketogenic diet has likely already finished a carbohydrate-rich meal by the time it is detected). Regardless, it is debatable if that is really the goal and what intervening during an eating event would look like.
4.4 Implications for clinical and research practice
Our review shows that several CGM-based options for the automatic detection of IA exist. Ultimately, the specific use case will dictate the most suitable approach. Different approaches might be appropriate depending on factors such as the budget, population, targeted level of wearing comfort, and goal of the automatic IA detection.
Notably, other innovative methods for the automatic detection of IA, such as those using wearable-, sensor-, and image-based methods (9, 15–18), are also promising. These methods may even be superior to CGM-based approaches regarding detection times. Wang and colleagues identified several devices that can quickly detect IA (16), such as a headband device that can detect eating events via chewing sounds within only 3 min (16, 90). Similarly, a pilot study by Kumar and colleagues investigating the use of abdominal sounds to detect IA found an average detection time of only 4.3 min (91). It has even been demonstrated that eating events can be predicted ahead of time (16, 92). Yang et al. used a camera, a GPS device, and an accelerometer to predict eating and food-purchasing events up to 4 min in advance (92). The authors found that a trained gradient-boosting model achieved a mean accuracy of 72.9% in predicting eating events 0–4 min in advance (92). This highlights that different methodologies might have inherent strengths and limitations. The suboptimal detection times might be considered an inherent limitation of CGM-based approaches. Recent advances have tried to solve the CGM-inherent lag time issue (93), but more research is needed. It remains to be seen whether these limitations inherent to using CGM for automatically detecting IA can be overcome. On the other hand, one key benefit of using CGM might be its unobtrusiveness, which could facilitate its acceptance in practice. This unobtrusiveness contrasts many other, more obtrusive approaches such as glasses and camera-based methods (9, 16, 18).
A promising prospect might be to use a sensitive CGM-based approach that sends a prompt to the patient/participant asking them to log IA in case of a true positive detection. Thus, the CGM-based approach would serve as an automated reminder. That way, a false positive detection does not automatically lead to erroneous IA information but needs to be verified by the person. In this context, the suboptimal detection times also likely would be acceptable.
4.5 Limitations and directions for future research
4.5.1 Sample characteristics
Unsurprisingly, most publications included samples with diabetes, as the primary use case for automated IA detection is AP systems. However, to examine the potential of CGM-based approaches for detecting IA in various populations, more research in more diverse populations, including healthy individuals, should be conducted. This is particularly important as the generalizability of previous findings to non-diabetic individuals is likely limited, for instance, due to the usually far lower variations in blood glucose levels in persons without diabetes (77) as compared to persons with diabetes (78). Thus, there may likely be systematic differences in the performance of such approaches in individuals with diabetes compared to those without diabetes. Moreover, in many studies, meals were announced to the system, and/or manual insulin boluses accompanied the registered meals. For example, Ornetzeder and colleagues evaluated the detection performance of three previously published algorithms (79–81) using meals accompanied by insulin boluses (75). While the resulting performance metrics of this publication and similar others are promising, they need to be interpreted considering the applied insulin boluses. Ornetzeder et al. argue that this potential distortion was deemed acceptable due to a lack of alternative, insulin bolus-free datasets and the time it takes for the administered insulin to achieve its peak action (75). However, it is still possible that the results of CGM-based IA detection approaches might differ in scenarios without exogenous insulin infusions. Specifically, the administered insulin might flatten the blood glucose excursions from the meal’s start, making its automatic detection less likely. In line with this, Faccioli et al. state that some of their false negatives might have been related to the attenuated postprandial CGM curves following the administration of meal-accompanying insulin therapy (66). At the same time, it should be considered that the postprandial glucose excursions of individuals with insulin-dependent diabetes would be much more pronounced without insulin treatment than in non-diabetic individuals (82). As such, it could be argued that by administering meal boluses, the postprandial glucose excursions of individuals with diabetes more closely approximate those of individuals without diabetes. Direct evidence is, of course, still necessary to increase confidence in any conclusions. Thus, future studies should ultimately enroll more individuals without diabetes.
4.5.2 Research focus
Moreover, it also needs to be considered that for the initialization of closed-loop systems, background information (e.g., treatment management, physical characteristics of the patient) is typically provided to the system (59). This information may only sometimes be readily available in other contexts. In addition, the goals of algorithms geared toward use in closed-loop/AP systems might differ from approaches aimed at the use for automatic detection of IA in general. For instance, in their AP-oriented work, Kölle et al. focused on glucose excursions caused by larger meals because smaller meals or snacks, which do not cause a substantial increase in blood glucose levels, do not necessarily need to be detected and trigger an insulin bolus to ensure adequate glucose control (73). Yet, in a scenario where the automatic detection of IA via CGM is meant to provide information on any IA – irrespective of its size – this argument does not hold up. This example highlights the potential differences in the setup of algorithms depending on the goal.
Taken together, fundamentally different circumstances and goals may be pursued, and thus, algorithms may be constructed differently, depending on the research question. Consequently, it might be possible to further optimize algorithms to automatically detect IA in research or clinical settings other than closed-loop/AP systems.
4.5.3 Comparability of approaches
There was substantial heterogeneity in how the performance of the investigated approaches was evaluated across the reviewed publications. Thus, as noted by others (66), a direct comparison between the approaches is difficult due to differences in the utilized datasets, preprocessing, and evaluation methods. Differences like these ultimately hamper the search for the best-performing approaches. Performance metrics reported in publications should include at least the following measures: the number of true positives, false positives, and false negatives, which can be used to calculate important metrics such as sensitivity and precision; the detection time, defined as the time from the start of the IA to the time the algorithm detects the IA, whose reporting allows researchers and practitioners to judge whether a specific approach could be used to administer JITAIs, for example. A short detection time followed by a prompt could also allow for more immediate self-reported IA. More accurate self-reports could be the consequence due to diminished recall bias.
4.5.4 Future avenues
In general, more research should be dedicated to using CGM for the specific goal of automatically detecting IA in a broad range of populations, particularly in individuals without diabetes. Such approaches have several potential benefits, but prior research has mainly focused on using CGM for diabetes care and AP systems. However, as explained, algorithms will likely be constructed differently for the specific goal of automatically detecting IA. Moreover, previous findings will have to be replicated and extended in non-diabetic samples to overcome the currently limited generalizability.
Depending on the use case, several advancements would be necessary to rely exclusively on a CGM-based/CGM-only approach for the remote monitoring of IA. To fully automate the logging of IA times in a reliable manner, most systems would have to be even more accurate than they currently are.
If the goal is to further automate IA timing and effectively log macronutrient intake, approaches would have to incorporate specific algorithms for this task. Several publications explored whether estimating macronutrients from CGM data is possible. For instance, Samadi et al. estimated the carbohydrate content of meals (84). Results were promising, with 64.1% of the detected IA events having an absolute carbohydrate estimation error of less than 25 g (84).
Similarly, if the goal is to administer JITAIs to impact acute IA, detection times would have to decrease further. However, as mentioned above, the lag time-caused suboptimal detection times might have to be considered an inherent limitation of CGM-based approaches. Only if future studies succeed at further reducing detection times will the application of CGM-based approaches for dietary JITAIs aiming to alter IA in the moment in the truest sense of the word become possible. This is especially true for cases in which meals are followed by only small and/or delayed postprandial glucose excursions (e.g., after high-fat meals) or when meals contain only a small amount of carbohydrates, as (timely) detection appears difficult here (66, 74). It would also be necessary to explicitly test the detection performance in cases of such challenging IA (e.g., ketogenic diets). Empirical data on such cases might enable the prediction of in which settings CGM-based approaches can be used for successfully detecting IA (e.g., only in contexts where at least moderate amounts of carbohydrates are consumed).
We advise that future studies use different approaches on the same dataset, providing comprehensive CGM and objective IA data, and then compare their performance using the abovementioned metrics. A starting point could be to compare the CGM-only approaches highlighted in Table 2. Such a fair and standardized comparison could further illuminate the currently most promising approach(es).
While CGM-only approaches are highly attractive because they only necessitate one single sensor (i.e., the CGM), multi-sensor solutions also hold great potential and should thus be further investigated. Specifically, combining the strengths of different sensors (e.g., CGM and wristbands) may yield superior results as compared to relying on only one sensor, although this remains to be determined empirically.
Lastly, similar to others (10), we strongly advise that researchers use interdisciplinary collaborations to develop new CGM-based dietary monitoring tools to combine technological and biological/nutritional expertise. Interdisciplinary collaborations should ensure that the resulting tools are useful and optimized from both perspectives.
4.6 Conclusion
Based on an exhaustive and systematic literature search, this scoping review shows that it is possible to automatically detect IA using CGM-based approaches. Despite methodological issues and substantial overall heterogeneity among publications, CGM-based dietary monitoring might complement clinical and research practice.
Data availability statement
The original contributions presented in the study are included in the article/supplementary material, further inquiries can be directed to the corresponding author.
Author contributions
JB: Conceptualization, Methodology, Writing – original draft, Writing – review & editing, Visualization. CG: Methodology, Writing – review & editing. SHF: Methodology, Writing – review & editing. KK: Conceptualization, Writing – review & editing. CH: Conceptualization, Methodology, Writing – original draft, Writing – review & editing.
Funding
The author(s) declare financial support was received for the research, authorship, and/or publication of this article. This work was funded internally by the Technical University of Munich. CH was supported by a Research Fellowship from the Alexander von Humboldt Foundation. These funding sources had no role in the design, execution, analyses, interpretation of the data, or decision to submit results. Where reported, most reviewed publications received funding primarily from national agencies. Some publications have also reported funding from non-profit organizations and industry partners.
Acknowledgments
The authors want to thank the authors of Bertrand et al. (60, 61), El Fathi et al. (64), and Palisaitis et al. (65) for kindly providing additional information that helped with the interpretation of their results.
Conflict of interest
The authors declare that the research was conducted without any commercial or financial relationships that could be construed as a potential conflict of interest.
CH and KK were an editorial board member of Frontiers, at the time of submission. This had no impact on the peer review process and the final decision.
Publisher’s note
All claims expressed in this article are solely those of the authors and do not necessarily represent those of their affiliated organizations, or those of the publisher, the editors and the reviewers. Any product that may be evaluated in this article, or claim that may be made by its manufacturer, is not guaranteed or endorsed by the publisher.
References
1. Afshin, A , Sur, PJ , Fay, KA , Cornaby, L , Ferrara, G , Salama, JS, et al. Health effects of dietary risks in 195 countries, 1990–2017: a systematic analysis for the global burden of disease study 2017. Lancet. (2019) 393:1958–72. doi: 10.1016/S0140-6736(19)30041-8
2. Cena, H , and Calder, PC . Defining a healthy diet: evidence for the role of contemporary dietary patterns in health and disease. Nutrients. (2020) 12:334. doi: 10.3390/nu12020334
3. Barbaresko, J , Lellmann, AW , Schmidt, A , Lehmann, A , Amini, AM , Egert, S, et al. Dietary factors and neurodegenerative disorders: an umbrella review of Meta-analyses of prospective studies. Adv Nutr. (2020) 11:1161–73. doi: 10.1093/advances/nmaa053
4. Neuenschwander, M , Ballon, A , Weber, KS , Norat, T , Aune, D , Schwingshackl, L, et al. Role of diet in type 2 diabetes incidence: umbrella review of meta-analyses of prospective observational studies. BMJ. (2019) 366:l2368. doi: 10.1136/bmj.l2368
5. Papadimitriou, N , Markozannes, G , Kanellopoulou, A , Critselis, E , Alhardan, S , Karafousia, V, et al. An umbrella review of the evidence associating diet and cancer risk at 11 anatomical sites. Nat Commun. (2021) 12:4579. doi: 10.1038/s41467-021-24861-8
6. Willett, WC , and Stampfer, MJ . Current evidence on healthy eating. Annu Rev Public Health. (2013) 34:77–95. doi: 10.1146/annurev-publhealth-031811-124646
7. English, LK , Ard, JD , Bailey, RL , Bates, M , Bazzano, LA , Boushey, CJ, et al. Evaluation of dietary patterns and all-cause mortality: a systematic review. JAMA Netw Open. (2021) 4:e2122277. doi: 10.1001/jamanetworkopen.2021.22277
8. Schwingshackl, L , Schwedhelm, C , Hoffmann, G , Lampousi, AM , Knüppel, S , Iqbal, K, et al. Food groups and risk of all-cause mortality: a systematic review and meta-analysis of prospective studies. Am J Clin Nutr. (2017) 105:1462–73. doi: 10.3945/ajcn.117.153148
9. Höchsmann, C , and Martin, CK . Review of the validity and feasibility of image-assisted methods for dietary assessment. Int J Obes. (2020) 44:2358–71. doi: 10.1038/s41366-020-00693-2
10. Klurfeld, DM , Hekler, EB , Nebeker, C , Patrick, K , and Khoo, CSH . Technology innovations in dietary intake and physical activity assessment: challenges and recommendations for future directions. Am J Prev Med. (2018) 55:e117–22. doi: 10.1016/j.amepre.2018.06.013
11. McClung, HL , Ptomey, LT , Shook, RP , Aggarwal, A , Gorczyca, AM , Sazonov, ES, et al. Dietary intake and physical activity assessment: current tools, techniques, and Technologies for use in adult populations. Am J Prev Med. (2018) 55:e93–e104. doi: 10.1016/j.amepre.2018.06.011
12. Ravelli, MN , and Schoeller, DA . An objective measure of energy intake using the principle of energy balance. Int J Obes. (2021) 45:725–32. doi: 10.1038/s41366-021-00738-0
13. Webb, GP . Methods of nutritional assessment and surveillance In: Nutrition: Maintaining and improving health. 5th. Boca Raton, FL: CRC Press (2019). 47–87.
14. Wong, WW , Roberts, SB , Racette, SB , das, SK , Redman, LM , Rochon, J, et al. The doubly labeled water method produces highly reproducible longitudinal results in nutrition studies. J Nutr. (2014) 144:777–83. doi: 10.3945/jn.113.187823
15. Bell, BM , Alam, R , Alshurafa, N , Thomaz, E , Mondol, AS , de la Haye, K, et al. Automatic, wearable-based, in-field eating detection approaches for public health research: a scoping review. NPJ Digit Med. (2020) 3:38. doi: 10.1038/s41746-020-0246-2
16. Wang, L , Allman-Farinelli, M , Yang, JA , Taylor, JC , Gemming, L , Hekler, E, et al. Enhancing nutrition care through real-time, sensor-based capture of eating occasions: a scoping review. Front Nutr. (2022) 9:852984. doi: 10.3389/fnut.2022.852984
17. Hassannejad, H , Matrella, G , Ciampolini, P , De Munari, I , Mordonini, M , and Cagnoni, S . Automatic diet monitoring: a review of computer vision and wearable sensor-based methods. Int J Food Sci Nutr. (2017) 68:656–70. doi: 10.1080/09637486.2017.1283683
18. Vu, T , Lin, F , Alshurafa, N , and Xu, W . Wearable food intake monitoring technologies: a comprehensive review. Computers. (2017) 6:4. doi: 10.3390/computers6010004
19. Bellido, V , Aguilera, E , Cardona-Hernandez, R , Diaz-Soto, G , González Pérez de Villar, N , Picón-César, MJ, et al. Expert recommendations for using time-in-range and other continuous glucose monitoring metrics to achieve patient-centered glycemic control in people with diabetes. J Diabetes Sci Technol. (2023) 17:1326–36. doi: 10.1177/19322968221088601
20. Holzer, R , Bloch, W , and Brinkmann, C . Continuous glucose monitoring in healthy adults—possible applications in health care, wellness, and sports. Sensors. (2022) 22:2030. doi: 10.3390/s22052030
21. Zheng, M , Ni, B , and Kleinberg, S . Automated meal detection from continuous glucose monitor data through simulation and explanation. J Am Med Inform Assoc. (2019) 26:1592–9. doi: 10.1093/jamia/ocz159
22. Lal, RA , Ekhlaspour, L , Hood, K , and Buckingham, B . Realizing a closed-loop (artificial pancreas) system for the treatment of type 1 diabetes. Endocr Rev. (2019) 40:1521–46. doi: 10.1210/er.2018-00174
23. American Diabetes Association Professional Practice Committee . 6. Glycemic targets: standards of medical Care in Diabetes—2022. Diabetes Care. (2022) 45:S83–96. doi: 10.2337/dc22-S006
24. Aleppo, G , and Webb, K . Continuous glucose monitoring integration in clinical practice: a stepped guide to data review and interpretation. J Diabetes Sci Technol. (2019) 13:664–73. doi: 10.1177/1932296818813581
25. Pease, A , Lo, C , Earnest, A , Kiriakova, V , Liew, D , and Zoungas, S . The efficacy of Technology in Type 1 diabetes: a systematic review, network Meta-analysis, and narrative synthesis. Diabetes Technol Ther. (2020) 22:411–21. doi: 10.1089/dia.2019.0417
26. Renard, E . Automated insulin delivery systems: from early research to routine care of type 1 diabetes. Acta Diabetol. (2022) 60:151–61. doi: 10.1007/s00592-022-01929-5
27. Ishihara, K , Uchiyama, N , Kizaki, S , Mori, E , Nonaka, T , and Oneda, H . Application of continuous glucose monitoring for assessment of individual carbohydrate requirement during ultramarathon race. Nutrients. (2020) 12:1121. doi: 10.3390/nu12041121
28. Alva, S , Bailey, T , Brazg, R , Budiman, ES , Castorino, K , Christiansen, MP, et al. Accuracy of a 14-day factory-calibrated continuous glucose monitoring system with advanced algorithm in pediatric and adult population with diabetes. J Diabetes Sci Technol. (2022) 16:70–7. doi: 10.1177/1932296820958754
29. Freckmann, G , Link, M , Kamecke, U , Haug, C , Baumgartner, B , and Weitgasser, R . Performance and usability of three Systems for Continuous Glucose Monitoring in direct comparison. J Diabetes Sci Technol. (2019) 13:890–8. doi: 10.1177/1932296819826965
30. Schembre, SM , Liao, Y , Robertson, MC , Dunton, GF , Kerr, J , Haffey, ME, et al. Just-in-time feedback in diet and physical activity interventions: systematic review and practical design framework. J Med Internet Res. (2018) 20:e106. doi: 10.2196/jmir.8701
31. Forman, EM , Goldstein, SP , Zhang, F , Evans, BC , Manasse, SM , Butryn, ML, et al. OnTrack: development and feasibility of a smartphone app designed to predict and prevent dietary lapses. Transl Behav Med. (2019) 9:236–45. doi: 10.1093/tbm/iby016
32. American Diabetes Association . Postprandial Blood Glucose. Diabetes Care. (2001) 24:775–8. doi: 10.2337/diacare.24.4.775
33. Bell, KJ , Smart, CE , Steil, GM , Brand-Miller, JC , King, B , and Wolpert, HA . Impact of fat, protein, and glycemic index on postprandial glucose control in type 1 diabetes: implications for intensive diabetes Management in the Continuous Glucose Monitoring era. Diabetes Care. (2015) 38:1008–15. doi: 10.2337/dc15-0100
34. Gingras, V , Bonato, L , Messier, V , Roy-Fleming, A , Smaoui, MR , Ladouceur, M, et al. Impact of macronutrient content of meals on postprandial glucose control in the context of closed-loop insulin delivery: A randomized cross-over study. Diabetes Obes Metab. (2018) 20:2695–9. doi: 10.1111/dom.13445
35. Furthner, D , Lukas, A , Schneider, AM , Mörwald, K , Maruszczak, K , Gombos, P, et al. The role of protein and fat intake on insulin therapy in Glycaemic control of Paediatric type 1 diabetes: a systematic review and research gaps. Nutrients. (2021) 13:3558. doi: 10.3390/nu13103558
36. Pinsker, JE , Lee, JB , Dassau, E , Seborg, DE , Bradley, PK , Gondhalekar, R, et al. Randomized crossover comparison of personalized MPC and PID control algorithms for the artificial pancreas. Diabetes Care. (2016) 39:1135–42. doi: 10.2337/dc15-2344
37. Shilo, S , Godneva, A , Rachmiel, M , Korem, T , Kolobkov, D , Karady, T, et al. Prediction of personal glycemic responses to food for individuals with type 1 diabetes through integration of clinical and microbial data. Diabetes Care. (2022) 45:502–11. doi: 10.2337/dc21-1048
38. Rein, M , Ben-Yacov, O , Godneva, A , Shilo, S , Zmora, N , Kolobkov, D, et al. Effects of personalized diets by prediction of glycemic responses on glycemic control and metabolic health in newly diagnosed T2DM: a randomized dietary intervention pilot trial. BMC Med. (2022) 20:56. doi: 10.1186/s12916-022-02254-y
39. Rossetti, P , Ampudia-Blasco, FJ , Laguna, A , Revert, A , Vehì, J , Ascaso, JF, et al. Evaluation of a novel continuous glucose monitoring-based method for mealtime insulin dosing--the iBolus--in subjects with type 1 diabetes using continuous subcutaneous insulin infusion therapy: a randomized controlled trial. Diabetes Technol Ther. (2012) 14:1043–52. doi: 10.1089/dia.2012.0145
40. Hall, KD , Guo, J , Courville, AB , Boring, J , Brychta, R , Chen, KY, et al. Effect of a plant-based, low-fat diet versus an animal-based, ketogenic diet on ad libitum energy intake. Nat Med. (2021) 27:344–53. doi: 10.1038/s41591-020-01209-1
41. Zaharieva, DP , Turksoy, K , McGaugh, SM , Pooni, R , Vienneau, T , Ly, T, et al. Lag time remains with newer real-time continuous glucose monitoring technology during aerobic exercise in adults living with type 1 diabetes. Diabetes Technol Ther. (2019) 21:313–21. doi: 10.1089/dia.2018.0364
42. Cobelli, C , Renard, E , and Kovatchev, B . Artificial pancreas: past, present, future. Diabetes. (2011) 60:2672–82. doi: 10.2337/db11-0654
43. Armario, A , Marti, O , Molina, T , de Pablo, J , and Valdes, M . Acute stress markers in humans: response of plasma glucose, cortisol and prolactin to two examinations differing in the anxiety they provoke. Psychoneuroendocrinology. (1996) 21:17–24. doi: 10.1016/0306-4530(95)00048-8
44. García-García, F , Kumareswaran, K , Hovorka, R , and Hernando, ME . Quantifying the acute changes in glucose with exercise in type 1 diabetes: a systematic review and Meta-analysis. Sports Med. (2015) 45:587–99. doi: 10.1007/s40279-015-0302-2
45. Park, SH , Yao, J , Chua, XH , Chandran, SR , Gardner, DSL , Khoo, CM, et al. Diet and physical activity as determinants of continuously measured glucose levels in persons at high risk of type 2 diabetes. Nutrients. (2022) 14:366. doi: 10.3390/nu14020366
46. Bennetsen, SL , Feineis, CS , Legaard, GE , Lyngbæk, MPP , Karstoft, K , and Ried-Larsen, M . The impact of physical activity on glycemic variability assessed by continuous glucose monitoring in patients with type 2 diabetes mellitus: a systematic review. Front Endocrinol. (2020) 11:486. doi: 10.3389/fendo.2020.00486
47. Colberg, SR , Sigal, RJ , Yardley, JE , Riddell, MC , Dunstan, DW , Dempsey, PC, et al. Physical activity/exercise and diabetes: a position statement of the American Diabetes Association. Diabetes Care. (2016) 39:2065–79. doi: 10.2337/dc16-1728
48. Wiesli, P , Schmid, C , Kerwer, O , Nigg-Koch, C , Klaghofer, R , Seifert, B, et al. Acute psychological stress affects glucose concentrations in patients with type 1 diabetes following food intake but not in the fasting state. Diabetes Care. (2005) 28:1910–5. doi: 10.2337/diacare.28.8.1910
49. Gonder-Frederick, LA , Grabman, JH , Kovatchev, B , Brown, SA , Patek, S , Basu, A, et al. Is psychological stress a factor for incorporation into future closed-loop systems? J Diabetes Sci Technol. (2016) 10:640–6. doi: 10.1177/1932296816635199
50. Sapolsky, RM , Romero, LM , and Munck, AU . How do glucocorticoids influence stress responses? Integrating permissive, suppressive, stimulatory, and preparative actions. Endocr Rev. (2000) 21:55–89. doi: 10.1210/edrv.21.1.0389
51. Bequette, BW . Challenges and recent Progress in the development of a closed-loop artificial pancreas. Annu Rev Control. (2012) 36:255–66. doi: 10.1016/j.arcontrol.2012.09.007
52. Colmegna, P , Garelli, F , De Battista, H , and Sánchez-Peña, R . Automatic regulatory control in type 1 diabetes without carbohydrate counting. Control Eng Pract. (2018) 74:22–32. doi: 10.1016/j.conengprac.2018.02.003
53. Reddy, R , Wittenberg, A , Castle, JR , el Youssef, J , Winters-Stone, K , Gillingham, M, et al. Effect of aerobic and resistance exercise on glycemic control in adults with type 1 diabetes. Can J Diabetes. (2019) 43:406–414.e1. doi: 10.1016/j.jcjd.2018.08.193
54. Cinar, A , and Turksoy, K . Multivariable control of glucose concentration In: Advances in artificial pancreas systems. Cham: Springer International Publishing (2018). 65–82.
55. Maahs, DM , DeSalvo, D , Pyle, L , Ly, T , Messer, L , Clinton, P, et al. Effect of acetaminophen on CGM glucose in an outpatient setting. Diabetes Care. (2015) 38:e158–9. doi: 10.2337/dc15-1096
56. Tricco, AC , Lillie, E , Zarin, W , O'Brien, KK , Colquhoun, H , Levac, D, et al. PRISMA extension for scoping reviews (PRISMA-ScR): checklist and explanation. Ann Intern Med. (2018) 169:467–73. doi: 10.7326/M18-0850
57. Diamond, T , Cameron, F , and Bequette, BW . A new meal absorption model for artificial pancreas systems. J Diabetes Sci Technol. (2022) 16:40–51. doi: 10.1177/1932296821990111
58. Ravelli, MN , and Schoeller, DA . Traditional self-reported dietary instruments are prone to inaccuracies and new approaches are needed. Front Nutr. (2020) 7:90. doi: 10.3389/fnut.2020.00090
59. Atlas, E , Nimri, R , Miller, S , Grunberg, EA , and Phillip, M . MD-logic artificial pancreas system. Diabetes Care. (2010) 33:1072–6. doi: 10.2337/dc09-1830
60. Bertrand, L. , Cleyet-Marrel, N. , and Liang, Z. The role of continuous glucose monitoring in automatic detection of eating activities. In 2021 IEEE 3rd global conference on life sciences and technologies (LifeTech). Nara, Japan: IEEE; (2021). p. 313–314.
61. Bertrand, L , Cleyet-Marrel, N , and Liang, Z . Recognizing eating activities in free-living environment using consumer wearable devices. In: Engineering Proceedings MDPI; (2021). p. 58.
62. Dassau, E , Bequette, BW , Buckingham, BA , and Doyle, FJ . Detection of a meal using continuous glucose monitoring. Diabetes Care. (2008) 31:295–300. doi: 10.2337/dc07-1293
63. Dovc, K , Piona, C , Yeşiltepe Mutlu, G , Bratina, N , Jenko Bizjan, B , Lepej, D, et al. Faster compared with standard insulin Aspart during day-and-night fully closed-loop insulin therapy in type 1 diabetes: a double-blind randomized crossover trial. Diabetes Care. (2020) 43:29–36. doi: 10.2337/dc19-0895
64. El Fathi, A , Palisaitis, E , Boulet, B , Legault, L , and Haidar, A . An Unannounced Meal Detection Module for artificial pancreas control systems. In: 2019 American control conference (ACC). Philadelphia, PA, USA: IEEE; (2019). p. 4130–4135.
65. Palisaitis, E , El Fathi, A , von Oettingen, JE , Haidar, A , and Legault, L . A meal detection algorithm for the artificial pancreas: a randomized controlled clinical trial in adolescents with type 1 diabetes. Diabetes Care. (2021) 44:604–6. doi: 10.2337/dc20-1232
66. Faccioli, S , Sala-Mira, I , Díez, JL , Facchinetti, A , Sparacino, G , del Favero, S, et al. Super–twisting-based meal detector for type 1 diabetes management: improvement and assessment in a real-life scenario. Comput Methods Prog Biomed. (2022) 219:106736. doi: 10.1016/j.cmpb.2022.106736
67. Anderson, SM , Raghinaru, D , Pinsker, JE , Boscari, F , Renard, E , Buckingham, BA, et al. Multinational home use of closed-loop control is safe and effective. Diabetes Care. (2016) 39:1143–50. doi: 10.2337/dc15-2468
68. Fushimi, E , Colmegna, P , De Battista, H , Garelli, F , and Sanchez-Pena, R . Unannounced meal analysis of the ARG algorithm. In: 2019 American control conference (ACC). Philadelphia, PA, USA: IEEE; (2019) p. 4740–4745.
69. Sánchez-Peña, R , Colmegna, P , Garelli, F , De Battista, H , García-Violini, D , Moscoso-Vásquez, M, et al. Artificial pancreas: clinical study in Latin America without Premeal insulin boluses. J Diabetes Sci Technol. (2018) 12:914–25. doi: 10.1177/1932296818786488
70. Godoy, JL , Sereno, JE , and Rivadeneira, PS . Meal detection and carbohydrate estimation based on a feedback scheme with application to the artificial pancreas. Biomed Signal Process Control. (2021) 68:102715. doi: 10.1016/j.bspc.2021.102715
71. Hoyos, J. D. , Laguna, A. J. , Carpintero, A. R. , Sala-Mira, I. , Diez, J. L. , and Bondia, J. Characterization of glycemic patterns in type 1 diabetes without insulin or meal input data. In: 2022 10th international conference on systems and control (ICSC). Marseille, France: IEEE; (2022). p. 576–581.
72. Aleppo, G , Ruedy, KJ , Riddlesworth, TD , Kruger, DF , Peters, AL , Hirsch, I, et al. REPLACE-BG: a randomized trial comparing continuous glucose monitoring with and without routine blood glucose monitoring in adults with well-controlled type 1 diabetes. Diabetes Care. (2017) 40:538–45. doi: 10.2337/dc16-2482
73. Kölle, K , Biester, T , Christiansen, S , Fougner, AL , and Stavdahl, O . Pattern recognition reveals characteristic postprandial glucose changes: non-individualized meal detection in diabetes mellitus type 1. IEEE J Biomed Health Inform. (2020) 24:594–602. doi: 10.1109/JBHI.2019.2908897
74. Mosquera-Lopez, C , Wilson, LM , El Youssef, J , Hilts, W , Leitschuh, J , Branigan, D, et al. Enabling fully automated insulin delivery through meal detection and size estimation using artificial intelligence. NPJ Digit Med. (2023) 6:39. doi: 10.1038/s41746-023-00783-1
75. Ornetzeder, C , Reiterer, F , Christensen, MB , Norgaard, K , Freckmann, G , and del Re, L . Feasibility of fully closed loop insulin delivery in type 2 diabetes. In: 2019 IEEE conference on control technology and applications (CCTA). Hong Kong, China: IEEE; (2019). p. 906–913.
76. Zschornack, E , Schmid, C , Pleus, S , Link, M , Klötzer, HM , Obermaier, K, et al. Evaluation of the performance of a novel system for continuous glucose monitoring. J Diabetes Sci Technol. (2013) 7:815–23. doi: 10.1177/193229681300700403
77. Shah, VN , DuBose, SN , Li, Z , Beck, RW , Peters, AL , Weinstock, RS, et al. Continuous glucose monitoring profiles in healthy nondiabetic participants: a multicenter prospective study. J Clin Endocrinol Metab. (2019) 104:4356–64. doi: 10.1210/jc.2018-02763
78. Gubitosi-Klug, RA , Braffett, BH , Bebu, I , Johnson, ML , Farrell, K , Kenny, D, et al. Continuous glucose monitoring in adults with type 1 diabetes with 35 years duration from the DCCT/EDIC study. Diabetes Care. (2022) 45:659–65. doi: 10.2337/dc21-0629
79. Harvey, RA , Dassau, E , Zisser, H , Seborg, DE , and Doyle, FJ . Design of the Glucose Rate Increase Detector: a meal detection module for the health monitoring system. J Diabetes Sci Technol. (2014) 8:307–20. doi: 10.1177/1932296814523881
80. Samadi, S , Turksoy, K , Hajizadeh, I , Feng, J , Sevil, M , and Cinar, A . Meal detection and carbohydrate estimation using continuous glucose sensor data. IEEE J Biomed Health Inform. (2017) 21:619–27. doi: 10.1109/JBHI.2017.2677953
81. Ramkissoon, C , Herrero, P , Bondia, J , and Vehi, J . Unannounced meals in the artificial pancreas: detection using continuous glucose monitoring. Sensors. (2018) 18:884. doi: 10.3390/s18030884
82. Palacios, V , Woodbridge, DMK , and Fry, JL . Machine learning-based meal detection using continuous glucose monitoring on healthy participants: an objective measure of participant compliance to protocol. In: 2021 43rd annual international conference of the IEEE engineering in Medicine & Biology Society (EMBC). Mexico: IEEE; (2021). p. 7032–7035.
83. Popp, CJ , Wang, C , Hoover, A , Gomez, LA , Curran, M , St-Jules, DE, et al. Objective determination of eating occasion timing (OREO): combining self-report, wrist motion, and continuous glucose monitoring to detect eating occasions in adults with pre-diabetes and obesity. J Diabetes Sci Technol. (2023):19322968231197205. doi: 10.1177/19322968231197205
84. Samadi, S , Rashid, M , Turksoy, K , Feng, J , Hajizadeh, I , Hobbs, N, et al. Automatic detection and estimation of unannounced meals for multivariable artificial pancreas system. Diabetes Technol Ther. (2018) 20:235–46. doi: 10.1089/dia.2017.0364
85. Turksoy, K , Samadi, S , Feng, J , Littlejohn, E , Quinn, L , and Cinar, A . Meal detection in patients with type 1 diabetes: a new module for the multivariable adaptive artificial pancreas control system. IEEE J Biomed Health Inform. (2016) 20:47–54. doi: 10.1109/JBHI.2015.2446413
86. Turksoy, K , Quinn, LT , Littlejohn, E , and Cinar, A . An integrated multivariable artificial pancreas control system. J Diabetes Sci Technol. (2014) 8:498–507. doi: 10.1177/1932296814524862
87. Weimer, J , Chen, S , Peleckis, A , Rickels, MR , and Lee, I . Physiology-invariant meal detection for type 1 diabetes. Diabetes Technol Ther. (2016) 18:616–24. doi: 10.1089/dia.2015.0266
88. Page, MJ , McKenzie, JE , Bossuyt, PM , Boutron, I , Hoffmann, TC , Mulrow, CD, et al. Statement: an updated guideline for reporting systematic reviews. BMJ. (2020) 2021:n71. doi: 10.1136/bmj.n71
89. Lee, H , and Wayne, Bequette , A closed-loop artificial pancreas based on model predictive control: Human-friendly identification and automatic meal disturbance rejection. Biomed. Signal Process. Control. (2009) 4:347–54. doi: 10.1016/j.bspc.2009.03.002
90. Bi, S , Wang, T , Tobias, N , Nordrum, J , Wang, S , Halvorsen, G, et al. Auracle: detecting eating episodes with an ear-mounted sensor. Proc ACM Interact Mob Wearable Ubiquitous Technol. (2018) 2:1–27. doi: 10.1145/3264902
91. Kumar, TS , Soiland, E , Stavdahl, O , and Fougner, AL . Pilot study of early meal onset detection from abdominal sounds. In: 2019 E-health and bioengineering conference (EHB). Iasi, Romania: IEEE; (2019). p. 1–4.
92. Yang, JA , Wang, J , Nakandala, S , Kumar, A , and Jankowska, MM . Predicting eating events in free living individuals. In 2019 15th international conference on eScience (eScience). San Diego, CA, USA: IEEE; (2019). p. 627–629.
93. Halvorsen, M , Benam, KD , Khoshamadi, H , and Fougner, AL . Blood glucose level prediction using subcutaneous sensors for in vivo study: compensation for measurement method slow dynamics using Kalman filter approach. In 2022 IEEE 61st conference on decision and control (CDC). Cancun, Mexico: IEEE; (2022). p. 6034–6039.
Keywords: meal detection, continuous glucose monitoring, dietary assessment, healthcare technology, closed loop, sensors, meal timing
Citation: Brummer J, Glasbrenner C, Hechenbichler Figueroa S, Koehler K and Höchsmann C (2024) Continuous glucose monitoring for automatic real-time assessment of eating events and nutrition: a scoping review. Front. Nutr. 10:1308348. doi: 10.3389/fnut.2023.1308348
Edited by:
Arpita Mukhopadhyay, St. John's Research Institute, IndiaReviewed by:
Andrea Tumminia, Università di Catania, ItalyAli Cinar, Illinois Institute of Technology, United States
Copyright © 2024 Brummer, Glasbrenner, Hechenbichler Figueroa, Koehler and Höchsmann. This is an open-access article distributed under the terms of the Creative Commons Attribution License (CC BY). The use, distribution or reproduction in other forums is permitted, provided the original author(s) and the copyright owner(s) are credited and that the original publication in this journal is cited, in accordance with accepted academic practice. No use, distribution or reproduction is permitted which does not comply with these terms.
*Correspondence: Christoph Höchsmann, christoph.hoechsmann@tum.de
†ORCID: Julian Brummer https://orcid.org/0000-0001-9022-5497
Christina Glasbrenner https://orcid.org/0009-0008-5420-5219
Sieglinde Hechenbichler Figueroa https://orcid.org/0000-0001-9147-8824
Karsten Koehler https://orcid.org/0000-0002-9618-2069
Christoph Höchsmann https://orcid.org/0000-0003-2007-3007