- 1Department of Public Health, Wuhan Fourth Hospital, Wuhan, China
- 2Department of Psychiatry, Wuhan Mental Health Center, Wuhan, China
- 3Department of Psychiatry, Wuhan Hospital for Psychotherapy, Wuhan, China
- 4Department of Pathophysiology, School of Basic Medicine and Tongji Medical College, Huazhong University of Science and Technology, Wuhan, China
- 5School of Public Health, Wuhan University, Wuhan, China
- 6Department of Health Promotion, XiaoGan Center for Disease Control and Pervention, Xiaogan, China
Objective: This study aimed to explore the association of the oxidative balance score (OBS) with total abdominal fat mass (TAFM) and visceral adipose tissue mass (VATM) percentages among young and middle-aged U.S. adults.
Methods: Young and middle-aged adults in the National Health and Nutrition Examination Survey (NHANES) from 2011 to 2018 were included. Analysis of variance and Rao-Scott adjusted chi-square tests were used to compare the characteristics across quartiles of OBS. Univariate and multivariate weighted logistic regression models were employed to explore the relationship between OBS and the risks of high TAFM or high VATM percentage in the general population and subgroups, while the interaction effects were tested with a likelihood test. Weighted restricted cubic spline analyses were utilized to assess the non-linear association of OBS with TAFM and VATM percentages.
Results: The final sample included 8,734 young and middle-aged non-institutionalized U.S. adults representing 134.7 million adults. Compared with adults in the first quartile of OBS, those with higher OBS were less likely to have a high TAFM percentage; the ORs and 95% CI for adults in the second, third, and highest quartiles of OBS were 0.70 (0.53–0.94), 0.49 (0.36–0.60), and 0.25 (0.18–0.36), respectively. Similar trends were observed in the association between OBS and VATM percentages. Moreover, similar effects were confirmed in the sensitivity analyses and subgroup analyses according to demographic characteristics. Regarding the OBS subclass, higher dietary OBS and lifestyle OBS were also correlated with decreased ORs of high TAFM and VATM percentages.
Conclusion: This study strongly suggests that higher OBS, as well as higher dietary OBS and lifestyle OBS, are significantly correlated with lower risks of abdominal obesity and visceral fat accumulation. The findings highlight the importance of an antioxidant-rich diet and maintaining a healthy lifestyle in reducing the risks.
1 Introduction
The obesity health issue, usually assessed by body mass index (BMI), continues its relentless advance globally, the prevalence of which has climbed from 3 to 11% among men and from 6 to 15% among women over the past 40 years (1). BMI values continued to rise until they crossed the threshold of normality and reached an average BMI of 27.8 in 2014 in the United States (2). Age-standardized prevalence of obesity and severe obesity in U.S. adults increased from 33.7 and 5.7% in 2007–2008 to 39.6 and 7.7% in 2015–2016, which has seen a significant increase over the years (3).
Obesity, gradually regarded as a chronic relapsing disease process, enlarges fat cells, and ectopic fat produces and secretes a variety of metabolic, hormonal, and inflammatory substances that are pernicious to organs, especially the liver and pancreas (4). Epidemiological studies have illustrated obesity as a significant risk factor for a variety of non-communicable chronic diseases, including diabetes, cardiovascular disease, gout, non-alcoholic fatty liver disease, and a set of cancers (5–10). Moreover, obesity could also result in a series of skeletal muscle disorders, probably on account of adipose tissue inflammation dominating skeletal muscle inflammation (11, 12). In addition to its impact on individual health, obesity also has significant economic consequences, particularly in terms of healthcare costs. The Global Burden of Disease group has estimated that elevated BMI values contributed to 4 million deaths in 2015, two-thirds of which were responsible for cardiovascular disease (13). In addition, Haijiang Dai et al. have estimated that the global number of disability-adjusted life years related to high BMI has more than doubled for both sexes between 1990 and 2017 (14).
Oxidative stress is defined as an imbalance between oxidants and antioxidants in favor of the oxidants, leading to a disruption of redox signaling and control and/or molecular damage (15). Nevertheless, it is impossible to reflect oxidative stress level by a single factor, and oxidative balance score (OBS) emerged at the time required. In addition, the exact index, with its higher simplicity and understandability, greatly improves the ability to identify individual oxidative stress levels. OBS, combining various dietary and lifestyle pro-oxidants and antioxidants, is provided to measure individual exposures to antioxidants and pro-oxidants as well as the balance, in which higher OBS indicate higher exposure to antioxidants and lower exposure to pro-oxidants (16). Moreover, OBS has been proven by Lingling Song et al. to be correlated with oxidative stress in two NHANES studies (17, 18). A number of epidemiological studies have explored the relationship between OBS and diabetes, non-alcoholic fatty liver disease, periodontitis, lung health, and vascular endothelial function (19–24). In addition, the molecular mechanisms underlying oxidative stress in relation to obesity have been widely acknowledged, such as altering regulatory factors of mitochondrial activity, promoting lipogenesis, stimulating the differentiation of preadipocytes into mature adipocytes, and regulating appetite-related hypothalamic neuron energy balance (25).
BMI, an inadequate indicator of obesity, fails to differentiate between fat mass, fat-free mass, and distribution of adipose tissue. In addition to BMI, total abdominal fat mass (TAFM) and visceral adipose tissue mass (VATM) are important indicators of abdominal obesity and visceral fat accumulation. Meanwhile, differences persist between different adipose tissues, including anatomical, cellular, molecular, physiological, clinical, and prognostic differences, leading to an elevated risk of diabetes and cardiovascular diseases in abdominal obesity compared to that in peripheral or gluteofemoral obesity (26). To the best of our knowledge, there is a lack of studies investigating the relationship between OBS and obesity. Furthermore, there is a dearth of studies that have assessed the associations between OBS and abdominal obesity, or visceral fat accumulation measured by whole-body fat distribution. Therefore, this study aimed to explore the associations between OBS and TAFM and VATM percentages in U.S. adults using the National Health and Nutrition Examination Survey (NHANES).
2 Materials and methods
2.1 Study populations
NHANES is a consecutive, population-based survey conducted by the National Center for Health Statistics of the Centers for Disease Control and Prevention that assesses nutrition and health status collected every 2 years in the U.S. population. NHANES consists of demographics, dietary, examination, laboratory, and questionnaire data, providing detailed information about demographic characteristics, socioeconomic status, physiological measurements, biochemical indicators, and standardized questionnaires about health in various aspects (27). To make the survey representative of the national population, a complex, multistage, probability sampling design with oversampling of different subpopulations is adopted, and corresponding weights are generated. Furthermore, the compensation for participants enables NHANES to collect reliable and high-quality data, which ensures the accuracy and validity of the information.1
To carry out this study, we included adults aged 20 years and older who had complete information about TAFM, VATM, and OBS in four cycles from NHANES 2011–2018. Details of the current study’s sampling and exclusion criteria are described in Figure 1. A total of 39,156 participants were initially included, of which 16,539 were aged less than 20 years old. After excluding participants with missing values of TAFM or VATM (n = 10,645), without two dietary recalls (n = 2,164), with missing values of BMI (n = 21), serum cotinine (n = 348), education level (n = 1), and family income level (n = 704), the final sample included 8,734 adults. All participants in NHANES provided written informed consent, and only publicly available data were used in the current analysis, so no ethical approval was needed in this study.
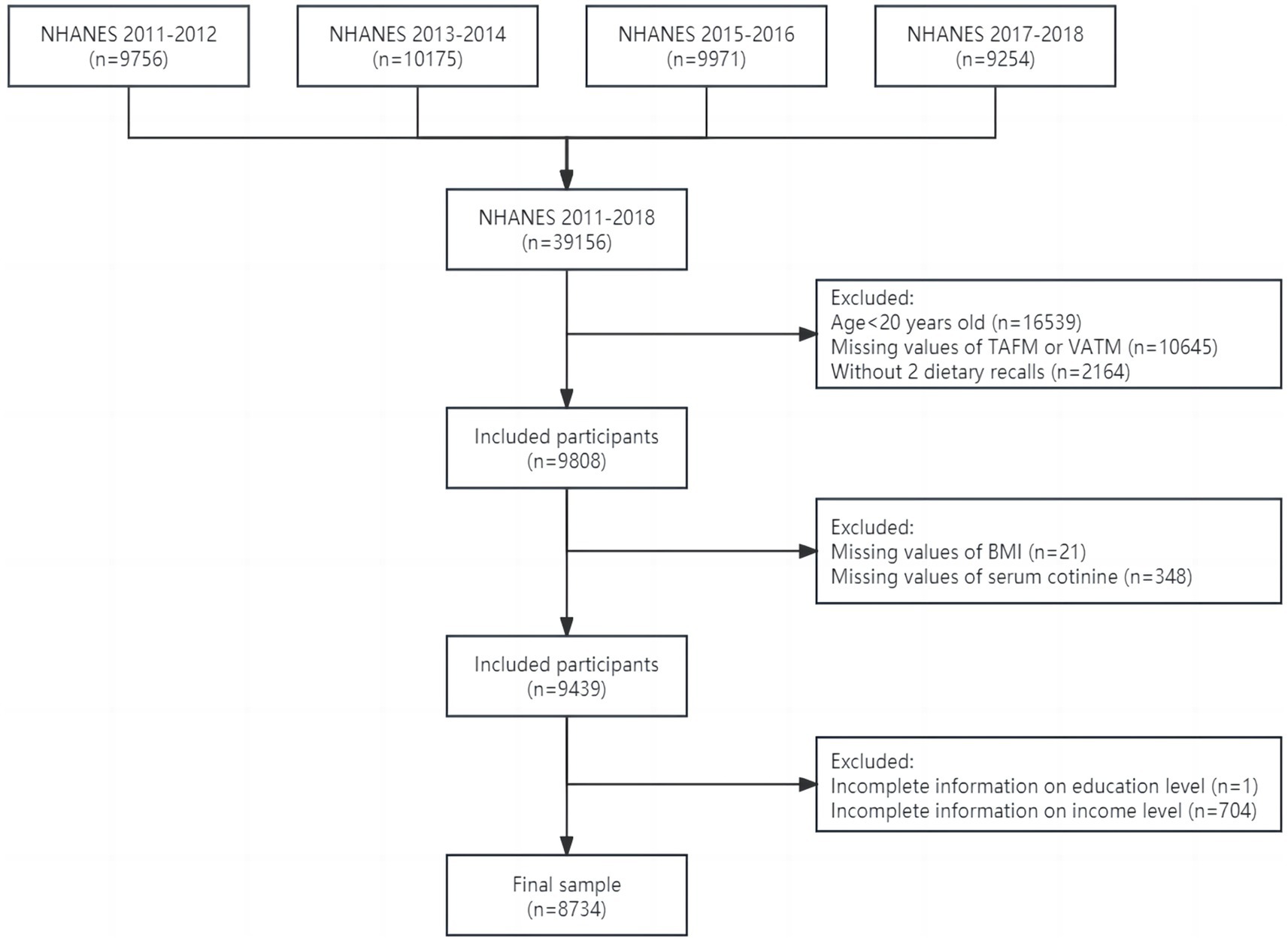
Figure 1. Flowchart of study design and exclusion criteria. BMI, body mass index; NHANES, National Health and Nutrition Examination Survey; TAFM, total abdominal fat mass; VATM, visceral adipose tissue mass.
2.2 Outcome ascertainment
TAFM and VATM were measured using dual-energy x-ray absorptiometry in participants aged 8–59 years old, which was the most widely accepted method to measure body composition due in part to its speed, simplicity, and low radiation exposure (28). Participants underwent whole-body dual-energy x-ray scans in the NHANES mobile examination center, in which visceral adipose tissue and subcutaneous adipose tissue were defined by the Hologic APEX software used in the scan analysis. For subsequent weighted logistic regression models, the medians of TAFM and VATM percentages in body weight were calculated, and participants were categorized into high and low percentage groups based on medians.
2.3 Exposures
OBS was initially established by Zhang et al. (16) and has been extensively validated and widely used in previous studies. It is composed of 16 dietary and 4 lifestyle factors (Supplementary Table S1). Dietary OBS includes fiber, carotene, riboflavin, niacin, vitamin B6, total folate, vitamin B12, vitamin C, vitamin E, calcium, magnesium, zinc, copper, selenium, total fat, and iron, and lifestyle OBS is composed of BMI, physical activity, alcohol consumption, and smoking status. According to the oxidative properties of these 20 factors, they could additionally be categorized into pro-oxidants (total fat, iron, alcohol intake, BMI, and smoking) and antioxidants (the other 15 components).
Dietary nutrient intakes and alcohol consumption were calculated with the mean of 2 24-h dietary recalls. BMI was calculated as weight in kilograms divided by height in meters squared, and smoking status was assessed by serum cotinine with an isotope-dilution high-performance liquid chromatography/atmospheric pressure chemical ionization tandem mass spectrometric method. In terms of physical activity, we specifically focused on leisure-time physical activity (LTPA), which was calculated with the formula: two times vigorous physical activity (VPA) plus moderate physical activity (MPA), as 1 min of VPA was defined as equivalent to 2 min of MPA according to PA guidelines (29).
OBS components were assigned scores by sex and tertiles, in which the highest tertile was scored 2 and the lowest tertile was scored 0, while the intermediate tertile was scored 1 in antioxidants, and the scoring method in pro-oxidants was just the opposite. OBS was obtained by summing the total scores of 20 components, and a higher OBS indicates a higher level of antioxidant exposure. In addition, OBS was further categorized with quartiles, and the lowest quartile was set as the reference group for comparison in the weighted logistic regression models.
2.4 Assessment of covariates
Demographic variables include sex (male and female), age group (young adults, 20–39 years; middle-aged adults, 40–59 years) race (non-Hispanic White, non-Hispanic Black, Mexican Americans, and other races), education level (less than high school degree, high school degree, and more than high school degree), family income level (measured as the ratio of family income to poverty (PIR), low family income, PIR ≤ 1.3; middle family income, 1.3 < PIR < 3.5; high family income, PIR ≥ 3.5), and marital status (married or living with partner; divorced, separated, or widowed; never married). Additionally, total energy intake (expressed as kilocalories) and an array of chronic non-communicable diseases, including hypertension, cardiovascular diseases, and diabetes, were included due to the associations with TAFM and VATM. Hypertension was defined as average systolic pressure ≥ 140 mm Hg and/or diastolic pressure ≥ 90 mm Hg in three tests or self-reported hypertension. Cardiovascular diseases were defined as a self-reported doctor’s diagnosis of congestive heart failure, coronary heart disease, angina, myocardial infarction, or stroke. Diabetes was defined as fasting plasma glucose ≥7.0 mmol/L, 2-h plasma glucose ≥11.0 mmol/L, hemoglobin A1c ≥ 6.5%, or self-reported diabetes by a professional doctor (27, 30–32).
2.5 Statistical analysis
Concerning the complex sampling design in NHANES, dietary 2-day sample weight, clustering, and stratification were taken into account. Dietary 2-day sample weight divided by 4 was utilized to ensure the current study results are representative of the national population on account of the combination of four consecutive cycles.
Continuous variables were expressed as weighted means (standard deviations), and categorical variables were presented with numbers (weighted percentages) in characteristic descriptions. To compare the characteristics across quartiles, analyses of variance and Rao-Scott adjusted chi-square tests were used to test the differences in characteristics between adults with different OBS quartiles.
Both univariate and multivariate weighted logistic regression models were employed to explore the relationships between OBS and TAFM with VATM percentages in the general population. Model 1 was adjusted for demographic data (sex, age group, race, education level, income level, and marital status), and Model 2 was additionally adjusted for healthy eating index-2015 (HEI-2015) and total energy intake, while Model 3 further expanded the adjustments of disease conditions (hypertension, cardiovascular diseases, and diabetes) based on Model 2. Trend tests (p for trend) were performed by entering the quartile-categorical OBS as a continuous variable and rerunning the corresponding regression models. Three sensitivity analyses were further conducted to validate the robustness of our results: (1) missing values except for TAFM, VATM, and OBS were interpolated using the random forest interpolation methodology to reduce the potential selection bias induced by missing values in other variables; (2) we explored the immediate correlations of OBS with high TAFM and VATM without converting TAFM and VATM into percentages; and (3) we replaced BMI by sedentary duration as one of the lifestyle factors to build a revised OBS considering the drastic impact of BMI on TAFM and VATM percentages. Stratified analyses were conducted to investigate whether the associations differ by demographic variables (gender, age, race, education level, income level, and marital status), and interaction effects were tested with a likelihood test. Moreover, dietary OBS and lifestyle OBS were separately employed to assess the associations between TAFM and VATM percentages. Weighted restricted cubic spline analyses were utilized to examine the non-linear correlations of OBS with TAFM and VATM percentages, while non-linearity was assessed using the Wald test.
Stata software (version 17.0, StataCorp LLC) was utilized for most statistical analyses except for analyses of variance in R. All statistical tests were two-sided, and significance was considered at α = 0.05.
3 Results
3.1 Characteristics
Characteristics of adults grouped by quartiles of OBS are presented in Table 1. The final sample included 8,734 young and middle-aged adults representative of 134.7 million non-institutionalized U.S. adults (4,291 [weighted 50.9%] men; 3,299 [weighted 62.3%] non-Hispanic white; mean [SD] TAFM percentage, 2.53% [0.85%]; mean [SD] VATM percentage, 0.59% [0.26%]). Meanwhile, the medians of TAFM and VATM percentages were 2.53 and 0.553%, respectively.
In comparison to the lowest OBS quartile, adults in the higher quartiles were more likely to be non-Hispanic white, married or living with a partner, less likely to be comorbid with hypertension, cardiovascular diseases and diabetes, had a higher education level, family income, HEI-2015 scores, and total energy intake, and had lower TAFM and VATM percentages. Nevertheless, no significant differences in sex and age groups were observed across quartiles.
3.2 Associations between OBS and TAFM with VATM percentages
As described in Table 2, weighted logistic regression analyses revealed the negative association of continuous OBS and OBS quartiles with TAFM and VATM percentages. Compared with the lowest quartile of OBS, the second (OR: 0.70, 95%CI: 0.53–0.94), the third (OR: 0.49, 95%CI: 0.36–0.60), and the highest quartiles (OR: 0.25, 95%CI: 0.18–0.36) of OBS were associated with lower risks of high TAFM percentage in the fully adjusted model (Model 3). Similarly, the third (OR: 0.70, 95%CI: 0.54–0.92) and highest quartiles (OR: 0.56, 95%CI: 0.41–0.77) of OBS were associated with lower risks of high VATM percentage in Model 3 in comparison to the lowest quartile of OBS, while no significant differences were observed between the second quartile and the lowest quartile of OBS.
Moreover, all three sensitivity analyses showed similar correlations and trends in Table 3, indicating the robustness of the associations. To be more specific, the second, third, and highest OBS quartiles were associated with a reduced risk of high TAFM percentage, and the third and highest quartiles were related to a lower risk of high VATM percentage, while no protective role of the second quartile of OBS against high VATM percentage was exhibited.
3.3 Subgroup analyses and interaction effects of the associations between OBS and TAFM with VATM percentages
Tables 4, 5 exhibit the associations between OBS and TAFM with VATM percentages in demographic subpopulations in the fully adjusted models. Higher OBS quartiles were correlated with lower risks of high TAFM percentage in all subgroups, and we observed the trends in ORs across OBS quartiles except for adults with high school education level. In addition, significant interaction effects of education (p = 0.0390) and income (p = 0.0031) on the relationships between OBS and the risks of a high TAFM percentage were discovered, while no other interactions were inferred. Higher OBS quartiles were associated with lower risks of high VATM percentage in various demographic subpopulations, and the trends across OBS quartiles were not present in Mexican Americans, adults with less than or equal to high school education level, adults with low and medium family income, and divorced, separated, or widowed and never married adults. Furthermore, significant interaction effects of sex (p = 0.0198) and education (p = 0.0423) were shown, manifesting as the ORs in male adults and adults with higher than high school education levels being the smallest in corresponding subpopulations.
3.4 Associations between dietary OBS and lifestyle OBS with TAFM and VATM percentages
To evaluate the individual protective effects of dietary OBS and lifestyle OBS separately, weighted logistic regression models were employed, and the results are shown in Table 6. When compared with the lowest quartile of dietary OBS in the fully adjusted model, both the third (OR: 0.71, 95%CI: 0.55–0.93) and the highest (OR: 0.59, 95%CI: 0.41–0.84) quartiles of dietary OBS were associated with lower risks of high TAFM percentage in Model 3, with the trends of ORs across OBS quartiles being observed. Meanwhile, the second (OR: 0.60, 95%CI: 0.47–0.76), the third (OR: 0.39, 95%CI: 0.29–0.51), and the highest (OR: 0.15, 95%CI: 0.11–0.18) quartiles of lifestyle OBS were strongly negatively associated with lower risks of high TAFM percentage.
In comparison to adults of the lowest quartile of dietary OBS in Model 3, adults of the third (OR: 0.66, 95%CI: 0.51–0.85) and highest (OR: 0.56, 95%CI: 0.42–0.73) quartiles had 34 and 44% reduced risks of high VATM percentage, while no such effect was observed in adults in the second quartile. Apart from this, adults of the second (OR: 0.79, 95%CI: 0.67–0.94), the third (OR: 0.69, 95%CI: 0.57–0.84), and the highest (OR: 0.30, 95%CI: 0.24–0.38) quartiles of lifestyle OBS had 21, 31, and 70% reduced risks, respectively.
3.5 Non-linear associations between OBS, dietary OBS, and lifestyle OBS with TAFM and VATM
Weighted restricted cubic splines were conducted to assess the non-linear associations of OBS, dietary OBS, and lifestyle OBS with the risks of high TAFM and VATM percentages, the results of which are displayed in Figure 2. Figures 2A–C show a significant non-linear correlation of OBS, dietary OBS, and lifestyle OBS with TAFM percentage, with higher OBS reflecting lower ORs. Similarly, the significant non-linear relationship between OBS, dietary OBS, and lifestyle OBS with VATM percentage is demonstrated in Figures 2D–F. The results suggest that as OBS, dietary OBS, and lifestyle OBS increase, the risks of high TAFM and VATM percentages decrease in a non-linear manner. Meanwhile, it must be pointed out that the protective role of dietary OBS against TAFM and VATM percentages was not significant when it was relatively low or medium.
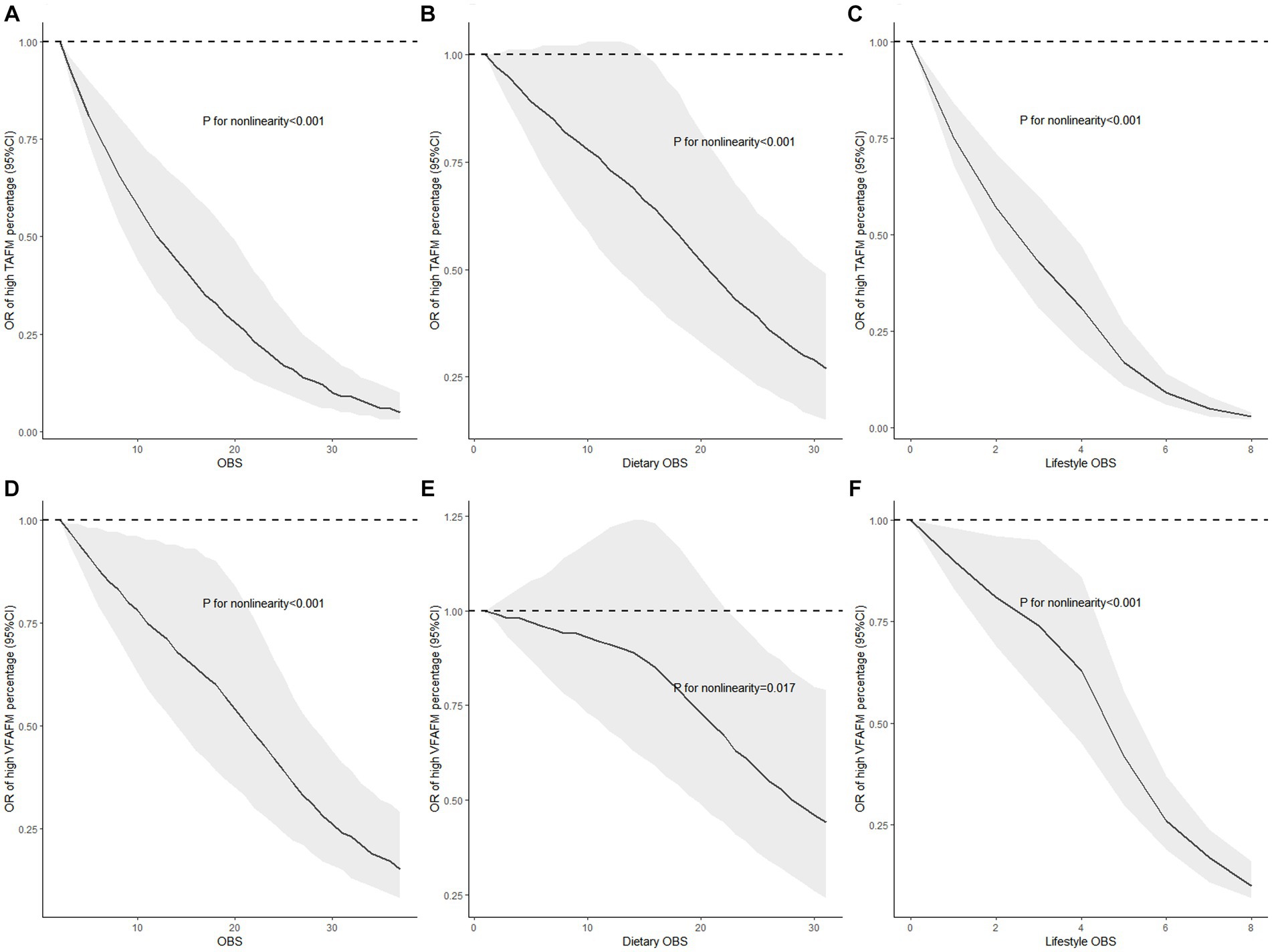
Figure 2. Non-linear associations of OBS, dietary OBS, and lifestyle OBS with the risks of high TAFM and VATM with restricted cubic splines. (A) OBS and TAFM percentage; (B) dietary OBS and TAFM percentage; (C) lifestyle OBS and TAFM percentage; (D) OBS and VATM percentage; (E) dietary OBS and VATM percentage; and (F) lifestyle OBS and VATM percentage. Models were fully adjusted for demographic data (sex, age group, race, education level, income level, and marital status), total energy intake, HEI-2015, and disease conditions (hypertension, cardiovascular diseases, and diabetes); TAFM, total abdominal fat mass; VATM, visceral adipose tissue mass.
4 Discussions
With the large cross-sectional survey, we found that OBS, dietary OBS, and lifestyle OBS were all strongly negatively associated with the risks of high TAFM and VATM percentages in U.S. young and middle-aged adults, and the results of three sensitivity analyses strengthened the robustness of the results. Moreover, the protective role of OBS against TAFM and VATM percentages was consistent in various demographic subgroups, and weighted restricted cubic splines revealed that the higher the OBS, dietary OBS, and lifestyle OBS, the lower the risks of high TAFM and VATM percentages.
OBS, based on dietary and lifestyle factors but not endogenous antioxidants or pro-oxidants, was adopted in our study to speculate on the individual oxidative stress level. OBS was proven to be positively related to leukocyte telomere length in females when it was developed, while telomere length was reported by multiple experiments and reviews to be correlated with oxidative stress (33–35). In addition, the associations of OBS with oxidative stress were verified in a study that assessed the mediation role of oxidative stress between OBS and cognitive function, as well as another study of the mediation role of oxidative stress between OBS and depressive symptoms (17, 18). Indeed, the original OBS was first developed in 2002, and it only included vitamin C, beta-carotene, and iron (36). With the deepening of research on antioxidants and oxidants, OBS was expanded and updated, considering part or all of dietary, biomarkers, lifestyle, and medication to include a wider range of factors in relation to oxidative stress, while the OBS utilized in our study was based on NHANES data, in which sex-specific differences were taken into account (37).
Numerous studies have explored the association between oxidative stress and obesity, and most researchers have approved the correlation, similar to our results. Marseglia L found an inverse association between body fat, central adiposity, and serum total antioxidant capacity in a population-based study (38). Moreover, a study of 2,367 Korean adults found a positive relationship between abdominal obesity and oxidative stress measured by derivatives of reactive oxygen metabolite concentration, in which abdominal obesity was thought to increase oxidative stress and affect the signaling pathways involved in obesity (39). Nevertheless, studies about visceral fat accumulation were relatively rare. A cross-sectional study found a relationship between visceral obesity and lipoperoxidation with oxidative DNA damage but not endogenous antioxidant defenses (40).
In addition, the associations of OBS with TAFM and VATM percentages were robust in demographic subpopulations, and the ORs of VATM in male adults were lower, indicating a stronger protective effect of OBS in men. Women were found to possess a lower oxidative stress level, lower oxidative stress biomarkers, lower reactive oxygen species production, and greater antioxidant potential, suggesting that women were less likely to be susceptible to oxidative stress (41–45). Meanwhile, women are more resistant than men to several diseases involving oxidative stress, and cell-autonomous mechanisms also contribute to the resistance of female cells to oxidative stress-induced apoptosis, suggesting that women have a higher ability to handle oxidative stress (46). Specifically, men have a higher oxidative stress level than women, and the reduction of oxidative stress levels across quartiles of OBS in men is greater than that in women. As a result, stronger changes in oxidative stress levels across quartiles in men lead to greater decreasing ORs, showing a stronger protective effect of OBS in men. Nevertheless, more studies are required to validate our sex-specific hypothesis and explore whether the interaction effects between education level and TAFM and VATM are accidental.
Some dietary nutrients, such as fiber, folate, vitamin C, total fats, calcium, magnesium, and copper, were thought to reduce the obesity risk, but more studies focused on serum levels, while dietary selenium intake was not shown to be correlated with obesity (47–53). Nevertheless, a population-based ecological study concluded that long-term exposure to high levels of B vitamins may be involved in the increased prevalence of obesity in the United States (54). Iron deficiency was found to be positively correlated with obesity, and iron metabolism was altered in obese people, even if iron promoted oxidative stress as a pro-oxidant (55, 56). Consequently, in-depth mechanism studies about the complex signaling pathways of iron metabolism, oxidative stress, and obesity were urgently required. Lifestyle OBS, consisting of physical activity, alcohol intake, BMI, and cotinine, was a platitude related to obesity (57). We replaced BMI with sedentary duration to eliminate the exceptionally strong effect of BMI, and the association persisted. Based on previous research, sedentary duration may also be a lifestyle indicator of oxidative stress (58). Furthermore, non-linear correlations between OBS, dietary OBS, and lifestyle OBS with risks of high TAFM and VATM percentages were exhibited in weighted restricted cubic splines, and the relationships between dietary OBS and the risks of high TAFM and VATM percentages were not linear and did not exist in adults with low and medium dietary OBS, suggesting that the protective effects may vary at different levels of dietary OBS and adults should maintain at relatively higher dietary OBS to reduce the risks of high TAFM and VATM percentages.
The findings underline the significance of adhering to an antioxidant diet and lifestyle in reducing the risks of high TAFM and VATM percentages indicating abdominal obesity and visceral fat accumulation, and it is crucial to make the adults aware of the strong association and thus encourage them to provide possible preventive measures and make necessary changes.
The major strength of this study is the use of a large, nationally representative U.S. survey and the combination of data in four cycles, increasing the sample size and enlarging the generalizability of our results. Furthermore, the adoption of subgroup analyses, interaction effects, and sensitivity analyses enhances the robustness and credibility of our results. Finally, restricted cubic splines demonstrated significant non-linear dose–response relationships, which were more intuitive. Nevertheless, it cannot be denied that our study also has some limitations. First, only associations rather than causality could be inferred from this study since NHANES were cross-sectional studies, and further longitudinal or interventional studies are required to better understand the causal relationship. Second, more prospective and high-quality studies are urgently needed to evaluate the effectiveness of OBS. Additionally, the heterogeneity in the definition of an OBS results in contradictory results among OBS studies for some outcomes. Consequently, some covariates were not based on clinical data but self-reported, the reliability of which is relatively weak. Finally, quartiles of OBS were used in our study population, weakening its comparability to other studies with different cut-points.
Data availability statement
The raw data supporting the conclusions of this article will be made available by the authors under reasonable requirements, without undue reservation.
Author contributions
KW: Data curation, Formal analysis, Methodology, Software, Supervision, Validation, Visualization, Writing – original draft. MD: Data curation, Formal analysis, Writing – review & editing. JW: Writing – review & editing. LL: Writing – review & editing. RC: Conceptualization, Methodology, Writing – review & editing. FL: Writing – review & editing. JN: Data curation, Formal analysis, Writing – review & editing. FT: Writing – review & editing. QL: Writing – review & editing. XL: Writing – review & editing. FX: Writing – review & editing.
Funding
The author(s) declare that no financial support was received for the research, authorship, and/or publication of this article.
Acknowledgments
We thank all the participants for their participation in this study.
Conflict of interest
The authors declare that the research was conducted in the absence of any commercial or financial relationships that could be construed as a potential conflict of interest.
Publisher’s note
All claims expressed in this article are solely those of the authors and do not necessarily represent those of their affiliated organizations, or those of the publisher, the editors and the reviewers. Any product that may be evaluated in this article, or claim that may be made by its manufacturer, is not guaranteed or endorsed by the publisher.
Supplementary material
The Supplementary material for this article can be found online at: https://www.frontiersin.org/articles/10.3389/fnut.2023.1306428/full#supplementary-material
Footnotes
References
1. Jaacks, LM, Vandevijvere, S, Pan, A, McGowan, CJ, Wallace, C, Imamura, F, et al. The obesity transition: stages of the global epidemic. Lancet Diabetes Endocrinol. (2019) 7:231–40. doi: 10.1016/S2213-8587(19)30026-9
2. Caballero, B . Humans against obesity: who will win? Adv Nutr. (2019) 10:S4–9. doi: 10.1093/advances/nmy055
3. Hales, CM, Fryar, CD, Carroll, MD, Freedman, DS, and Ogden, CL. Trends in obesity and severe obesity prevalence in us youth and adults by sex and age, 2007–2008 to 2015–2016. JAMA. (2018) 319:1723–5. doi: 10.1001/jama.2018.3060
4. GA Bray, KK Kim, and W JPH, World Obesity F eds. Obesity: a chronic relapsing progressive disease process. A position statement of the world obesity federation. Obes Rev. (2017) 18:715–23. doi: 10.1111/obr.12551
5. Maggio, CA, and Pi-Sunyer, FX. Obesity and type 2 diabetes. Endocrinol Metab Clin N Am. (2003) 32:805–22, viii. doi: 10.1016/s0889-8529(03)00071-9
6. Piche, ME, Tchernof, A, and Despres, JP. Obesity phenotypes, diabetes, and cardiovascular diseases. Circ Res. (2020) 126:1477–500. Epub 20200521. doi: 10.1161/CIRCRESAHA.120.316101
7. Yang, Y, Xian, W, Wu, D, Huo, Z, Hong, S, Li, Y, et al. The role of obesity, type 2 diabetes, and metabolic factors in gout: a mendelian randomization study. Front Endocrinol (Lausanne). (2022) 13:917056. doi: 10.3389/fendo.2022.917056
8. Polyzos, SA, Kountouras, J, and Mantzoros, CS. Obesity and nonalcoholic fatty liver disease: from pathophysiology to therapeutics. Metabolism. (2019) 92:82–97. doi: 10.1016/j.metabol.2018.11.014
9. Avgerinos, KI, Spyrou, N, Mantzoros, CS, and Dalamaga, M. Obesity and cancer risk: emerging biological mechanisms and perspectives. Metabolism. (2019) 92:121–35. doi: 10.1016/j.metabol.2018.11.001
10. Ruze, R, Liu, T, Zou, X, Song, J, Chen, Y, Xu, R, et al. Obesity and type 2 diabetes mellitus: connections in epidemiology, pathogenesis, and treatments. Front Endocrinol (Lausanne). (2023) 14:1161521. doi: 10.3389/fendo.2023.1161521
11. Kalinkovich, A, and Livshits, G. Sarcopenic obesity or obese sarcopenia: a cross talk between age-associated adipose tissue and skeletal muscle inflammation as a main mechanism of the pathogenesis. Ageing Res Rev. (2017) 35:200–21. doi: 10.1016/j.arr.2016.09.008
12. Tomlinson, DJ, Erskine, RM, Morse, CI, Winwood, K, and Onambele-Pearson, G. The impact of obesity on skeletal muscle strength and structure through adolescence to old age. Biogerontology. (2016) 17:467–83. doi: 10.1007/s10522-015-9626-4
13. Collaborators, GBDO, Afshin, A, Forouzanfar, MH, Reitsma, MB, Sur, P, Estep, K, et al. Health effects of overweight and obesity in 195 countries over 25 years. N Engl J Med. (2017) 377:13–27. doi: 10.1056/NEJMoa1614362
14. Dai, H, Alsalhe, TA, Chalghaf, N, Ricco, M, Bragazzi, NL, and Wu, J. The global burden of disease attributable to high body mass index in 195 countries and territories, 1990–2017: an analysis of the global burden of disease study. PLoS Med. (2020) 17:e1003198:e1003198. doi: 10.1371/journal.pmed.1003198
15. Sies, H . Oxidative stress: a concept in redox biology and medicine. Redox Biol. (2015) 4:180–3. doi: 10.1016/j.redox.2015.01.002
16. Zhang, W, Peng, SF, Chen, L, Chen, HM, Cheng, XE, and Tang, YH. Association between the oxidative balance score and telomere length from the national health and nutrition examination survey 1999–2002. Oxidative Med Cell Longev. (2022) 2022:1345071–11. doi: 10.1155/2022/1345071
17. Song, L, Li, H, Fu, X, Cen, M, and Wu, J. Association of the oxidative balance score and cognitive function and the mediating role of oxidative stress: evidence from the National Health and Nutrition Examination Survey (NHANES) 2011–2014. J Nutr. (2023) 153:1974–83. doi: 10.1016/j.tjnut.2023.05.014
18. Li, H, Song, L, Cen, M, Fu, X, Gao, X, Zuo, Q, et al. Oxidative balance scores and depressive symptoms: mediating effects of oxidative stress and inflammatory factors. J Affect Disord. (2023) 334:205–12. doi: 10.1016/j.jad.2023.04.134
19. Wu, C, Ren, C, Song, Y, Gao, H, Pang, X, and Zhang, L. Gender-specific effects of oxidative balance score on the prevalence of diabetes in the us population from NHANES. Front Endocrinol (Lausanne). (2023) 14:1148417. doi: 10.3389/fendo.2023.1148417
20. Kwon, YJ, Park, HM, and Lee, JH. Inverse association between oxidative balance score and incident type 2 diabetes mellitus. Nutrients. (2023) 15:2497. doi: 10.3390/nu15112497
21. Cho, AR, Kwon, YJ, and Lee, JH. Oxidative balance score is inversely associated with the incidence of non-alcoholic fatty liver disease. Clin Nutr. (2023) 42:1292–300. doi: 10.1016/j.clnu.2023.06.015
22. Qu, H . The association between oxidative balance score and periodontitis in adults: a population-based study. Front Nutr. (2023) 10:1138488. doi: 10.3389/fnut.2023.1138488
23. Xu, Z, Xue, Y, Wen, H, and Chen, C. Association of oxidative balance score and lung health from the National Health and Nutrition Examination Survey 2007–2012. Front Nutr. (2022) 9:961950. doi: 10.3389/fnut.2022.961950
24. Liu, J, He, L, Wang, A, Lv, Y, He, H, Wang, C, et al. Oxidative balance score reflects vascular endothelial function of Chinese community dwellers. Front Physiol. (2023) 14:1076327. doi: 10.3389/fphys.2023.1076327
25. Perez-Torres, I, Castrejon-Tellez, V, Soto, ME, Rubio-Ruiz, ME, Manzano-Pech, L, and Guarner-Lans, V. Oxidative stress, plant natural antioxidants, and obesity. Int J Mol Sci. (2021) 22:1786. doi: 10.3390/ijms22041786
26. Ibrahim, MM . Subcutaneous and visceral adipose tissue: structural and functional differences. Obes Rev. (2010) 11:11–8. doi: 10.1111/j.1467-789X.2009.00623.x
27. Wang, K, Xia, F, Li, Q, Luo, X, and Wu, J. The associations of weekend warrior activity patterns with the visceral adiposity index in us adults: repeated cross-sectional study. JMIR Public Health Surveill. (2023) 9:e41973. doi: 10.2196/41973
28. Nana, A, Slater, GJ, Stewart, AD, and Burke, LM. Methodology review: using dual-energy X-ray absorptiometry (DXA) for the assessment of body composition in athletes and active people. Int J Sport Nutr Exerc Metab. (2015) 25:198–215. doi: 10.1123/ijsnem.2013-0228
29. Piercy, KL, Troiano, RP, Ballard, RM, Carlson, SA, Fulton, JE, Galuska, DA, et al. The physical activity guidelines for Americans. JAMA. (2018) 320:2020–8. doi: 10.1001/jama.2018.14854
30. Patel, JS, Oh, Y, Rand, KL, Wu, W, Cyders, MA, Kroenke, K, et al. Measurement invariance of the patient health questionnaire-9 (Phq-9) depression screener in U.S. adults across sex, race/ethnicity, and education level: NHANES 2005–2016. Depress Anxiety. (2019) 36:813–23. doi: 10.1002/da.22940
31. Deng, MG, Liu, F, Liang, Y, Chen, Y, Nie, JQ, Chai, C, et al. Associations of serum zinc, copper, and selenium with sleep disorders in the American adults: data from NHANES 2011–2016. J Affect Disord. (2023) 323:378–85. doi: 10.1016/j.jad.2022.11.088
32. Nie, J, Deng, MG, Wang, K, Liu, F, Xu, H, Feng, Q, et al. Higher Hei-2015 scores are associated with lower risk of gout and hyperuricemia: results from the National Health and Nutrition Examination Survey 2007–2016. Front Nutr. (2022) 9:921550. doi: 10.3389/fnut.2022.921550
33. Houben, JM, Moonen, HJ, van Schooten, FJ, and Hageman, GJ. Telomere length assessment: biomarker of chronic oxidative stress? Free Radic Biol Med. (2008) 44:235–46. doi: 10.1016/j.freeradbiomed.2007.10.001
34. Lin, J, and Epel, E. Stress and telomere shortening: insights from cellular mechanisms. Ageing Res Rev. (2022) 73:101507. doi: 10.1016/j.arr.2021.101507
35. Gordon, CA, Madamanchi, NR, Runge, MS, and Jarstfer, MB. Effect of oxidative stress on telomere maintenance in aortic smooth muscle cells. Biochim Biophys Acta Mol basis Dis. (2022) 1868:166397. doi: 10.1016/j.bbadis.2022.166397
36. Van Hoydonck, PG, Temme, EH, and Schouten, EG. A dietary oxidative balance score of vitamin C, beta-carotene and iron intakes and mortality risk in male smoking Belgians. J Nutr. (2002) 132:756–61. doi: 10.1093/jn/132.4.756
37. Hernandez-Ruiz, A, Garcia-Villanova, B, Guerra-Hernandez, E, Amiano, P, Ruiz-Canela, M, and Molina-Montes, E. A review of a priori defined oxidative balance scores relative to their components and impact on health outcomes. Nutrients. (2019) 11:774. doi: 10.3390/nu11040774
38. Chrysohoou, C, Panagiotakos, DB, Pitsavos, C, Skoumas, I, Papademetriou, L, Economou, M, et al. The implication of obesity on total antioxidant capacity in apparently healthy men and women: the attica study. Nutr Metab Cardiovasc Dis. (2007) 17:590–7. doi: 10.1016/j.numecd.2006.05.007
39. Na, IJ, Park, JS, and Park, SB. Association between abdominal obesity and oxidative stress in Korean adults. Korean J Fam Med. (2019) 40:395–8. doi: 10.4082/kjfm.18.0086
40. Garcia-Sanchez, A, Gamez-Nava, JI, Diaz-de la Cruz, EN, Cardona-Munoz, EG, Becerra-Alvarado, IN, Aceves-Aceves, JA, et al. The effect of visceral abdominal fat volume on oxidative stress and proinflammatory cytokines in subjects with Normal weight, overweight and obesity. Diabetes Metab Syndr Obes. (2020) 13:1077–87. doi: 10.2147/DMSO.S245494
41. Barp, J, Araujo, AS, Fernandes, TR, Rigatto, KV, Llesuy, S, Bello-Klein, A, et al. Myocardial antioxidant and oxidative stress changes due to sex hormones. Braz J Med Biol Res. (2002) 35:1075–81. doi: 10.1590/s0100-879x2002000900008
42. Ide, T, Tsutsui, H, Ohashi, N, Hayashidani, S, Suematsu, N, Tsuchihashi, M, et al. Greater oxidative stress in healthy young men compared with premenopausal women. Arterioscler Thromb Vasc Biol. (2002) 22:438–42. doi: 10.1161/hq0302.104515
43. Matarrese, P, Colasanti, T, Ascione, B, Margutti, P, Franconi, F, Alessandri, C, et al. Gender disparity in susceptibility to oxidative stress and apoptosis induced by autoantibodies specific to Rlip76 in vascular cells. Antioxid Redox Signal. (2011) 15:2825–36. doi: 10.1089/ars.2011.3942
44. Bhatia, K, Elmarakby, AA, El-Remessy, AB, and Sullivan, JC. Oxidative stress contributes to sex differences in angiotensin ii-mediated hypertension in spontaneously hypertensive rats. Am J Physiol Regul Integr Comp Physiol. (2012) 302:R274–82. doi: 10.1152/ajpregu.00546.2011
45. Sumien, N, Cunningham, JT, Davis, DL, Engelland, R, Fadeyibi, O, Farmer, GE, et al. Neurodegenerative disease: roles for sex, hormones, and oxidative stress. Endocrinology. (2021) 162:bqab185. doi: 10.1210/endocr/bqab185
46. Tower, J, LCD, P, and KJA, D. Sex differences in the response to oxidative and proteolytic stress. Redox Biol. (2020) 31:101488. doi: 10.1016/j.redox.2020.101488
47. Schafer, AL, Eichhorst, A, Hentze, C, Kraemer, AN, Amend, A, Sprenger, DTL, et al. Low dietary fiber intake links development of obesity and lupus pathogenesis. Front Immunol. (2021) 12:696810. doi: 10.3389/fimmu.2021.696810
48. Pereira, GA, Bressan, J, Oliveira, FLP, Sant'Ana, HMP, Pimenta, AM, Lopes, LL, et al. Dietary folate intake is negatively associated with excess body weight in Brazilian graduates and postgraduates (CUME project). Nutrients. (2019) 11:518. doi: 10.3390/nu11030518
49. Garcia-Diaz, DF, Lopez-Legarrea, P, Quintero, P, and Martinez, JA. Vitamin C in the treatment and/or prevention of obesity. J Nutr Sci Vitaminol (Tokyo). (2014) 60:367–79. doi: 10.3177/jnsv.60.367
50. Celis-Morales, CA, Lyall, DM, Gray, SR, Steell, L, Anderson, J, Iliodromiti, S, et al. Dietary fat and total energy intake modifies the association of genetic profile risk score on obesity: evidence from 48 170 Uk biobank participants. Int J Obes. (2017) 41:1761–8. doi: 10.1038/ijo.2017.169
51. Tremblay, A, and Gilbert, JA. Human obesity: is insufficient calcium/dairy intake part of the problem? J Am Coll Nutr. (2011) 30:449S–53S. doi: 10.1080/07315724.2011.10719989
52. Pelczynska, M, Moszak, M, and Bogdanski, P. The role of magnesium in the pathogenesis of metabolic disorders. Nutrients. (2022) 14:1714. doi: 10.3390/nu14091714
53. Wang, W, Liu, L, Shan, R, and Wang, C. Associations between dietary copper intake, general obesity and abdominal obesity risk: a nationwide cohort study in China. Front Nutr. (2022) 9:1009721. doi: 10.3389/fnut.2022.1009721
54. Zhou, SS, Li, D, Zhou, YM, Sun, WP, and Liu, QG. B-vitamin consumption and the prevalence of diabetes and obesity among the us adults: population based ecological study. BMC Public Health. (2010) 10:746. doi: 10.1186/1471-2458-10-746
55. Zhao, L, Zhang, X, Shen, Y, Fang, X, Wang, Y, and Wang, F. Obesity and iron deficiency: a quantitative meta-analysis. Obes Rev. (2015) 16:1081–93. Epub 20150923. doi: 10.1111/obr.12323
56. Qiu, F, Wu, L, Yang, G, Zhang, C, Liu, X, Sun, X, et al. The role of iron metabolism in chronic diseases related to obesity. Mol Med. (2022) 28:130. doi: 10.1186/s10020-022-00558-6
57. Wadden, TA, Tronieri, JS, and Butryn, ML. Lifestyle modification approaches for the treatment of obesity in adults. Am Psychol. (2020) 75:235–51. doi: 10.1037/amp0000517
Keywords: abdominal adiposity, oxidative balance score, total abdominal fat mass, visceral adipose tissue mass, visceral fat accumulation
Citation: Wang K, Deng M, Wu J, Luo L, Chen R, Liu F, Nie J, Tao F, Li Q, Luo X and Xia F (2023) Associations of oxidative balance score with total abdominal fat mass and visceral adipose tissue mass percentages among young and middle-aged adults: findings from NHANES 2011–2018. Front. Nutr. 10:1306428. doi: 10.3389/fnut.2023.1306428
Edited by:
Sandra M. Colorado-Yohar, CIBER Epidemiología y Salud Pública (CIBERESP), SpainReviewed by:
Silvia Isabel Rech Franke, Universidade de Santa Cruz do Sul, BrazilDongdong Zhang, Shenzhen Luohu People's Hospital, China
Niloufar Rasaei, Tehran University of Medical Sciences, Iran
Copyright © 2023 Wang, Deng, Wu, Luo, Chen, Liu, Nie, Tao, Li, Luo and Xia. This is an open-access article distributed under the terms of the Creative Commons Attribution License (CC BY). The use, distribution or reproduction in other forums is permitted, provided the original author(s) and the copyright owner(s) are credited and that the original publication in this journal is cited, in accordance with accepted academic practice. No use, distribution or reproduction is permitted which does not comply with these terms.
*Correspondence: Fang Xia, MTYyMTM4NzM0MkBxcS5jb20=