- 1Department of Nutrition, Universidade Federal de Minas Gerais, Belo Horizonte, Brazil
- 2Department of Psychiatry and Behavioral Sciences, The University of Texas Health Science Center, Houston, TX, United States
Introduction: Migraine is a common and disabling primary headache, and its pathophysiology is not fully understood. Previous studies have suggested that pain can increase humans’ Resting Energy Expenditure (REE). However, no previous study has investigated whether the REE of individuals with migraine differs from the general population. Therefore, this study aims to assess whether the REE of women with migraine differs from that of women without headaches. We also tested the accuracy of REE predictive formulas in the migraine patients.
Methods: This cross-sectional study involves 131 adult women aged between 18 and 65 years, 83 with migraine and 48 without (controls). We collected clinical, demographic, and anthropometric data. Migraine severity was measured using the Migraine Disability Test and Headache Impact Test, version 6. The REE was measured by indirect calorimetry, and it was compared with the predicted REE calculated by formulas.
Results: Patients with migraine had higher REE when compared to controls (p < 0.01). There was a positive correlation between REE and the patient-reported number of migraine attacks per month (Rho = 0.226; p = 0.044). Mifflin-St Jeor and Henry and Rees were the predictive formulas that have more accuracy in predicting REE in women with migraine.
Discussion: Considering the benefits of nutritional interventions on treating migraines, accurately measuring REE can positively impact migraine patient care. This study enhances our understanding of the relationship between pain and energy expenditure. Our results also provide valuable insights for healthcare professionals in selecting the most effective predictive formula to calculate energy expenditure in patients with migraine.
Introduction
Migraine is a common and disabling primary headache. Migraine attacks last from four to 72 h and are typically characterized by unilateral location pulsating quality, moderate or severe intensity, associated with nausea, photophobia, and phonophobia, and can be accompanied by visual disturbance called aura (1).
The migraine pathophysiology is not fully understood. One widely accepted theory suggests that endogenous or exogenous factors can promote the activation of trigeminal afferent fibers that innervate cranial structures. Trigeminal activation induces the release of vasoactive neuropeptides by sensory fibers triggering a cascade events with the release of inflammatory mediators such as bradykinin and prostaglandins, leading to neurogenic inflammation (2).
Previous studies have shown that pain can increase the Resting Energy Expenditure (REE) in humans (3–6). For instance, Holland-Fischer et al. (5) evaluated the effect of non-traumatic skin pain caused by transcutaneous painful electrical stimulation on REE and energy substrate utilization in 10 healthy volunteers. Pain caused an acute and reversible increase by 62% in REE, probably mediated by adrenergic activity and increased muscle tone. Older studies involving critically ill patients have also shown that pain increases energy expenditure, while analgesia and sedation decrease energy expenditure (3, 4, 6). Recent studies have further suggested that pain increases energy expenditure during routine daily activities (7, 8). In a study conducted by Ko et al. (7), older adults with knee pain consumed more oxygen while walking than those without pain, indicating an increase in energy expenditure. However, the association between energy expenditure and pain remains a largely unexplored area in the literature.
Despite the evidence suggesting the effect of pain on REE, no previous study has investigated whether the energy expenditure of individuals with migraine differs from the general population. However, a clear understanding of the pattern of energy expenditure in patients with migraine is relevant for the adequacy of dietary interventions, especially knowing that nutritional intervention may provide benefits in treating migraines (9–12).
Therefore, the primary objective of this study was to assess whether the REE of women with migraine differs from that of women without migraine. The secondary aim was to investigate the accuracy of predictive formulas to determine REE in women with migraine.
Methods
This cross-sectional study involves adult women aged between 18 and 65 years, both with a diagnosis of migraine and without (control). We chose to include only women in our sample due to the higher prevalence and greater severity of migraine among this population (1, 13).
A neurologist diagnosed patients with migraine according to the International Classification of Headache Disorders - 2nd edition (1). Exclusion criteria were: (i) Other Headaches (not characterized as migraine); (ii) Any chronic health conditions (e.g., diabetes mellitus, arterial hypertension, chronic renal failure, and endocrine diseases such as hypo- or hyperthyroidism); (iii) Pregnancy and breastfeeding; (iv) Smoking, and (v) Alcohol abuse (> 2 doses/day). Patients were evaluated during a headache-free period.
This study took place at the Headache Outpatient Clinic of Hospital das Clínicas, Universidade Federal de Minas Gerais (UFMG) (Belo Horizonte, Minas Gerais, Brazil). Ethical approval for this study was obtained from the Human Research Ethics Committee of UFMG (CAAE: 28236814.3.0000.5149), and all procedures were conducted in accordance with the principles of the Declaration of Helsinki.
Demographic and clinical data
Clinical and demographic data (age, sex, marital status, years of education, physical activity in minutes per week, and the patient-reported number of migraine attacks per month) were collected using a semi-structured questionnaire specifically created for this study. Migraine severity was measured using the Migraine Disability Test (MIDAS) and Headache Impact Test, version 6 (HIT-6). The MIDAS provides information about the impact of headaches on the number of days lost in social, domestic, and work activities in the last 3 months. The MIDAS scores were classified into: no or little disability (a score up to 5), mild disability (a score from 6 to 10), moderate disability (a score from 11 to 20), and severe disability (a score above 21) (14). The HIT-6 measures the incapacity caused by headaches in the previous month. The HIT-6 scores were classified into: little or no impact (scores up to 49), some impact (a score from 50 to 55), substantial impact (a score from 56 to 59), and very severe impact (a score above 60) (15).
Anthropometric evaluation
The body weight and height were measured, and the Body Mass Index (BMI = weight/height2) was calculated. Patients were classified as follows: Underweight (BMI ≤ 18.5), Normal Weight (BMI from 18.5 to 24.9), Overweight (BMI from 25.0 to 29.9), or Obesity (BMI ≥ 30) (16).
After an overnight fast, body composition was evaluated by electric bioimpedance using a Biodynamics® (model 310e) device. The test was performed in a comfortable and quiet room. The patients were instructed to: (i) not to exercise at least 24 h before and to abstain from alcoholic beverages, coffee, or black tea (ii) to remain to lie still throughout the test. The percentage of fat mass was classified according to the categories proposed by Shea et al. (17).
Assessment of resting energy expenditure
As validated by previous studies, REE was defined by Indirect Calorimetry using the MetaCheckTM device (model 7,100; Korr Medical Technologies, Salt Lake City, UT, United States). Patients underwent the evaluation in the morning after overnight fasting. Patients were seated in a comfortable position and breathing through a disposable mouthpiece or mask attached to the device. Nasal clips were used to ensure that all air passed through the mouthpiece. Patients were instructed to relax, breathe normally, avoid coughing, and minimize their movements until the test was completed. The test duration was approximately 20 min. Oxygen consumption was measured, and the REE was calculated using the equation proposed by Weir with an assumed respiratory quotient of 0.83 (18).
Since body composition affects REE (19), the measured REE (mREE) was corrected for lean body mass (mREE/FFM). The correction was made by dividing the mREE (in kcal/dia) by fat-free mass (in kilograms).
Finally, mREE was compared with the predicted REE (pREE) calculated using the formulas presented in Table 1. These formulas were selected based on their research and clinical relevance (20–30).
Statistical analyses
Categorical variables were described as frequencies (%) and compared using Chi-square or Fisher’s exact test. The distribution of quantitative variables was tested using the Kolmogorov–Smirnov for analyses of all the groups (sample size > 50) and Shapiro–Wilk tests for analyses just with the data of the migraine group (sample size < 50) (31). Variables with a normal distribution (parametric) are presented as means (±standard deviation) and non-parametric variables are presented as medians (minimum-maximum). Independent Sample t-test and Mann–Whitney were used to compare of mean and median between groups, respectively. Pearson’s or Spearman’s coefficients were calculated to assess the correlation between parametric and non-parametric variables, respectively. A Binary Logistic Regression test was performed to adjust the comparison of mREE/FFM values between groups by weekly minutes of physical activity. Paired Student’s t-test (parametric variables) and Wilcoxon’s test (non-parametric variables) were used to compare mREE and pREE.
In addition, the 95% confidence interval (95% CI) of the difference between pREE and mREE was calculated. The pREE was considered accurate when it was between 90 and 110% of the mREE at the individual level. pREE values lower than 90% or higher than 110% of mREE were classified as underestimated and overestimated, respectively (20, 32–35). The mean percentage difference between pREE and mREE (bias) was considered the measure of accuracy at the group level and the individual accuracy was determined ≥50%. The level of agreement between mREE and pREE was examined using frequencies and quartile similarities and the Bland and Altman method (36). We use the Linear Regression analyses for investigated the proportionality bias between the formulas.
The statistical analyses were performed using SPSS, version 19 (IBM Corp., Armonk, NY, United States) and p < 0.05 was considered statistically significant.
Our simple size was enough to obtain a statistical power greater than >90% for mREE/FFM, considering the simple size minimum of 48 individuals per group, independent sample t-test, and alpha error of 5%.
Results
Clinical data
Eighty-three women diagnosed with episodic migraine and 48 controls were enrolled in the study. The presence of aura was identified in 59.0% (n = 49) of the patients with migraine. The median (range) age was 30 (19–65) and 33 (18–65) years old for the migraine and control groups, respectively (p = 0.101). Most participants were single and had graduate degrees (p = 0.477 and p = 0.445 comparison between groups, respectively). The groups were similar in body composition parameters (Table 2). Most patients with migraine were classified with “moderate to severe disability” according to MIDAS (67%) and “substantial or very severe impact” according to HIT (94%).
Patients with migraine had higher mREE/FFM when compared to controls (30.92 ± 4.99 vs. 24.51 ± 4.34, respectively; p < 0.001) (Figure 1). The mREE/FFM positively correlated with the number of migraine attacks per month (Rho = 0.226; p = 0.044), but not with HIT (Rho = 0.080, p = 0.472) and MIDAS (Rho = 0.114, p = 0.313). The physical activity was different between groups, however, the difference in mREE/FFM between the groups remains after adjustment for minutes of physical activity per week (p < 0.001).
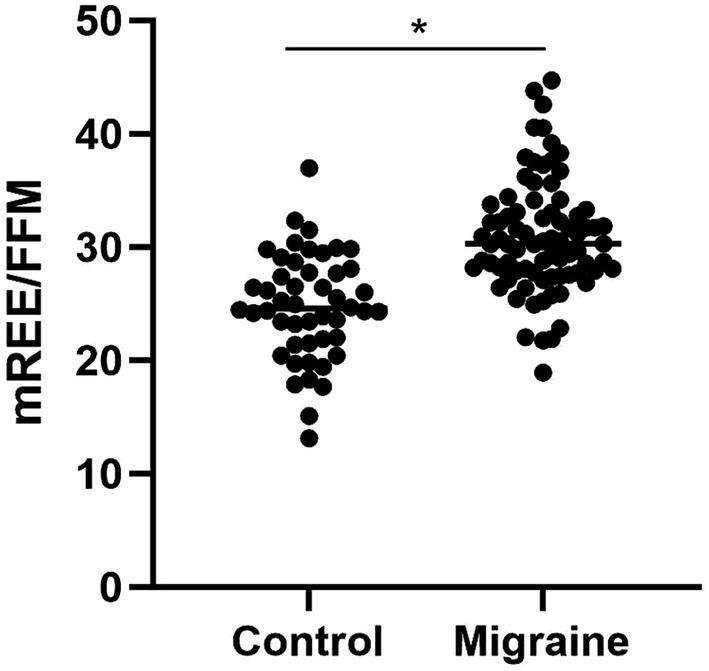
Figure 1. Comparison of resting energy expenditure per kilogram of fat-free mass (REE/FFM) between women with and without migraine. The symbol * in the figure represents that there was a statistical difference (p<0.001).
Predictive formulas to estimate REE
Considering that the mREE of women with migraine differed from women without migraine, we evaluated the accuracy of REE predictive formulas for this population.
The mean mREE of female patients with migraine was 1326.22 (±243.75) kcal/day. Only 25% of the formulas were able to predict the REE similar to the mREE. The Mifflin-St Jeor formula had the lowest mean difference (7.69 ± 184.52) kcal/day compared to mREE. Individual accuracy (precision) was >50% for the Mifflin-St Jeor, Owen, Schofield, and Henry and Rees formulas.
The formula that most underestimated the REE was Owen’s (30.1%), while Cunningham (54.2%), Harris & Benedict (47.0%), and FAO WH (45.8%) overestimated the REE as shown in Table 3.
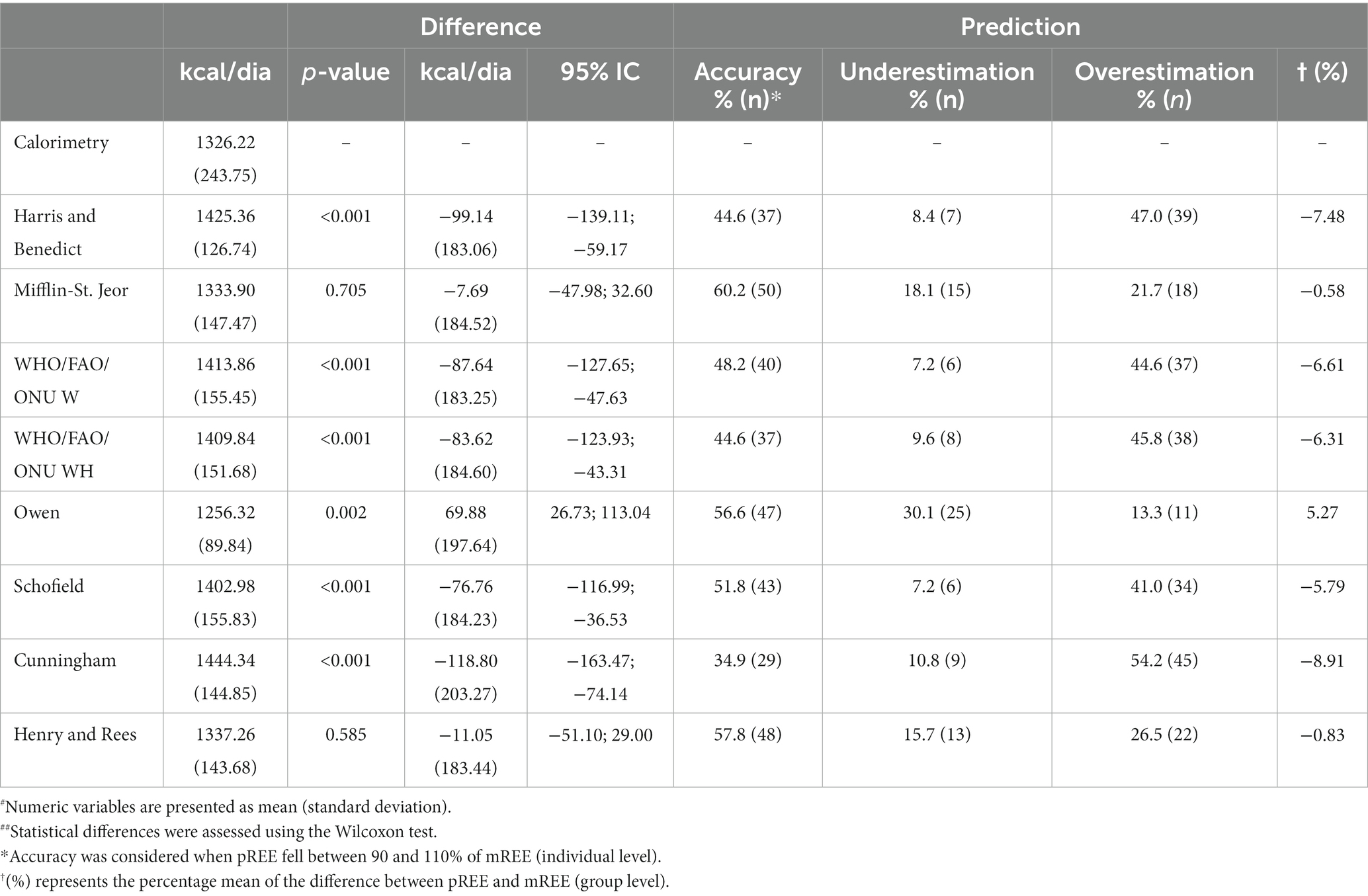
Table 3. Comparison between resting energy expenditure measured by indirect calorimetry and calculated by predictive formulas in women with migraine.
Figure 2 shows the individual agreement between mREE and pREE by quartile similarity in women with migraine. All formulas presented individual accuracy higher than 60%. Four equations (50.0%) showed no agreement between the mREE lowest quartile. Cunningham showed the highest individual agreement for the highest values measured by IC (28.80%). The pREE by Mifflin-St. Jeor and Henry and Rees and Owen showed individual agreement for all quartiles of the mREE.
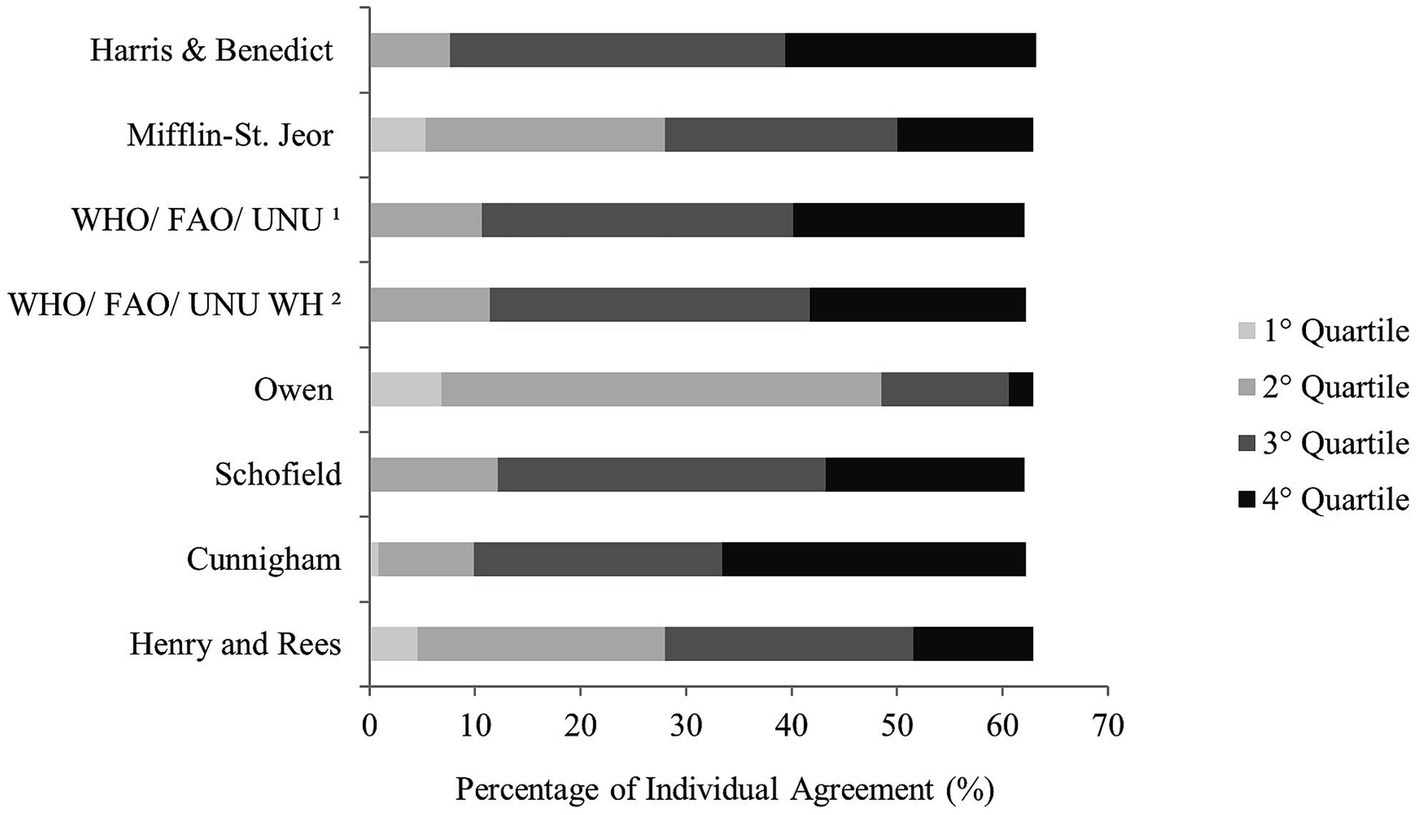
Figure 2. Percentage of individual agreement between resting energy expenditure measured by indirect calorimetry and predictive formulas by quartile in women with migraine.
Discussion
In this study, we showed that women with migraine had a higher REE when compared to individuals without migraine. The mREE/FFM positively correlated with the number of migraine attacks, indicating that the more episodes of pain, the higher the patient’s REE. Regarding the predictive formulas, Mifflin-St Jeor and Henry and Rees were those that have more accuracy to predict REE in patients with migraine. To the best of our knowledge, this is the first study to evaluate differences in the REE in patients with migraine and the adequacy of predictive formulas.
Our findings are in line with the aforementioned previous studies that suggested that pain increases energy expenditure (3–6). In our study, we observed a positive correlation between mREE/FFM and the frequency of migraine attacks. In our study, we observed a positive correlation between mREE/FFM and the frequency of migraine attacks. This indicates that higher energy expenditure is linked to the frequency of pain rather than its severity, as assessed by HIT and MIDAS, in patients with episodic migraines. Indeed, previous studies have suggested the presence of an energy metabolism disturbance in migraines, potentially caused by mitochondrial dysfunction, which may be associated with increased neuronal excitability and susceptibility to migraines (37–39). Interestingly, a recent study observed a high prevalence of lifetime migraine (61%) among patients affected by mitochondrial disorders and suggested that migraine may be a clinical manifestation of brain energy dysfunction (37). Our findings corroborate the theory that migraine is associated with energy metabolism disturbance. However, more studies are needed to explore the link between mitochondrial dysfunction, REE, and migraine. Furthermore, it’s worth noting that in our present study, patients were evaluated during pain-free periods. Therefore, another intriguing aspect to explore in future investigations is the relationship between REE and pain intensity during migraine attacks.
Nutrition plays a crucial role in migraine pathophysiology, as certain foods have been identified as triggers for migraine attacks (40). Furthermore, overweight has been linked to the severity of migraines and is influenced by diet (12). Accurately predicting REE can assist dietitians and nutritionists in providing more effective guidance on healthier dietary patterns for patients with migraines. While indirect calorimetry is considered the gold standard method for determining REE, its use in clinical practice is limited due to cost, time requirements, and the need for trained staff to perform the examination. Therefore, predictive equations remain the most used tool for estimating REE (20, 41, 42). In this study, we assessed the accuracy of various predictive formulas in determining REE in patients with migraine. Our findings suggest that the Mifflin-St Jeor and Henry and Rees formulas demonstrated the highest accuracy at the individual level, lower mean percentage differences at the group level, and better representation in classifying adequacy by quartile for the migraine group. Cunningham’s (28) formula was included to explore whether using lean mass in the calculation improves formula accuracy. However, our results indicated that Cunningham’s formula had the highest percentage of overestimation of mREE in women with migraine. Although Cunningham’s formula was originally developed using data from participants of the Harris-Benedict study (i.e., predominantly normal-weight women, excluding male athletes), previous studies have shown high accuracy when this formula is applied to estimate REE in athletes (43, 44). Considering that migraine patients, in general, may have less muscle mass than athletes, the coefficient of 22 in the Cunningham formula may be excessive for them, leading to an overestimation of REE.
The distinct characteristics of the reference population used to establish the predictive formulas may be a reason that justifies the discrepancy between them. REE can be influenced by several demographic, clinical, and environmental factors, including sex, age, race, physical activity level, diet, body composition, obesity-related comorbidities, and environmental temperatures (19, 45). Of note, the reference population used to develop Henry and Rees formula was composed by mostly normal-weight individuals with varied ethnicities and from tropical countries, resembling our sample. On the other hand, the Mifflin-St Jeor equation was developed based on a sample that included individuals of both sexes, ranging from 19 to 78 years old, with normal weight and overweight, from a US cohort. The accuracy of these formulas in comparison to indirect calorimetry to measure REE was tested in various groups within the Brazilian population, yielding mixed results. While most studies suggested that the Henry and Rees or Mifflin-St Jeor formulas tend to overestimate REE in a Brazilian sample of adults (46, 47), patients with severe obesity (48, 49), nonalcoholic fatty liver disease (50), postmenopausal women (51), and adolescent football athletes (52), results from other studies involving patients with pulmonary hypertension (53), type 2 diabetes (54), and liver transplant recipients (55) indicated an underestimation of these formulas when compared with REE measured by calorimetry.
Of note, in the current study, we used a reduced steady-state system to assess REE. We opted for this approach since previous research had employed the same equipment to assess the reliability of predictive formulas within the Brazilian population (35, 56). Nevertheless, other systems that measure a five-minute steady state over a 30-min interval may offer a more precise REE measurement (57). Given that this is the initial study to evaluate the accuracy of predictive formulas in individuals with migraine, further investigations employing different REE measurement systems are warranted to validate our findings.
The current study is subject to certain limitations, including its cross-sectional design, the exclusive inclusion of women with episodic migraine in the sample, and the absence of biomarkers such as triiodothyronine and thyroxine that are associated with REE. However, to ensure the validity of our findings, we carefully selected participants by excluding individuals with metabolic diseases and thyroid abnormalities. Furthermore, as aforementioned, we chose to include only women in our sample due to the higher prevalence and greater severity of migraine among women (1, 13). Therefore, to further explore the relationship between REE and pain mechanisms in patients with migraine, future longitudinal studies encompassing both men and women are warranted.
Given the potential impact of nutritional interventions in reducing the impact and severity of migraines (12), it is crucial to have a reliable tool for determining resting energy expenditure (REE) when developing personalized dietary intervention. In clinical practice, the selection of the most accurate REE predictive formulas specific to a particular population is essential. Our findings not only support existing literature on the relationship between pain and increased energy expenditure but also demonstrate that the Mifflin-St Jeor and Henry and Rees predictive formulas are particularly accurate for women with migraines. However, further longitudinal studies involving chronic migraine patients and other pain-related disorders are needed to validate these results.
Data availability statement
The raw data supporting the conclusions of this article will be made available by the authors, without undue reservation.
Ethics statement
The studies involving humans were approved by Human Research Ethics Committee of UFMG (CAAE: 28236814.3.0000.5149). The studies were conducted in accordance with the local legislation and institutional requirements. The participants provided their written informed consent to participate in this study.
Author contributions
LM: Conceptualization, Data curation, Investigation, Methodology, Supervision, Writing – review & editing, Funding acquisition. JR: Conceptualization, Data curation, Writing – original draft, Funding acquisition. AR: Data curation, Writing – original draft. LS: Data curation, Writing – original draft. AT: Writing – review & editing. AF: Writing – review & editing, Conceptualization.
Funding
The author(s) declare financial support was received for the research, authorship, and/or publication of this article. This study was funded by the Brazilian government funding agencies: Conselho Nacional de Desenvolvimento Científico e Tecnológico (CNPq), Coordenação de Aperfeiçoamento de Pessoal de Nível Superior (CAPES) and Fundação de Amparo à Pesquisa do Estado de Minas Gerais a Pesquisa do Estado de Minas Gerais (FAPEMIG). The Neuropsychiatry Program is funded by the UT Health Houston, Department of Psychiatry and Behavioral Sciences.
Acknowledgments
The authors would like to thank for the students Isabel Myrian Guimarães Campos, Tamara Carneiro Medeiros de Souza, Luciana Alves de Andrade, Jenneffer Rayane Braga Tibães, and Matheus Amaral for their help during data collection.
Conflict of interest
The authors declare that the research was conducted in the absence of any commercial or financial relationships that could be construed as a potential conflict of interest.
The author(s) declared that they were an editorial board member of Frontiers, at the time of submission. This had no impact on the peer review process and the final decision.
Publisher’s note
All claims expressed in this article are solely those of the authors and do not necessarily represent those of their affiliated organizations, or those of the publisher, the editors and the reviewers. Any product that may be evaluated in this article, or claim that may be made by its manufacturer, is not guaranteed or endorsed by the publisher.
References
1. Headache Classification Committee of the International Headache Society (IHS) . The international classification of headache disorders, 3rd edition. Cephalalgia. (2018) 38:1–211. doi: 10.1177/0333102417738202
2. Ferrari, MD , Goadsby, PJ , Burstein, R , Kurth, T , Ayata, C , Charles, A, et al. Migraine. Nat Rev Dis Prim. (2022) 8:2. doi: 10.1038/s41572-021-00328-4
3. Weissman, C , Kemper, M , Damask, MC , Askanazi, J , Hyman, AI , and Kinney, JM . Effect of routine intensive care interactions on metabolic rate. Chest. (1984) 86:815–8. doi: 10.1378/chest.86.6.815
4. Weissman, C , Kemper, M , Elwyn, DH , Askanazi, J , Hyman, AI , and Kinney, JM . The energy expenditure of the mechanically ventilated critically ill patient. Chest. (1986) 89:254–9. doi: 10.1378/chest.89.2.254
5. Holland-Fischer, P , Greisen, J , Grøfte, T , Jensen, TS , Hansen, PO , and Vilstrup, H . Increased energy expenditure and glucose oxidation during acute nontraumatic skin pain in humans. Eur J Anaesthesiol. (2009) 26:311–7. doi: 10.1097/EJA.0b013e328324b5e9
6. Bruder, N , Lassegue, D , Pelissier, D , Graziani, N , and Francois, G . Energy expenditure and withdrawal of sedation in severe head-injured patients. Crit Care Med. (1994) 22:1114–9. doi: 10.1097/00003246-199407000-00011
7. Ko, S , Simonsick, EM , and Ferrucci, L . Gait energetic efficiency in older adults with and without knee pain: results from the Baltimore longitudinal study of aging. Age (Omaha). (2015) 37:15. doi: 10.1007/s11357-015-9754-4
8. Gardner, AW , Ritti-Dias, RM , Stoner, JA , Montgomery, PS , Scott, KJ , and Blevins, SM . Walking economy before and after the onset of claudication pain in patients with peripheral arterial disease. J Vasc Surg. (2010) 51:628–33. doi: 10.1016/j.jvs.2009.09.053
9. De CL, NL , Ferraris, C , Catalano, G , Guglielmetti, M , Pasca, L , Pezzotti, E, et al. Ketosis and migraine: a systematic review of the literature and meta-analysis. Front Nutr. (2023) 10:10. doi: 10.3389/fnut.2023.1204700
10. Liu, H , Wang, D , Wu, F , Dong, Z , and Yu, S . Association between inflammatory potential of diet and self-reported severe headache or migraine: a cross-sectional study of the National Health and nutrition examination survey. Nutrition. (2023) 113:112098. doi: 10.1016/j.nut.2023.112098
11. Balali, A , Karimi, E , Kazemi, M , Hadi, A , Askari, G , Khorvash, F, et al. Associations between diet quality and migraine headaches: a cross-sectional study. Nutr Neurosci. (2023) 1–11. doi: 10.1080/1028415X.2023.2244260
12. Costa, ABP , Rodrigues, AMDS , Martins, LB , Dos Santos, LC , Gomez, RS , Teixeira, AL, et al. Nutritional intervention may improve migraine severity: a pilot study. Arq Neuropsiquiatr. (2019) 77:723–30. doi: 10.1590/0004-282X20190121
13. Vos, T , Flaxman, AD , Naghavi, M , Lozano, R , Michaud, C , Ezzati, M, et al. Years lived with disability (YLDs) for 1160 sequelae of 289 diseases and injuries 1990-2010: a systematic analysis for the global burden of disease study 2010. Lancet. (2012) 380:2163–96. doi: 10.1016/S0140-6736(12)61729-2
14. Fragoso, YD . MIDAS (migraine disability assessment): a valuable tool for work-site identification of migraine in workers in Brazil. São Paulo Med J. (2002) 120:118–21. doi: 10.1590/S1516-31802002000400006
15. Martin, M , Blaisdell, B , Kwong, JW , and Bjorner, JB . The short-form headache impact test (HIT-6) was psychometrically equivalent in nine languages. J Clin Epidemiol. (2004) 57:1271–8. doi: 10.1016/j.jclinepi.2004.05.004
16. World Health Organization. Obesity: preventing and managing the global epidemic. Report of a WHO consultation. World Health Organ Tech Rep Ser. (2000) 894:i–xii, 1–253.
17. Shea, JL , Randell, EW , and Sun, G . The prevalence of metabolically healthy obese subjects defined by BMI and dual-energy X-ray absorptiometry. Obesity. (2011) 19:624–30. doi: 10.1038/oby.2010.174
18. De V, WJB . New methods for calculating metabolic rate with special reference to protein metabolism. J Physiol. (1949) 109:1–9. doi: 10.1113/jphysiol.1949.sp004363
19. National Academies of Sciences; Engineering and Medicine; Health and Medicine Division; Food and Nutrition Board; Committee on the Dietary Reference Intakes for Energy . Factors affecting energy expenditure and requirements. Nationl Academic Press (2023). Available at: https://www.ncbi.nlm.nih.gov/books/NBK591031/ (Accessed July 27, 2023).
20. Frankenfield, D , Roth-Yousey, L , and Compher, C . Comparison of predictive equations for resting metabolic rate in healthy nonobese and obese adults: a systematic review. J Am Diet Assoc. (2005) 105:775–89. doi: 10.1016/j.jada.2005.02.005
21. Frankenfield, DC . Bias and accuracy of resting metabolic rate equations in non-obese and obese adults. Clin Nutr. (2013) 32:976–82. doi: 10.1016/j.clnu.2013.03.022
22. Schofield, WN . Predicting basal metabolic rate, new standards and review of previous work. Hum Nutr Clin Nutr. (1985) 39:5–41.
23. Amaro-Gahete, F , Jurado-Fasoli, L , De-la-O, A , Gutierrez, Á , Castillo, M , and Ruiz, J . Accuracy and validity of resting energy expenditure predictive equations in middle-aged adults. Nutrients. (2018) 10:1635. doi: 10.3390/nu10111635
24. Amaro-Gahete, F , Sanchez-Delgado, G , Alcantara, J , Martinez-Tellez, B , Muñoz-Hernandez, V , Merchan-Ramirez, E, et al. Congruent validity of resting energy expenditure predictive equations in young adults. Nutrients. (2019) 11:223. doi: 10.3390/nu11020223
25. Harris, JA , and Benedict, FG . A biometric study of human basal metabolism. Proc Natl Acad Sci. (1918) 4:370–3. doi: 10.1073/pnas.4.12.370
26. Mifflin, M , St Jeor, S , Hill, L , Scott, B , Daugherty, S , and Koh, Y . A new predictive equation for resting energy expenditure in healthy individuals. Am J Clin Nutr. (1990) 51:241–7. doi: 10.1093/ajcn/51.2.241
27. Owen, O , Kavle, E , Owen, R , Polansky, M , Caprio, S , Mozzoli, M, et al. A reappraisal of caloric requirements in healthy women. Am J Clin Nutr. (1986) 44:1–19. doi: 10.1093/ajcn/44.1.1
28. Cunningham, JJ . A reanalysis of the factors influencing basal metabolic rate in normal adults. Am J Clin Nutr. (1980) 33:2372–4. doi: 10.1093/ajcn/33.11.2372
29. Henry, CJ , and Rees, DG . New predictive equations for the estimation of basal metabolic rate in tropical peoples. Eur J Clin Nutr. (1991) 45:177–85.
30. Food and Agriculture Organization, Word Health Organization, United Nations University . Energy and protein requirements: Report of a joint FAO/WHO/UNU expert consultation. Rome: (1981).
31. Mishra, P , Pandey, CM , Singh, U , Gupta, A , Sahu, C , and Keshri, A . Descriptive statistics and normality tests for statistical data. Ann Card Anaesth. (2019) 22:67–72. doi: 10.4103/aca.ACA_157_18
32. Weijs, PJ . Validity of predictive equations for resting energy expenditure in US and Dutch overweight and obese class I and II adults aged 18–65 y. Am J Clin Nutr. (2008) 88:959–70. doi: 10.1093/ajcn/88.4.959
33. Hasson, RE , Howe, CA , Jones, BL , and Freedson, PS . Accuracy of four resting metabolic rate prediction equations: effects of sex, body mass index, age, and race/ethnicity. J Sci Med Sport. (2011) 14:344–51. doi: 10.1016/j.jsams.2011.02.010
34. Weijs, PJM , and Vansant, GAAM . Validity of predictive equations for resting energy expenditure in Belgian normal weight to morbid obese women. Clin Nutr. (2010) 29:347–51. doi: 10.1016/j.clnu.2009.09.009
35. Dos S, RAM , ABP, C , Campos, DL , MPS, S , Cândido, AL , Dos, SLC, et al. Low validity of predictive equations for calculating resting energy expenditure in overweight and obese women with polycystic ovary syndrome. J Hum Nutr Diet. (2018) 31:266–75. doi: 10.1111/jhn.12498
36. Bland, JM , and Altman, DG . Statistical methods for assessing agreement between two methods of clinical measurement. Lancet (London, England). (1986) 1:307–10.
37. Terrin, A , Bello, L , Valentino, ML , Caporali, L , Sorarù, G , Carelli, V, et al. The relevance of migraine in the clinical spectrum of mitochondrial disorders. Sci Rep. (2022) 12:4222. doi: 10.1038/s41598-022-08206-z
38. Fila, M , Pawłowska, E , and Blasiak, J . Mitochondria in migraine pathophysiology – does epigenetics play a role? Arch Med Sci. (2019) 15:944–56. doi: 10.5114/aoms.2019.86061
39. Wang, Y , Wang, Y , Yue, G , and Zhao, Y . Energy metabolism disturbance in migraine: from a mitochondrial point of view. Front Physiol. (2023) 14:14. doi: 10.3389/fphys.2023.1133528
40. Rockett, FC , de Oliveira, VR , Castro, K , Chaves, MLF , Perla, ADS , and Perry, IDS . Dietary aspects of migraine trigger factors. Nutr Rev. (2012) 70:337–56. doi: 10.1111/j.1753-4887.2012.00468.x
41. Jésus, P , Achamrah, N , Grigioni, S , Charles, J , Rimbert, A , Folope, V, et al. Validity of predictive equations for resting energy expenditure according to the body mass index in a population of 1726 patients followed in a nutrition unit. Clin Nutr. (2015) 34:529–35. doi: 10.1016/j.clnu.2014.06.009
42. Wilms, B , Schmid, SM , Ernst, B , Thurnheer, M , Mueller, MJ , and Schultes, B . Poor prediction of resting energy expenditure in obese women by established equations. Metabolism. (2010) 59:1181–9. doi: 10.1016/j.metabol.2009.11.011
43. Thompson, J , and Manore, MM . Predicted and measured resting metabolic rate of male and female endurance athletes. J Am Diet Assoc. (1996) 96:30–4. doi: 10.1016/S0002-8223(96)00010-7
44. ten Haaf, T , and Weijs, PJM . Resting energy expenditure prediction in recreational athletes of 18–35 years: confirmation of Cunningham equation and an improved weight-based alternative. PLoS One. (2014) 9:e108460. doi: 10.1371/journal.pone.0108460
45. Martin-Rincon, M , Perez-Valera, M , Morales-Alamo, D , Perez-Suarez, I , Dorado, C , Gonzalez-Henriquez, JJ, et al. Resting energy expenditure and body composition in overweight men and women living in a temperate climate. J Clin Med. (2020) 9:203. doi: 10.3390/jcm9010203
46. Wahrlich, V , Teixeira, TM , and Dos, ALA . Validity of a population-specific BMR predictive equation for adults from an urban tropical setting. Clin Nutr. (2018) 37:208–13. doi: 10.1016/j.clnu.2016.12.005
47. Anjos, LA , Wahrlich, V , and Vasconcellos, MT . BMR in a Brazilian adult probability sample: the nutrition, physical activity and health survey. Public Health Nutr. (2014) 17:853–60. doi: 10.1017/S1368980012005381
48. de Oliveira, BAP , Nicoletti, CF , de Oliveira, CC , De S, PMA , DCG, Q , Noronha, NY, et al. A new resting metabolic rate equation for women with class III obesity. Nutrition. (2018) 49:1–6. doi: 10.1016/j.nut.2017.11.024
49. Horie, LM , Gonzalez, MC , Torrinhas, RS , Cecconello, I , and Waitzberg, DL . New specific equation to estimate resting energy expenditure in severely obese patients. Obesity. (2011) 19:1090–4. doi: 10.1038/oby.2010.326
50. Oliveira, A , Fernandes, SA , Carteri, RB , and Tovo, CV . Evaluation of rest energy expenditure in patients with non-alcoholic fatty liver disease. Arq Gastroenterol. (2021) 58:157–63. doi: 10.1590/s0004-2803.202100000-27
51. Bonganha, V , Libardi, CA , Santos, CF , De Souza, GV , Conceiçaõ, MS , Chacon-Mikahil, MPT, et al. Predictive equations overestimate the resting metabolic rate in postmenopausal women. J Nutr Health Aging. (2013) 17:211–4. doi: 10.1007/s12603-012-0395-3
52. Oliveira, TM , Penna-Franca, PA , Dias-Silva, CH , Bittencourt, VZ , Cahuê, FFLC , Fonseca-Junior, SJ, et al. Predictive equations for resting metabolic rate are not appropriate to use in Brazilian male adolescent football athletes. PLoS One. (2021) 16:e0244970. doi: 10.1371/journal.pone.0244970
53. Zanella, PB , Ávila, CC , and de Souza, CG . Estimating resting energy expenditure by different methods as compared with indirect calorimetry for patients with pulmonary hypertension. Nutr Clin Pract. (2018) 33:217–23. doi: 10.1177/0884533617727731
54. Grassi, T , Boeno, FP , de Freitas, MM , de Paula, TP , Viana, LV , de Oliveira, AR, et al. Predictive equations for evaluation for resting energy expenditure in Brazilian patients with type 2 diabetes: what can we use? BMC Nutr. (2020) 6:56. doi: 10.1186/s40795-020-00384-1
55. Pinto, AS , Chedid, MF , Guerra, LT , Álvares-da-Silva, MR , De, AA , Guimarães, LS, et al. Estimating basal energy expenditure in liver transplant recipients: the value of the Harris-Benedict equation. ABCD Arq Bras Cir Dig (São Paulo). (2016) 29:185–8. doi: 10.1590/0102-6720201600030013
56. de Oliveira, FCE , Alves, RDM , Zuconi, CP , Ribeiro, AQ , and Bressan, J . Agreement between different methods and predictive equations for resting energy expenditure in overweight and obese Brazilian men. J Acad Nutr Diet. (2012) 112:1415–20. doi: 10.1016/j.jand.2012.06.004
Keywords: resting energy expenditure, migraine, predictive formulas, pain, nutrition
Citation: Martins LB, Ribeiro JS, Rodrigues AMdS, Santos LCd, Teixeira AL and Ferreira AVM (2023) High resting energy expenditure in women with episodic migraine: exploring the use of predictive formulas. Front. Nutr. 10:1296937. doi: 10.3389/fnut.2023.1296937
Edited by:
Montserrat Esteve Ràfols, University of Barcelona, SpainReviewed by:
Lenycia De Cassya Lopes Neri, University of Pavia, ItalyMonica Guglielmetti, University of Pavia, Italy
Copyright © 2023 Martins, Ribeiro, Rodrigues, Santos, Teixeira and Ferreira. This is an open-access article distributed under the terms of the Creative Commons Attribution License (CC BY). The use, distribution or reproduction in other forums is permitted, provided the original author(s) and the copyright owner(s) are credited and that the original publication in this journal is cited, in accordance with accepted academic practice. No use, distribution or reproduction is permitted which does not comply with these terms.
*Correspondence: Laís Bhering Martins, bGFpc2JtbnV0cmlAZ21haWwuY29t; Adaliene Versiani Matos Ferreira, YWRhbGllbmVAZ21haWwuY29t
†These authors have contributed equally to this work