- 1Nancy Atmospera-Walch School of Nursing, University of Hawaii at Manoa, Honolulu, HI, United States
- 2College of Nursing, University of South Florida, Tampa, FL, United States
- 3College of Nursing, University of Tennessee-Knoxville, Knoxville, TN, United States
- 4College of Medicine Pediatrics, Morsani College of Medicine, University of South Florida, Tampa, FL, United States
Introduction: Very Low Birth Weight (VLBW) infants, born weighing less than 1,500 grams, are at risk for both gut dysbiosis and later neuropsychological developmental deficits. Behavioral effects, while related to neurodevelopment, are often more subtle and difficult to measure. The extent of later neurobehavioral consequences associated with such microbial dysbiosis has yet to be determined. We explored associations between the infants’ gut microbiome and early childhood behavior at 4 years of age and identified the bacterial taxa through a multivariate analysis by linear models.
Methods: Parents completed the Child Behavior Checklist (CBCL) focused on different DSM diagnostic categories: affective, anxiety, pervasive developmental, attention deficit/hyperactivity, and oppositional defiant. All the CBCL scores were corrected for gender, delivery method, gestational age, infant birth weight, occurrence of sepsis, and days on antibiotics prior statistical analyses. Canonical correlation analysis (CCA) was performed to determine the relationship between early life gut microbiome and the adjusted CBCL scores. The association of bacterial Amplicon sequence Variants (ASVs) to the CBCL scores were tested with multivariate analysis by linear models (MaAsLin).
Results: Nineteen children who were previously born with very low birth weight and studied while hospitalized in the Neonatal Intensive Care Unit (NICU) were included in this study. Statistically significant associations were observed between early life gut bacteria such as Veillonella dispar, Enterococcus, Escherichia coli, and Rumincococcus to later behavior at 4 years. No significant association could be observed with early-life gut microbiome alpha diversity and behavioral measures at 4 years.
Discussion: These preliminary observational data provide insight into the relationships between VLBW gut microbiome dysbiosis and childhood behavior. This study contributes to the literature on gut microbiome analysis by examining various behavioral domains using a standardized tool linked to the Diagnostic and Statistical Manual of Mental Disorders (DSM).
1 Introduction
Very Low Birth Weight (VLBW) infants confront unique developmental challenges and a heightened risk of experiencing behavioral issues in their future. Their premature birth means that their organ systems, including the brain, might not have fully matured, leading to potential long-term consequences for neurodevelopment (1, 2). Furthermore, many VLBW infants undergo extended stays in the neonatal intensive care unit (NICU), which can result in sensory deprivation and emotional stress during a critical phase of brain development. Additionally, VLBW infants are more prone to medical complications, such as respiratory distress syndrome, intraventricular hemorrhage, and infections, all of which can have lasting impacts on both their brain, behavior, and growth (3–5). Therefore, it is crucial to identify potential biomarkers or other biological patterns to recognize these challenges early in life.
Numerous studies have illuminated the gut-brain axis, involving bidirectional communication between the gut and the brain, which directly and indirectly impacts the host’s growth, development, and behavioral functions (6–8). Consequently, comprehending the role of gut microbiota in infants could be pivotal for averting the future risk of behavioral issues by enabling the provision of suitable nutrition and early intervention.
Our previous study demonstrated a relationship between the gut microbiota of Very Low Birth Weight (VLBW) and neurodevelopment, assessed using the Battelle Development Inventory-2 Screening Test (BDI-2ST) (2). Behavioral effects, while related to neurodevelopment, are often more subtle and difficult to measure. The use of a parent qualitative scale to describe child behavior is a nuanced approach used in the current study. The Child Behavior Checklist (CBCL) is a standardized tool with six scales related to Diagnostic and Statistical Manual of Mental Disorders (DSM) diagnostic categories. The CBCL has been widely for many years and has shown a high accuracy of diagnostic efficiency (9).
The VLBW infants in this study experienced significant gut microbial dysbiosis during the first 6 weeks of life in the Neonatal Intensive Care Unit (NICU) which was characterized by a dominant abundance of Gammaproteobacteria (10). They are more likely to suffer various brain insults and injuries in their early life. Immaturity, coupled with the intensive care that is necessary, predisposes these infants to gut dysbiosis, a disequilibrium of the gut microbial community (11, 12). The roles of the dysbiotic infant gut microbiome in later childhood neurodevelopment and behavior are understudied. Pathogens present during sensitive developmental periods are associated with later anxiety-like behavior and cognitive impairment (13–16). This may happen because proinflammatory bacterial metabolites from the gut can alter the blood brain barrier or cross into the brain, altering microglia, and contributing to the development of neurological injury (17) which then translates into later neurodevelopmental and behavioral problems. Intestinal dysbiosis often includes reduced microbial alpha diversity and increased intestinal barrier permeability (18). Lower alpha diversity is often correlated to poorer health status (19, 20). The stability, diversity, and developmental succession of the early life gut microbiome may be associated with long-term health consequences (21).
This study explored associations between the VLBW infant’s gut microbiome and scales related to the Diagnostic and Statistical Manual of Mental Disorders (DSM) from the Child Behavior Checklist (CBCL) at 4 years old. We also identified the bacterial Amplicon Sequence Variants (ASVs) related to the DSM-related scores. This study adds to the gut microbiome analysis literature by including analysis related to different behavioral DSM-related scales using a standardized tool.
2 Materials and methods
2.1 Study design and participants
Upon approval by the university Institutional Review Board (IRB), parents of VLBW infants admitted to the NICU of a large Florida tertiary care hospital were invited to be in the initial cohort (IRB#Pro00003468, R21 NR013094). Parents gave written informed consent to participate in the study and in additional follow up studies. Eighty-three VLBW infants were measured during the first 6 weeks of their NICU admission. Parents who consented were contacted for the follow-up study (IRB#Pro00019955, NIH grant R01NR015446) that explored relationships between the gut microbiome and later health, growth, and development. A total of 25 VLBW infants were followed from birth to 4 years of age. Home visits were done, and multiple types of data were collected. In the current paper, we report on data collected in the NICU, including stool microbiome data, and later behavioral outcomes at 4 years of age. In 19 cases, there were complete data from the 5 and 6 weeks of life for the microbiome analysis, adjustments, and behavioral follow-ups at 4 years of age. Supplementary Figure 1 shows a flow diagram describing clearly the longitudinal follow up design.
2.2 Sample processing for measurement of infant and childhood follow up of stool microbiome
Infant stool samples were collected weekly from diapers during the first 6 weeks of life and aliquots were stored at −80 C prior to sequencing. At the 4 year home visit the investigators collected stool samples from the children and their mothers. Stool was collected from the diaper or from the toilet using the ALPCO Easy Sampler® Stool Collection kit. The stool was delivered to the lab and immediately frozen at −80 C until processing for DNA extraction. Microbial genomic DNA was extracted using the PowerSoil DNA Isolation Kit (MoBio) (22). The microbial content was profiled by one contiguous region of 16S rRNA V3-V4 sequencing on an Illumina MiSeq that generated ∼100,000 250 bp paired-end reads per sample. Sequencing quality was assessed, errors corrected, Amplicon Sequence Variants (ASVs) were generated, and their taxonomic annotations were obtained against Silva v138 using the DADA2 pipeline (23). ASVs were used to calculate the alpha diversity, which measures the bacterial diversity as a function of richness and evenness within each sample. For all statistical analyses, only the most abundant ASVs in the dataset were utilized, wherein all ASVs with less than 0.01% abundance in all samples, and ASVs observed in less than 10% of the samples were discarded employing the filter_taxa command implemented in OTU table R package (24).
2.3 Behavioral measures
Parents completed the Child Behavior Checklist (CBCL) at home visits. The CBCL is a standardized instrument used to assess behavioral problems in children between 18 and 71 months old (25). It contains 99 items, and each is rated on a three-point Likert scale. This study focused on the six-DSM scales consistent with DSM diagnostic categories: affective, anxiety, pervasive developmental, attention deficit/hyperactivity, and oppositional defiant. The scale is a first level screening, reporting symptoms aligned with diagnostic areas such as autism spectrum disorder (ASD), attention deficit disorder (ADHD), depression, and oppositional defiant disorder (26, 27). Notably, depression symptoms in this age group are manifested mostly as emotional irritability and dysregulation, often differing from manifestations later in development (anhedonia, hopelessness, persistent sadness) (28). The results are age-normed into T-scores with a mean of 50 and standard deviation of 10. Consequently, ranges between 65–69 are considered borderline and scores of 70 or higher are indicative of clinical-range problem (29). A previous publication provides the CBCL descriptive statistics in this sample with means and standard deviations (28).
2.4 Statistical analysis
IBM SPSS Statistics version 25 [IBM, (30)] was used to calculate descriptive and frequency statistics for demographic and clinical data. Scores from the CBCL were analyzed using Spearman correlations because of non-normal bivariate distributions.
The microbiome measures were corrected for potential confounding factors by calculating the residual values for each CBCL score after correcting for gender, delivery method, gestational age, infant birth weight, occurrence of sepsis, and days on antibiotics. For all downstream statistical analyses, the residual values of the CBCL scores were utilized. The microbiome data is already available in NCBI database of Genotypes and Phenotypes (dbGaP) with study accession phs001578.v1.p1 (https://www.ncbi.nlm.nih.gov/projects/gap/cgi-bin/study.cgi?study_id=phs001578.v1.p1). The data generated towards achieving the aims of the study are shared through tables and supplementary data described in this study.
2.5 Canonical correlation analysis
Canonical correlation analyses (CCA) were performed to identify correlations between infant microbiomes and later behavior. This analysis models correlations between two multivariate sets of data (31). For this purpose, the alpha diversity indices at the infant stage were considered to represent the microbiome, while the residual CBCL values represented the behavioral measures. The R package CCA (version 1.2.1) was utilized for this purpose.
2.6 Association of microbiome to later behavior
We examined the associations between early-life microbiome and later behavior (at 4 years) by employing multivariate analysis by linear models (MaAsLin) (32) implemented in the galaxy server (33). The abundant ASVs obtained previously were considered as predictors while the corrected CBCL scores were the outcome. The bacterial counts were converted to relative abundances which were subsequently utilized as input for MaAsLin (34, 35). Apart from ASVs, we further conducted association testing employing MaAsLin for different taxonomic levels including phylum, class, order, family and genus. Associations were considered to be significant if p < 0.05.
3 Results
3.1 Demographics
The 19 children were born early and at very low birth weight (Table 1-sample characteristics and S1-newborn and NICU stay characteristics). They were followed by home visits at 50.3 ± 1.7 months of age. Most were born by Cesarean section (63%), received courses of antibiotics for 15.8 ± 14.8 days, were fed varying amounts of mothers’ own milk and experienced multiple illnesses associated with prematurity. Table 1 provides sample, NICU stay characteristics, and CBCL t-scores at pre-school age.
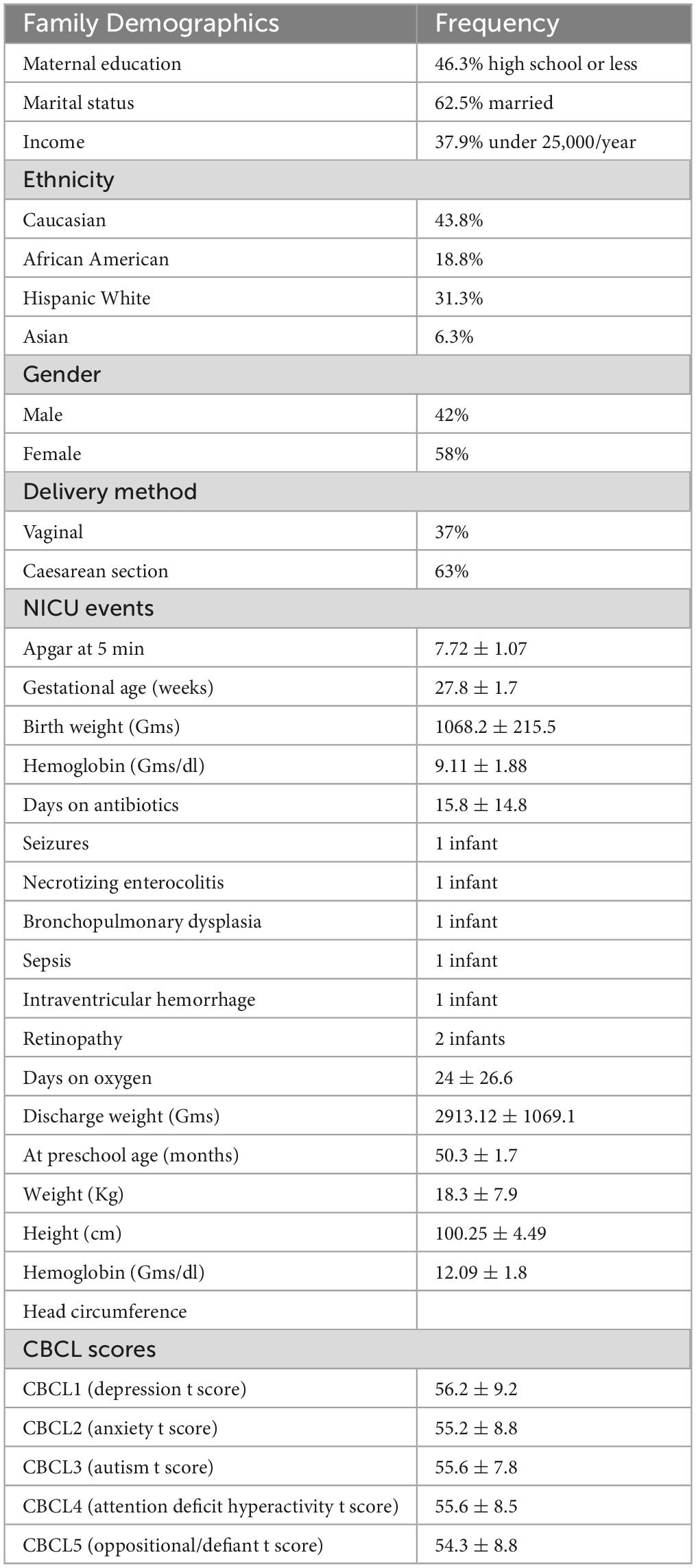
Table 1. Population characteristics, neonatal intensive care unit clinical data and DSM-related childhood behavior checklist t-scores.
3.2 Association between alpha diversity and residual CBCL values
Our previous publications (3–5) have summarized the microbiome features obtained in the cohort. For the samples included in this study, we have obtained 103 unique ASVs whose classifications are listed in Supplementary Table 1. The correlations between the alpha diversity and the adjusted CBCL scores are shown in Figure 1. Strong positive correlations were evident among the different CBCL scores but the associations between the alpha diversity measures and CBCL scores were not statistically significant although weak negative correlations were observed between them. The Spearman rank correlation between the adjusted CBCL scores and the alpha diversity measures are listed in Supplementary Table 1, while the corresponding p-values are listed in Supplementary Table 2.
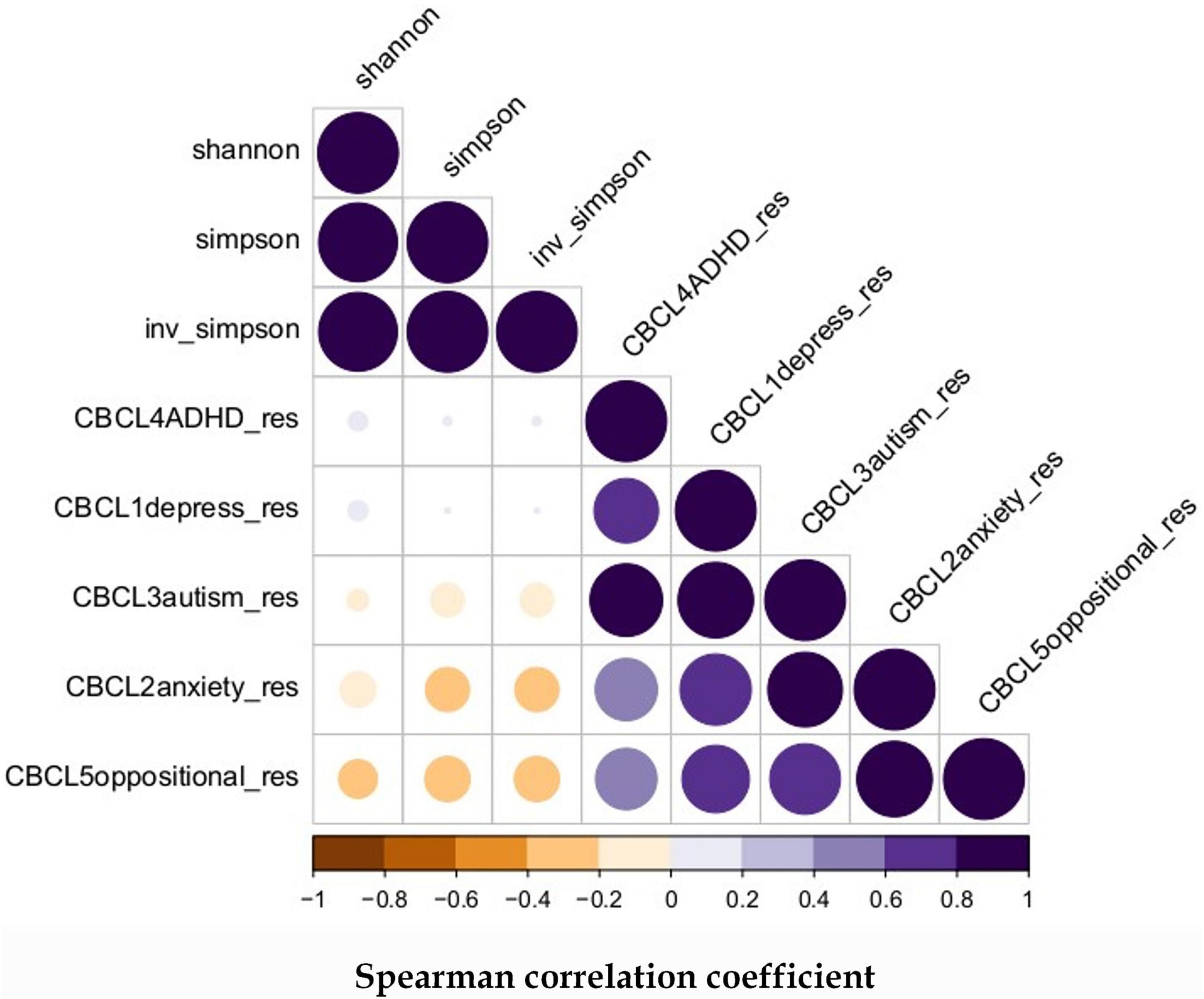
Figure 1. Spearman correlation between microbiome alpha diversity and later childhood behavior: The alpha diversity were derived from ASVs distribution in the samples and all the CBCL scores were adjusted for gender, delivery method, gestational age, infant birth weight, occurrence of sepsis, and days on antibiotics.
3.3 Canonical correlation analysis
The relationship between the gut microbiome parameters in early life was compared with the adjusted CBCL scores at 4 years using canonical correlation analysis (CCA). As shown in Figure 2 the adjusted CBCL measures were highly related to each other but not with the alpha diversity at early life. However, upon investigating the CCA between CBCL scores and prominent ASVs, close relationships were discovered as shown in Figure 3. For example, ASV_1 (family Enterobacteriaceae), ASV_5 (Streptococcus), ASV_7 (Staphylococcus) and ASV_13 (Enterococcus) were all highly associated with CBCL_ADHD adjusted values. Similarly, ASV_10 (Citrobacter) was related to CBCL_oppositional. ASV_4 (Enterobacteriaceae) was associated with both CBCL autism and CBCL ADHD adjusted scores.
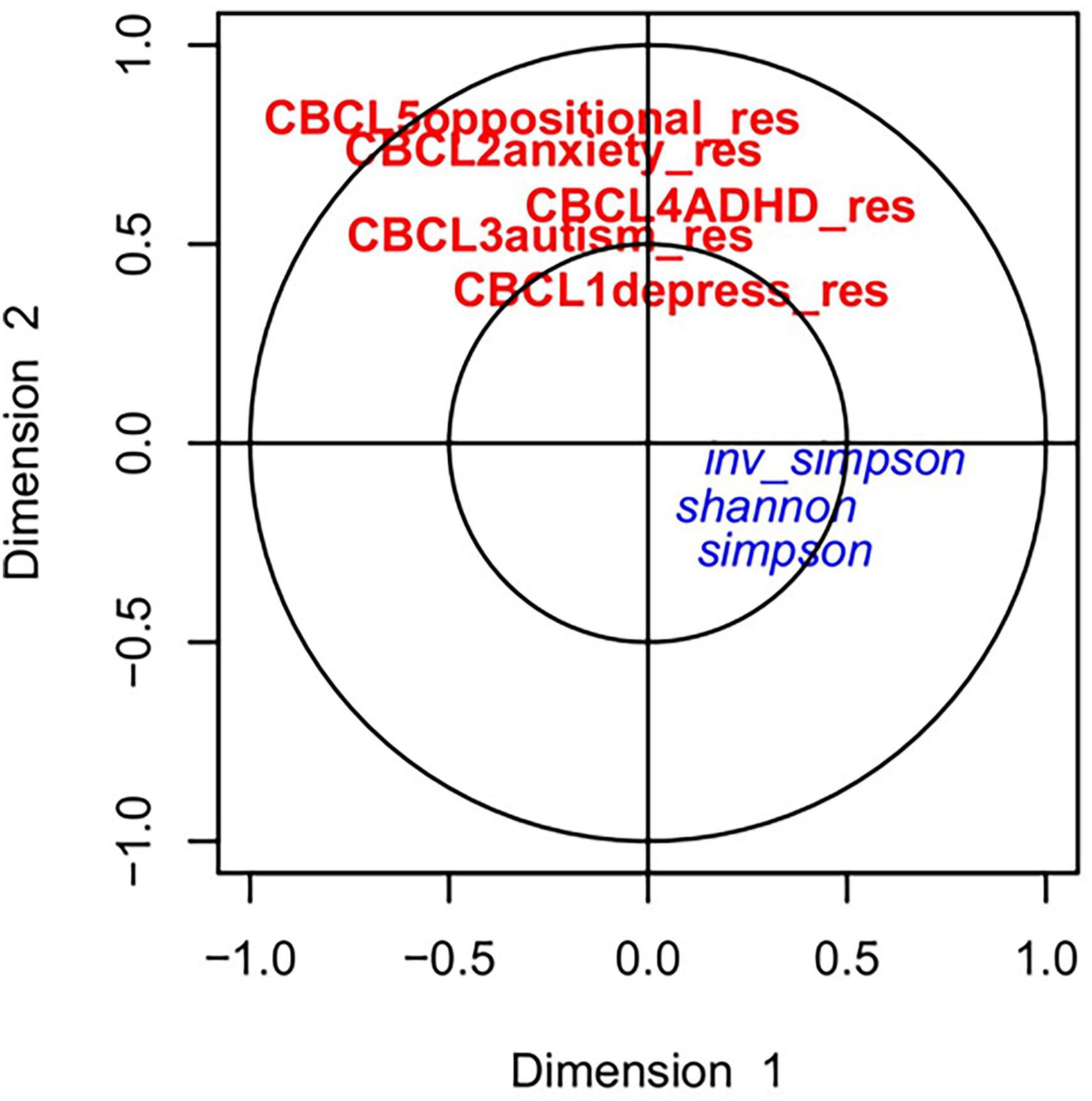
Figure 2. Canonical correlation analyses between alpha diversity at early life and childhood behavior at 4 years: The red and blue color represents the CBCL scores and alpha diversity, respectively. No strong associations between the CBCL scores and alpha diversity were observed.
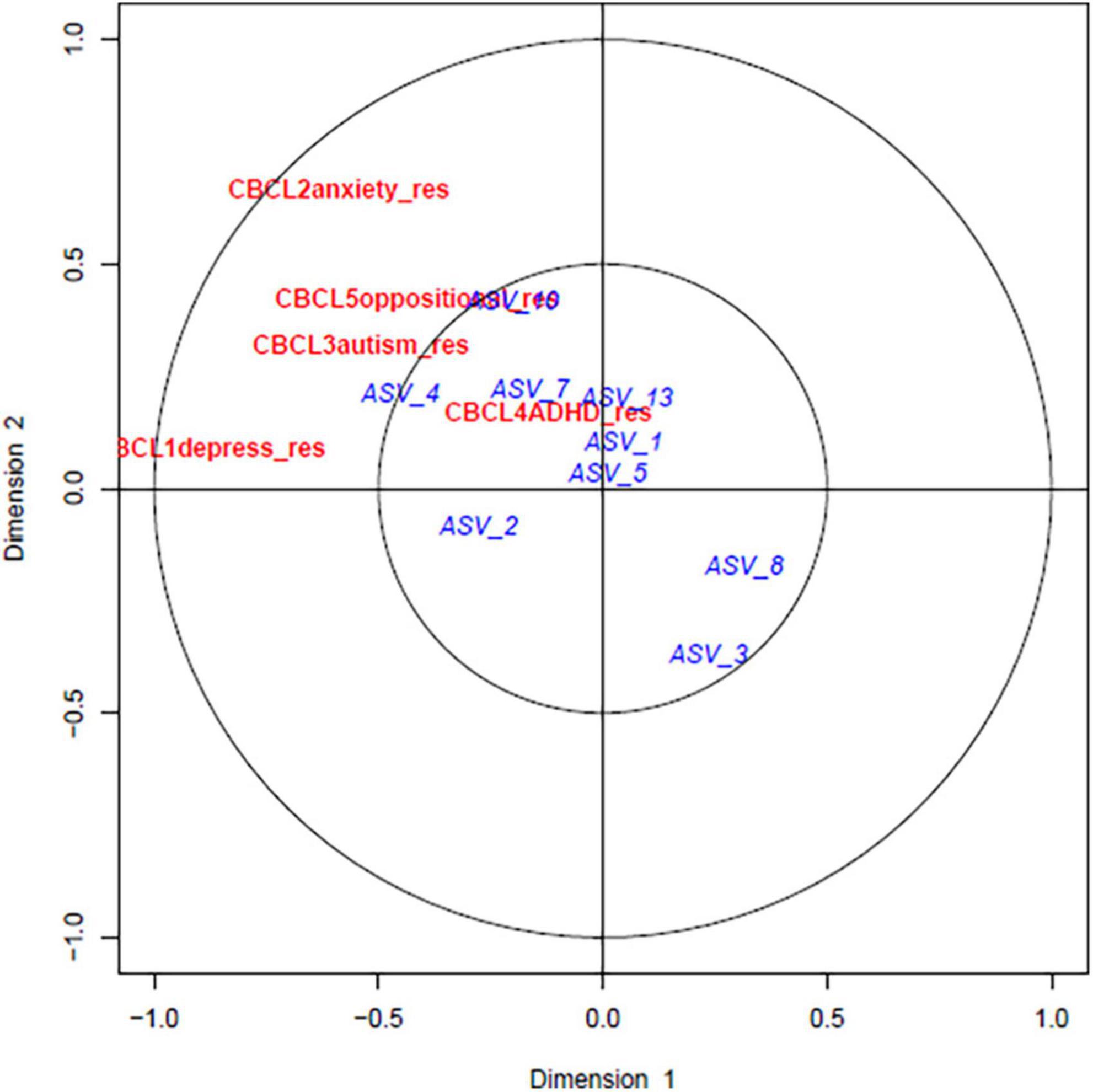
Figure 3. Canonical correlation analyses between predominant bacterial ASVs at early life and childhood behavior at 4 years: The red and blue color represents the CBCL scores and ASVs, respectively. ASV_1 (family Enterobacteriaceae), ASV_5 (Streptococcus), ASV_7 (Staphylococcus) and ASV_13 (Enterococcus) was all strongly associated with CBCL_ADHD adjusted values. Similarly, ASV_10 (Citrobacter) was related to CBCL_oppositional. ASV_4 (Enterobacteriaceae) was associated with both CBCL autism and CBCL ADHD adjusted scores.
3.4 Association of early-stage microbiome to behavior at 4 years
Several significant associations were observed between different ASVs and the CBCL scores. In the domain of depression, there was a positive association with Veillonella dispar (p = 0.0007) and Escherichia coli (p = 0.02), whereas Enterococcus (p = 0.03) and Ruminococcus (p = 0.04) exhibited negative associations. Conversely, in the domain of anxiety, a positive association was observed with Enterococcus (p = 0.04), while Veillonella dispar displayed a negative association (p = 0.01). They are summarized in Table 2. The Supplementary Table 3 lists all the associations exported by MaAsLin with the difference CBCL scores. However, for other taxonomic levels including phylum, class, order, family and genus, we could not observe significant associations and the results are listed in Supplementary Table 4.
4 Discussion
The composition of the gut microbiome showed significant relationships with DSM-based behavioral scales from the CBCL. In this sample, the alpha diversity was not significantly associated with the adjusted CBCL scores. However, specific Amplicon Sequence Variants analyses were significantly associated with the adjusted CBCL scores, some with positive and some with negative associations.
Enterococcus and Veillonella dispar showed significant associations with the CBCL adjusted scales of depression and anxiety. Additionally, there was a significant association of the anxiety CBCL scale with the presence of Escherichia Coli and Ruminococcus.
Aerobic microbes such as Enterococcus and Escherichia are the first to colonize the newborn under normal conditions, with the shift occurring to more anaerobic microbes (including Veillonella) by 4 months of age (36). However, our sample had high abundance of Veillonella within the first weeks in the NICU. Additionally, children born via C-section, which was true for most of the infants, have a higher pathogen abundance of Klebsiella and Enterococcus, which are associated with a later higher incidence of respiratory infections within the first year of life (37).
The gut microbiome’s relevance to mood disorders is supported by the relationship of microbes with mechanisms affecting mood and behavior. Enterococcus faecalis converts naturally occurring levodopa into dopamine via a decarboxylation reaction. Because levodopa is able to cross the blood-brain barrier, but not dopamine, the conversion may lead to reduced central dopamine availability in the brain (38). Veillonella abundance has been associated with negative emotions at school age and to cognitive outcomes from birth to adolescence (39). Veillonella has also been linked to increased stress levels, as indicated by negative events and emotions reported by parents, emotional problems and low happiness reported by children, and a parasympathetic response to stress. These findings were independent of age, gender, parental education, BMI z-score, fiber, protein, sweet and fat food intake, physical activity, and sleep (40). The links between behavior and ASVs exists, but the direction may differ. Further research is necessary to understand better how gut bacteria and moods are connected. Studies that follow individuals over time would be beneficial in exploring these relationships.
A lower abundance of Ruminococcus was related to higher scores in the depression DSM-related scale of the CBCL. Major Depressive Disorder in adults was associated with lower abundance of Ruminococcus (41). Contradictory results have been reported for other taxa, but not for Ruminococcus (41), indicating that this microbe may be a potential biomarker for depressive disorder.
These children, as infants in the NICU, had dysbiotic gut microbiomes with an overabundance of Gammaproteobacteria (10). Dysbiosis could disrupt the normal gut-brain axis in developing infants. Gut microbial products reach the blood and then the brain. These chemicals are capable of modulating neuronal signaling and possibly neurodevelopment. Metabolites of bacterial origin were found in blood samples of children with autism spectrum disorder (ASD), which may cause oxidative stress, mitochondrial dysfunction, and structural changes in the amygdala, cortex, hippocampus, and cerebellum (42).
In conclusion, it is hypothesized that a connection exists between the gut microbiome and behavior. However, most previous studies (43–45) have concentrated on term infants and assessed behavior at 2 years of age or even earlier, which might not entirely align with the conditions at 4 years. Our aim was to investigate gut dysbiosis in VLBW infants and explore the relationships between the early-life gut microbiome and behavior during the preschool years at the age of 4. There were significant relationships between gut microbiome ASVs and DSM-based behavioral scales from the CBCL. Adjusted CBCL scores were significantly associated with ASVs representing Veillonella dispar, Enterococcus, E. coli, and Rumincococcus. It appears that the gut microbiome dysbiosis of VLBWs may have relationships to later childhood behavior. This study contributes to the gut microbiome literature by adding analyses related to different behavioral domains using a standardized tool linked to the DSM.
These results are preliminary due to the limited sample size. Other factors aside from gender, delivery method, gestational age, infant birth weight, occurrence of sepsis, and days on antibiotics are potentially important in these later childhood relationships. These include human milk, growth, parenting, and development characteristics after discharge.
Dysbiosis in the gut microbiome has shown associations with diverse behavioral disorders, encompassing conditions such as anxiety, depression, and even neurodevelopmental disorders like autism. A comprehensive understanding of the mechanisms through which the infant gut microbiome influences these disorders is of paramount importance for timely intervention and proactive prevention strategies.
While this pilot study offers insights into the association between infancy gut dysbiosis and preschool behavioral functions, a limitation of this manuscript is the small sample size utilized in the study. To validate these findings, further investigation with a larger sample size is necessary. Additionally, there may be other potentially significant factors in these later childhood relationships, such as human milk, growth, parenting, and developmental characteristics after discharge, which were not accounted for in this study.
Author’s note
SO also worked on this research while affiliated with the University of South Florida, College of Nursing and the University of Tennessee-Knoxville, College of Nursing. AS, JY, and MG also worked on this research while affiliated with the University of South Florida, College of Nursing.
Data availability statement
The datasets presented in this study can be found in online repositories. The names of the repository/repositories and accession number(s) can be found below: https://www.ncbi.nlm.nih.gov/gap/, phs001578.
Ethics statement
The studies involving humans were approved by the University of South Florida Institutional Review Board. The studies were conducted in accordance with the local legislation and institutional requirements. Written informed consent for participation in this study was provided by the participants’ legal guardians/next of kin.
Author contributions
SO: Conceptualization, Data curation, Formal analysis, Investigation, Methodology, Project administration, Resources, Software, Supervision, Validation, Visualization, Writing-original draft, Writing-review and editing. AS: Conceptualization, Data curation, Formal analysis, Investigation, Methodology, Project administration, Resources, Software, Supervision, Validation, Visualization, Writing-original draft, Writing-review and editing. JY: Investigation, Methodology, Resources, Validation, Writing-review and editing. ES-H: Conceptualization, Methodology, Resources, Validation, Writing-review and editing. MG: Conceptualization, Formal analysis, Funding acquisition, Investigation, Methodology, Project administration, Resources, Supervision, Validation, Visualization, Writing-original draft, Writing-review and editing.
Funding
The author(s) declare financial support was received for the research, authorship, and/or publication of the article. This work was supported by the National Institutes of Health under grants R21 NR013094 and R01NR015446 (MG, P.I.).
Acknowledgments
SO acknowledges support from the Brazilian Federal Agency for Support and Evaluation of Graduate Education (CAPES) for graduate education. Partial Funding for open access to this research was provided by the University of Tennessee’s Open Publishing Support Fund.
Conflict of interest
The authors declare that the research was conducted in the absence of any commercial or financial relationships that could be construed as a potential conflict of interest.
Publisher’s note
All claims expressed in this article are solely those of the authors and do not necessarily represent those of their affiliated organizations, or those of the publisher, the editors and the reviewers. Any product that may be evaluated in this article, or claim that may be made by its manufacturer, is not guaranteed or endorsed by the publisher.
Supplementary material
The Supplementary Material for this article can be found online at: https://www.frontiersin.org/articles/10.3389/fnut.2023.1294549/full#supplementary-material
References
1. Cutland C, Lackritz E, Mallett-Moore T, Bardají A, Chandrasekaran R, Lahariya C, et al. Brighton collaboration low birth weight working group low birth weight: case definition & guidelines for data collection, analysis, and presentation of maternal immunization safety data. Vaccine. (2017) 35(48 Pt. A):6492–500.
2. Sarkar AP, Dutra S, Youn Yoo J, Gordon J, Shaffer E, McSkimming D, et al. Relationships of the very low birth weight infant microbiome with neurodevelopment at 2 and 4 years of age. Dev Psychobiol. (2022) 64:e22317. doi: 10.1002/dev.22317
3. Sanders MR, Hall SL. Trauma-informed care in the newborn intensive care unit: promoting safety, security and connectedness. J Perinatol. (2018) 38:3–10.
4. Shaw RJ, Givrad S, Poe C, Loi EC, Hoge MK, Scala M. Neurodevelopmental, mental health, and parenting issues in preterm infants. Children. (2023) 10:1565.
5. Groer M, Miller EM, Sarkar A, Dishaw LJ, Dutra SV, Youn Yoo J, et al. Predicted metabolic pathway distributions in stool bacteria in very-low-birth-weight infants: potential relationships with NICU faltered growth. Nutrients. (2020) 12:1345.
6. Martin CR, Osadchiy V, Kalani A, Mayer EA. The brain-gut-microbiome axis. Cell Mol Gastroenterol Hepatol. (2018) 6:133–48.
7. Luna R, Savidge T, Williams K. The brain-gut-microbiome axis: What role does it play in autism spectrum disorder? Curr Dev Disord Rep. (2016) 3:75–81.
8. Cryan JF, O’Riordan KJ, Cowan CS, Sandhu KV, Bastiaanssen TF, Boehme M, et al. The microbiota-gut-brain axis. Physiol Rev. (2019) 99:1877–2013.
9. Skarphedinsson G, Jarbin H, Andersson M, Ivarsson T. Diagnostic efficiency and validity of the DSM-oriented child behavior checklist and youth self-report scales in a clinical sample of Swedish youth. PLoS One. (2021) 16:e0254953. doi: 10.1371/journal.pone.0254953
10. Yee A, Miller E, Dishaw L, Gordon J, Ji M, Dutra S, et al. Longitudinal microbiome composition and stability correlate with increased weight and length of very-low-birth-weight infants. mSystems. (2019) 4:e229–218. doi: 10.1128/mSystems.00229-18
11. Aylward GP. Neurodevelopmental outcomes of infants born prematurely. J Dev Behav Pediatr. (2014) 35:394–407.
12. D’Agata AL, Wu J, Welandawe MK, Dutra SV, Kane B, Groer MW. Effects of early life NICU stress on the developing gut microbiome. Dev Psychobiol. (2019) 61:650–60.
13. Diaz Heijtz R. Fetal, neonatal, and infant microbiome: Perturbations and subsequent effects on brain development and behavior. Semin Fetal Neonatal Med. (2016) 21:410–7. doi: 10.1016/j.siny.2016.04.012
14. Goehler L, Park S, Opitz N, Lyte M, Gaykema R. Campylobacter jejuni infection increases anxiety-like behavior in the holeboard: Possible anatomical substrates for viscerosensory modulation of exploratory behavior. Brain Behav Immun. (2008) 22:354–66. doi: 10.1016/j.bbi.2007.08.009
15. Manco M. Gut Microbiota and Developmental Programming of the Brain: From Evidence in Behavioral Endophenotypes to Novel Perspective in Obesity. Front Cell Infect Microbiol. (2012) 2:109. doi: 10.3389/fcimb.2012.00109
16. Sullivan R, Wilson D, Feldon J, Yee B, Meyer U, Richter-Levin G, et al. The international society for developmental psychobiology annual meeting symposium: Impact of early life experiences on brain and behavioral development. Dev Psychobiol. (2006) 48:583–602. doi: 10.1002/dev.20170
17. Volpe J. Encephalopathy of prematurity includes neuronal abnormalities. Pediatrics. (2005) 116:221–5. doi: 10.1542/peds.2005-0191
18. Harbison JE, Roth-Schulze AJ, Giles LC, Tran CD, Ngui KM, Penno MA, et al. Gut microbiome dysbiosis and increased intestinal permeability in children with islet autoimmunity and type 1 diabetes: A prospective cohort study. Pediatr Diab. (2019) 20:574–83.
19. Moran-Ramos S, Lopez-Contreras BE, Villarruel-Vazquez R, Ocampo-Medina E, Macias-Kauffer L, Martinez-Medina JN, et al. Environmental and intrinsic factors shaping gut microbiota composition and diversity and its relation to metabolic health in children and early adolescents: a population-based study. Gut Microbes. (2020) 11:900–17.
20. Prehn-Kristensen A, Zimmermann A, Tittmann L, Lieb W, Schreiber S, Baving L, et al. Reduced microbiome alpha diversity in young patients with ADHD. PLoS One. (2018) 13:e0200728. doi: 10.1371/journal.pone.0200728
21. Sarkar A, Yoo JY, Valeria Ozorio Dutra S, Morgan KH, Groer M. The association between early-life gut microbiota and long-term health and diseases. J Clin Med. (2021) 10:459.
22. QIAGEN. MO BIO’s Powersoil DNA Isolation Kit Handbook: QIAGEN. (2013). Available online at: https://www.qiagen.com/us/resources/resourcedetail?id=5c00f8e4-c9f5-4544-94fa-653a5b2a6373&lang=en (accessed March 2017).
23. Callahan B, McMurdie P, Rosen M, Han A, Johnson A, Holmes S. DADA2: High-resolution sample inference from Illumina amplicon data. Nat Methods. (2016) 13:581–3. doi: 10.1038/nmeth.3869
24. Linz A, Crary B, Shade A, Owens S, Gilbert J, Knight R, et al. Bacterial community composition and dynamics spanning five years in freshwater bog lakes. mSphere. (2017) 2:169–117. doi: 10.1128/mSphere.00169-17
25. Achenbach T. Manual for the Teacher’s Report Form and 1991 profile. Burlington: University of Vermont Department of Psychiatry (1991).
26. Arias A, Rea M, Adler E, Haendel A, Van Hecke A. Utilizing the child behavior checklist (CBCL) as an Autism spectrum disorder preliminary screener and outcome measure for the peers® intervention for autistic adolescents. J Autism Dev Disord. (2021) 52:2061–74. doi: 10.1007/s10803-021-05103-8
27. So P, Greaves-Lord K, van der Ende J, Verhulst F, Rescorla L, de Nijs P. Using the child behavior checklist and the teacher’s report form for identification of children with autism spectrum disorders. Autism. (2013) 17: 595–607.
28. Dutra SVO, Gordon J, Shaffer E, Miller E, Harville C, Yoo JY, et al. An exploratory principal factor analysis of very low birth weight clinical data and development-behavior outcomes at 4 years of age. Pediatr Nurs. (2022) 48:21–33.
30. IBM. IBM Support: Downloading IBM SPSS Statistics 25. (2023). Available online at: https://www.ibm.com/support/pages/downloading-ibm-spss-statistics-25 (accessed July 2023).
31. Thompson B. Canonical Correlation Analysis. Reading and Understanding MORE Multivariate Statistics. Washington, DC: American Psychological Association (2000). p. 285–316.
32. Mallick H, Rahnavard A, McIver L, Ma S, Zhang Y, Nguyen L, et al. Multivariable association discovery in population-scale meta-omics studies. PLoS Comput Biol. (2021) 17:e1009442. doi: 10.1371/journal.pcbi.1009442
33. Huttenhower Lab. Galaxy/Hutlab: Department of Biostatistics. Harvard T.H. chan School of Public Health. Boston, MA: Huttenhower Lab (2023).
34. Collins KH, Schwartz DJ, Lenz KL, Harris CA, Guilak F. Taxonomic changes in the gut microbiota are associated with cartilage damage independent of adiposity, high fat diet, and joint injury. Sci Rep. (2021) 11:14560.
35. Hoskinson C, Zheng K, Gabel J, Kump A, German R, Podicheti R, et al. Composition and functional potential of the human mammary microbiota prior to and following breast tumor diagnosis. Msystems. (2022) 7: e1489–1421.
36. Bäckhed F, Roswall J, Peng Y, Feng Q, Jia H, Kovatcheva-Datchary P, et al. Dynamics and stabilization of the human gut microbiome during the first year of life. Cell Host Microbe. (2015) 17:690–703. doi: 10.1016/j.chom.2015.04.004
37. Reyman M, van Houten M, van Baarle D, Bosch A, Man W, Chu M, et al. Impact of delivery mode-associated gut microbiota dynamics on health in the first year of life. Nat Commun. (2019) 10:4997.
38. Maini Rekdal V, Bess E, Bisanz J, Turnbaugh P, Balskus E. Discovery and inhibition of an interspecies gut bacterial pathway for Levodopa metabolism. Science. (2019) 364:1055. doi: 10.1126/science.aau6323
39. McMath A, Aguilar-Lopez M, Cannavale C, Khan N, Donovan SM. A systematic review on the impact of gastrointestinal microbiota composition and function on cognition in healthy infants and children. Front Neurosci. (2023) 17:1171970. doi: 10.3389/fnins.2023.1171970
40. Michels N, Van de Wiele T, De Henauw S. Chronic psychosocial stress and gut health in children: associations with calprotectin and fecal short-chain fatty acids. Psychosom Med. (2017) 79:927–35. doi: 10.1097/PSY.0000000000000413
41. Maes M, Vasupanrajit A, Jirakran K, Klomkliew P, Chanchaem P, Tunvirachaisakul C, et al. Exploration of the gut microbiome in thai patients with major depressive disorder shows a specific bacterial profile with depletion of the ruminococcus genus as a putative biomarker. Cells. (2023) 12:1240. doi: 10.3390/cells12091240
42. Srikantha P, Mohajeri M. The possible role of the microbiota-gut-brain-axis in autism spectrum disorder. Int J Mol Sci. (2019) 20:2115.
43. Carlson AL, Xia K, Azcarate-Peril MA, Rosin SP, Fine JP, Mu W, et al. Infant gut microbiome composition is associated with non-social fear behavior in a pilot study. Nat Commun. (2021) 12:3294.
44. Loughman A, Ponsonby A-L, O’Hely M, Symeonides C, Collier F, Tang MLK, et al. Gut microbiota composition during infancy and subsequent behavioural outcomes. EBioMedicine. (2020) 52:102640.
Keywords: gut microbiome, gut-brain axis, childhood, behavior, gastrointestinal microbiome, microbiota, CBCL scores
Citation: Ozorio Dutra SV, Sarkar A, Yoo JY, Shaffer-Hudkins E and Groer M (2024) Premature Infant Gut Microbiome relationships with childhood behavioral scales: preliminary insights. Front. Nutr. 10:1294549. doi: 10.3389/fnut.2023.1294549
Received: 14 September 2023; Accepted: 20 November 2023;
Published: 14 February 2024.
Edited by:
Tomás Cerdó, Maimonides Biomedical Research Institute of Cordoba (IMIBIC), SpainCopyright © 2024 Ozorio Dutra, Sarkar, Yoo, Shaffer-Hudkins and Groer. This is an open-access article distributed under the terms of the Creative Commons Attribution License (CC BY). The use, distribution or reproduction in other forums is permitted, provided the original author(s) and the copyright owner(s) are credited and that the original publication in this journal is cited, in accordance with accepted academic practice. No use, distribution or reproduction is permitted which does not comply with these terms.
*Correspondence: Samia Valeria Ozorio Dutra, c2FtaWF2YWxAaGF3YWlpLmVkdQ==; c2FtaWFkdXRyYUBnbWFpbC5jb20=
†These authors have contributed equally to this work and share first authorship