- 1Dorsia Clinics, Madrid, Spain
- 2Catedra UCAM Dorsia, Catholic University San Antonio of Murcia, Guadalupe, Spain
- 3Independent Researcher, Barcelona, Spain
- 4Fagron Genomics US, LLC, Austin, TX, United States
- 5Fagron Genomics, Barcelona, Spain
- 6Fagron (Netherlands), Rotterdam, Netherlands
Purpose: Obesity is a multifactorial condition with a relevant genetic correlation. Recent advances in genomic research have identified several single nucleotide polymorphisms (SNPs) in genes such as FTO, MCM6, HLA, and MC4R, associated with obesity. This study aimed to evaluate the association of 102 SNPs with BMI and weight loss treatment response in a multi-ethnic population.
Methods: The study analyzed 9,372 patients for the correlation between SNPs and BMI (dataset A). The correlation between SNP and weight loss was accessed in 474 patients undergoing different treatments (dataset B). Patients in dataset B were further divided into 3 categories based on the type of intervention: dietary therapy, intragastric balloon procedures, or surgeries. SNP association analysis and multiple models of inheritance were performed.
Results: In dataset A, ten SNPs, including rs9939609 (FTO), rs4988235 (MCM6), and rs2395182 (HLA), were significantly associated with increased BMI. Additionally, other four SNPs, rs7903146 (TCF7L2), (rs6511720), rs5400 (SLC2A2), and rs7498665 (SH2B1), showed sex-specific correlation. For dataset B, SNPs rs2016520 (PPAR-Delta) and rs2419621 (ACSL5) demonstrated significant correlation with weight loss for all treatment types. In patients who adhered to dietary therapy, SNPs rs6544713 (ABCG8) and rs762551 (CYP1A2) were strongly correlated with weight loss. Patients undergoing surgical or endoscopic procedures exhibited differential correlations with several SNPs, including rs1801725 (CASR) and rs12970134 (MC4R), and weight loss.
Conclusion: This study provides valuable insights into the genetic factors influencing BMI and weight loss response to different treatments. The findings highlight the potential for personalized weight management approaches based on individual genetic profiles.
1 Introduction
Obesity is a complex condition resulting from an imbalance between energy intake and expenditure. Affecting over 2 billion people worldwide, it has significant negative impacts on quality of life and health, including an increased risk of numerous comorbidities such as type 2 diabetes, cardiovascular disease, and cancer. It is important to also consider the intricate interplay between the increase in adipose tissue and the modifications in it that contribute to the development of insulin resistance and type 2 diabetes (1, 2). Obesity treatment necessarily involves lifestyle changes; however, surgical and endoscopic procedures may also be necessary. Understanding the multifactorial causes of obesity, including genetic and environmental factors, is crucial for effective treatment and prevention strategies (3). The genomic markers related to the development of obesity are essential in understanding and predicting this condition. Nevertheless, there is also evidence showing that there are loci related to weight loss in response to the treatment of choice (3–6).
Nutrigenomics is an interdisciplinary field that examines the intricate interactions between genetic and dietary factors on health outcomes. By combining genetics, nutrition, and molecular biology, researchers aim to understand how individual genetic variations influence a person’s response to nutrients, food components, and dietary patterns. The goal of nutrigenomics research is to provide personalised dietary recommendations and promote optimal health outcomes. This emerging field has significant potential for improving health and preventing chronic diseases such as type 2 diabetes, obesity, and cardiovascular diseases by tailoring dietary interventions to the specific patient genotype (7–11).
This approach has garnered considerable attention in clinical research due to its potential for personalising nutritional recommendations based on an individual’s genetic profile. For instance, individuals with variations in the FTO gene have been found to be more susceptible to obesity and may benefit from personalised dietary interventions (8, 12, 13). Nutrigenetic testing has also been employed to identify individuals at risk of developing lactose intolerance, coeliac disease, and phenylketonuria, providing them with tailored dietary management. These examples illustrate the potential of nutrigenetics to improve clinical outcomes by enabling more targeted and effective nutritional interventions (7, 11).
A genome-wide association study (GWAS) conducted on over 21,000 subjects from the Taiwan Biobank identified several significant adiposity-associated trait-loci pairs. Among these genes, RALGAPA1 was found to be a specific genetic predisposing factor for high BMI in the Taiwanese population. Additionally, the study detected a moderate genetic correlation between waist-hip ratio (WHR) and BMI, indicating that different genetic determinants exist for abdominal adiposity and overall adiposity. Intriguingly, the study also uncovered the importance of neural pathways that might influence body fat percentage (BF%), waist circumference (WC), and WHR in the Taiwanese population. These findings suggest that multiple genetic factors contribute to obesity and BMI in the Taiwanese population, necessitating further research to better understand the specific pathways involved (14).
In a study by Locke et al., the researchers investigated the heterogeneity of BMI-associated SNPs’ effects, observing stronger effects in women for two SNPs near SEC16B and ZFP64 and significant heterogeneity between European and African-descent samples for two SNPs near NEGR1 and PRKD1, as well as between European and East Asian individuals for an SNP near GBE1. The majority of BMI-associated SNPs demonstrated comparable effects across ancestries and sexes. Utilizing LD differences across populations, the study fine-mapped association signals, revealing that the inclusion of non-European individuals in the meta-analysis refined the genomic region or reduced the credible set’s SNPs for 10 of the 27 BMI loci fine-mapped on Metabochip (5, 6, 15).
Examining the combined effects of lead SNPs at 97 loci in an independent European-descent sample (n = 8,164), the study found an average increase of 0.1 BMI units per BMI-increasing allele and a significant mean BMI difference between individuals with the most and least BMI-increasing alleles. Additionally, incorporating genetic risk scores into a model with age, sex, and four genotype-based principal components marginally yet significantly enhanced obesity prediction accuracy (5, 6, 15).
Subsequent evaluations analyzed the association between BMI and approximately 2.8 million SNPs in up to 123,865 individuals, confirming 14 known obesity susceptibility loci and identifying 18 new loci, including a copy number variant near GPRC5B. Notably, several loci were situated near hypothalamic energy balance regulators (e.g., MC4R, POMC, SH2B1, BDNF) and an incretin receptor (GIPR), potentially providing novel insights into human body weight regulation (16). Overall, these findings suggest that obesity is a complex trait influenced by a combination of genetic and environmental factors. Nevertheless, there is clear genetic contribution to the development of obesity and alterations in body composition. Therefore, finding and employing genomic markers to predict and better understand this pathology might be of great help in dealing with patients presenting this condition.
Genetic variants seem to play a crucial role in the outcomes of bariatric surgeries, such as Roux-en-Y gastric bypass (RYGB). A diverse range of weight loss responses has been observed among patients, and several genes have been associated with these differences. The 15q26.1 locus near ST8SIA2 and SLCO3A1 has been significantly linked to weight loss after RYGB, with the expression of ST8SIA2 in omental fat at baseline correlating with post-surgery weight loss. Additionally, genes such as PKHD1, HTR1A, NMBR, and IGF1R have been identified as potential contributors to the variation in weight loss outcomes after RYGB. However, genetic risk scores (GRSs) for adiposity and the rs1358980-T risk allele in the VEGFA locus did not predict weight loss after gastric bypass surgery in one study. The association between a GRS for abdominal obesity and the response to bariatric surgery may be dependent on the association between the GRS and baseline BMI. Overall, these findings highlight the importance of considering genetic factors when evaluating weight loss outcomes following bariatric surgeries, as they may significantly influence individual responses (17–19).
While there have been previous studies that investigate the impact of genetic factors on the efficacy of surgical and endoscopic interventions for obesity, these studies often involve limited sample sizes or narrow scopes. Consequently, there remains a significant need for comprehensive evaluations that encompass larger, multi-ethnic populations. The identification of specific genetic variations associated with obesity and the predisposition to respond favourably to various therapeutic approaches serves a dual purpose. Firstly, it aids in elucidating the underlying biological mechanisms that contribute to weight loss following therapeutic interventions. Secondly, it provides valuable insights that could facilitate personalized medical decision-making, enabling clinicians to predict patient response to different treatments with greater accuracy. In this context, our study aims to fill the existing gaps in the literature by providing a broader evaluation of the role of genetic variations in influencing the effectiveness of weight loss therapies.
Additionally, it is significant to emphasise that there are numerous factors which ought to be taken into consideration when deciding upon the clinical approach to a patient, whether through a reversible procedure, such as an intragastric balloon, or a non-temporary one, like bariatric surgery or endoscopic interventions. While guidelines exist for the selection of patients for each type of procedure, several of the clinical markers overlap, thus posing a considerable challenge in evaluating the risk versus benefit ratio (20, 21). In the current study, the efficacy of each type of procedure was correlated with the genotypes present in several genomic markers with the aim to enhance the decision-making process for the choice between temporary and non-temporary procedures (20–22).
Therefore, comprehending the genetic factors involved in obesity, high BMI, and the weight loss process is crucial for a more in-depth understanding of these processes and determining optimal management strategies. In this study, we analysed two patient databases, which were genotyped for a panel of 102 SNPs. These SNPs were identified as significant in various energy metabolism pathways, as well as genes implicated in the behavioural aspects of eating.
2 Methods
2.1 Study design
We analysed two distinct databases containing matched genotypes of patients for the 102 SNPs examined, in relation to either their BMI at a single time point or weight loss and BMI changes in patients who underwent weight loss treatment. The complete data comprised two sets of patient information: (A) 9,372 patients whose genotypes were matched to their BMI at the time of DNA collection; (B) 474 patients who were both genotyped and had their weight and BMI assessed before and after follow-up for dietary treatment, endoscopic intragastric balloon procedures, or surgical interventions.
The datasets are composed of patients who had been previously genotyped as part of their nutritional counselling, as per request of the patient for the treatment offered to them, and had their BMI and anthropometric data recorded on their medical record in a fully anonymised database. Only the genotype and BMI data were recovered with no identifiable features associated. Both datasets were fully anonymised before any data processing, ensuring that only the BMI, weight loss, and genotype for each patient were compiled, according to the dataset. These patients had their genotypes and clinical data stored as medical records within a repository, which included individuals from a diverse range of ethnic backgrounds.
2.2 Genotyping procedure
Briefly, all the genotyping assays were performed by qPCR from genomic DNA previously collected from the patients. The DNA was extracted with the automated bead-based method using the KingFisher system (Thermo Fisher). Nucleic acid were quantified with the RNAseP method in order to ensure sample quality control. The SNPs analysed are summarized on Table 1. All SNP genotyping was done with the Taqman probe assay designed on the Genotyping software offered by the Thermo Fisher platform, the raw qPCR amplification data was updated to the cloud service to ensure genotyping to be performed automatically.
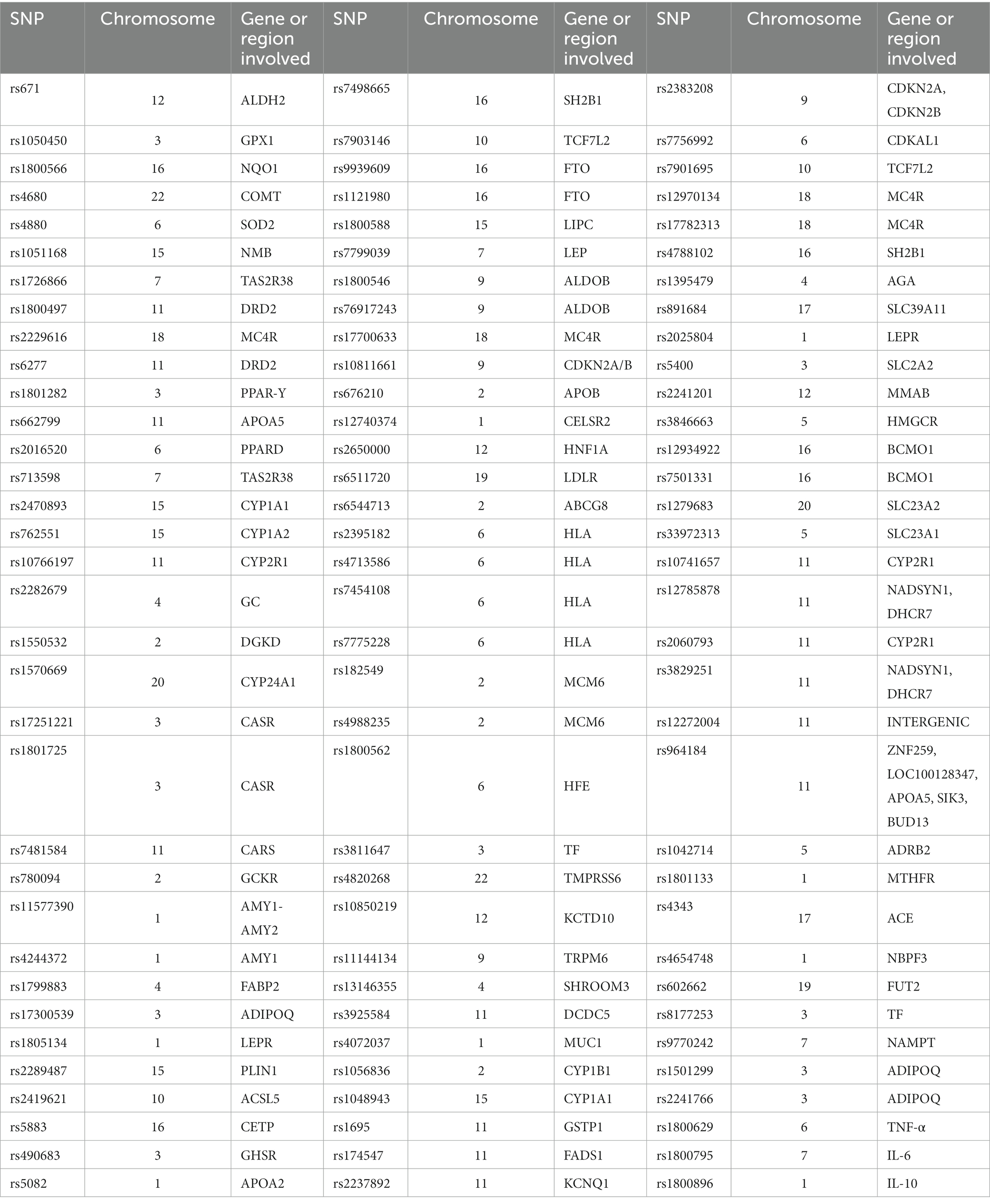
Table 1. Summary of genomic information of the SNPs analysed in patients included in datasets A and B.
2.3 Association analyses and statistical framework
The association between genotype and clinical features was analysed according to the data group. In dataset A, patients’ genotypes were associated with their BMI at the time of DNA collection. The dataset B was composed of patients who were treated with one of the weight loss therapeutic interventions studied. The patients were categorised into groups based on clinical, surgical, or endoscopic interventions for weight loss. Subsequently, the genotypes were associated with weight and BMI loss. Additional data were also evaluated for significance; in dataset B, the follow-up duration until the last weight measurement was assessed, patients were not enrolled to the studied, as it was a retrospective medical record analysis, therefore, there was no information related to the beginning of the intervention. The baseline BMI information was employed to infer weight loss when comparing to the final results. For dataset A, SNP association was tentatively performed for subsets of male and female patients; however, since no relevant data were found, this will not be discussed further.
Statistical analyses were performed using R (version 4.2.2) as the computational environment. The SNPassoc package was chosen for the necessary calculations (23). We evaluated the genotype–phenotype association without restrictions to the dominance model and applied Bonferroni correction. The dominance models applied were: (1) codominance, in which alleles exhert effect; (2) dominance, SNP allele is dominant in determining the phenotype; (3) recessive, SNP allele is recessive the determination of the phenotype; (4) overdominant, heterozygous does not determine a phenotype within the two homozygous possibilities; and (5) additive, neither allele is dominant. The p-values reported are the ones depicting the highest statistical correlation. We aimed to assess the clinical and molecular significance according to the specific study of each SNP. Statistical significance was defined as p < 0.01.
3 Results
3.1 Association between SNPs and BMI
An SNP association analysis was conducted to correlate BMI with genotype for the studied SNPs. Upon analysing the 9,372 patients belonging to dataset A, ten SNPs demonstrated statistically significant correlations with increased BMI. Since the raw genotype data were generated by qPCR for a limited number of SNPs, the genotyping rate for all SNPs was 100%, as only patients with complete genotypes were considered for this study. Table 2 summarises the p-values found for each SNP under the evaluated model of genotype association and Figure 1 displays the Manhattan Plot for visualization of the results.
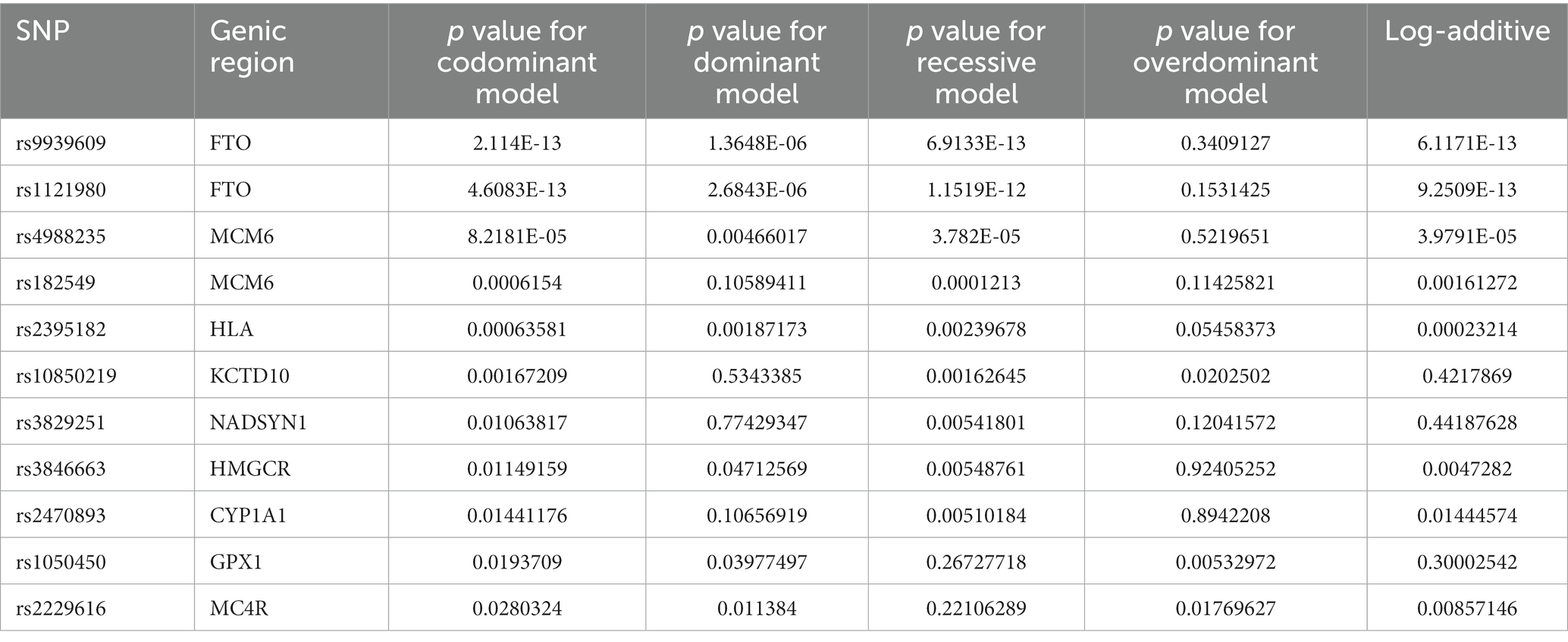
Table 2. p values obtained upon statistic evaluation of the association between BMI (phenotype) to the 102 SNPs analysed.
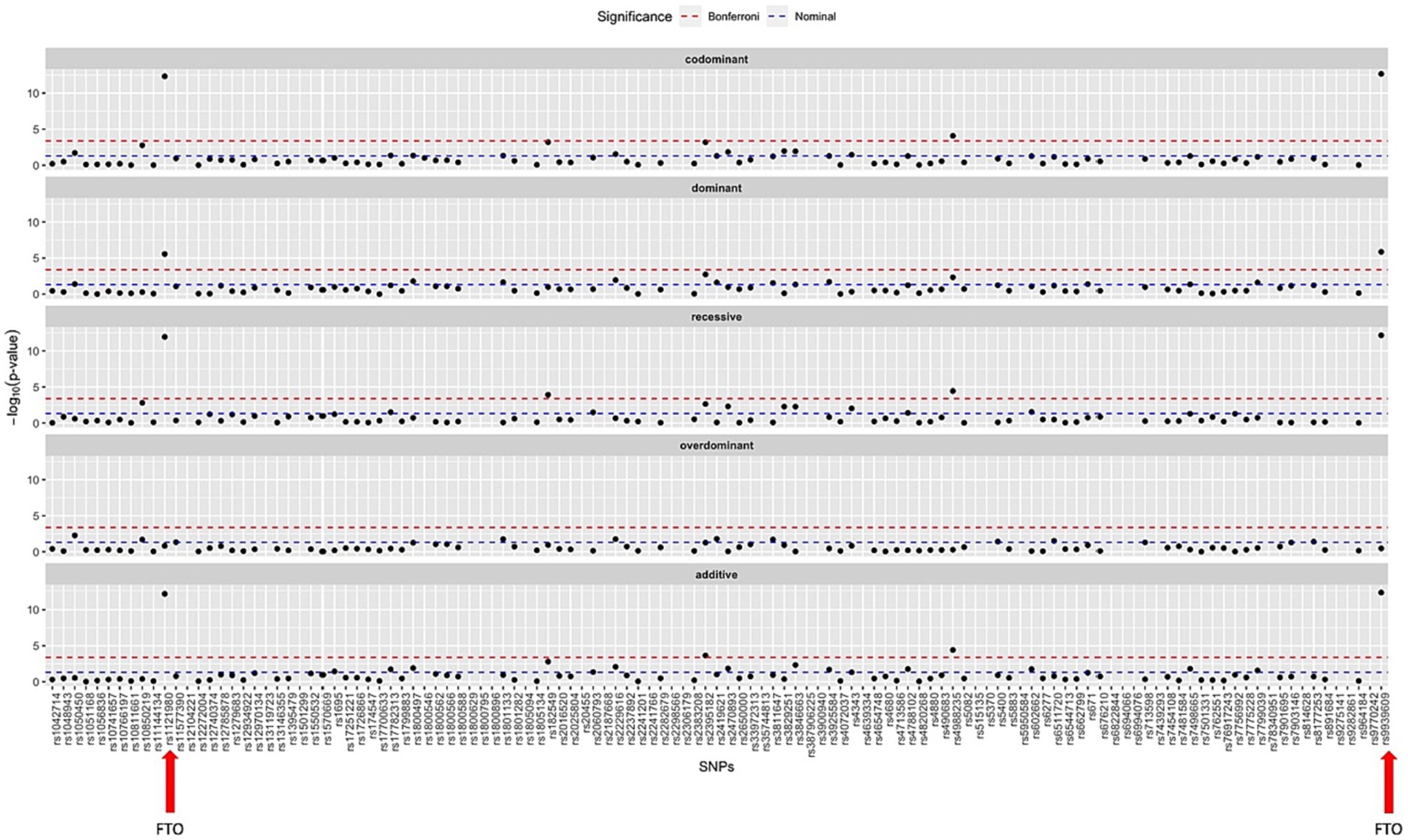
Figure 1. Manhattan plot summarizing the statistic results for all SNPs studied under all models of association to the BMI as phenotype. The SNPs studied in the FTO gene, found to be the most significant ones, are marked with a red arrow.
3.2 Correlation between SNPs and female patients
Interestingly, four additional SNPs were found to have statistically significant associations only among the female population of dataset A. Among female patients, SNPs in the LDL receptor (LDLR), transcription factor TCF7L2, glucose transporter protein SLC2A2, and SH2B1 were positively associated. The following p-values were found for the SNPs under all different models of inheritance: rs7903146 (TCF7L2) p = 0.0027, rs6511720 (LDLR) p = 0.003, rs5400 (SLC2A2) p = 0.003, and rs7498665 (SH2B1) p = 0.008.
3.3 Follow-up length of patients under weight loss therapy
In dataset B, which comprised patients who underwent either dietary therapy or surgical or endoscopic procedures, these patients were monitored to assess weight loss. The overall mean follow-up time for the patients in dataset B was 188.72 days. Among patients exclusively receiving dietary intervention, the mean time was 123.97 days. However, for patients monitored for their diet, 58 were assigned zero days of follow-up in the dataset, indicating that there was no adherence to the treatment at all. Consequently, the rate of adherence to the follow-up program for patients previously tested with this panel of SNPs was 78.27%.
3.4 SNPs on the PPAR-delta and ACSL5 genes predict weight loss in all patients accompanied
When considering all patients in dataset B, who were treated for weight loss, including those who underwent dietary intervention, intragastric balloon procedures, or surgeries, the SNPs rs2016520 and rs2419621 demonstrated significant correlation between genotype and weight loss using the recessive model. Figure 2 summarises the data found for this dataset.
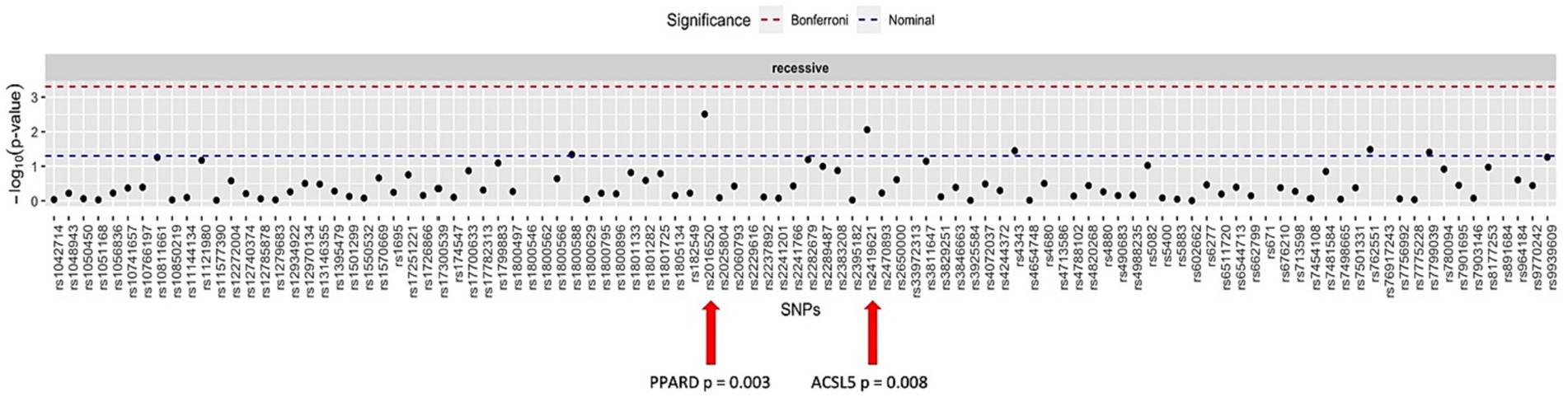
Figure 2. Manhattan plot showing the distribution of p-values for the association between the genotypes and weight loss in all patients.
3.5 Response to diet therapy
Weight loss was documented in patients in dataset B who followed appropriate dietary management and were monitored by nutrition professionals. The difference in BMI and weight was strongly correlated with the genotypes of two SNPs: rs6544713 (p = 0.00001) and rs762551 (p = 0.00033). It is also worth noting that the SNP studied in the COMT enzyme, rs4680, exhibited some degree of correlation when using the recessive model of association, with a p-value of 0.02.
3.6 SNP-association to the weight loss in patients subject to surgical or endoscopic procedures
Weight loss in patients undergoing surgical or endoscopic procedures was greater, as expected and supported by the literature (24, 25). Moreover, several SNPs could also be differentially correlated with these procedures.
Patients who underwent intragastric balloon procedures demonstrated a positive correlation between weight loss and the genotype of the following SNPs: rs1801725 (CASR gene) p = 0.00131, rs4820268 (TMPRSS6) p = 0.002, rs17300539 (ADIPOQ) p = 0.003, and rs17251221 (CASR) p = 0.007.
The difference in weight before and after endoscopic sleeve or POSE procedures exhibited a high degree of statistical significance with 5 SNPs. The genotypes of the following SNPs were correlated using a recessive model to the amount of weight lost: rs12970134 (MC4R) p = 0.00003, rs17782313 (MC4R) p = 0.00003, rs4072037 (MUC1) p = 0.00052, rs1550532 (ACE) p = 0.001, and rs1550532 (DGKD) p = 0.00078. It is also worth mentioning that rs4343, in the leptin gene, showed a p-value of 0.02. Furthermore, the PPAR-delta SNP rs2016520 has been associated with weight loss in patients who underwent all types of procedures, including balloon, endoscopic sleeve, i.e., and POSE.
4 Discussion
In this study, we analysed two groups of patients, separate into: dataset A (9,372 patients), and dataset B (474 patients). We aimed to investigate the association between selected SNPs and BMI, as well as their potential role in predicting weight loss response to various treatments in a diverse population. Our findings revealed that several of the studied SNPs were significantly correlated with BMI. We also identified a subset of SNPs that were uniquely associated with BMI in female patients. Moreover, we observed that specific SNPs were significantly linked to weight loss outcomes in patients undergoing different weight loss treatments. These results highlight the complex interplay between genetic factors and obesity, as well as the response to weight loss interventions.
Furthermore, the difference in potential genomic markers that predict increased BMI and weight loss in different procedures highlights the importance of nutrigenetics in the management of patients to control obesity and its comorbidities (7). By understanding the genetic predispositions and individual responses to weight loss interventions, we can develop personalized treatment approaches that optimize weight loss outcomes and overall health. Table 3 summarises the findings.
Moreover, the strong interaction between the two SNPs on the FTO gene, rs9939609 and rs1121980, and increased BMI as a phenotype reinforces the importance of the FTO gene in the development of obesity, as previously reported in the literature (26–28). FTO has been associated with leptin concentration, as well as alterations in HDL and triglyceride levels (29, 30). These SNPs have also been linked to dietary intake, leptin levels, and body mass distribution (31, 32). However, our study did not find any association between these SNPs and weight loss in any of the intervention groups. This finding suggests that while the FTO gene may play a significant role in the development of obesity, its involvement in weight loss response might be limited or influenced by other factors. Our results warrant further investigation to better understand the underlying mechanisms.
The process of losing weight involves creating a caloric deficit to restore the energy balance. However, biochemical and psychological factors can determine the success of sustaining the caloric deficit to achieve significant weight loss. This has led to the search for genomic markers to predict the ideal type of diet based on individual factors. Personalising the diet through nutrigenomics could be a successful approach to improving weight loss outcomes (4).
The relevance of genetics in predicting diets and weight loss has become increasingly apparent, as recent advances in genomics have enabled researchers to uncover key genes that influence individual responses to specific dietary interventions. Understanding these genetic factors can help tailor personalised nutrition plans, ultimately leading to more effective weight loss and better overall health outcomes. Among the most significant genes related to diet and weight loss are FTO, MC4R, and PPARγ, which have been implicated in the regulation of appetite (4, 33), energy expenditure, and fat metabolism. Studies employing Mendelian randomisation have further strengthened the causal links between these genes, dietary patterns, and obesity risk. As our understanding of the complex interplay between genetics and diet continues to grow, nutrigenomic approaches will likely play an increasingly important role in developing effective, personalised weight loss strategies (4, 34).
Apart from the dietary and life-style approach, surgical and endoscopic methods do also play an important role in aiding patients achieve significative weight loss. Nevertheless, the efficacy of those methods is also variable, which has to be balanced with the risks involved in the procedures (24, 25). Therefore, the search for genomic markers of success related to surgical, endoscopic, and dietary interventions seem an interesting possibility to guide the decisions.
In this study, SNPs rs1050450 (GPX1) and rs2229616 (MC4R) have been associated with increased BMI and body weight. Both genes have previously been linked to obesity (35), and it is noteworthy that MC4R is involved in appetite regulation (36–38). Although the GPX1 gene does not show any apparent functional connection to weight gain, our results support previous findings. The presence of variations in the melanocortin receptor could serve as a crucial marker for determining patient management, as it indicates both the risk of obesity and a predisposition to poor appetite control. Consequently, appropriate nutritional intervention may be guided by the genotype.
In the female population, the SNP rs5400 (SLC2A2) was associated with increased BMI. In addition to corroborating previous studies (39), this finding offers valuable insights for designing nutrigenomic diets for both weight loss and type 2 diabetes management. This SNP indicates that individuals may have altered sensitivity to sugar, leading to higher sugar intake (40, 41). The rs7498665 in the SH2B1 gene has also been found to be associated with BMI in our female subset (31, 42–44).
Apart from variations previously associated with BMI, we also found a positive correlation between BMI and SNPs previously linked to alterations in lipid and lipoprotein levels: rs10850219 (KCTD10) (45, 46); rs3846663 (HMGCR) (33, 47, 48); and rs6511720 (LDLR) (49–51).
The SNP rs2470893, which influences the function of the CYP1A1 enzyme, has also been found to be associated with an increase in BMI. Although there appears to be no direct mechanistic link, a previous study has shown this polymorphism to be associated with both alterations in coffee consumption and BMI. Researchers speculated that this connection might be due to differences in appetite control, including variations in circulating levels of asprosin (52).
Another intriguing correlation of interest was discovered in the MCM6 gene. The two SNPs studied in this gene, rs4988235 and rs182549, were strongly linked with alterations in body mass, in our study. Variants in the lactase gene are widely known to cause lactose intolerance, which, per se, has no direct correlation to weight gain (53). A few studies have shown the rs4988235 SNP to be linked to changes in body composition and lipid concentrations (35, 54). The rs182549 has also been correlated with changes in food consumption patterns (55) These previous findings, combined with the strong connection to BMI increase observed in our data, suggest that this is a relevant target for nutrigenetic management. Variations in the MCM6 also drive changes in the microbiota, which can potentially be therapeutically manipulated (56).
With regards to the genotype–phenotype correlations discovered in dataset B of this study, we identified several genomic markers capable of determining predispositions for success under specific therapeutic approaches to weight loss. Variations in the ABCG8 and COMT genes (SNPid rs6544713), previously linked to cholesterol levels (57), and in the COMT gene, widely recognised for its relevance in the behavioural aspects of eating (58, 59), have been associated with weight loss in patients exclusively undergoing dietary therapy. By understanding the implications of alterations in eating patterns, dietitians may be able to further tailor diets for patients presenting alterations in the COMT gene, who are predisposed to behavioural changes.
Among the SNPs associated with success in intragastric balloon procedures, the genotypes of rs17300539 in the adiponectin gene were positively correlated. Variations in the adiponectin gene have been previously linked to obesity, likely due to altered expression of this marker (60, 61). In our study, we identified the SNP rs17300539 as a novel genomic marker correlated with weight loss. In patients undergoing sleeve or POSE surgery, SNPs in the melanocortin receptor were strongly associated with the capacity to lose weight. This finding offers both an interesting target for nutrigenetic management and a mechanistic understanding of weight gain and loss in light of the central regulation of appetite. In addition, the rs4343 in the leptin gene, previously associated with weight gain and satiety (62, 63), was a novel finding related to weight loss in patients undergoing these procedures.
The discovery of markers linked to the success of treating patients via either a temporary procedure, i.e., the intragastric balloon, or a definitive surgical or endoscopic intervention, contributes significantly to the existing body of literature. It offers the potential to more effectively guide physicians in determining the optimal therapeutic approach. From a biological perspective, it is noteworthy that polymorphisms in the adiponectin gene were correlated with success in patients treated with the intragastric balloon. This association could potentially be attributed to the role of adiponectin in mediating signals from adipose tissue, which is involved in insulin resistance, glucose level regulation, and ultimately, energy metabolism (64–66). Previous studies have linked other adiponectin gene polymorphisms to the response to aerobic exercise (67).
Simultaneously, polymorphisms in the melanocortin-4 receptor and leptin genes were linked to successful outcomes in non-temporary procedures, namely surgical or endoscopic interventions. This suggests an inherent correlation between the response to these procedures and the central mechanisms of appetite control. In light of these novel findings, the utilisation of genomic markers could supplement the clinical data used in the decision-making process to determine the most appropriate type of procedure (3, 68).
In addition to the findings related to genetic analysis, we also observed interesting results regarding the overall utility of this approach. In dataset B, 78.27% of patients demonstrated a global adherence to dietarian changes, which is considerably higher compared to studies with non-genotyped patients (1, 2, 69, 70). This suggests that nutrigenetic tools might also impact the efficacy of diet due to increased adherence.
5 Conclusion
This study sheds light on the complex interplay between genetic factors and obesity, as well as the response to weight loss interventions. The identification of significant correlations between specific SNPs and BMI, along with SNPs uniquely associated with BMI in female patients, underscores the importance of considering personalized treatment approaches based on individual genetic profiles. The findings also reveal the potential of nutrigenetics in guiding patient management by targeting key genes, such as FTO, GPX1, MC4R, SLC2A2, and SH2B1. These results contribute to our understanding of the genetic predispositions and individual responses to weight loss interventions, enabling the development of tailored treatment strategies to optimize weight loss outcomes and overall health.
Moreover, the study highlights the potential impact of nutrigenetic tools on the efficacy of diet due to increased adherence. The higher global adherence to diet observed among genotyped patients suggests that incorporating genetic information into weight loss interventions may lead to improved patient engagement and success. As obesity and its comorbidities continue to pose significant public health challenges, the insights gained from this research pave the way for more targeted and effective approaches to weight management, ultimately leading to better health outcomes for patients.
Data availability statement
The original contributions presented in the study are included in the article, further inquiries can be directed to the corresponding author.
Ethics statement
Ethical approval was not required for the studies involving humans because the whole study was conducted solely with data obtained from a database of medical records and genetic data from patients previously collected. All the data is anonymised completely so as to comply with not needing ethical approval. The studies were conducted in accordance with the local legislation and institutional requirements. The human samples used in this study were acquired from a by-product of routine care or industry. Written informed consent to participate in this study was not required from the participants or the participants’ legal guardians/next of kin in accordance with the national legislation and the institutional requirements.
Author contributions
AM-C: Writing – original draft, Writing – review & editing. FN-L: Conceptualization, Data curation, Writing – original draft. MF: Conceptualization, Writing – review & editing. PA: Conceptualization, Formal analysis, Supervision, Writing – original draft. LV-V: Conceptualization, Investigation, Writing – original draft, Writing – review & editing. VR: Formal analysis, Investigation, Methodology, Writing – original draft. CS: Investigation, Validation, Writing – review & editing. GS: Data curation, Formal analysis, Investigation, Methodology, Project administration, Writing – original draft, Writing – review & editing.
Funding
The author(s) declare that no financial support was received for the research, authorship, and/or publication of this article.
Conflict of interest
AM-C and FN-L are affiliated with Dorsia Clinics, Medical Department, Madrid, Spain, with FN-L also affiliated with Universidad Católica San Antonio de Murcia (UCAM), Murcia, Spain. MF is employed by Fagron Ibérica, Barcelona, Spain. PA is employed by Gx Sciences, Austin, Texas, United States. LV-V, VR, and GS are employed by Fagron Genomics, Barcelona, Spain. CS is employed by Fagron BV, Rotterdam, Netherlands.
Despite these affiliations and employments with commercial entities, the authors declare that the research was conducted in the absence of any commercial or financial relationships that could be construed as a potential conflict of interest. We would like to reiterate that although the authors are associated or employed by industry or commercial entities, we declare no conflict of interest as the data generated is not intended for commercial use. Rather, the aim is to utilise data acquired to corroborate and contribute to the existing basic literature, thereby enhancing the quality of health care and contributing to scientific knowledge in the field.
Publisher’s note
All claims expressed in this article are solely those of the authors and do not necessarily represent those of their affiliated organizations, or those of the publisher, the editors and the reviewers. Any product that may be evaluated in this article, or claim that may be made by its manufacturer, is not guaranteed or endorsed by the publisher.
References
1. Selvam, J, Thangaraj, P, Hemalatha, K, and Subbian, P. Diet adherence and factors associated with nonadherence among type 2 diabetics at an urban health center in Tamil Nadu, India. Int J Acad Med. (2023) 9:25. doi: 10.4103/ijam.ijam_10_22
2. Ayele, AA, Emiru, YK, Tiruneh, SA, Ayele, BA, Gebremariam, AD, and Tegegn, HG. Level of adherence to dietary recommendations and barriers among type 2 diabetic patients: a cross-sectional study in an Ethiopian hospital. Clin Diabetes Endocrinol. (2018) 4:21. doi: 10.1186/s40842-018-0070-7
3. González-Muniesa, P, Mártinez-González, M-A, Hu, FB, Després, J-P, Matsuzawa, Y, Loos, RJF, et al. Obesity. Nat Rev Dis Primers. (2017) 3:17034. doi: 10.1038/nrdp.2017.34
4. Chen, M, and Chen, W. Single-nucleotide polymorphisms in medical nutritional weight loss: challenges and future directions. J Transl Int Med. (2022) 10:1–4. doi: 10.2478/jtim-2022-0002
5. Zaitlen, N, Kraft, P, Patterson, N, Pasaniuc, B, Bhatia, G, Pollack, S, et al. Using extended genealogy to estimate components of heritability for 23 quantitative and dichotomous traits. PLoS Genet. (2013) 9:e1003520. doi: 10.1371/journal.pgen.1003520
6. Fall, T, and Ingelsson, E. Genome-wide association studies of obesity and metabolic syndrome. Mol Cell Endocrinol. (2014) 382:740–57. doi: 10.1016/j.mce.2012.08.018
7. Roosan, D, Wu, Y, Tran, M, Huang, Y, Baskys, A, and Roosan, MR. Opportunities to integrate nutrigenomics into clinical practice and patient counseling. Eur J Clin Nutr. (2023) 77:36–44. doi: 10.1038/s41430-022-01146-x
8. Ghosh, D, Skinner, MA, and Laing, WA. Pharmacogenomics and nutrigenomics: synergies and differences. Eur J Clin Nutr. (2007) 61:567–74. doi: 10.1038/sj.ejcn.1602590
9. Robinson, K, Rozga, M, Braakhuis, A, Ellis, A, Monnard, CR, Sinley, R, et al. Effect of incorporating genetic testing results into nutrition counseling and care on dietary intake: An evidence analysis center systematic review—part I. J Acad Nutr Diet. (2021) 121:553–581.e3. doi: 10.1016/j.jand.2020.04.001
10. Trujillo, E, Davis, C, and Milner, J. Nutrigenomics, proteomics, metabolomics, and the practice of dietetics. J Am Diet Assoc. (2006) 106:403–13. doi: 10.1016/j.jada.2005.12.002
11. Barrea, L, Annunziata, G, Bordoni, L, Muscogiuri, G, Colao, A, and Savastano, S. Nutrigenetics—personalized nutrition in obesity and cardiovascular diseases. Int J Obes Suppl. (2020) 10:1–13. doi: 10.1038/s41367-020-0014-4
12. Merritt, DC, Jamnik, J, and El-Sohemy, A. FTO genotype, dietary protein intake, and body weight in a multiethnic population of young adults: a cross-sectional study. Genes Nutr. (2018) 13:4. doi: 10.1186/s12263-018-0593-7
13. Santos, KD, Rosado, EL, da Fonseca, ACP, Belfort, GP, da Silva, LBG, Ribeiro-Alves, M, et al. FTO and ADRB2 genetic polymorphisms are risk factors for earlier excessive gestational weight gain in pregnant women with Pregestational diabetes mellitus: results of a randomized Nutrigenetic trial. Nutrients. (2022) 14:1050. doi: 10.3390/nu14051050
14. Wong, HS-C, Tsai, S-Y, Chu, H-W, Lin, M-R, Lin, G-H, Tai, Y-T, et al. Genome-wide association study identifies genetic risk loci for adiposity in a Taiwanese population. PLoS Genet. (2022) 18:e1009952. doi: 10.1371/journal.pgen.1009952
15. Locke, AE, Kahali, B, Berndt, SI, Justice, AE, Pers, TH, Day, FR, et al. Genetic studies of body mass index yield new insights for obesity biology. Nature. (2015) 518:197–206. doi: 10.1038/nature14177
16. Speliotes, EK, Willer, CJ, Berndt, SI, Monda, KL, Thorleifsson, G, Jackson, AU, et al. Association analyses of 249,796 individuals reveal 18 new loci associated with body mass index. Nat Genet. (2010) 42:937–48. doi: 10.1038/ng.686
17. Rinella, ES, Still, C, Shao, Y, Wood, GC, Chu, X, Salerno, B, et al. Genome-wide Association of Single-Nucleotide Polymorphisms with Weight Loss Outcomes after Roux-en-Y Gastric Bypass Surgery. J Clin Endocrinol Metab. (2013) 98:E1131–6. doi: 10.1210/jc.2012-3421
18. Hatoum, IJ, Greenawalt, DM, Cotsapas, C, Daly, MJ, Reitman, ML, and Kaplan, LM. Weight loss after gastric bypass is associated with a variant at 15q26.1. Am J Hum Genet. (2013) 92:827–34. doi: 10.1016/j.ajhg.2013.04.009
19. Aasbrenn, M, Svendstrup, M, Schnurr, TM, Lindqvist Hansen, D, Worm, D, Balslev-Harder, M, et al. Genetic markers of abdominal obesity and weight loss after gastric bypass surgery. PLoS One. (2021) 16:e0252525. doi: 10.1371/journal.pone.0252525
20. Bawahab, MA, Abbas, KS, El, MWMA, Abdelgadir, RS, Altumairi, K, Alqahtani, AR, et al. Factors affecting weight reduction after Intragastric balloon insertion: a retrospective study. Healthcare. (2023) 11:600. doi: 10.3390/healthcare11040600
21. Eisenberg, D, Shikora, SA, Aarts, E, Aminian, A, Angrisani, L, Cohen, RV, et al. 2022 American Society for Metabolic and Bariatric Surgery (ASMBS) and International Federation for the Surgery of obesity and metabolic disorders (IFSO): indications for metabolic and bariatric surgery. Surg Obes Relat Dis. (2022) 18:1345–56. doi: 10.1016/j.soard.2022.08.013
22. Stavrou, G, Shrewsbury, A, and Kotzampassi, K. Six intragastric balloons: which to choose? World J Gastrointest Endosc. (2021) 13:238–59. doi: 10.4253/wjge.v13.i8.238
23. González, JR, Armengol, L, Solé, X, Guinó, E, Mercader, JM, Estivill, X, et al. SNPassoc: an R package to perform whole genome association studies. Bioinform. (2007) 23:654–55. doi: 10.1093/bioinformatics/btm025
24. Kang, JH, and Le, QA. Effectiveness of bariatric surgical procedures. Medicine. (2017) 96:e8632. doi: 10.1097/MD.0000000000008632
25. Vantanasiri, K, Matar, R, Beran, A, and Jaruvongvanich, V. The efficacy and safety of a Procedureless gastric balloon for weight loss: a systematic review and Meta-analysis. Obes Surg. (2020) 30:3341–6. doi: 10.1007/s11695-020-04522-3
26. Hinney, A, Nguyen, TT, Scherag, A, Friedel, S, Brönner, G, Müller, TD, et al. Genome wide association (GWA) study for early onset extreme obesity supports the role of fat mass and obesity associated gene (FTO) variants. PLoS One. (2007) 2:e1361. doi: 10.1371/journal.pone.0001361
27. Burton, PR, Clayton, DG, Cardon, LR, Craddock, N, Deloukas, P, Duncanson, A, et al. Genome-wide association study of 14,000 cases of seven common diseases and 3,000 shared controls. Nature. (2007) 447:661–78. doi: 10.1038/nature05911
28. Frayling, TM, Timpson, NJ, Weedon, MN, Zeggini, E, Freathy, RM, Lindgren, CM, et al. A common variant in the FTO gene is associated with body mass index and predisposes to childhood and adult obesity. Science. (2007) 316:889–94. doi: 10.1126/science.1141634
29. Willer, CJ, Schmidt, EM, Sengupta, S, Peloso, GM, Gustafsson, S, Kanoni, S, et al. Discovery and refinement of loci associated with lipid levels. Nat Genet. (2013) 45:1274–83. doi: 10.1038/ng.2797
30. Yaghootkar, H, Zhang, Y, Spracklen, CN, Karaderi, T, Huang, LO, Bradfield, J, et al. Genetic studies of leptin concentrations implicate leptin in the regulation of early adiposity. Diabetes. (2020) 69:2806–18. doi: 10.2337/db20-0070
31. Pulit, SL, Stoneman, C, Morris, AP, Wood, AR, Glastonbury, CA, Tyrrell, J, et al. Meta-analysis of genome-wide association studies for body fat distribution in 694 649 individuals of European ancestry. Hum Mol Genet. (2019) 28:166–74. doi: 10.1093/hmg/ddy327
32. Goh, Y, and Choi, J-H. Genetic variation rs1121980 in the fat mass and obesity-associated gene (FTO) is associated with dietary intake in Koreans. Food. Nutr Res. (2022):66. doi: 10.29219/fnr.v66.8059
33. Wojcik, GL, Graff, M, Nishimura, KK, Tao, R, Haessler, J, Gignoux, CR, et al. Genetic analyses of diverse populations improves discovery for complex traits. Nature. (2019) 570:514–8. doi: 10.1038/s41586-019-1310-4
34. Pirastu, N, McDonnell, C, Grzeszkowiak, EJ, Mounier, N, Imamura, F, Merino, J, et al. Using genetic variation to disentangle the complex relationship between food intake and health outcomes. PLoS Genet. (2022) 18:e1010162. doi: 10.1371/journal.pgen.1010162
35. Huang, LO, Rauch, A, Mazzaferro, E, Preuss, M, Carobbio, S, Bayrak, CS, et al. Genome-wide discovery of genetic loci that uncouple excess adiposity from its comorbidities. Nat Metab. (2021) 3:228–43. doi: 10.1038/s42255-021-00346-2
36. Geller, F, Reichwald, K, Dempfle, A, Illig, T, Vollmert, C, Herpertz, S, et al. Melanocortin-4 receptor gene variant I103 is negatively associated with obesity. Am J Hum Genet. (2004) 74:572–81. doi: 10.1086/382490
37. Ahituv, N, Kavaslar, N, Schackwitz, W, Ustaszewska, A, Martin, J, Hébert, S, et al. Medical sequencing at the extremes of human body mass. Am J Hum Genet. (2007) 80:779–91. doi: 10.1086/513471
38. Heid, IM, Vollmert, C, Kronenberg, F, Huth, C, Ankerst, DP, Luchner, A, et al. Association of the MC4R V103I polymorphism with the metabolic syndrome: the KORA study. Obesity. (2008) 16:369–76. doi: 10.1038/oby.2007.21
39. Hwang, L-D, Lin, C, Gharahkhani, P, Cuellar-Partida, G, Ong, J-S, An, J, et al. New insight into human sweet taste: a genome-wide association study of the perception and intake of sweet substances. Am J Clin Nutr. (2019) 109:1724–37. doi: 10.1093/ajcn/nqz043
40. Gaulton, KJ, Willer, CJ, Li, Y, Scott, LJ, Conneely, KN, Jackson, AU, et al. Comprehensive association study of type 2 diabetes and related quantitative traits with 222 candidate genes. Diabetes. (2008) 57:3136–44. doi: 10.2337/db07-1731
41. Eny, KM, Wolever, TMS, Fontaine-Bisson, B, and El-Sohemy, A. Genetic variant in the glucose transporter type 2 is associated with higher intakes of sugars in two distinct populations. Physiol Genomics. (2008) 33:355–60. doi: 10.1152/physiolgenomics.00148.2007
42. Karlsson, T, Rask-Andersen, M, Pan, G, Höglund, J, Wadelius, C, Ek, WE, et al. Contribution of genetics to visceral adiposity and its relation to cardiovascular and metabolic disease. Nat Med. (2019) 25:1390–5. doi: 10.1038/s41591-019-0563-7
43. Kichaev, G, Bhatia, G, Loh, P-R, Gazal, S, Burch, K, Freund, MK, et al. Leveraging polygenic functional enrichment to improve GWAS power. Am J Hum Genet. (2019) 104:65–75. doi: 10.1016/j.ajhg.2018.11.008
44. Thorleifsson, G, Walters, GB, Gudbjartsson, DF, Steinthorsdottir, V, Sulem, P, Helgadottir, A, et al. Genome-wide association yields new sequence variants at seven loci that associate with measures of obesity. Nat Genet. (2009) 41:18–24. doi: 10.1038/ng.274
45. Junyent, M, Parnell, LD, Lai, C-Q, Lee, Y-C, Smith, CE, Arnett, DK, et al. Novel variants at KCTD10, MVK, and MMAB genes interact with dietary carbohydrates to modulate HDL-cholesterol concentrations in the genetics of lipid lowering drugs and diet network study. Am J Clin Nutr. (2009) 90:686–94. doi: 10.3945/ajcn.2009.27738
46. Chen, Y, Zhu, J, Lum, PY, Yang, X, Pinto, S, MacNeil, DJ, et al. Variations in DNA elucidate molecular networks that cause disease. Nature. (2008) 452:429–35. doi: 10.1038/nature06757
47. Kathiresan, S, Willer, CJ, Peloso, GM, Demissie, S, Musunuru, K, Schadt, EE, et al. Common variants at 30 loci contribute to polygenic dyslipidemia. Nat Genet. (2009) 41:56–65. doi: 10.1038/ng.291
48. Noordam, R, Bos, MM, Wang, H, Winkler, TW, Bentley, AR, Kilpeläinen TO, et al. Multi-ancestry sleep-by-SNP interaction analysis in 126,926 individuals reveals lipid loci stratified by sleep duration. Nat Commun. (2019) 10:5121. doi: 10.1038/s41467-019-12958-0
49. Trompet, S, de Craen, AJ, Postmus, I, Ford, I, Sattar, N, Caslake, M, et al. Replication of LDL GWAs hits in PROSPER/PHASE as validation for future (pharmaco)genetic analyses. BMC Med Genet. (2011) 12:131. doi: 10.1186/1471-2350-12-131
50. Middelberg, RPS, Heath, AC, Madden, PAF, Montgomery, GW, Martin, NG, and Whitfield, JB. Evidence of differential allelic effects between adolescents and adults for plasma high-density lipoprotein. PLoS One. (2012) 7:e35605. doi: 10.1371/journal.pone.0035605
51. Mohlke, KL, Boehnke, M, and Abecasis, GR. Metabolic and cardiovascular traits: an abundance of recently identified common genetic variants. Hum Mol Genet. (2008) 17:R102–8. doi: 10.1093/hmg/ddn275
52. Gkouskou, KG, Georgiopoulos, G, Vlastos, I, Lazou, E, Chaniotis, D, Papaioannou, TG, et al. CYP1A2 polymorphisms modify the association of habitual coffee consumption with appetite, macronutrient intake, and body mass index: results from an observational cohort and a cross-over randomized study. Int J Obes. (2022) 46:162–8. doi: 10.1038/s41366-021-00972-6
53. Bersaglieri, T, Sabeti, PC, Patterson, N, Vanderploeg, T, Schaffner, SF, Drake, JA, et al. Genetic signatures of strong recent positive selection at the lactase gene. Am J Hum Genet. (2004) 74:1111–20. doi: 10.1086/421051
54. Hoffmann, TJ, Choquet, H, Yin, J, Banda, Y, Kvale, MN, Glymour, M, et al. A large multiethnic genome-wide association study of adult body mass index identifies novel loci. Genetics. (2018) 210:499–515. doi: 10.1534/genetics.118.301479
55. Cole, JB, Florez, JC, and Hirschhorn, JN. Comprehensive genomic analysis of dietary habits in UK biobank identifies hundreds of genetic associations. Nat Commun. (2020) 11:1467. doi: 10.1038/s41467-020-15193-0
56. Qin, Y, Havulinna, AS, Liu, Y, Jousilahti, P, Ritchie, SC, Tokolyi, A, et al. Combined effects of host genetics and diet on human gut microbiota and incident disease in a single population cohort. Nat Genet. (2022) 54:134–42. doi: 10.1038/s41588-021-00991-z
57. Schroor, MM, Mokhtar, FBA, Plat, J, and Mensink, RP. Associations between SNPs in intestinal cholesterol absorption and endogenous cholesterol synthesis genes with cholesterol metabolism. Biomedicine. (2021) 9:1475. doi: 10.3390/biomedicines9101475
58. Avsar, O, Kuskucu, A, Sancak, S, and Genc, E. Are dopaminergic genotypes risk factors for eating behavior and obesity in adults? Neurosci Lett. (2017) 654:28–32. doi: 10.1016/j.neulet.2017.06.023
59. Kring, SII, Werge, T, Holst, C, Toubro, S, Astrup, A, Hansen, T, et al. Polymorphisms of serotonin receptor 2A and 2C genes and COMT in relation to obesity and type 2 diabetes. PLoS One. (2009) 4:e6696. doi: 10.1371/journal.pone.0006696
60. Warodomwichit, D, Shen, J, Arnett, DK, Tsai, MY, Kabagambe, EK, Peacock, JM, et al. ADIPOQ polymorphisms, monounsaturated fatty acids, and obesity risk: the GOLDN study. Obesity. (2009) 17:510–7. doi: 10.1038/oby.2008.583
61. Cauchi, S, Nead, KT, Choquet, H, Horber, F, Potoczna, N, Balkau, B, et al. The genetic susceptibility to type 2 diabetes may be modulated by obesity status: implications for association studies. BMC Med Genet. (2008) 9:45. doi: 10.1186/1471-2350-9-45
62. Mohamed, AA, Alrohaimi, AH, Sayed Abdelgeliel, A, Albogami, S, Jafri, I, Fayad, E, et al. The impact of LEP rs7799039 polymorphism and obesity on the severity of coronavirus Disease-19. Diabetes Metab Syndr Obes. (2023) 16:515–22. doi: 10.2147/DMSO.S391869
63. Martins, MC, Trujillo, J, Farias, DR, and Kac, G. Polymorphisms in the leptin (rs7799039) gene are associated with an increased risk of excessive gestational weight gain but not with leptin concentration during pregnancy. Nutr Res. (2017) 47:53–62. doi: 10.1016/j.nutres.2017.09.003
64. Kaur, H, Badaruddoza, B, Bains, V, and Kaur, A. Genetic association of ADIPOQ gene variants (−3971A>G and +276G>T) with obesity and metabolic syndrome in north Indian Punjabi population. PLoS One. (2018) 13:e0204502. doi: 10.1371/journal.pone.0204502
65. Uddin, MS, Rahman, MM, Sufian, MA, Jeandet, P, Ashraf, GM, Bin-Jumah, MN, et al. Exploring the new horizon of AdipoQ in obesity-related Alzheimer’s dementia. Front Physiol. (2021) 11:567678. doi: 10.3389/fphys.2020.567678
66. Truong, S, Tran, NQ, Ma, PT, Hoang, CK, Le, BH, Dinh, T, et al. Association of ADIPOQ single-nucleotide polymorphisms with the two clinical phenotypes type 2 diabetes mellitus and metabolic syndrome in a Kinh Vietnamese population. Diabetes Metab Syndr Obes. (2022) 15:307–19. doi: 10.2147/DMSO.S347830
67. Leońska-Duniec, A, Grzywacz, A, Jastrzębski, Z, Jażdżewska, A, Lulińska-Kuklik, E, Moska, W, et al. ADIPOQ polymorphisms are associated with changes in obesityrelated traits in response to aerobic training programme in women. Biol Sport. (2018) 35:165–73. doi: 10.5114/biolsport.2018.72762
68. Greenhill, C. Link between MC4R mutations and adiposity. Nat Rev Endocrinol. (2021) 17:449–9. doi: 10.1038/s41574-021-00524-y
69. Del Corral, P, Chandler-Laney, PC, Casazza, K, Gower, BA, and Hunter, GR. Effect of dietary adherence with or without exercise on weight loss: a mechanistic approach to a global problem. J Clin Endocrinol Metab. (2009) 94:1602–7. doi: 10.1210/jc.2008-1057
Keywords: obesity, weight loss, genetics, single nucleotide polymorphism, bariatric surgery
Citation: Mera-Charria A, Nieto-Lopez F, Francès MP, Arbex PM, Vila-Vecilla L, Russo V, Silva CCV and De Souza GT (2023) Genetic variant panel allows predicting both obesity risk, and efficacy of procedures and diet in weight loss. Front. Nutr. 10:1274662. doi: 10.3389/fnut.2023.1274662
Edited by:
Omar Ramos-Lopez, Universidad Autónoma de Baja California, Tijuana, MexicoReviewed by:
Karina Gonzalez-Becerra, Universidad de Guadalajara, MexicoElisa Barron-Cabrera, Autonomous University of Sinaloa, Mexico
Copyright © 2023 Mera-Charria, Nieto-Lopez, Francès, Arbex, Vila-Vecilla, Russo, Silva and De Souza. This is an open-access article distributed under the terms of the Creative Commons Attribution License (CC BY). The use, distribution or reproduction in other forums is permitted, provided the original author(s) and the copyright owner(s) are credited and that the original publication in this journal is cited, in accordance with accepted academic practice. No use, distribution or reproduction is permitted which does not comply with these terms.
*Correspondence: Gustavo Torres De Souza, gustavo.torres@fagrongenomics.com
†These authors have contributed equally to this work