- 1OSU Nutrition Interdisciplinary Graduate Program, The Ohio State University, Columbus, OH, United States
- 2College of Arts and Sciences, William & Mary, Williamsburg, VA, United States
- 3Independent Contractor, Warren, MN, United States
- 4Johns Hopkins Center for a Livable Future, Johns Hopkins University, Baltimore, MD, United States
- 5Department of Environmental Health and Engineering, Johns Hopkins Bloomberg School of Public Health, Baltimore, MD, United States
- 6Department of Food Science and Technology, The Ohio State University, Columbus, OH, United States
- 7Department of Kinesiology, William & Mary, Williamsburg, VA, United States
- 8Global Research Institute, William & Mary, Williamsburg, VA, United States
Introduction: Fifty-two percent of adults in the United States reported following a popular diet pattern in 2022, yet there is limited information on daily micronutrient intakes associated with these diet patterns. The objective of the present study was to model the impact on micronutrient intake when foods highest in added sugar and sodium were replaced with healthier alternatives to align with the Dietary Guidelines for Americans recommendations.
Methods: Dietary data were acquired from 34,411 adults ≥ 20 y in the National Health and Nutrition Examination Survey, 2005–2018. The National Cancer Institute methodology was used to estimate usual dietary intake at baseline of 17 micronutrients using information from up to two dietary recalls per person. A food substitution model was used to evaluate the impact on micronutrient intake when three servings of foods highest in added sugar and sodium were substituted with healthier alternatives.
Results: Dietary modeling to replace foods highest in added sugar with healthier alternatives increased the mean intake of fat-soluble vitamins (0.15% for vitamin A to 4.28% for vitamin K), most water-soluble vitamins (0.01% for vitamin B1 to 12.09% for vitamin C), and most minerals (0.01% for sodium to 4.44% for potassium) across all diet patterns. Replacing foods highest in sodium had mixed effects on the mean intake of micronutrients. The intake of most fatsoluble vitamins increased by 1.37–6.53% (particularly vitamin A and D), yet while the intake of some water-soluble vitamins and minerals increased by 0.18–2.64% (particularly vitamin B2, calcium, and iron) others decreased by 0.56–10.38% (notably vitamin B3 and B6, magnesium, sodium, and potassium).
Discussion: Modeled replacement of foods highest in added sugar led to more favorable changes in mean micronutrient intake compared to modeled replacement of foods highest in sodium. Due to the composite nature of mixed dishes that include multiple ingredients, food substitutions may result in both favorable and unfavorable changes in micronutrient intake. These findings highlight the challenges of making singleitem food substitutions to increase micronutrient intake and call for further research to evaluate optimal combinations of replacement foods to maximize the intake of all micronutrients simultaneously.
1. Introduction
The proportion of people following popular diet patterns, which typically modify the intake of macronutrients (e.g., restricted-carbohydrate), food groups (e.g., vegetarian), or eating time (e.g., time-restricted), in the United States (US) has risen from 38% in 2019 to 52% in 2022 (1, 2). During the 2005–2018 period, an average of 44% of people in the US tried at least one popular diet pattern on a given day that restricted macronutrients, food groups, or eating time (3).
Prior research has demonstrated that the overall quality of popular diet patterns when measured using the Healthy Eating Index (HEI)-2015–which measures adherence to the Dietary Guidelines for Americans (DGA)–is poor, ranging from 52–65 points out of 100 (3, 4). When these diets were modified by replacing foods highest in added sugar, sodium, saturated fat, and refined grains with healthier alternatives, diet quality improved modestly (2.5 to 9.8 points), pulling HEI scores slightly above the average score of the US population (58 out of 100), but all diet patterns remained far below optimal (3). Others have shown that dietary modeling to accommodate DGA recommendation had heterogenous effect on the intake of food groups, such as elevating the intake of fruits and vegetables, respectively, up to 47% and 10%, but decreasing the intake of dairy by as much as 11%, across different popular diet patterns (5). To our knowledge, dietary modeling has not been used to assess micronutrient intake in popular diet patterns that restrict food groups, macronutrients, or eating time.
The 2020–2025 DGA recommends limiting the intake of foods high in added sugar and sodium and increasing the intake of fruits, vegetables, whole grains, and sources of unsaturated fats (6). About 13% of the total energy intake of US adults is derived from added sugar (about 17 teaspoons or 85 grams per day) whereas the DGA-recommended intake is <10% (6–8). Most adults (about 90%) have very high sodium intake with a mean of 3,468 mg/day, 51% higher than the Chronic Disease Risk Reduction (CDRR) (2,300 mg/day) (9). People in the US who consume more than 10% of energy from added sugar have a 7–103% increased risk of death from cardiovascular diseases (CVD) compared to those who consume 7.4% energy from added sugar (10). Others have shown that CVD risk increases by 17% for every additional 1,000 mg/day of sodium intake (11).
Many foods are consumed as mixed dishes that contain multiple ingredients, so limiting the intake of foods high in added sugar and sodium may also impact the intake of some micronutrients. Hence, the purpose of any food substitution to comply with the DGA recommendation is not only to improve a single component in diet but also to improve overall diet quality, including micronutrient intake. It is therefore critical to evaluate whether replacing foods highest in added sugar and sodium with healthier alternatives impacts micronutrient intake among those that follow popular diet patterns. To address this research need, the present study models the changes in the mean daily intake of 17 micronutrients for three popular diet patterns (low grain, restricted-carbohydrate, and time-restricted) in the US when 3 servings of foods highest in added sugar or sodium were replaced with healthier alternatives as per the DGA recommendations.
2. Materials and methods
2.1. Data acquisition
Individual-level data on food intake, nutrient intake from foods and supplements, and sociodemographic status were retrieved from the National Health and Nutrition Examination Survey (NHANES), 2005–2018. NHANES collects data from approximately 5,000 non-institutionalized participants per year using a multi-stage, stratified, clustered sampling design, and some population groups are oversampled (12). Trained interviewers collect dietary data using the computer-assisted Automated Multiple Pass Method to minimize respondent burden and increase reliability and validity (13, 14). Most (80%) participants complete a second recall by telephone 3–10 days later (15). The salt adjustment was appropriately removed from dietary data collected from 2005–2008 to standardize measurement of sodium intake across all data years (16). Many participants reported consuming mixed dishes that include multiple food groups, so the Food Patterns Equivalents Database (FPED) was used to convert each NHANES mixed dish into one or more food groups that represent those included in the DGA (17). This study is a secondary analysis of de-identified and publicly available data and was exempted from human studies ethical review by the Institutional Review Board at William & Mary. Pre-registration for this study can be found elsewhere (18).
2.2. Baseline (current) dietary intake
The National Cancer Institute’s (NCI) usual intake methodology was used to estimate current (baseline) dietary intake of kcal and nutrients. This method estimates within-person variation of the entire sample using data from two 24-h recalls collected from most participants (19). The SAS macros MIXTRAN (v2.21) and INDIVINT (v2.3) were used to estimate predicted intake at the individual level. The probability of consumption was assumed to be correlated to the amount consumed for nutrients consumed episodically (20); which include the polyunsatured fatty acids, namely eicosapentaenoic acid (20:5) and docosahexaenoic acid (22:6). The SAS macros NLMIXED_UNIVARIATE (v1.2), NLMIXED_BIVARIATE (v1.2) and PREDICT_INTAKE_DENSITY (v1.2) were used to estimate nutrient densities (e.g., percent energy from carbohydrate) (21–23).
2.3. Diet pattern categorization
Each participant was categorized into one or more diet patterns using data on usual food and nutrient intake at baseline: low grain, restricted-carbohydrate, and time-restricted. Supplementary Table 1 describes the characteristics of these diet patterns, which was informed by published literature (24–28). Low grain, restricted-carbohydrate, and time-restricted diet patterns were categorized, respectively, using data on daily intake of food groups from FPED, daily nutrient intake from NHANES nutrient files, and the amount of time between each eating occasion for each participant from the NHANES individual foods file. It was possible for participants to be categorized into more than one diet pattern. The NCI methodology does not predict zero intake, so non-consumers were identified if they did not consume any amount of a given food group on both days of recall. To provide relevance to the general population, an additional diet pattern was established that included all participants that met the inclusion criteria specified below, regardless of whether they were categorized into one of the dietary patterns described above.
2.4. Food categories and serving sizes
The categorization scheme used by the Food and Nutrient Database for Dietary Studies (FNDDS) (29) and FPED (17) was used to categorize each food and beverage consumed by participants on the first day of dietary recall into 89 mutually exclusive categories. For example, pasta dishes were identified using FNDDS and were further disaggregated into whole grain and refined grain pasta dishes using FPED (Supplementary Table 2). Data on the gram weight of each food consumed as well as their kcal and nutrient content were acquired from NHANES files, and data on the amount of added sugar present in each food were acquired from FPED files. Average serving sizes of each food category were estimated from these data, as well as the average amount of kcal, added sugar, and sodium present per serving of each food category, as described below.
Serving sizes for each food category were estimated for the entire sample by averaging the gram weight of each food consumed within each category at each eating occasion. For example, there were 174 types of refined grain pasta dishes that were consumed on 5,424 occasions, and the average amount (in grams) of refined grain pasta dishes consumed at each eating occasion was used as the serving size for this food category. All computed serving sizes for packaged foods and beverages were consistent with serving sizes on the Nutrition Facts Panel of these products (30), and the computed serving sizes for non-packaged foods were consistent with serving sizes provided by FPED documentation (31). To estimate the amount of kcal, nutrients, and added sugar per serving of each food category, the amount of these food components per gram of each food was averaged across all foods within each food category and was multiplied by the average serving size (in grams) of each food category.
2.5. Target foods and alternative foods
For each diet pattern, food categories to be removed during modeling (target foods) were those that represented the greatest daily intake of added sugar and sodium using data from the first day of dietary recall. Alternative food categories were selected based on four criteria: (1) adhered to the principles of each diet pattern (e.g., a grain dish was not used as an alternative food for the low grain diet pattern), (2) represented a reasonable dietary substitution that an individual may make, as determined through consultation and consensus with multiple Registered Dietitian Nutritionists (RDN) as described by Conrad et al. (3) (e.g., dishes were replaced with dishes, beverages with beverages, snacks with snacks, and desserts with desserts), (3) it was consumed in the greatest quantity of the remaining options, and (4) it had a lower content of added sugar and sodium per serving compared to the target food.
2.6. Diet modeling
A diet model was constructed to evaluate the effects on nutrient intake if up to 3 servings of food categories highest in added sugar or sodium (target foods) were substituted with up to 3 servings of alternative foods, which is consistent with DGA 2020–2025 recommendations to limit these components in the diet (32). To allow for discretionary intake, the model only performed substitutions if a participant consumed at least the number of servings of the target food compared to the alternative food. For example, if a participant consumed 0.9 servings of a target food it would not get substituted with an alternative food, but if they consumed 1.1 servings of a target food it would get substituted with 1 serving of an alternative food. Similarly, if a participant consumed 2.9 servings of a target food it would get substituted with 2 servings of an alternative food. All substitutions were made on the basis of whole servings (1, 2, or 3 servings) rather than mass quantity or kcal to reflect the units in which individuals typically consume foods and beverages. Individual-level intake of kcal and nutrients were estimated at baseline and after modeling.
2.7. Statistical analyses
Micronutrient intake was evaluated at baseline (i.e., current intake) and after modeling. Means were unadjusted and tests of significance were adjusted for age, sex, and NHANES survey cycle using linear regression models. Survey weights and design variables from NHANES were used to account for the multistage probability sampling design and to produce nationally representative estimates. SAS 9.4 (SAS Institute; Cary, NC, USA) was used to estimate usual intakes using the NCI macros, and Stata 16.1 (StataCorp; College Station, TX, USA) was used for data management and all other analyses.
3. Results
3.1. Sample characteristics
Participants were excluded from the present study if they were less than 20 years old (n = 26,375) or pregnant or lactating women or did not provide complete and reliable dietary information (n = 896). Of the 61,682 participants in NHANES 2005–2018 that provided dietary data, 34,411 participants met the inclusion criteria.
Details of the participant characteristics are included in Table 1. In the general population group, the mean age of participants was 48 years, approximately half were female (51%), and the majority were non-Hispanic white (67%). The mean income-to-poverty ratio of those in the general population was 3.0, and 61% of the participants attended some college. Of participants that were categorized into a popular diet pattern, most followed a restricted-carbohydrate diet (29%), followed by low grain (10%), and time-restricted (9%) (Table 1). Participants that followed a low grain diet pattern had a mean age of 50 years, were mostly female (70%) and non-Hispanic white (70%), 59% attended some college, and had a mean income-to-poverty ratio of 2.8. Participants that consumed a restricted-carbohydrate diet pattern had a mean age of 48 years, over half were male (56%), most were non-Hispanic white (73%) and attended some college (66%), and had a mean income-to-poverty ratio of 3.3. Participants that followed a time-restricted diet were younger (43 years), had less educational attainment (48% attended college), and had a lower income-to-poverty ratio (2.4). About half of the participants in the time-restricted diet pattern group were female (52%) and non-Hispanic white (52%).
3.2. Sources of added sugar and sodium
All diet patterns evaluated in the present study had the same food sources that were highest contributors of added sugar (soft drinks with added sugar) and sodium (poultry dishes; Supplementary Table 3). Soft drinks with added sugar contributed to 17.9–35.6% of daily intake of added sugar for all diet patterns and poultry dishes contributed to 8.5–9.5% of daily sodium intake for all diet patterns. To generate modeled diets, soft drinks with added sugar were replaced by soft drinks without added sugar in the general population, restricted-carbohydrate, and time-restricted diet patterns, but were replaced by 100% fruit juice in the low grain diet pattern. In the case of modeled diets for sodium, poultry dishes were substituted with egg dishes in all diet patterns. The nutrient content per serving of target food items and their healthier alternatives are presented in the Supplementary Table 4.
3.3. Micronutrient intake at baseline
Mean baseline intake of nutrients and energy are presented in Supplementary Tables 5–8. Intakes of all fat-soluble vitamins were lower among participants that followed a time-restricted diet pattern than the general population. The low grain diet pattern had lower mean intake of vitamin A (825.51 μg; 95% CI: 786.98, 864.05) and vitamin E (7.11 IU; 6.94, 7.3) compared to the general population, whereas the restricted-carbohydrate diet pattern had higher mean intake of vitamin E (8.64 IU; 8.53, 8.75) and vitamin K (114.89 μg; 113.34, 116.44) than the general population. Similar to the fat-soluble vitamins, intakes of all water-soluble vitamins were lower among participants that followed a time-restricted diet pattern compared to the general population. The mean intakes of folate among participants in each of the three popular diet patterns (454.74–575.92 μg) were lower compared with the general population (614.80 μg; 609.38, 620.22). Compared to the general population, participants that followed a low grain diet pattern had a lower mean intake of vitamin B3 (25.43 mg; 24.43, 26.43), and participants that followed a restricted-carbohydrate diet pattern had a lower intake of vitamin B6 (4.39 mg; 4.07, 4.71) but a higher intake of vitamin B3 (34.18 mg; 33.3, 35.05) compared to the general population.
The intake of six minerals (calcium, magnesium, iron, zinc, sodium, and potassium) were lower in the low grain and time-restricted diet patterns compared to the general population. The restricted-carbohydrate diet pattern had higher mean intake of magnesium (337.15 mg; 332.64, 341.66), zinc (15.29 mg; 15.07, 15.5), sodium (3,705.48 mg; 3,677.95, 3,733.01), and potassium (2,735.06 mg; 2,711.45, 2,758.67) than the general population.
3.4. Micronutrient intake in response to modeled replacement of foods highest in added sugar
Figure 1 presents the percent changes in mean micronutrient intake after modeling, and Supplementary Tables 5–8 presents the mean and the 95% confidence interval (CI) of micronutrient intakes before and after modeling. Modeled replacement of foods highest in added sugar with healthier alternatives led to reduced energy intake (0.92–4.5%) and increased intake of most micronutrients across popular diet patterns (0.01–12.09% increase) and the general population (0.03–5.38% increase). The highest increase among fat-soluble vitamins was estimated for vitamin K (4.28%; corresponding to 4.55 μg increase from baseline) in the low grain diet pattern, which also had the highest increase for vitamin C intake (12.09%; 20 mg). The lowest change in vitamin C intake was estimated for the restricted-carbohydrate diet pattern (1.93% increase), corresponding to a 2.97 mg increase from baseline (154 mg). Of the minerals, the highest change in intake was estimated for potassium (4.44%; 104.86 mg increase), followed by calcium (3.55%; 31.08 mg increase) among participants with a low grain diet pattern. The rest of the changes in micronutrient intakes were relatively small (0.01–2.44%), especially when there were decreases in intake (less than 1%). No changes were evident for vitamin D intakes among participants in the restricted-carbohydrate and time-restricted diet patterns, and among participants in the general population; and no changes were observed for vitamin B12 and iron intakes among participants in the restricted-carbohydrate diet pattern. All changes in the energy intake and intakes of micronutrient are statistically significant (p < 0.05 using paired Wald tests), except for the vitamin D.
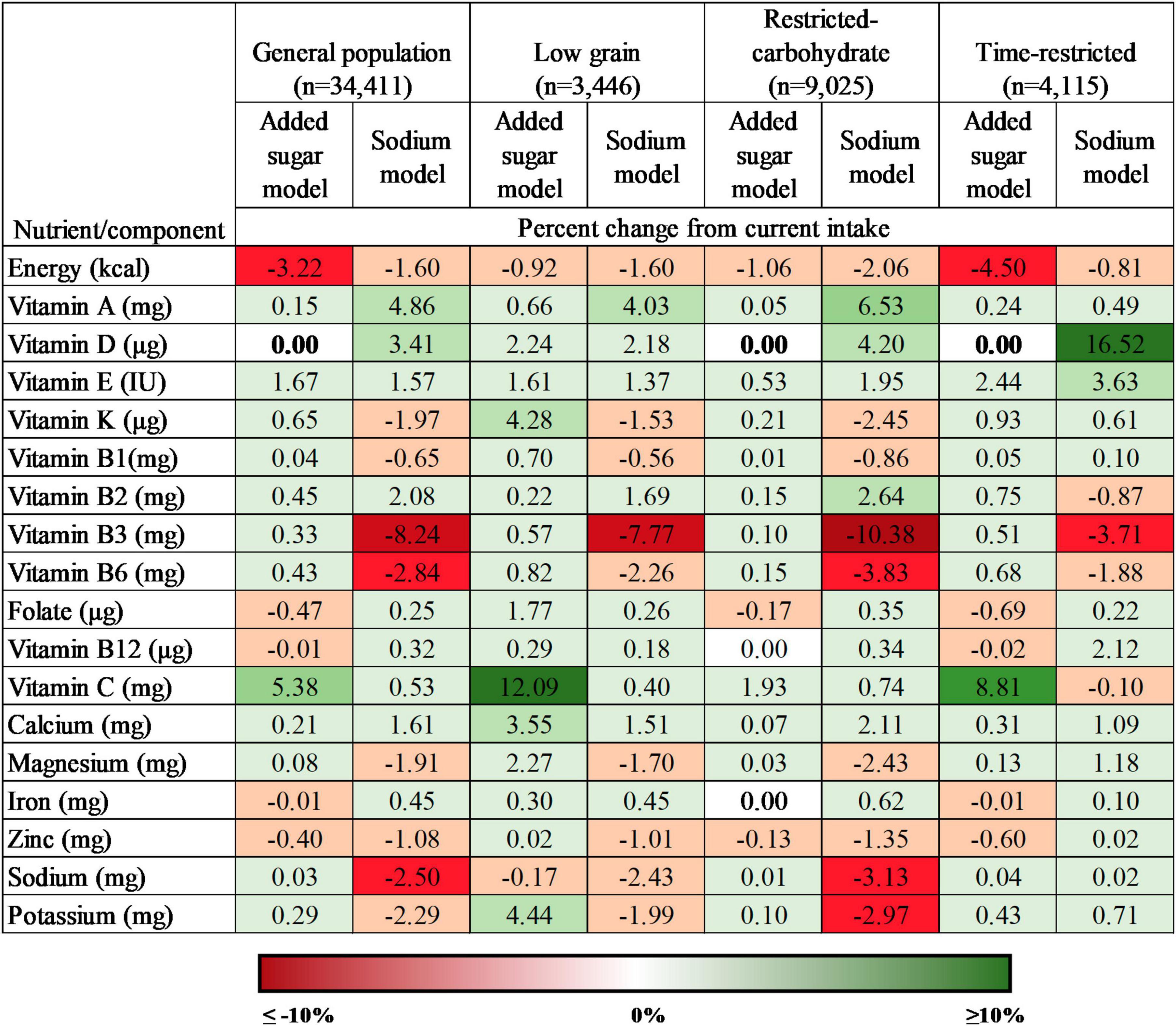
Figure 1. Percent change in intake of energy and micronutrients under conditions of modeled food replacement for popular diet patterns and the general population, 2005–2018 (n = 34,411). All values are statistically significant at P < 0.05 using paired Wald tests, except those in bold text.
3.5. Micronutrient intake in response to modeled replacement of foods highest in sodium
Modeled replacement of sodium rich foods (Figure 1 and Supplementary Tables 5–8) decreased mean energy intake (1.6–2.06%) as well as increased mean intake of vitamins A (4.03–6.53%; corresponding to 33–58 μg), D (2.18–5.77%; 0.43–0.54 μg), and E (1.37–1.95%; 0.1–0.17 IU), and decreased intakes of vitamin K (1.53–2.08%; 1.63–1.95 μg), vitamin B1 (0.56–0.86%; 0.02–0.03 mg), vitamin B3 (7.77–10.38%; 1.98–3.55 mg), B6 (2.26–3.83%; 0.1–0.17 mg), magnesium (1.7–2.43%; 4.73–8.19 mg), zinc (1.01–1.21%; 0.12–0.14 mg), sodium (2.43–3.13%; 67–116 mg), and potassium (1.99–2.5%; 47–56 mg) among all diet patterns. The maximum increase in water-soluble vitamins was estimated for vitamin B2 (2.64%; 0.1 mg) for the restricted-carbohydrate diet pattern. Among the six minerals assessed in this study, only calcium (1.51–2.11%; 13.21–22.85 mg) and iron (0.45–0.62%; 0.06–0.1 mg) increased across all diet patterns. All results were statistically significant (p < 0.05) using paired Wald tests.
4. Discussion
In this nationally representative modeling study of individuals that consumed popular diet patterns, replacing foods highest in added sugar and sodium with healthier alternatives reduced energy intake and increased intake of many micronutrients, with some exceptions. Modeled replacement of foods highest in added sugar led to a modest decrease in the mean intake of folate, vitamin B12, iron, and zinc for most diet patterns, and modeled replacement of foods highest in sodium led to larger decreases in the mean intake of vitamins B3 and B6 for most diet patterns. These findings highlight the challenges of making single-item food substitutions to increase micronutrient intake, which can lead to favorable changes in intake of some micronutrients but not others.
In the US, high consumption of added sugar and sodium is associated with elevated risk of morbidity and mortality from obesity and CVD (33). Added sugar consumption in the US decreased from 18% of total energy in 1999–2000 (34) to 13% in 2017–2018 (35), but remained above the recommended threshold of 10% (6). In the present study, added sugar contributed to more than 10% of total energy, except for participants that consumed the restricted-carbohydrate diet pattern. Soft drinks are the principal source of added sugar in the diet of adults in the US (7, 36), which was also confirmed in the present study. After modeled replacement, the percentage of total energy from added sugar decreased by 0.7 to 2.8 percentage points across all diet patterns. Almost 90% of US adults exceed the CDRR threshold for daily sodium intake (9, 37) of 2,300 mg/day. Dietary modeling to substitute foods highest in added sugar and sodium with healthier alternatives reduced energy intake as expected, but dietary modeling to substitute three servings of sodium-rich items was not enough to bring sodium intake below 2,300 mg/day. To have a significant reduction in CVD related mortality in the US, reducing daily sodium intake by 40% or to 1,500 mg has been proposed (38), whereas the present study estimated less than a 5% (corresponds to 5–115 mg) reduction.
Factors that drive the extent of changes in micronutrient intake in the modeled diet patterns are the nutrient composition of replaced and alternative foods, the content of undesired food components in alternative foods, and the mean intake of micronutrients at baseline. The nutrient composition of the replaced items and the alternative items determines the level of change in micronutrient content of modeled diets. For instance, others showed that replacing typical breakfast foods with ready-to-eat cereals improved the intake of several micronutrients (vitamin D, folate, iron, and dietary fiber), and an additional increase for calcium and potassium was achieved when milk was added (39).
Modeled diet patterns that replaced foods highest in added sugar with soft drinks without added sugar led to moderately lower intakes of folate (measured as dietary folate equivalent; 0.17–0.69%), vitamin B12 (0.01–0.02%), iron (0.01%), and zinc (0.04–0.06%). However, in the low grain diet pattern, foods highest in added sugar (soft drinks with added sugar) were replaced with 100% fruit juice which resulted in increased intake of all micronutrients (0.02–12.09%, with the highest increase observed for vitamin C). The high vitamin C content of 100% fruit juices (40) (Supplementary Table 4) contributed to the increase of vitamin C in the modeled diet patterns, which might also be true for the increase in other micronutrients as micronutrient content per serving of the 100% fruit juice is higher than that of soft-drinks without added sugar. Similarly, the nutrient compositions of soft drinks with added sugar and soft drinks without added sugar are similar, except for energy and a few minerals (calcium, magnesium, and potassium) (Supplementary Table 4), which may have led to minute or no changes in intake of vitamins (e.g., vitamin D, K, B1, B2, B12) and minerals (iron, zinc, calcium, sodium, etc.) after dietary modeling in the general population, restricted-carbohydrate, and time-restricted diet patterns.
Improving the intake of a single dietary component might increase the intake of other undesired food components (41) and limit the intake of under-consumed micronutrients. In the present study, modeled replacement of foods highest in sodium (poultry dishes) with healthier alternatives (egg dishes) increased the intake of saturated fat (1.43–1.75%) and cholesterol (12.6–17.37%). Similarly, modeled replacement of foods highest in sodium increased the intake of several under-consumed micronutrients (e.g., vitamin A, vitamin D, folate, and calcium), but at the same time it decreased the intake of several important micronutrients such vitamin B3, vitamin B6, and potassium. The mixed nature of replacement dishes (i.e., including nutrients to encourage as well as nutrients to limit) may result in both favorable and unfavorable changes. This is consistent with a similar study that assessed changes in consumption of food groups using modeled substitutions, and found an increase in modeled intake of fruits and whole grains but a decrease in modeled intake of vegetables in some popular diet patterns (5). Further research is needed to utilize optimization modeling to identify food substitutions that lead to favorable changes in all or most micronutrients.
Finally, because the present study used the percentage change from baseline intake as the unit of measure, the level of intake of a micronutrient at baseline affected the percent increase in the modeled diets. For example, identical food items with equivalent weight were substituted, yet a lower baseline intake of vitamin C among participants that consumed time-restricted diet patterns (122.05 mg) than participants that consumed restricted-carbohydrate diet patterns (154.0 mg) resulted in a higher increase in vitamin C intake (8.8 vs. 1.93%) after dietary modeling.
This study provides a foundation for future studies that could evaluate intake of micronutrient from popular diet patterns. Other tools are available to examine micronutrient intake, including indices that measure the intake of multiple nutrients collectively, such as total nutrient intake (TNI), mean probability of adequacy (MPA), and the Nutrient Rich Food Index 9.3 (NRF9.3) (42, 43). Further research could apply these tools to popular diet patterns and could be used to gauge the extent of achievable improvement through modeled replacement with different foods. In addition, studies could estimate the proportion of people within each diet pattern at risk of developing micronutrient inadequacy by comparing with the Recommended Dietary Allowance (RDA) and the Tolerable Upper Intake Level (UL), which can help inform dietary interventions. It will also be useful to compare different alternative foods, especially those with and without artificial sweeteners as the World Health Organization (WHO) suggests that long-term intake of high amounts of artificial sweeteners may present adverse health effects (44).
The present study has several strengths. To the authors’ knowledge, this is the first study to report the mean micronutrient intake of low grain, restricted-carbohydrate, and time-restricted diet patterns in the US. Data were collected from a large, nationally representative sample of over 34,000 participants over a 14-year period, making these findings generalizable to the US population. For the first time, this study also evaluated the changes in micronutrient intake for people following popular diet patterns when foods highest in added sugar and sodium were replaced with healthier alternatives. Diet patterns were categorized using data from multiple 24-h recalls instead of participants’ self-reported adherence to diet patterns, because mischaracterization of diet patterns by study participants is common (45, 46). Serving sizes were also estimated from this nationally representative data and were consistent with those provided on the Nutrition Facts Panel (30) and published in the United States Department of Agriculture (USDA) FPED (17). Additionally, the use of a serving in lieu of mass quantity reflects the units in which most people make food substitutions.
This study also has some limitations. As with all self-reported dietary data, 24-h recalls are subject to recall bias and social desirability bias which might lead to under- or over-estimation of micronutrient intake (47, 48). However, 24-h dietary recalls provide valuable and highly detailed dietary information and are especially useful when assessing dietary information from large samples (47, 48). This study evaluated replacing one target food with one alternative food, and other substitutions may yield different results. Similarly, substitution with alternative foods of equal weight or energy could produce different results than the present study. The present study included three popular diet patterns, and other diet patterns such as vegetarian and pescatarian were not included since less than 3% of the participants in our sample followed them, which has been reported elsewhere (3). However, diet patterns that are commonly reported but have low adherence in practice could be a potent future research area to explore. Also, micronutrient intake was not assessed by any nutrient adequacy index in the present study, thus future research could use such nutrient indices to evaluate micronutrient adequacy of popular diet patterns for guiding people to improve nutrition and health outcomes. Finally, these results represent average dietary changes of population subgroups which can produce overgeneralized results for some individuals.
5. Conclusion
Dietary modeling to replace foods highest in added sugar and sodium with healthier alternatives, as per DGA 2020–2025 recommendations, had heterogeneous effects on mean micronutrient intake across popular diet patterns. Modeled replacement of foods highest in added sugar led to more dramatic improvements in micronutrient intake with more modest reduction of energy intake than replacement of foods highest in sodium. When interpreting these results, one should consider the difference in nutrient composition of replacement foods, which determines the extent of benefits for micronutrient intake. The results of the present study give directions for future research regarding micronutrient adequacy of popular diet patterns. Optimization modeling approaches may be useful in estimating the appropriate combinations of different alternative foods items to maximize micronutrient adequacy.
Data share policy
Data described in this manuscript, code book, and analytic code will be made publicly and freely available without restriction at https://archive.org/details/osf-registrations-z3fme-v1. This study was pre-registered at the Center for Open Science, Open Science Framework at https://archive.org/details/osf-registrations-z3fme-v1.
Data availability statement
Publicly available datasets were analyzed in this study. This data can be found here: https://wwwn.cdc.gov/nchs/nhanes/.
Ethics statement
Ethical review and approval were waived for this study because it is a secondary analysis of publicly available and de-identified data (William & Mary Institutional Review Board, PHSC-2022-03-02-15499-zsconrad, 03/02/2022).
Author contributions
ZC and MB: conceptualization, methodology, and funding acquisition. ZC and LJ: software, validation, formal analysis, and investigation. ZC: resources, supervision, and project administration. ZC, ABT, SR, and LJ: data curation. ABT: writing—original draft preparation. ZC, ABT, and DL: visualization. All authors wrote, reviewed and edited the manuscript, read, and agreed to the published version of the manuscript.
Funding
This work was supported by the Institute for the Advancement of Food and Nutrition Sciences Carbohydrate Committee (IAFNS) and the Margaret S. Glauber Faculty-Student Fellowship at William & Mary. IAFNS is a non-profit science organization that pools funding from industry collaborators and advances science through in-kind and financial contributions from public and private sector participants. DL was supported by the Johns Hopkins Center for a Livable Future with a gift from the Greater Kansas City Community Foundation. The funders had no role in the design, analysis, interpretation, or presentation of the data and results. View this project on the Open Science Framework at https://archive.org/details/osf-registrations-z3fme-v1.
Conflict of interest
ZC: had research awards from the Thomas F. and Kate Miller Jeffress Memorial Trust for a project unrelated to the present study; and received honoraria from Routledge, Princeton University Press, MKYoung Food & Nutrition Strategies, National Geographic Society, The Ohio State University, and Nutrition Today for professional activities unrelated to the present research. MB: had a research award from the United Soybean Board that has no relevance to the present project and serves on the Board of Directors for the American Society for Nutrition in a role that is unrelated to this project.
The remaining authors declare that the research was conducted in the absence of any commercial or financial relationships that could be construed as a potential conflict of interest.
Publisher’s note
All claims expressed in this article are solely those of the authors and do not necessarily represent those of their affiliated organizations, or those of the publisher, the editors and the reviewers. Any product that may be evaluated in this article, or claim that may be made by its manufacturer, is not guaranteed or endorsed by the publisher.
Supplementary material
The Supplementary Material for this article can be found online at: https://www.frontiersin.org/articles/10.3389/fnut.2023.1217774/full#supplementary-material
Abbreviations
CVDs, cardiovascular disease; DGA, Dietary Guidelines for Americans; FNDDS, Food and Nutrient Database for Dietary Studies; FPED, Food Patterns Equivalents Database; NCI, National Cancer Institute; MPA, mean probability of adequacy; NHANES, National Health and Nutrition Examination Survey; (NRF9.3), Nutrient Rich Food Index 9.3; RDA, Recommended Dietary Allowance; RDN, Registered Dietitian Nutritionist; TNI, total nutrient intake; US, United States.
References
1. International Food Information Council [IFIC]. Food and Health Survey. Washington, DC: IFIC (2022).
2. Dietary Guidelines Advisory Committee. Dietary Patterns. Scientific Report of the 2020 Dietary Guidelines Advisory Committee: Advisory Report to the Secretary of Agriculture and the Secretary of Health and Human Services. Washington, DC: U.S. Department of Agriculture (2020).
3. Conrad Z, Kowalski C, Dustin D, Johnson L, McDowell A, Salesses M, et al. Quality of popular diet patterns in the united states: evaluating the effect of substitutions for foods high in added sugar, sodium, saturated fat, and refined grains. Curr Dev Nutr. (2022) 6:nzac119. doi: 10.1093/cdn/nzac119
4. Krebs-Smith S, Pannucci T, Subar A, Kirkpatrick S, Lerman J, Tooze J, et al. Update of the healthy eating index: HEI-2015. J Acad Nutr Diet. (2018) 118:1591–602.
5. Rowe S, Tukun A, Johnson L, Love D, Belury M, Conrad Z. Consumption of Key Food Groups by Individuals Consuming Popular Diet Patterns: Mixed Effects of Replacing Foods High in Added Sugar, Sodium, Saturated Fat, and Refined Grains. Nutrients. (2022) 14:5226. doi: 10.3390/nu14245226
6. US Department of Agriculture. Dietary Guidelines for Americans 2020–2025. Make Every Bite Count with the Dietary Guidelines. Washington, DC: U.S. Department of Agriculture (2020).
7. Bailey R, Fulgoni V, Cowan A, Gaine P. Sources of added sugars in young children, adolescents, and adults with low and high intakes of added sugars. Nutrients. (2018) 10:102.
8. U.S. Department of Agriculture ARS. Food Patterns Equivalents Intakes from Food: Mean Amounts Consumed per Individual, by Gender and Age. Washington, DC: U.S. Department of Agriculture (2020).
9. Clarke L, Overwyk K, Bates M, Park S, Gillespie C, Cogswell M. Temporal Trends in Dietary Sodium Intake Among Adults Aged ≥19 Years - United States, 2003-2016. MMWR Morb Mortal Wkly Rep. (2021) 70(42):1478–82. doi: 10.15585/mmwr.mm7042a4
10. Yang Q, Zhang Z, Gregg E, Flanders W, Merritt R, Hu F. Added sugar intake and cardiovascular diseases mortality among US adults. JAMA Intern Med. (2014) 174:516–24.
11. Cook N, Appel L, Whelton P. Lower Levels of Sodium Intake and Reduced Cardiovascular Risk. Circulation. (2014) 129:981–9.
12. Chen T, Clark J, Riddles M, Mohadjer L, Fakhouri T. The National Health and Nutrition Examination Survey, 2015-2018: Sample Design and estimation procedures. Hyattsville, MD: National Center for Health Statistics (2020).
13. US Department of Agriculture, Agricultural Research Service, Food Surveys Research Group. Automated Multiple Pass Method. Washington, DC: U.S. Department of Agriculture (2021).
14. Moshfegh A, Rhodes D, Baer D, Murayi T, Clemens J, Rumpler W, et al. The US Department of agriculture automated multiple-pass method reduces bias in the collection of energy intakes. Am J Clin Nutr. (2008) 88:324–32. doi: 10.1093/ajcn/88.2.324
15. US Department of Agriculture, Agricultural Research Service, Food Surveys Research Group. What We Eat In America: overview. Washington, DC: U.S. Department of Agriculture (2021).
16. Sebastian R, Wilkinson Enns C, Steinfeldt L, Goldman J, Moshfegh A. Monitoring sodium intake of the US population: impact and implications of a change in what we eat in America, National Health and Nutrition Examination Survey dietary data processing. J Acad Nutr Diet. (2013) 113:942–9. doi: 10.1016/j.jand.2013.02.009
17. US Department of Agriculture, Agricultural Research Service. Food Patterns Equivalents Database (FPED). Washington, DC: U.S. Department of Agriculture (2023).
18. Center for Open Science, Open Science Framework. Macronutrient intakes and diet quality for contemporary consumer diets. Charlottesville, VA: Center for Open Science (2021).
19. National Cancer Institute, National Institutes for Health. Software for measurement error in nutrition research. Bethesda, MA: National Cancer Institute (2021).
20. Krebs-Smith S, Guenther P, Subar A, Kirkpatrick S, Dodd K. Americans do not meet federal dietary recommendations. J Nutr. (2010) 140:1832–8.
21. Freedman L, Guenther P, Dodd K, Krebs-Smith S, Midthune D. The population distribution of ratios of usual intakes of dietary components that are consumed every day can be estimated from repeated 24-hour recalls. J Nutr. (2010) 140:111–6.
22. Tooze J, Kipnis V, Buckman D, Carroll R, Freedman L, Guenther P, et al. A mixed-effects model approach for estimating the distribution of usual intake of nutrients: the NCI method. Stat Med. (2010) 29:2857–68. doi: 10.1002/sim.4063
23. Kipnis V, Midthune D, Buckman D, Dodd K, Guenther P, Krebs-Smith S, et al. Modeling data with excess zeros and measurement error: application to evaluating relationships between episodically consumed foods and health outcomes. Biometrics. (2009) 65:1003–10. doi: 10.1111/j.1541-0420.2009.01223.x
24. Freire R. Scientific evidence of diets for weight loss: Different macronutrient composition, intermittent fasting, and popular diets. Nutrition. (2020) 69:110549. doi: 10.1016/j.nut.2019.07.001
25. Feinman R, Pogozelski W, Astrup A, Bernstein R, Fine E, Westman E, et al. Dietary carbohydrate restriction as the first approach in diabetes management: critical review and evidence base. Nutrition. (2015) 31:1–13.
26. Kirkpatrick C, Bolick J, Kris-Etherton P, Sikand G, Aspry K, Soffer D, et al. Review of current evidence and clinical recommendations on the effects of low-carbohydrate and very-low-carbohydrate (including ketogenic) diets for the management of body weight and other cardiometabolic risk factors: A scientific statement from the National Lipid Association Nutrition and Lifestyle Task Force. J Clin Lipid. (2019) 13:689–711.e1. doi: 10.1016/j.jacl.2019.08.003
27. Chenard C, Rubenstein L, Snetselaar L, Wahls T. Nutrient composition comparison between a modified paleolithic diet for multiple sclerosis and the recommended healthy U.S. style eating pattern. Nutrients. (2019) 11:537.
28. International Food Information Council. Food and Health Survey. Washington, DC: International Food Information Council (2020).
29. US Department of Agriculture, Agricultural Research Service. Food and Nutrient Database for Dietary Studies. Washington, DC: US Department of Agriculture (2023).
30. U.S. Food and Drug Administration. CFR - Code of Federal Regulations Title 21: 21CFR101.12. Washington, DC: U.S. Food and Drug Administration (2023).
31. United States Department of Agriculture. Food Patterns Equivalents Database (FPED) 2017-2018: Methodology and User Guide [Internet]. Washington, DC: United States Department of Agriculture (2020).
32. US Department of Health and Human Services, US Department of Agriculture. Dietary Guidelines for Americans 2020-2025: Guideline 4. Washington, DC: US Department of Agriculture (2020).
33. Yin J, Zhu Y, Malik V, Li X, Peng X, Zhang F, et al. Intake of sugar-sweetened and low-calorie sweetened beverages and risk of cardiovascular disease: a meta-analysis and systematic review. Adv Nutr. (2020) 12:89–101. doi: 10.1093/advances/nmaa084
34. Welsh J, Sharma A, Grellinger L, Vos M. Consumption of added sugars is decreasing in the United States. Am J Clin Nutr. (2011) 94:726–34.
35. Ricciuto L, Fulgoni IV, Gaine P, Scott M, DiFrancesco L. Sources of added sugars intake among the US population: Analysis by selected sociodemographic factors using the National Health and Nutrition Examination Survey 2011–18. Front Nutr. (2021) 8:687643. doi: 10.3389/fnut.2021.687643
36. Borges M, Louzada M, de Sá T, Laverty A, Parra D, Garzillo J, et al. Artificially sweetened beverages and the response to the global obesity crisis. PLoS Med. (2017) 14:e1002195. doi: 10.1371/journal.pmed.1002195
37. Jackson S, King S, Zhao L, Cogswell M. Prevalence of Excess Sodium Intake in the United States - NHANES, 2009-2012. Atlanta: U.S. Center for Disease Control (2016).
38. Coxson P, Cook N, Joffres M, Hong Y, Orenstein D, Schmidt S, et al. Mortality benefits from US population-wide reduction in sodium consumption. Hypertension. (2013) 61:564–70.
39. Rehm C, Drewnowski A. Replacing American Breakfast Foods with Ready-To-Eat (RTE) cereals increases consumption of key food groups and nutrients among US children and adults: results of an NHANES modeling study. Nutrients. (2017) 9:1010. doi: 10.3390/nu9091010
40. Haytowitz D, Jaspreet KC, Wu X, Somanchi M, Nickle M, Nguyen QA, et al. USDA National Nutrient Database for Standard Reference, Legacy Release. Washington, DC: USDA (2019).
41. Salomé M, Huneau J, Le Baron C, Kesse-Guyot E, Fouillet H, Mariotti F. Substituting meat or dairy products with plant-based substitutes has small and heterogeneous effects on diet quality and nutrient security: a simulation study in french adults (INCA3). J Nutr. (2021) 151:2435–45.
42. Cowan A, Jun S, Tooze J, Dodd K, Gahche J, Eicher-Miller H, et al. A narrative review of nutrient based indexes to assess diet quality and the proposed total nutrient index that reflects total dietary exposures. Crit Rev Food Sci Nutr. (2021) 63:1722–32. doi: 10.1080/10408398.2021.1967872
43. Imai C, Takimoto H, Fudono A, Tarui I, Aoyama T, Yago S, et al. Application of the nutrient-rich food index 9.3 and the dietary inflammatory index for assessing maternal dietary quality in Japan: a single-center birth cohort study. Nutrients. (2021) 13:2854. doi: 10.3390/nu13082854
44. World Health Organization. Use of non-sugar sweeteners: WHO guideline. Geneva: World Health Organization (2023).
45. Whybrow S, Stubbs R, Johnstone A, O’Reilly L, Fuller Z, Livingstone M, et al. Plausible self-reported dietary intakes in a residential facility are not necessarily reliable. Eur J Clin Nutr. (2016) 70:130–5. doi: 10.1038/ejcn.2015.124
46. Jones L, Ness A, Emmett P. Misreporting of energy intake from food records completed by adolescents: associations with sex, body image, nutrient, and food group intake. Front Nutr. (2021) 8:749007. doi: 10.3389/fnut.2021.749007
47. Gibson R, Charrondiere U, Bell W. Measurement errors in dietary assessment using self-reported 24-hour recalls in low-income countries and strategies for their prevention. Adv Nutr. (2017) 8:980–91. doi: 10.3945/an.117.016980
Keywords: NHANES, popular diet, micronutrient intake, low grain diet, restricted-carbohydrate diet, time-restricted diet
Citation: Basak Tukun A, Rowe S, Johnson LK, Love DC, Belury M and Conrad Z (2023) Micronutrient intake from three popular diet patterns in the United States: modeled replacement of foods highest in added sugar and sodium using the National Health and Nutrition Examination Survey, 2005–2018. Front. Nutr. 10:1217774. doi: 10.3389/fnut.2023.1217774
Received: 05 May 2023; Accepted: 25 September 2023;
Published: 13 October 2023.
Edited by:
Angie Jackson-Morris, RTI International, United StatesReviewed by:
Gemma Bridge, Independent Researcher, Leeds, United KingdomLeah Jamison, RTI International, United States
Copyright © 2023 Basak Tukun, Rowe, Johnson, Love, Belury and Conrad. This is an open-access article distributed under the terms of the Creative Commons Attribution License (CC BY). The use, distribution or reproduction in other forums is permitted, provided the original author(s) and the copyright owner(s) are credited and that the original publication in this journal is cited, in accordance with accepted academic practice. No use, distribution or reproduction is permitted which does not comply with these terms.
*Correspondence: Zach Conrad, enNjb25yYWRAd20uZWR1