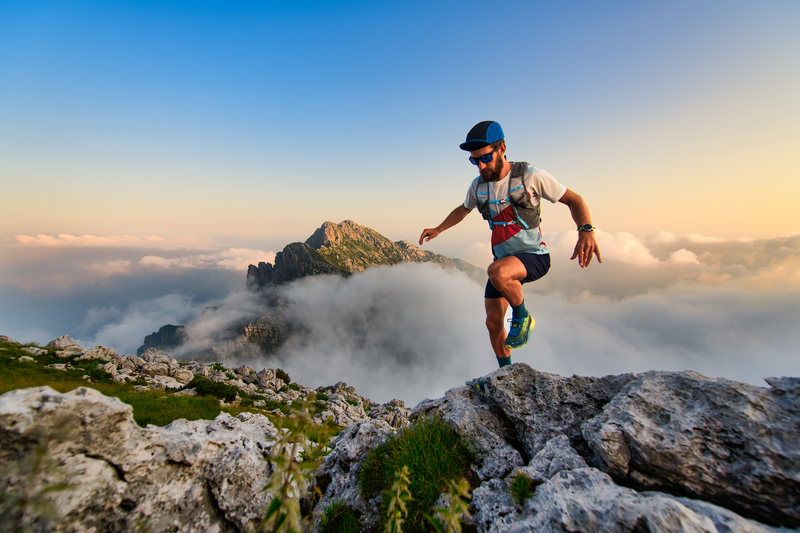
94% of researchers rate our articles as excellent or good
Learn more about the work of our research integrity team to safeguard the quality of each article we publish.
Find out more
SYSTEMATIC REVIEW article
Front. Nutr. , 28 July 2023
Sec. Clinical Nutrition
Volume 10 - 2023 | https://doi.org/10.3389/fnut.2023.1213255
Background: Prognostic nutritional index (PNI) has been identified as a reliable prognostic factor for cancer adjuvant therapy. However, its prognostic value in lung cancer patients receiving immune checkpoint inhibitors (ICIs) remains inconclusive.
Method: A systematic literature review and meta-analysis was performed based on online databases before March 1th 2023. The correlation of PNI with overall survival (OS) or progression-free survival (PFS) was determined using the hazard ratios (HRs) coupled with 95% confidence intervals (CIs). Then, a retrospective cohort enrolling 123 ICI-treated lung cancer patients from two hospitals was utilized for validation and further investigation.
Results: A total of 14 studies enrolling 1,260 lung cancer patients were included in the meta-analysis. The high PNI level was significantly correlated with better OS (HR = 2.56, 95% CI = 1.86–3.54) and PFS (HR = 1.91, 95% CI = 1.53–2.40) of the lung cancer patients. The subgroup analysis confirmed the results except for the PFS in patients receiving anti-PD-1 therapy (HR = 1.51, 95% CI = 0.86–2.65). In the retrospective study, the high PNI level was identified as a favorable factor for OS and PFS not only in the whole cohort but also in the subgroups stratified by non-small cell lung cancer and small cell lung cancer. The high PNI was also correlated with better anti-cancer therapy response and performed better than body mass index and serum albumin level in OS prediction. Finally, we established a novel prognostic nomogram based on PNI and other clinical parameters. The nomogram was found to perform well in predicting the 1-year OS of ICI-treated lung cancer patients.
Conclusion: Both the meta-analysis and retrospective work demonstrate the PNI is a reliable prognostic factor for advanced lung cancer patients receiving ICI-based therapies. Our study further highlights the crucial role of nutrition assessment and intervention in cancer immunotherapy.
Systematic review registration: https://www.crd.york.ac.uk/prospero/, identifier: CRD42023424146.
Lung cancer is the second most frequently diagnosed human malignancy worldwide, ranking the first for cancer mortality among cancers (1). Despite advances in tobacco control and pre-cancer screening, the 5-year relative survival rate for lung cancer is only ~21–23% (2). Targeted therapies based on sequencing have dramatically improved the prognosis of lung cancer patients, however, drug resistance commonly occurs (3). Moreover, the therapeutical strategies for advanced lung cancer without gene mutation were extremely limited. The immune checkpoints inhibitors (ICIs) have shown promise in durable tumor control through taking advantage of host immunity (4). However, in addition to genetic background, various clinical factors were found to affect the actual efficacy of ICIs. For instance, our team previously had proved the correlation between concomitant medications (antibiotics, corticosteroids, proton pump inhibitor and β-blockers) and ICI efficacy (5–8). Therefore, further investigations into the impact of clinical factors on ICI efficacy is urgent and will contribute to more precise therapy decision.
Among lung cancer patients, ~70% are found to suffer from malnutrition or muscle loss, suggesting the crucial role of nutrition assessment during anti-cancer therapy (9). Traditional nutrition related markers such as body mass index (BMI) and albumin (ALB) level have been utilized to predict lung cancer risk or patient survival (10, 11). The prognostic nutritional index (PNI), calculated by serum albumin level and lymphocyte count, has recently been identified as a novel easy-to-perform and standardized tool for prognosis prediction in various cancers (12). In lung cancer, pretreatment PNI has been found to associate with the clinical efficacy of targeted therapy, chemotherapy and radiotherapy (13–15). With regard to ICI-based immunotherapy, in a small cohort of non-small cell lung cancer (NSCLC) patients treated with atezolizumab (n = 24), high PNI level was correlated with prolonged time to treatment failure and overall survival (OS) (16). The pre-treatment PNI was identified as an independent prognostic factor for OS instead of progression-free survival (PFS) in advanced non-small cell lung cancer (NSCLC) patients receiving anti-PD-1 therapy (17). A recent comprehensive study has investigated the prognostic value of PNI and inflammatory indexes in immunotherapy-treated lung cancer patients (18). The univariate analysis in this study demonstrated high PNI level was correlated with prolonged PFS instead of OS, and the multivariate analysis failed to identify it as an independent prognostic factor. Therefore, the prognostic impact of PNI in ICI-treated lung cancer patients remains inconclusive and more clinical validations are essential.
In this study, firstly, a systematic literature review and meta-analysis was performed to objectively assess the prognostic value of PNI in lung cancer patients receiving ICI therapy. Then, a retrospective cohort enrolling 123 lung cancer patients from two hospitals was utilized to confirm the prognostic value of PNI and establish a novel PNI-based prognostic nomogram. This study will not only contribute to the establishment of a non-invasive and low-cost predictive approach for ICI therapy, but also further highlight the crucial role of nutrition assessment and intervention in patient management.
The systematic review and meta-analysis was carried out according to the guidelines of Preferred Reporting Items for Systematic Reviews and meta-Analyses (PRISMA). The literatures related with the prognostic role of PNI in ICI-treated lung cancer patients were searched in PubMed, Embase, Cochrane Library, Web of Science, and Clinicaltrials.gov. The deadline for literature publication date was March 1st 2023. The following key words were used for literature search: prognostic nutritional index, PNI, lung, cancer, tumor, neoplasm, programmed death receptor 1 inhibitor, PD-1 inhibitor, programmed death-ligand 1 inhibitor, PD-L1 inhibitor, immunotherapy, cytotoxic T lymphocyte antigen-4 inhibitor, and CTLA-4 inhibitor. For avoiding missing the eligible studies, the relevant literatures or meeting abstracts published in the supplementary issues were carefully reviewed.
The inclusion criteria for literature search were as follows: (1) lung cancer patients; (2) ICI therapy with or without other standard therapies such as chemotherapy, radiotherapy and targeted therapy; (3) case-control or cohort studies containing the high-PNI and low-PNI group; (4) available hazard ratios (HRs) with 95% confidence intervals (CIs) for OS and/or PFS. The exclusion criteria were as follows: (1) duplicate reports; (2) irrelevant topics; (3) meta-analysis or reviews; (4) non-clinical studies; (5) lack of key patient information.
The flowchart of literature search was shown in Figure 1A. A total of 217 literatures were initially selected from online databases and other sources. Then, the literatures were excluded due to duplications, irrelevant topics, meta-analysis or review, no posted result and repeated study cohorts. Finally, a total of 14 studies were included in the meta-analysis.
Figure 1. Flowchart of the literature search for meta-analysis (A) and patient recruitment in the retrospective study (B).
As shown in Table 1, the following information was extracted from the included studies: author, country, sample size, median age, cut-off value of PNI, anti-cancer treatment and survival data. In case that the HRs of the univariate and multivariate analysis were available, the latter were selected to minimize the impact of confounding factors. In case that the HRs were not provided directly in the literatures, they were estimated using the method described in a previous study (19). The literature quality was assessed using the Newcastle-Ottawa Scale. The literature scored no <6 points was considered to be high-quality.
The lung cancer patients who received ICI therapies at the Department of Oncology, Hefei Cancer Hospital or Affiliated Hospital of Yangzhou University between January 2018 and October 2022 were recruited in the study. The inclusion criteria were as follows: (1) patients pathologically diagnosed as lung cancer; (2) patients receiving ICI-based anti-cancer treatments. The exclusion criteria were as follows: (1) multiple primary tumors; (2) lack of baseline information; (3) incomplete follow-up records; (4) patients receiving insufficient ICI therapy (no more than two cycles); (5) long-term administration of antibiotic and/or corticosteroids that were known to affect the ICI efficacy; (6) suspected hyperprogression. Finally, a total of 123 patients were included for analysis and the recruitment flowchart was shown in Figure 1B. The clinical characteristics of the cohort were shown in Table 4. This study was approved by the ethics committees of Affiliated Hospital of Yangzhou University (No. 2022-YKL11-05) and Hefei Cancer Hospital (No. PJ-KY2022-007). The written informed consents for using patient information in scientific researches were obtained from patients or their authorizers.
The PNI was calculated as follows: PNI = albumin (g/L) + 5 × peripheral serum lymphocyte count (109/L). The cut-off value of PNI in the retrospective clinical study was defined as the median value of PNIs in the meta-analysis (median value = 45.05, Table 1). The BMI was calculated as follows: BMI = weight (Kg)/height (m)2. The cut-off values of BMI and albumin (ALB) level were defined as 25 kg/m2 and 35 g/L respectively according to previous studies (20, 21).
Oncological assessment was performed based on radiological examination and tumor marker detection every two or three cycle. The Response Evaluation Criteria in Solid Tumors (RECIST) 1.1 criteria was utilized to assess the tumor responses to ICI-based therapies, which was classified as complete response (CR), partial response (PR), progressive disease (PD) and stable disease (SD). The OS and PFS were used for outcome assessment, where OS was defined as the time period from the first ICI therapy to the death from any cause and PFS was defined as the time period from the first ICI therapy to disease progression.
The meta-analysis was performed using the Stata SE 14.0 software. The Cochran Q-test and Higgins inconsistency index (I2) were utilized to determine the study heterogeneity. The sensitivity analysis was used to assess the result stability. The Begg's and Egger's test was used to assess the publication bias. In the retrospective clinical study, the statistical analysis was carried out using SPSS 21.0 statistical software and the figures were created using GraphPad Prism 8.0 software. The correlations of PNI with clinical characteristics were determined by the chi-squared test. The survival curves were created using the Kaplan-Meier model and the difference between the survival curves were analyzed using the log rank test. The independent prognostic factors were identified using the univariate and multivariate analysis, and the results were visualized using the R 4.2.1 software. A p-value < 0.05 was considered to be statistically significant.
A total of 14 studies were included in the meta-analysis and the details were shown in Table 1 (16, 18, 22–33). Majority of the patients were diagnosed as NSCLC with their median age ranging from 59.9 to 75 years old. The cut-off values of the PNIs ranged from 40 to 50. All the included studies were retrospective work, among which OS and PFS data were unavailable in one and four studies, respectively. According to the Newcastle-Ottawa Scale, all the included literatures were scored more than six points, suggesting the high quality of our data sources.
As shown in Figure 2A, the pooled meta-analysis demonstrated the PNI was positively associated with the OS of the ICI-treated lung cancer patients (HR = 2.56, 95% CI = 1.86–3.54, I2 = 53.6%, p = 0.011). The similar result was also observed in the PFS of the ICI-treated lung cancer patients (HR = 1.91, 95% CI = 1.53–2.40, I2 = 30.3%, p = 0.167, Figure 2B). The sensitivity analysis revealed both the results could not be significantly affected by any single study (Figures 2C, D). The Begg's test demonstrated no publication bias for the pooled HR values of OS (Figure 2E, p = 0.807) and PFS (Figure 2F, p = 0.128), as subsequently confirmed by the Egg's test (p = 0.754 for OS and p = 0.146 for PFS).
Figure 2. Prognostic significance of prognostic nutritional index (PNI) in the meta-analysis. (A, B) Forest plots of the hazard ratios (HRs) for the correlations of PNI with overall survival (OS) (A) and progression-free survival (PFS) (B). (C, D) Sensitivity analysis of the studies assessing OS (C) and PFS (D). (E, F) Begg's funnel plots for evaluating publication bias of OS (E) and PFS (F).
As shown in Table 2, the positive correlation between PNI and OS was observed in all the subgroups of region (Asia: HR = 2.69, 95% CI = 1.68–4.32, No-Asia: HR = 2.70, 95% CI = 1.93–3.79), age (>65 year old: HR = 2.23, 95% CI = 1.71–2.89; ≤65 year old: HR = 4.75, 95% CI = 2.18–10.32), PNI cut-off value (≤45.05: HR = 2.52, 95% CI = 1.92–3.31; >45.05: HR = 2.81, 95% CI = 1.49–5.30) and therapy type (ICI drug: HR = 2.61, 95% CI = 1.98–3.46; anti-PD-1 drug: HR = 2.60, 95% CI = 1.88–3.59; combined therapy: HR = 2.61, 95% CI = 1.98–3.46). As shown in Table 3, the similar correlation between PNI and PFS was observed in all the subgroups of region (Asia: HR = 1.80, 95% CI = 1.35–2.40; No-Asia: HR = 2.14, 95% CI = 1.41–3.24), age (>65 year old: HR = 1.67, 95% CI = 1.27–2.21, ≤65 year old: HR = 2.35, 95% CI = 1.69–3.28), and PNI cut-off value (≤45.05: HR = 1.71, 95% CI = 1.29–2.26; >45.05: HR = 2.34, 95% CI = 1.67–3.27). In terms of therapy type, the PNI was positively correlated with PFS in the patients receiving ICI (HR = 1.60, 95% CI = 1.02–2.52) or combined therapy (HR = 2.14, 95% CI = 1.67–2.73), instead of those receiving anti-PD-1 drug alone (HR = 1.51, 95% CI = 0.86–2.65).
For validating the results of the meta-analysis, an independent retrospective cohort enrolling 123 lung cancer patients from two hospitals was utilized. As shown in Table 4, no significant correlation was found between the PNI level and gender, smoking status, ECOG PS scores, immuno-checkpoint inhibitors related adverse effects (iRAEs), BMI and ALB, except for age (p = 0.031). The survival analysis demonstrated the patients with high PNI level had a significant better OS and PFS than those with low PNI level (Figures 3A, B). The univariate analysis indicated the PNI level was significantly correlated with the OS and PFS of the lung cancer patients (Figures 3C, D). In addition, the multivariate analysis identified high PNI level as an independent favorable factor affecting both OS and PFS (Figures 3E, F).
Figure 3. Prognostic significance of prognostic nutritional index (PNI) in the validation cohort. (A, B) Kaplan-Meier curves for the association of PNI with overall survival (OS) (A) and progression-free survival (PFS) (B) of the entire validation cohort. (C, D) Univariate analysis for identifying the factors significantly correlated with the OS (C) and PFS (D) of the entire validation cohort. (E, F) Multivariate analysis for identifying the significantly independent factors affecting OS (E) and PFS (F) of the entire validation cohort.
For further investigating the prognostic impact of PNI, the subgroup analysis was carried out according to NSCLC (n = 72) or SCLC (n = 51). As shown in Supplementary Figures 1A, B, the survival analysis demonstrated the positive correlation of the PNI level with both the OS and PFS of the NSCLC patients. The result was then confirmed by the univariate analysis (Supplementary Figures 1C, D). Moreover, the multivariate analysis suggested the high PNI level was an independent favorable factor for both OS and PFS (Supplementary Figures 1E, F). The similar results were also observed in the SCLC subgroup (Supplementary Figures 2A–F). In addition, we noted that the patients with high PNI level had a significant better therapy response than those with low PNI level in both the entire cohort and subgroups stratified by cancer types (Figures 4A–C). The ROC analysis was also used to compare the predictive performance among PNI, BMI and albumin level (Figure 4D). The result demonstrated the PNI was superior to BMI or albumin level in predicting OS (AUC: 71.0% for PNI, 56.5% for BMI and 57.9% for albumin level). Finally, for better utilization of PNI in prognostic prediction, a nomogram was established based on PNI and other clinical parameters including gender, ECOG scores, smoking and BMI, which predicts the 1-year OS of ICI-treated lung cancer patients (Figure 5A). The calibration curve model was constructed to successfully validate the reasonable consistency between the predicted and actual survival (Figure 5B).
Figure 4. Correlation of prognostic nutritional index (PNI) with immune checkpoint inhibitor (ICI) based therapy response. (A) The high PNI level is significantly correlated with better therapy response in the entire validation cohort. (B, C) The high PNI level is significantly correlated with better therapy response in patients with NSCLC (B) and SCLC (C). (D) The receiver operator characteristic (ROC) curve model was used to compare the predictive performance of PNI, Body Mass Index (BMI) and albumin level in the overall survival (OS) of the entire validation cohort.
Figure 5. Construction and validation of a novel prognostic nomogram based on PNI. (A) A nomogram integrating PNI, gender, ECOG, smoking, and BMI was constructed to predict the 1-year survival probability of the entire validation cohort. (B) The calibration curve model was used to validate the accuracy of the nomogram in predicting survival probability.
The ICI-based immunotherapy has significantly improved the overall prognosis of advanced lung cancer patients through its unique sustained tumor control and tolerable adverse effects (34). Since cachexia is common before or during anti-cancer treatment, therefore nutritional assessment and intervention are vital in patient management (35). The PNI, an easily available nutritional marker, has been utilized to predict the clinical outcome of the lung cancer patients in numerous studies. For instance, the low preoperative PNI level was proved to be an independent unfavorable factor affecting the OS and recurrence-free survival of the patients with resectable NSCLC (36). The high pretreatment PNI level was also correlated with better OS and PFS in advanced lung cancer patients receiving anlotinib (37). In extensive-stage SCLC, patients with high PNI levels had a significant better OS and PFS than those with low PNI level (38). However, on the contrary, a recent study found high PNI was associated with worse 1-year OS and therapy response in ALK-positive NSCLC patients receiving crizotinib, suggesting its prognostic impact may depend on gene mutations (39). With regard to ICI-treated lung cancer patients, whether the PNI could function as a reliable prognostic indicator remains inconclusive (16, 18, 25). Therefore, for addressing this issue, we performed a meta-analysis based on current evidences, followed by an independent clinical cohort validation. These efforts will contribute to the clinical utilization of PNI in the precise management of lung cancer patients.
In this meta-analysis, firstly, we found the high PNI level was significantly correlated with better OS and PFS of the lung cancer patients receiving ICI based therapies. The following sensitivity and publication bias analysis confirmed the stability and reliability of the results. This pooled result was in accordance with some included studies. For instance, in NSCLC patients receiving PD-1 inhibitors, PNI ≥ 45 was an independent favorable factor affecting OS and PFS (24). The similar result was also observed in another study suggesting low PNI level also served as an independent predictor of early progression (27). A recent report suggested the nutritional status may be correlated with the proportion and function of immune cells (such as T and memory cells) in the tumor microenvironment, therefore affecting the clinical efficacy of the immunotherapies (40). In hepatocellular carcinoma, nutrition deprivation impaired the anti-cancer function of tumor-infiltrating CD8+T cells, partly explaining why low PNI was correlated with reduced ICI efficacy (41). On the other hand, it should be noted that the PNI was not correlated with patient prognosis or identified as an independent prognostic indicator in several included studies (16, 18, 25, 28, 29). These divergent results may be partly attributed to some uncontrollable factors of retrospective studies such as sample size, patient selection, therapy strategy and follow-up period. For minimizing the potential impact of these factors, the subgroup analysis was performed based on specific characteristics. As result, the positive correlation between PNI level and OS/PFS was significant regardless of region, age and PNI cut-off value. However, with regard to therapy type, we found the PNI level was not significantly correlated with PFS instead of OS in the patients receiving anti-PD-1 therapy. This result was in accordance with only one of the included study demonstrating that pretreatment PNI, BMI and presence of sarcopenia were not identified as independent predictors for PFS in the NSCLC patients receiving pembrolizumab as the first-line treatment (31). Considering the limited included studies, we suggested the correlation between pretreatment PNI level and the clinical efficacy of anti-PD-1 drugs needs to be clarified by more evidences.
For validating the result of the meta-analysis, an independent retrospective cohort from two hospitals was utilized. For the whole cohort, the high PNI level was identified as a favorable independent prognostic factor for both OS and PFS, which was subsequently confirmed by the subgroup analysis of NSCLC and other lung cancer types. In addition, the high PNI level was found to correlate with better therapy response in the lung cancer patients. These evidences collectively supported the speculation that the pretreatment PNI level may predict the efficacy of ICI therapy in the lung cancer patients. On the other hand, both the meta- and retrospective analysis further highlighted the crucial role of immunonutrition intervention in cancer immunotherapy (42). Although the nutritional support in cancer neoadjuvant and adjuvant therapies was widely acknowledged and routinely performed, its benefits in ICI-based therapy remains unclear and needs to be clarified by more clinical trials (43, 44). Previous studies have suggested BMI has the potential to be a prognostic indicator in ICI-treated lung cancer patients. For instance, a multicenter study has showed BMI variation was significantly correlated with OS and PFS in metastatic NSCLC patients receiving first-line pembrolizumab treatment (45). A real-world clinical study demonstrated BMI⩾25 kg/m2 served as an independent favorable factor for the OS of ICI-treated NSCLC patients (46). Consistently, BMI > 25 kg/m2 was significantly correlated with longer PFS in EGFR mutated NSCLC patients receiving ICI therapy (47). The mechanism investigations attributed this correlation partly to the activated leptin signaling in overweight patients, which increases PD-1 expression to induce tumors more responsive to ICI drugs (48). In our study, we found the high BMI level was correlated with better OS and PFS in NSCLC patients, but failed to independently predict the clinical outcome in the multivariate analysis. A recent study has found the association between BMI level and the survival of ICI-treated patients may be affected by some clinical confounders, which partly explains the inconsistent results in our study (49). Moreover, a narrative review has implied the unreliability of BMI as a clinical indicator for lung cancer patients and recommended novel radiological strategies to define obesity (50). The serum albumin is recently identified as a novel non-invasive biomarker for predicting the clinical efficacy of ICI therapy in numerous cancers (21, 51). In this study, the increased serum albumin was correlated with better OS but failed to be an independent predictor in the ICI-treated NSCLC patients. Then, the ROC analysis demonstrated the PNI performed better in stratifying OS than the BMI and serum albumin, suggesting PNI may be the priority for clinicians in predicting the clinical outcome. Finally, considering the increasing utility of nomogram models in cancer management, we integrated the PNI and other factors to establish a novel nomogram for predicting 1-year OS. Using the calibration curves, the predictive performance of the nomogram was well-validated, further supporting the application of PNI in clinical practice.
There are some mechanism investigations to support the role of PNI in predicting ICI efficacy. The PNI value was calculated based on the serum albumin level and lymphocyte count, both of which are closely linked to the clinical efficacy of ICI drugs. The ICI therapy is dependent on IgG-antibody drugs and their catabolism and recycling are regulated by neonatal Fc receptor (FcRn) mediated mechanisms (52). The abundance and efficiency of FcRn could be reflected by the serum albumin level (51). The lymphocyte count may be correlated with the apoptosis of tumor infiltrating lymphocytes, which is crucial for determining the individual response to ICI therapy (53). On the other hand, considering the complexity of tumor microenvironment, the roles of albumin and lymphocyte in cancer immunotherapy are expected to be investigated by more fundamental work based advanced techniques such as single-cell sequencing. In addition, whether increasing pretreatment PNI level (such as albumin supplement or other nutritional interventions) is actually beneficial for the ICI therapy still needs to be clarified by well-designed prospective studies in future.
There are several limitations in our study. First, in the meta-analysis, the therapy strategies varied among the included studies, which may result in study heterogeneity. Meanwhile, the included literatures were relatively limited and therefore the results of the subgroup analysis need further validations. Secondly, a multicenter study demonstrated the PNI level was not an independent prognostic factor for the OS of the patients with SCLC, which is inconsistent with our retrospective study (29). Considering the few relevant published reports and limited sample size of our study, more attention should be paid to the prognostic value of PNI in ICI-treated SCLC patients. Thirdly, since the PNI and other parameters (such as BMI and albumin) may vary with disease progression, we failed to investigate the clinical values of their dynamic changes during the therapy period. Finally, our study mainly focused on the prognostic significance of pretreatment PNI in ICI-treated lung cancer patients, how to develop precise nutritional support strategies and their potential benefits on ICI efficacy should be emphasized in our following work.
In conclusion, our findings collectively demonstrate the PNI level can be utilized as a reliable prognostic indicator for lung cancer patients treated with ICI therapy. These findings not only contribute to the clinical utility of PNI in cancer patient management, but also further highlight the crucial role of nutrition assessment and intervention in ICI therapy. Considering the study limitations, more clinical validations are needed in future. Moreover, relevant mechanism investigations may benefit developing novel intervention strategies to modulate PNI levels before or during ICI therapy.
The raw data supporting the conclusions of this article will be made available by the authors, without undue reservation.
XY and JW wrote the main manuscript text. JM and YW collected the data and prepared all the figures. HQ analyzed the data and revised the manuscript text. XW and MY designed the study and revised the manuscript text. All authors reviewed the manuscript. All authors contributed to the article and approved the submitted version.
This research was financially supported by the China National Natural Science Foundation (No. 81902422), Program of Jiangsu Commission of Health (No. M2020024), and Clinical Translational Foundation of Yangzhou University (No. AHYZUZHXM 202104).
The authors declare that the research was conducted in the absence of any commercial or financial relationships that could be construed as a potential conflict of interest.
All claims expressed in this article are solely those of the authors and do not necessarily represent those of their affiliated organizations, or those of the publisher, the editors and the reviewers. Any product that may be evaluated in this article, or claim that may be made by its manufacturer, is not guaranteed or endorsed by the publisher.
The Supplementary Material for this article can be found online at: https://www.frontiersin.org/articles/10.3389/fnut.2023.1213255/full#supplementary-material
Supplementary Figure 1. Prognostic significance of prognostic nutritional index (PNI) in the patients with non-small cell lung cancer (NSCLC). (A, B) Kaplan-Meier curves for the association of PNI with overall survival (OS) (A) and progression-free survival (PFS) (B) of the NSCLC subgroup. (C, D) Univariate analysis for identifying the factors significantly correlated with the OS (C) and PFS (D) of the NSCLC subgroup. (E, F) Multivariate analysis for identifying the significantly independent factors affecting OS (E) and PFS (F) of the NSCLC subgroup.
Supplementary Figure 2. Prognostic significance of prognostic nutritional index (PNI) in the patients with small cell lung cancer (SCLC). (A, B) Kaplan-Meier curves for the association of PNI with overall survival (OS) (A) and progression-free survival (PFS) (B) of the SCLC subgroup. (C, D) Univariate analysis for identifying the factors significantly correlated with the OS (C) and PFS (D) of the SCLC subgroup. (E, F) Multivariate analysis for identifying the significantly independent factors affecting OS (E) and PFS (F) of the SCLC subgroup.
1. Sung H, Ferlay J, Siegel RL, Laversanne M, Soerjomataram I, Jemal A, et al. Global cancer statistics 2020: GLOBOCAN estimates of incidence and mortality worldwide for 36 cancers in 185 countries. CA Cancer J Clin. (2021) 71:209–49. doi: 10.3322/caac.21660
2. Siegel RL, Miller KD, Wagle NS, Jemal A. Cancer statistics, 2023. CA Cancer J Clin. (2023) 73:17–48. doi: 10.3322/caac.21763
3. Waarts MR, Stonestrom AJ, Park YC, Levine RL. Targeting mutations in cancer. J Clin Invest. (2022) 132:JCI154943. doi: 10.1172/JCI154943
4. Catalano M, Shabani S, Venturini J, Ottanelli C, Voltolini L, Roviello G. Lung cancer immunotherapy: beyond common immune checkpoints inhibitors. Cancers. (2022) 14:246145. doi: 10.3390/cancers14246145
5. Yang M, Wang Y, Yuan M, Tao M, Kong C, Li H, et al. Antibiotic administration shortly before or after immunotherapy initiation is correlated with poor prognosis in solid cancer patients: an up-to-date systematic review and meta-analysis. Int Immunopharmacol. (2020) 88:106876. doi: 10.1016/j.intimp.2020.106876
6. Wang Y, Yang M, Tao M, Liu P, Kong C, Li H, et al. Corticosteroid administration for cancer-related indications is an unfavorable prognostic factor in solid cancer patients receiving immune checkpoint inhibitor treatment. Int Immunopharmacol. (2021) 99:108031. doi: 10.1016/j.intimp.2021.108031
7. Liu C, Guo H, Mao H, Tong J, Yang M, Yan X. An up-to-date investigation into the correlation between proton pump inhibitor use and the clinical efficacy of immune checkpoint inhibitors in advanced solid cancers: a systematic review and meta-analysis. Front Oncol. (2022) 12:753234. doi: 10.3389/fonc.2022.753234
8. Yan X, Liu P, Li D, Hu R, Tao M, Zhu S, et al. Novel evidence for the prognostic impact of beta-blockers in solid cancer patients receiving immune checkpoint inhibitors. Int Immunopharmacol. (2022) 113:109383. doi: 10.1016/j.intimp.2022.109383
9. Kiss N, Curtis A. Current insights in nutrition assessment and intervention for malnutrition or muscle loss in people with lung cancer: a narrative review. Adv Nutr. (2022) 13:2420–32. doi: 10.1093/advances/nmac070
10. Yoon H-S, Shu X-O, Shidal C, Wu J, Blot WJ, Zheng W, et al. Associations of pre-diagnostic serum levels of total bilirubin and albumin with lung cancer risk: results from the southern community cohort study. Front Oncol. (2022) 12:895479. doi: 10.3389/fonc.2022.895479
11. Oswalt C, Liu Y, Pang H, Le-Rademacher J, Wang X, Crawford J. Associations between body mass index, weight loss and overall survival in patients with advanced lung cancer. J Cachexia Sarcopenia Muscle. (2022) 13:2650–60. doi: 10.1002/jcsm.13095
12. Yan L, Nakamura T, Casadei-Gardini A, Bruixola G, Huang YL, Hu ZD. Long-term and short-term prognostic value of the prognostic nutritional index in cancer: a narrative review. Ann Transl Med. (2021) 9:1630. doi: 10.21037/atm-21-4528
13. Chen T, Liang G, Xiang Z, He J, Xu X, Tang M. Prognostic value of prognostic nutritional index and its variations in advanced non-small-cell lung cancer patients treated with anlotinib monotherapy. J Clin Lab Anal. (2022) 36:e24300. doi: 10.1002/jcla.24772
14. Zhang Q, Bao J, Zhu ZY, Jin MX. Prognostic nutritional index as a prognostic factor in lung cancer patients receiving chemotherapy: a systematic review and meta-analysis. Eur Rev Med Pharmacol Sci. (2021) 25:5636–52. doi: 10.26355/eurrev_202109_26783
15. Li L, Wang Y, Yang P, Xu L, Liu S, Zhang S, et al. Correlation of the controlling nutritional status score and the prognostic nutritional index with the prognosis of patients treated with radiotherapy for small-cell lung cancer. Ann Palliat Med. (2021) 10:11635–42. doi: 10.21037/apm-21-2740
16. Matsubara T, Takamori S, Haratake N, Toyozawa R, Miura N, Shimokawa M, et al. The impact of immune-inflammation-nutritional parameters on the prognosis of non-small cell lung cancer patients treated with atezolizumab. J Thorac Dis. (2020) 12:1520–8. doi: 10.21037/jtd.2020.02.27
17. Fang Q, Yu J, Li W, Luo J, Deng Q, Chen B, et al. Prognostic value of inflammatory and nutritional indexes among advanced NSCLC patients receiving PD-1 inhibitor therapy. Clin Exp Pharmacol Physiol. (2023) 50:178–90. doi: 10.1111/1440-1681.13740
18. Zaitsu J, Yamashita Y, Ishikawa A, Saito A, Kagimoto A, Mimura T, et al. Systemic inflammatory score predicts response and prognosis in patients with lung cancer treated with immunotherapy. Anticancer Res. (2021) 41:3673–82. doi: 10.21873/anticanres.15158
19. Altman DG, Bland JM. How to obtain the confidence interval from a P-value. Br Med J. (2011) 343:d2090. doi: 10.1136/bmj.d2090
20. Johannet P, Sawyers A, Qian Y, Kozloff S, Gulati N, Donnelly D, et al. Baseline prognostic nutritional index and changes in pretreatment body mass index associate with immunotherapy response in patients with advanced cancer. J Immunother Cancer. (2020) 8:e001674. doi: 10.1136/jitc-2020-001674
21. Guven DC, Sahin TK, Erul E, Rizzo A, Ricci AD, Aksoy S, et al. The association between albumin levels and survival in patients treated with immune checkpoint inhibitors: a systematic review and meta-analysis. Front Mol Biosci. (2022) 9:1039121. doi: 10.3389/fmolb.2022.1039121
22. Cipriano É, Magalhães H, Estevinho F, Sottomayor C. The prognostic nutritional index and neutrophil-to-lymphocyte ratio as prognostic factors in advanced non-small cell lung cancer patients treated with immunotherapy. Annals of Oncology. (2019) 30:xi6. doi: 10.1093/annonc/mdz447.017
23. Shoji F, Takeoka H, Kozuma Y, Toyokawa G, Yamazaki K, Ichiki M, et al. Pretreatment prognostic nutritional index as a novel biomarker in non-small cell lung cancer patients treated with immune checkpoint inhibitors. Lung Cancer. (2019) 136:45–51. doi: 10.1016/j.lungcan.2019.08.006
24. Peng L, Wang Y, Liu F, Qiu X, Zhang X, Fang C, et al. Peripheral blood markers predictive of outcome and immune-related adverse events in advanced non-small cell lung cancer treated with PD-1 inhibitors. Cancer Immunol Immunother. (2020) 69:1813–22. doi: 10.1007/s00262-020-02585-w
25. Baldessari C, Pecchi A, Marcheselli R, Guaitoli G, Bonacini R, Valoriani F, et al. Body composition and inflammation impact in non-small-cell lung cancer patients treated by first-line immunotherapy. Immunotherapy. (2021) 13:1501–19. doi: 10.2217/imt-2021-0038
26. Cipriano É, Magalhães H, Estevinho F. The impact of inflammatory serum biomarkers in non-small cell lung cancer patients treated with immune checkpoint inhibitors. J Thoracic Oncol. (2021) 16:S255. doi: 10.1016/j.jtho.2021.01.369
27. Liu N, Jiang A, Zheng X, Fu X, Zheng H, Gao H, et al. Prognostic nutritional index identifies risk of early progression and survival outcomes in advanced non-small cell lung cancer patients treated with PD-1 inhibitors. J Cancer. (2021) 12:2960–7. doi: 10.7150/jca.55936
28. Ogura Y, Kataoka N, Kunimatsu Y, Tachibana Y, Sugimoto T, Tani N, et al. Predictors of survival among Japanese patients receiving first-line chemoimmunotherapy for advanced non-small cell lung cancer. Thorac Cancer. (2021) 12:97–105. doi: 10.1111/1759-7714.13720
29. Qi WX, Xiang Y, Zhao S, Chen J. Assessment of systematic inflammatory and nutritional indexes in extensive-stage small-cell lung cancer treated with first-line chemotherapy and atezolizumab. Cancer Immunol Immunother. (2021) 70:3199–206. doi: 10.1007/s00262-021-02926-3
30. Shi Y, Liu X, Liu J, Zhang D, Liu X, Yue Y, et al. Correlations between peripheral blood biomarkers and clinical outcomes in advanced non-small cell lung cancer patients who received immunotherapy-based treatments. Transl Lung Cancer Res. (2021) 10:4477–93. doi: 10.21037/tlcr-21-710
31. Shijubou N, Sumi T, Yamada Y, Nakata H, Mori Y, Chiba H. Immunological and nutritional predictive factors in patients receiving pembrolizumab for the first-line treatment of non-small cell lung cancer. J Cancer Res Clin Oncol. (2022) 148:1893–901. doi: 10.1007/s00432-022-03941-2
32. Stares M, Ding TE, Stratton C, Thomson F, Baxter M, Cagney H, et al. Biomarkers of systemic inflammation predict survival with first-line immune checkpoint inhibitors in non-small-cell lung cancer. ESMO Open. (2022) 7:100445. doi: 10.1016/j.esmoop.2022.100445
33. Tanaka S, Uchino J, Yokoi T, Kijima T, Goto Y, Suga Y, et al. Prognostic nutritional index and lung immune prognostic index as prognostic predictors for combination therapies of immune checkpoint inhibitors and cytotoxic anticancer chemotherapy for patients with advanced non-small cell lung cancer. Diagnostics. (2022) 12:423. doi: 10.3390/diagnostics12020423
34. Lahiri A, Maji A, Potdar PD, Singh N, Parikh P, Bisht B, et al. Lung cancer immunotherapy: progress, pitfalls, and promises. Mol Cancer. (2023) 22:40. doi: 10.1186/s12943-023-01740-y
35. Zarogoulidis P, Kosmidis C, Kesisoglou I, Tsakiridis K, Hohenforst-Schmidt W, Huang H, et al. Nutrition and NSCLC; should we administer food supplements? Curr Pharm Des. (2021) 27:3602–8. doi: 10.2174/1381612827999210111193133
36. Takahashi M, Tokumasu H, Ota S, Okada H, Aoyama A. Clinical significance of the preoperative prognostic nutritional index on age/comorbidity burdens in patients with resectable non-small cell lung cancer. Surg Today. (2023) 53:681–91. doi: 10.1007/s00595-023-02650-8
37. Fan B, Tan X, Lou Y, Zheng Y, Zhang L, Wu X. Prognostic factors of patients with advanced lung cancer treated with anlotinib: a retrospective cohort study. J Int Med Res. (2021) 49:3000605211046173. doi: 10.1177/03000605211046173
38. Yilmaz H, Yersal Ö. Prognostic significance of novel inflammatory markers in extensive-stage small-cell lung cancer. J Cancer Res Ther. (2022) 18:691–6. doi: 10.4103/jcrt.jcrt_1937_21
39. Olmez OF, Bilici A, Gursoy P, Cubukcu E, Sakin A, Korkmaz T, et al. Impact of systemic inflammatory markers in patients with ALK-positive non-small cell lung cancer treated with crizotinib. Pulmonology. (2022) 2022:6. doi: 10.1016/j.pulmoe.2022.11.006
40. Pilotto S, Agustoni F, Morelli AM, Lobascio F, Cereda E, Bironzo P, et al. Nutritional support in lung cancer: time to combine immunonutrition with immunotherapy? Nutrition. (2022) 98:111637. doi: 10.1016/j.nut.2022.111637
41. Zhang CY, Liu S, Yang M. Nutrition deprivation affects the cytotoxic effect of CD8 T cells in hepatocellular carcinoma. World J Gastrointest Oncol. (2022) 14:1887–91. doi: 10.4251/wjgo.v14.i9.1887
42. Cuyàs E, Verdura S, Martin-Castillo B, Alarcón T, Lupu R, Bosch-Barrera J, et al. Tumor cell-intrinsic immunometabolism and precision nutrition in cancer immunotherapy. Cancers. (2020) 12:1757. doi: 10.3390/cancers12071757
43. Lovey J, Molnar A, Banky B. Long-term nutrition in patients candidate to neoadjuvant and adjuvant treatments. Eur J Surg Oncol. (2023) 2:7. doi: 10.1016/j.ejso.2023.02.007
44. Mulazzani GEG, Corti F, Della Valle S, Di Bartolomeo M. Nutritional support indications in gastroesophageal cancer patients: from perioperative to palliative systemic therapy. A comprehensive review of the last decade. Nutrients. (2021) 13:2766. doi: 10.3390/nu13082766
45. Cortellini A, Ricciuti B, Tiseo M, Bria E, Banna GL, Aerts JG, et al. Baseline BMI and BMI variation during first line pembrolizumab in NSCLC patients with a PD-L1 expression ≥ 50%: a multicenter study with external validation. J Immunother Cancer. (2020) 8:e001403. doi: 10.1007/s00262-020-02613-9
46. Zhang S, Pease DF, Kulkarni AA, Kumar M, Shanley RM, Xu B, et al. Real-world outcomes and clinical predictors of immune checkpoint inhibitor monotherapy in advanced lung cancer. Clin Med Insights Oncol. (2021) 15:1179554921100. doi: 10.1177/11795549211004489
47. Bai M, Wang W, Gao X, Wu L, Jin P, Wu H, et al. Efficacy of immune checkpoint inhibitors in patients with EGFR mutated NSCLC and potential risk factors associated with prognosis: a single institution experience. Front Immunol. (2022) 13:83. doi: 10.3389/fimmu.2022.832419
48. Gelibter A, Occhipinti M, Pisegna S, Cortellini A, Cortesi E, Marchetti P. Status of correlation between BMI and response to immunocheck-point inhibitor in advanced non-small-cell lung cancer. Lung Cancer Manag. (2020) 9:Lmt26. doi: 10.2217/lmt-2019-0016
49. Antoun S, Lanoy E, Ammari S, Farhane S, Martin L, Robert C, et al. Protective effect of obesity on survival in cancers treated with immunotherapy vanishes when controlling for type of cancer, weight loss and reduced skeletal muscle. Eur J Cancer. (2023) 178:49–59. doi: 10.1016/j.ejca.2022.10.013
50. Vedire Y, Kalvapudi S, Yendamuri S. Obesity and lung cancer-a narrative review. J Thorac Dis. (2023) 15:2806–2823. doi: 10.21037/jtd-22-1835
51. Zheng M. Serum albumin: a pharmacokinetic marker for optimizing treatment outcome of immune checkpoint blockade. J Immunother Cancer. (2022) 10:e005670. doi: 10.1136/jitc-2022-005670
52. Chaudhury C, Mehnaz S, Robinson JM, Hayton WL, Pearl DK, Roopenian DC, et al. The major histocompatibility complex-related Fc receptor for IgG (FcRn) binds albumin and prolongs its lifespan. J Exp Med. (2003) 197:315–22. doi: 10.1084/jem.20021829
Keywords: lung cancer, prognostic nutritional index, immune checkpoint inhibitors, prognosis, nomogram
Citation: Yan X, Wang J, Mao J, Wang Y, Wang X, Yang M and Qiao H (2023) Identification of prognostic nutritional index as a reliable prognostic indicator for advanced lung cancer patients receiving immune checkpoint inhibitors. Front. Nutr. 10:1213255. doi: 10.3389/fnut.2023.1213255
Received: 27 April 2023; Accepted: 12 July 2023;
Published: 28 July 2023.
Edited by:
Clelia Madeddu, University of Cagliari, ItalyReviewed by:
Yaxu Wang, Chongqing Medical University, ChinaCopyright © 2023 Yan, Wang, Mao, Wang, Wang, Yang and Qiao. This is an open-access article distributed under the terms of the Creative Commons Attribution License (CC BY). The use, distribution or reproduction in other forums is permitted, provided the original author(s) and the copyright owner(s) are credited and that the original publication in this journal is cited, in accordance with accepted academic practice. No use, distribution or reproduction is permitted which does not comply with these terms.
*Correspondence: Xiangjun Wang, d3hqdW5fMDMyNkAxNjMuY29t; Mengxue Yang, eW14XzY3OEAxNjMuY29t; Hong Qiao, MTU5NTI1MzUyNTVAMTYzLmNvbQ==
†These authors have contributed equally to this work
Disclaimer: All claims expressed in this article are solely those of the authors and do not necessarily represent those of their affiliated organizations, or those of the publisher, the editors and the reviewers. Any product that may be evaluated in this article or claim that may be made by its manufacturer is not guaranteed or endorsed by the publisher.
Research integrity at Frontiers
Learn more about the work of our research integrity team to safeguard the quality of each article we publish.