- Department of Cadre Ward, The First Hospital of Jilin University, Changchun, Jilin, China
Background: Malnutrition is an often unrecognized problem, but it is common in older patients and leads to adverse outcomes.
Aims: The purpose of this study is to analyze the prevalence of the risk of undernutrition in elderly patients and the correlation between CGA and nutritional status, and to determine the nutritional status of elderly patients.
Methods: This is a real-world cross-sectional study of continuously enrolled elderly patients aged 65 years or older with a complete CGA database. CGA inventory was prepared by compiling and screening general information, body composition and blood biochemical results. MNA was also conducted for each elderly patient to screen for malnutrition. A multivariable logistic regression analysis was used to determine the association between the CGA and nutritional assessment.
Result: The average age of the 211 selected elderly patients (160 men and 51 women) was 79.60 ± 9.24 years, and their ages ranged from 65 to 96 years. After controlling for confounders, patients with a history of PUD (OR = 2.353, p = 0.044), increased ADLs & IADLs scores (OR = 1.051, p = 0.042) or GDS scores (OR = 6.078, p < 0.001) may increase the incidence of the risk of undernutrition respectively, while an increase in BMI (OR = 0.858, p = 0.032) may lower the incidence of malnutrition risk. In addition, increased ADLs & IADLs scores (OR = 1.096, p = 0.002) or GDS scores (OR = 11.228, p < 0.001) may increase the incidence of undernutrition. However, increased MMSE (OR = 0.705, p < 0.001), BMI (OR = 0.762, p = 0.034), UAC (OR = 0.765, p = 0.048) and CC (OR = 0.721, p = 0.003) may decrease the incidence of undernutrition, respectively.
Conclusion: The study found that the prevalence of risk of undernutrition in elderly patients was the highest. Risk of undernutrition was independently associated with peptic ulcer disease, ADLs & IADLs, GDS and BMI. However, we found that when the nutritional status reached the level of undernutrition, it was related to more factors, including ADLs & IADLs, MMSE, GDS, BMI, UAC and CC. Determining the level of malnutrition through CGA may help to prevent and intervene malnutrition as early as possible.
1 Introduction
Malnutrition is a chronic and often imperceptible process and identifying it at an early stage is crucial to reduce adverse outcomes (1). There are many related factors to malnutrition during the aging process, which may seriously affect the health status of the elderly (2). In addition, as people grow older, they are more likely to suffer from chronic diseases such as heart disease, cancer and diabetes, which will increase the risk of cognitive decline, weakness and disability. As is well known, improving nutrition will bring tangible benefits to the elderly, and many age-related diseases and conditions can be prevented, adjusted or improved by increasing nutritional levels (3). However, malnutrition in elderly patients has often been overlooked so far, so there is an urgent need to identify methods for malnutrition in order to prevent and treat it in a timely manner.
Comprehensive Geriatric Assessment (CGA) is a multi-dimensional and multidisciplinary diagnosis and treatment process, which assesses the physical diseases, psychological and functional abilities of the elderly; identifies high-risk patients; and further guides the disease prevention, treatment and follow-up (4, 5). It may also help to formulate long-term diagnosis and treatment plans (6). One study on health workers has shown that the CGA is related to nutritional indicators (7). In clinical practice, it has been found that elderly patients are willing to accept CGA, which may help identify the nutritional status of the elderly in clinical practice.
In this study, we hypothesized that CGA was related to nutritional status. If the hypothesis holds, we can use CGA to identify the different nutritional status of elderly patients, which can lead to early prevention and treatment.
2 Methods
2.1 Participants
This is a real-world cross-sectional study. We conducted an experimental study on consecutive outpatients and inpatients in the cadre ward department of the First Hospital of Jilin University from June 2020 to June 2021. The inclusion criteria were the elderly patients who had a complete CGA database and were 65 years old or older. The exclusion criteria were patients younger than 65 years old who did not participate in CGA or whose data were incomplete. The study was in line with the Helsinki Declaration and was approved by the Ethics Committee of the First Hospital of Jilin University (2021–478).
2.2 The process of CGA
The personnel conducting CGA receive specialized training in scale evaluation and instrument inspection (8). The detailed CGA assessment tools vary depending on institutions, medical environments and needs, but the definition of CGA is consistent, including the assessment of needs in multiple fields and the development of treatment plans (9). The measurement process of this study was personally tested by the same physician from the Cadre Ward Department of the First Hospital of Jilin University. Firstly, routine examinations should be conducted on enrolled patients. The collection of general information by face-to-face inquiry includes gender, age, educational background, smoking history, drinking history, sports and chronic diseases, such as cardiovascular disease (CVD), diabetes and peptic ulcer disease (PUD). The evaluation of the scale includes quality of daily life, cognitive test and geriatric depression scale. The evaluator dictates the survey content and records the patient’s answers. The next step for human body composition testing includes body mass index (BMI), upper arm circumference (UAC), calf circumference (CC), body fat percentage, and visceral fat area. Collect fasting venous blood from all patients the morning after admission and send them to the Laboratory Department of the First Hospital of Jilin University. After the results are reported, collect data on hemoglobin, albumin, prealbumin, triglycerides and low-density lipoprotein cholesterol (LDL-C). The experimental personnel collect and organize the data, and a total of 211 valid and complete data were obtained.
2.3 Detailed content of CGA
2.3.1 General information
Collect personal information of patients, including gender, age, educational background, smoking history, drinking history, exercise (It refers to the patient walks for a total of more than 1 h per day and more than 5 days per week.) and chronic diseases (It refers to non-communicable chronic diseases, most commonly diabetes, obesity, metabolic syndrome, chronic kidney disease, cardiovascular disease, cancer and chronic respiratory diseases) (10). The patient has been diagnosed with the disease in the past and is in the process of disease monitoring and treatment.
2.3.2 Quality of daily living
The living ability of elderly people was measured with the activities of daily living and instrumental activities of daily living (ADLs & IADLs). The ADLs & IADLs are important to older adults (11). ADLs includes physical care activities. These activities are essential for living in the social world. They enable people to obtain basic survival and well-being, such as eating, dressing, bathing and toileting; IADLs includes activities of daily life in families and communities, which usually are more complex than ADLs, such as making phone calls, taking medicine, financial management, purchasing groceries and housekeeping services (12). When the total score is greater than 16, it indicates that the patient has functional decline to varying degrees.
2.3.3 Cognitive tests
Cognitive dysfunction was assessed using the Mini Mental State Examination (MMSE), a widely used cognitive test (13). The total MMSE score ranges from 0 to 30 (14), which consists of 30 items, including orientation tests, short-term memory and recall tests, attention and computing ability tests, naming tests, oral command tests, judgment tests and repeated pentagons tests (15). Cognitive impairment is evaluated based on differences in educational levels. A score of less than 18 was used to define cognitive impairment in participants without any formal education, less than 21 for those with six or fewer years of education, and less than 25 for those with more than six years of education (16, 17). All patients in this article have more than 6 years of education, the cut-off point for MMSE is 24, and a score of 24 or lower indicates cognitive impairment, which has been widely used as a screening tool in epidemiological research (17, 18).
2.3.4 Geriatric depression
Depression was determined using the 30-item Geriatric Depression Scale (GDS) (19), which is a standardized self-reported questionnaire consisting of 30 dichotomous questions. Sum of the 30 items produced a score ranging from 0 to 30, with greater values indicating increased severity. A GDS score of 11 or above is considered depression (20, 21). One study on depression in elderly people in Amsterdam showed that the GDS-30 scale with a critical value of 11 is the most effective in screening for depression in terms of sensitivity and specificity for screening for severe and mild depression (22). And other studies have shown this cutoff has been shown to have 100% sensitivity and 84% specificity with other depression criteria (23).
2.3.5 Body composition
Height and weight were measured according to clinical standards. BMI is calculated by dividing weight (kg) by height (m2).
Measure the UAC at the level of the thickest part of the biceps muscle when the upper arm drops naturally (accurate to 0.1 cm). Participants stood with their feet shoulder-width apart and measured the CC around the most prominent part of the gastrocnemius muscle (accurate to 0.1 cm). During the experiment, we tried our best to appease the patients and encourage them to cooperate with our measurements. We strictly followed the experimental steps and methods when measuring height, weight, UAC, and CC, proficiently used measuring instruments, accurately read, and conducted three measurements to minimize possible measurement errors and ensure the accuracy and reliability of the measurement results.
Body fat percentage and visceral fat area were measured by bioelectrical impedance analysis (BIA) using the Inbody S10 (Biospace, Seoul, Korea) (24).
2.3.6 Blood biochemical results
Blood biochemical indicators such as hemoglobin, albumin, prealbumin, triglycerides, and LDL-C were obtained from the test results in electronic medical record.
2.4 Nutritional assessment
Nutritional status was assessed using the Mini Nutritional Assessment (MNA) questionnaire, which included 18 questions related to important factors of nutritional status, including food intake, weight loss, mobility, whether there is acute stress or disease, neurological problems, drug intake, BMI, UAC and CC. The highest score is 30 points. A score of 24 or more indicates a good nutritional status, a score between 23.5 and 17 indicates a risk of undernutrition, and a score below 17 indicates undernutrition (25). According to the MNA score results, the experiment was divided into three groups: normal nutrition group for scores ≥24, risk of undernutrition group for scores 17–23.5, and undernutrition group for scores <17.
2.5 Data analysis
The SPSS/WIN 23.0 software (IBM Corp., Armonk, NY, United States) and GraphPad Prism 8 (GraphPad Prism Software Inc., San Diego, CA, United States) were used for the statistical analysis. The Kolmogorov–Smirnov test was used to test the normality of the continuous variables. According to the distribution characteristics of data, continuous variables are expressed as mean ± standard deviation (SD) or median (interquartile range, IQR), and Student’s t-test or Mann–Whitney U-test was used to test the difference between the two groups. The categorical variables were expressed as absolute values and percentages, and chi-squared test were used to test the differences between the two groups. A multivariable logistic regression analysis was used to determine the correlation between PUD, ADLs & IADLs, MMSE, GDS, BMI, UAC, CC and MNA. Variables with p < 0.05 were selected for the multivariate analysis. The values of p < 0.05 were considered statistically significant.
3 Results
3.1 Baseline data of general characteristics, chronic diseases, ADLs & IADLs, MMSE and GDS
The average age of the 211 selected elderly patients (160 men and 51 women) was 79.60 ± 9.24 years, and their ages ranged from 65 to 96 years. As shown in Table 1, among the elderly patients, the incidence rate of undernutrition risk is the highest (43.60%), and older patients have poorer nutritional status. Among them, the median age of normal nutritional patients (n = 85, 40.28%) was 76.00, the median age of patients at risk of undernutrition (n = 92, 43.60%) was 81.50 and the median age of undernutritional patients (n = 34, 16.11%) was 90.00. Compared to patients with normal nutrition, the number of malnourished patients who exercise regularly is smaller. Moreover, as the nutritional status worsens, the ability of daily living of the elderly gradually declined, and the prevalence of related chronic diseases also gradually increased, especially CVD, PUD, depression and cognitive disorders (p < 0.05).
3.2 Baseline data of the anthropometric parameters and the blood biochemical indicators
As shown in Table 2, with the gradual decrease of BMI, UAC and CC, the nutritional status gradually deteriorates. Compared with elderly patients with normal nutrition, patients at risk of undernutrition and undernutrition have lower hemoglobin, albumin, prealbumin and triglyceride (p < 0.05).
3.3 Multivariable logistic regression analysis for risk of undernutrition.
As shown in Table 3 and Figure 1, we analyzed the relationship between factors in CGA and risk of undernutrition. In model 1, after adjusting the age and the type of chronic diseases, patients with PUD were 2.353 times more likely to be at risk of undernutrition than those without PUD (95%CI: 1.024–5.408, p = 0.044). The probability of developing the risk of undernutrition increases by 5.1% for each 1- point increase in ADLs & IADLs (95%CI: 1.002–1.102, p = 0.042). In addition, depressed patients are 6.078 times more likely to suffer from the risk of undernutrition than those without depression (95%CI: 2.408–15.338, p < 0.001); In model 2, after adjusting for age, types of chronic diseases, PUD, ADLs & IADLs and GDS, for every 1 point increase in BMI, the elderly patients were 14.2% less likely to be at risk of undernutrition (95%CI: 0.746–0.987, p = 0.032). The area under the receiver operating characteristic of comprehensive PUD, ADL, GDS and BMI is 0.756.
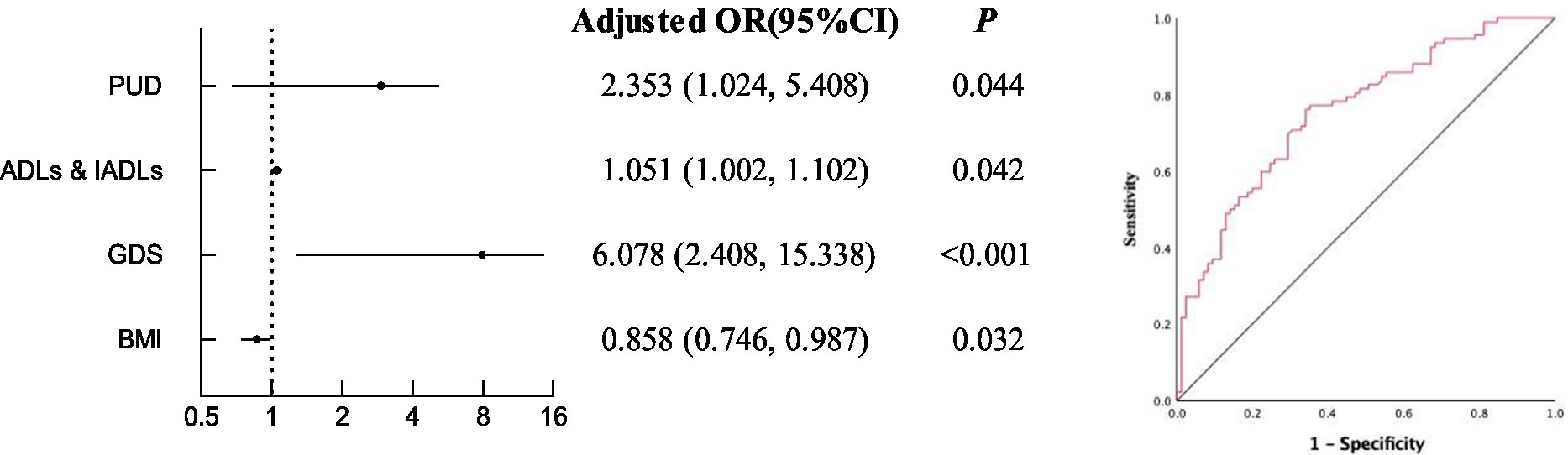
Figure 1. Multivariable logistic regression analysis for risk of undernutrition and the receiver operating characteristic of comprehensive PUD, ADL, GDS and BMI.
3.4 Multivariable logistic regression analysis for undernutrition
As shown in Table 4 and Figure 2, we analyzed the relationship between factors in CGA and undernutrition. In model 1, after adjusting the age, exercise and the type of chronic diseases, the probability of undernutrition increases by 9.6% for each 1-point increase in ADLs & IADLs (95%CI: 1.035–1.161, p = 0.002), the probability of undernutrition decreased by 29.5% for each 1 value increase in MMSE (95%CI: 0.592–0.839, p < 0.001). In addition, depressed patients are 11.228 times more likely to suffer from undernutrition than those without depression (95%CI: 3.585–35.165, p < 0.001); In model 2, after adjusting for age, exercise, types of chronic diseases, CVD, PUD, ADLs & IADLs, MMSE and GDS, for each 1-point increase in BMI, the probability of undernutrition decreased by 23.8% (95%CI: 0.593–0.979, p = 0.034), for each 1-point increase in UAC, the probability of undernutrition decreased by 23.5% (95%CI: 0.587–0.998, p = 0.048), or for each 1-point increase in CC, the probability of undernutrition decreased by 27.9% (95%CI: 0.582–0.894, p = 0.003). The area under the receiver operating characteristic of comprehensive ADLs & IADLs, MMSE, GDS, BMI, UAC and CC is 0.753.
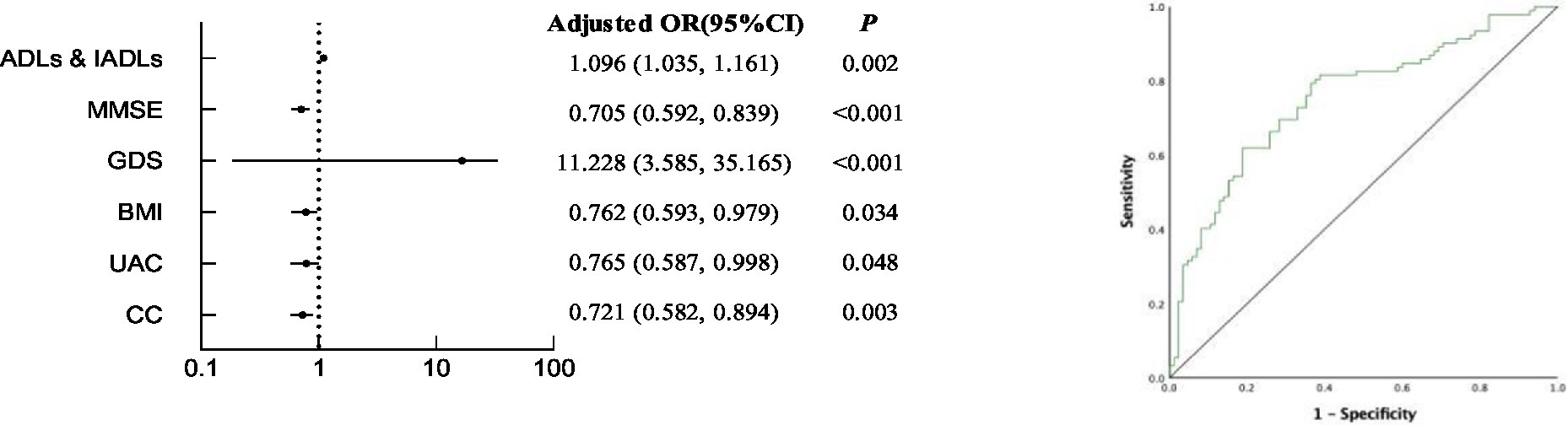
Figure 2. Multivariable logistic regression analysis for undernutrition and receiver operating characteristic of comprehensive ADLs & IADLs, MMSE, GDS, BMI, UAC and CC.
4 Discussion
Our study is the first to report the identification of different nutrition in elderly patients by CGA in the real world. Our results showed that many parameters of CGA are related to nutrition. In analyzing this relationship, we found that PUD, ADLs & IADLs, GDS, BMI, MMSE, UAC, CC were independently associated with nutritional status in elderly patients. The area under the ROC curve of the combined indicators under different nutritional states is greater than 0.7, therefore, the combination of corresponding indicators has certain diagnostic value for different nutritional states.
Scholars at home and abroad have been keen on the study of malnutrition and have found many factors related to malnutrition. Previous studies have demonstrated that PUD is associated with malnutrition (26). People with peptic ulcers such as duodenal ulcers often experience hunger or nocturnal abdominal pain, while people with gastric ulcers often experience postprandial abdominal pain, nausea, vomiting and weight loss (27).
Several large sample studies on the elderly have shown that depression is also related to malnutrition and these two diseases are common among the elderly in China (28, 29). And depression is a common mental illness characterized by decreased appetite, self-care, apathy and physical weakness. These characteristics may well explain the relationship between malnutrition and depression (30). In addition, the ability of daily living in elderly patients gradually decreases with age, and it has been reported that poor ability of daily living is associated with malnutrition (31). Others have found a link between nutritional status and cognition (32), the decline of cognitive function may affect the nutritional status, leading to a reduction in nutrient intake, and the resulting weight loss and malnutrition, which in turn may worsen cognitive function (33). Another important aspect is that there are many important indicators involved in nutritional assessment, such as BMI, UAC and CC (34, 35), which are valuable information for common nutrition-related diseases (36). Therefore, factors related to chronic diseases and body composition can affect the nutritional status of patients, and malnutrition can in turn affect the course of disease (37).
As age advances, the elderly are more likely to suffer from malnutrition or chronic energy deficiency, due to the deterioration of various physical functions, economic dependence and social isolation. Several small studies have demonstrated that the prevalence of malnutrition in the elderly ranges from 14 to 52% (38–40). It is well known that malnutrition is an underrecognized and undertreated problem throughout the health care system. Clinically, hospital malnutrition may lead to an increase in the number and severity of disease complications by increasing morbidity and mortality (41). The objective of this study was to analyze the factors of undernutrition and risk of undernutrition in order to identify different levels of nutrition. Through the identification of different nutritional conditions, early prevention and appropriate intervention can be carried out.
Previous studies have also analyzed the correlation between CGA parameters and nutrition. One of the cross-sectional studies of health professionals showed that CGA was associated with nutrition-related factors such as sarcopenia, sarcopenic dysphagia, cachexia. The appropriate care plan, including rehabilitation and nutrition management are provided according to the patient’s condition (7). Another study of older adults showed that age, drinking, chronic diseases, depression, BMI, ADLs & IADLs, number of recent falls, cognitive impairment, insomnia, low hemoglobin and albumin levels were independently associated with malnutrition. CGA can provide detailed information of elderly patients and is of great value for nutritional assessment (42). However, different from previous studies, this study was a real-world cross-sectional study that included elderly patients in outpatients and inpatients over a continuous period of time. In this study, we not only analyzed the general characteristics, scales and blood biochemical indicators of patients, but also included body composition factors such as UAC, CC, body fat percentage and visceral fat area. More importantly, this study specifically analyzed the different factors in CGA related to different levels of nutrition, so as to more specifically identify the current level of malnutrition in addition to more targeted prevention and treatment. This is a real- world cross-sectional study aimed at understanding the real living conditions of elderly patients and examining the relevance of geriatric diseases to the field of geriatric assessment, contributing to the prevention of many diseases and reducing the growing disease burden of the elderly.
There are some limitations to consider in our study. Firstly, since the patients included in our study are all patients in the cadre ward of the First Hospital of Jilin University, and these patients are groups with relatively high educational level, socioeconomic status and healthcare treatment level, and there is no significant difference. Considering that the impact on the experiment is small, there is not too much elaboration on this part of mixed factors in the experiment. In the future experiment, multi center, large sample research is very valuable, and excluding the possible confounding factors mentioned above may have more generalizability for the experimental results. Secondly, because this experiment is a small sample cross-sectional study, the results can only show the correlation between various factors and nutrition, which limits the ability to infer the causal relationship between malnutrition and observed results. Therefore, large sample cohort study in the future is very worthwhile and valuable and is very meaningful for malnutrition identification. Thirdly, based on clinical experience and literature review, it has been learned that medication use in the elderly may have an impact on their nutritional status. However, in this experiment, we did not include multiple medication use in the elderly. It is necessary to enrich the CGA database in future studies, such as including factors related to multiple medication use. Our CGA only included a small number of factors. In the future, it is necessary to further enrich the CGA content to have a more comprehensive understanding of nutrition-related factors in elderly patients.
5 Conclusion
The study found that the prevalence of risk of undernutrition in elderly patients was the highest. Risk of undernutrition was independently associated with peptic ulcer disease, ADLs & IADLs, GDS and BMI. However, we found that when the nutritional status reached the level of undernutrition, it was related to more factors, including ADLs & IADLs, MMSE, GDS, BMI, UAC and CC. Determining the level of malnutrition through CGA may help to prevent and intervene malnutrition as early as possible. And we found that the combination of corresponding indicators has significant diagnostic value for different nutritional states. It is suggested that the elderly should take appropriate exercise according to their physical conditions, improve their quality of life, improve their mood, eat high protein, low salt and low-fat food and regularly go to community hospital for medical treatment.
Data availability statement
The original contributions presented in the study are included in the article/supplementary material, further inquiries can be directed to the corresponding author.
Ethics statement
The studies involving humans were approved by the Ethics Committee of the First Hospital of Jilin University. The studies were conducted in accordance with the local legislation and institutional requirements. The participants provided their written informed consent to participate in this study.
Author contributions
YJ, JL, and XL drafted the manuscript. YJ and XL revised the manuscript. KZ, DY, and MC drew the figures. XL, DY, and HJ were responsible for the data acquisition. YMJ and JYL performed the data analysis. YJ, HJ, and MC performed the statistical analyses. YJ, KZ and JL conceived of and designed the study. All authors contributed to the article and approved the submitted version.
Funding
The author(s) declare financial support was received for the research, authorship, and/or publication of this article. This research was supported by the Development and Reform Commission in Jilin Province of China (2022C043-7) and Medical and Health Talent Special Project in Jilin Province of China (JLSWSRCZX2023-31) to JL.
Conflict of interest
The authors declare that the research was conducted in the absence of any commercial or financial relationships that could be construed as a potential conflict of interest.
Publisher’s note
All claims expressed in this article are solely those of the authors and do not necessarily represent those of their affiliated organizations, or those of the publisher, the editors and the reviewers. Any product that may be evaluated in this article, or claim that may be made by its manufacturer, is not guaranteed or endorsed by the publisher.
References
1. Lahmann, NA, Tannen, A, and Suhr, R. Underweight and malnutrition in home care: a multicenter study. Clin Nutr. (2016) 35:1140–6. doi: 10.1016/j.clnu.2015.09.008
2. Volkert, D, Beck, AM, Cederholm, T, Cereda, E, Cruz-Jentoft, A, Goisser, S, et al. Management of Malnutrition in older patients-current approaches, evidence and open questions. J Clin Med. (2019) 8:974. doi: 10.3390/jcm8070974
3. Shlisky, J, Bloom, DE, Beaudreault, AR, Tucker, KL, Keller, HH, Freund-Levi, Y, et al. Nutritional considerations for healthy aging and reduction in age-related chronic disease. Adv Nutr. (2017) 8:17–26. doi: 10.3945/an.116.013474
4. Rubenstein, LZ, Goodwin, M, Hadley, E, Patten, SK, Rempusheski, VF, Reuben, D, et al. Working group recommendations: targeting criteria for geriatric evaluation and management research. J Am Geriatr Soc. (1991) 39:37S–41S.
5. Fagard, K, Casaer, J, Wolthuis, A, Flamaing, J, Milisen, K, Lobelle, JP, et al. Value of geriatric screening and assessment in predicting postoperative complications in patients older than 70 years undergoing surgery for colorectal cancer. J Geriatr Oncol. (2017) 8:320–7. doi: 10.1016/j.jgo.2017.07.008
6. Rubenstein, LZ, Stuck, AE, Siu, AL, and Wieland, D. Impacts of geriatric evaluation and management programs on defined outcomes: overview of the evidence. J Am Geriatr Soc. (1991) 39:8S–16S. doi: 10.1111/j.1532-5415.1991.tb05927.x
7. Ueshima, J, Maeda, K, Wakabayashi, H, Nishioka, S, Nakahara, S, and Kokura, Y. Comprehensive geriatric assessment and nutrition-related assessment: a cross-sectional survey for health professionals. Geriatrics (Basel). (2019) 4:23. doi: 10.3390/geriatrics4010023
8. Kong, C, Zhang, Y, Wang, C, Wang, P, Li, X, Wang, W, et al. Comprehensive geriatric assessment for older orthopedic patients and analysis of risk factors for postoperative complications. BMC Geriatr. (2022) 22:644. doi: 10.1186/s12877-022-03328-5
9. Scheepers, ERM, Vondeling, AM, Thielen, N, van der Griend, R, Stauder, R, and Hamaker, ME. Geriatric assessment in older patients with a hematologic malignancy: a systematic review. Haematologica. (2020) 105:1484–93. doi: 10.3324/haematol.2019.245803
10. Yang, Y, Sun, X, Wang, J, Yang, C, and Zhang, L. Incidence rates of four major non-communicable chronic diseases in the Chinese adult population from 2007 to 2016: a study based on a National Commercial Claims Database. Clin Epidemiol. (2020) 12:215–22. doi: 10.2147/CLEP.S238804
11. Depp, CA, and Jeste, DV. Definitions and predictors of successful aging: a comprehensive review of larger quantitative studies. Am J Geriatr Psychiatry. (2006) 14:6–20. doi: 10.1097/01.JGP.0000192501.03069.bc
12. Boop, C, Cahill, SM, Davis, C, Dorsey, J, Gibbs, V, Herr, B, et al. Occupational therapy practice framework: domain and process-fourth edition. Am J Occup Ther. (2020) 74:7412410010p1–7412410010p87. doi: 10.5014/ajot.2020.74S2001
13. Christensen, K, Thinggaard, M, Oksuzyan, A, Steenstrup, T, Andersen-Ranberg, K, Jeune, B, et al. Physical and cognitive functioning of people older than 90 years: a comparison of two Danish cohorts born 10 years apart. Lancet. (2013) 382:1507–13. doi: 10.1016/S0140-6736(13)60777-1
14. Duan, J, Lv, YB, Gao, X, Zhou, JH, Kraus, VB, Zeng, Y, et al. Association of cognitive impairment and elderly mortality: differences between two cohorts ascertained 6-years apart in China. BMC Geriatr. (2020) 20:29. doi: 10.1186/s12877-020-1424-4
15. Folstein, MF, Folstein, SE, and McHugh, PR. "Mini-mental state". A practical method for grading the cognitive state of patients for the clinician. J Psychiatr Res. (1975) 12:189–98. doi: 10.1016/0022-3956(75)90026-6
16. Cui, GH, Yao, YH, Xu, RF, Tang, HD, Jiang, GX, Wang, Y, et al. Cognitive impairment using education-based cutoff points for CMMSE scores in elderly Chinese people of agricultural and rural Shanghai China. Acta Neurol Scand. (2011) 124:361–7. doi: 10.1111/j.1600-0404.2010.01484.x
17. Zhang, MY, Katzman, R, Salmon, D, Jin, H, Cai, GJ, Wang, ZY, et al. The prevalence of dementia and Alzheimer's disease in Shanghai, China: impact of age, gender, and education. Ann Neurol. (1990) 27:428–37. doi: 10.1002/ana.410270412
18. Li, G, Shen, YC, Chen, CH, Zhau, YW, Li, SR, and Lu, M. A three-year follow-up study of age-related dementia in an urban area of Beijing. Acta Psychiatr Scand. (1991) 83:99–104. doi: 10.1111/j.1600-0447.1991.tb07373.x
19. Yesavage, JA, Brink, TL, Rose, TL, Lum, O, Huang, V, Adey, M, et al. Development and validation of a geriatric depression screening scale: a preliminary report. J Psychiatr Res. (1982) 17:37–49. doi: 10.1016/0022-3956(82)90033-4
20. Chen, X, Han, P, Yu, X, Zhang, Y, Song, P, Liu, Y, et al. Relationships between sarcopenia, depressive symptoms, and mild cognitive impairment in Chinese community-dwelling older adults. J Affect Disord. (2021) 286:71–7. doi: 10.1016/j.jad.2021.02.067
21. Wang, L, Wang, X, Song, P, Han, P, Fu, L, Chen, X, et al. Combined depression and malnutrition as an effective predictor of first fall onset in a Chinese community-dwelling population: a 2-year prospective cohort study. Rejuvenation Res. (2020) 23:498–507. doi: 10.1089/rej.2019.2188
22. Jongenelis, K, Pot, AM, Eisses, AM, Gerritsen, DL, Derksen, M, Beekman, AT, et al. Diagnostic accuracy of the original 30-item and shortened versions of the geriatric depression scale in nursing home patients. Int J Geriatr Psychiatry. (2005) 20:1067–74. doi: 10.1002/gps.1398
23. Lyness, JM, Noel, TK, Cox, C, King, DA, Conwell, Y, and Caine, ED. Screening for depression in elderly primary care patients. A comparison of the Center for Epidemiologic Studies-Depression Scale and the geriatric depression scale. Arch Intern Med. (1997) 157:449–54. doi: 10.1001/archinte.1997.00440250107012
24. Ringaitiene, D, Gineityte, D, Vicka, V, Zvirblis, T, Norkiene, I, Sipylaite, J, et al. Malnutrition assessed by phase angle determines outcomes in low-risk cardiac surgery patients. Clin Nutr. (2016) 35:1328–32. doi: 10.1016/j.clnu.2016.02.010
25. Guigoz, Y, Vellas, B, and Garry, PJ. Assessing the nutritional status of the elderly: the Mini nutritional assessment as part of the geriatric evaluation. Nutr Rev. (1996) 54:S59–65. doi: 10.1111/j.1753-4887.1996.tb03793.x
26. Chen, JY, Cheng, TJ, Chang, CY, Lan, KM, Weng, SF, Sheu, MJ, et al. Increased incidence of herpes zoster in adult patients with peptic ulcer disease: a population-based cohort study. Int J Epidemiol. (2013) 42:1873–81. doi: 10.1093/ije/dyt213
27. Lanas, A, and Chan, FKL. Peptic ulcer disease. Lancet. (2017) 390:613–24. doi: 10.1016/S0140-6736(16)32404-7
28. Wei, J, Fan, L, Zhang, Y, Li, S, Partridge, J, Claytor, L, et al. Association between malnutrition and depression among community-dwelling older Chinese adults. Asia Pac J Public Health. (2018) 30:107–17. doi: 10.1177/1010539518760632
29. Chrzastek, Z, Guligowska, A, Soltysik, B, Piglowska, M, Borowiak, E, Kostka, J, et al. Association of lower nutritional status and education level with the severity of depression symptoms in older adults-a cross sectional survey. Nutrients. (2021) 13:515. doi: 10.3390/nu13020515
30. Ulger, Z, Halil, M, Kalan, I, Yavuz, BB, Cankurtaran, M, Gungor, E, et al. Comprehensive assessment of malnutrition risk and related factors in a large group of community-dwelling older adults. Clin Nutr. (2010) 29:507–11. doi: 10.1016/j.clnu.2010.01.006
31. Neumann, SA, Miller, MD, Daniels, L, and Crotty, M. Nutritional status and clinical outcomes of older patients in rehabilitation. J Hum Nutr Diet. (2005) 18:129–36. doi: 10.1111/j.1365-277X.2005.00596.x
32. Mantzorou, M, Vadikolias, K, Pavlidou, E, Serdari, A, Vasios, G, Tryfonos, C, et al. Nutritional status is associated with the degree of cognitive impairment and depressive symptoms in a Greek elderly population. Nutr Neurosci. (2020) 23:201–9. doi: 10.1080/1028415X.2018.1486940
33. Landi, F, Sieber, C, Fielding, RA, Rolland, Y, and Guralnik, J. Nutritional intervention in sarcopenia: report from the international conference on frailty and sarcopenia research task force. J Frailty Aging. (2018) 7:247–52. doi: 10.14283/jfa.2017.26
34. Velazquez-Alva, MC, Irigoyen-Camacho, ME, Cabrer-Rosales, MF, Lazarevich, I, Arrieta-Cruz, I, Gutierrez-Juarez, R, et al. Prevalence of malnutrition and depression in older adults living in nursing homes in Mexico City. Nutrients. (2020) 12:2429. doi: 10.3390/nu12082429
35. Selvaraj, K, Jayalakshmy, R, Yousuf, A, Singh, AK, Ramaswamy, G, and Palanivel, C. Can mid-upper arm circumference and calf circumference be the proxy measures to detect undernutrition among elderly? Findings of a community-based survey in rural Puducherry. India J Family Med Prim Care. (2017) 6:356–9. doi: 10.4103/jfmpc.jfmpc_357_16
36. Holmes, CJ, and Racette, SB. The utility of body composition assessment in nutrition and clinical practice: an overview of current methodology. Nutrients. (2021) 13:2493. doi: 10.3390/nu13082493
37. Segall, L, Mardare, NG, Ungureanu, S, Busuioc, M, Nistor, I, Enache, R, et al. Nutritional status evaluation and survival in haemodialysis patients in one Centre from Romania. Nephrol Dial Transplant. (2009) 24:2536–40. doi: 10.1093/ndt/gfp110
38. Barro, M, Baro, MD, Cisse, D, Zagre, N, Ba, T, Neff Baro, S, et al. Upper arm length along with mid-upper arm circumference to enhance wasting prevalence estimation and diagnosis: sensitivity and specificity in 6-59-months-old children. Fam med. Community Health. (2021) 9:e000748. doi: 10.1136/fmch-2020-000748
39. Vedantam, A, Subramanian, V, Rao, NV, and John, KR. Malnutrition in free-living elderly in rural South India: prevalence and risk factors. Public Health Nutr. (2010) 13:1328–32. doi: 10.1017/S1368980009991674
40. Arlappa, N, Venkaiah, K, Rao, KM, Reddy, CG, Kumar, SS, Ravindranath, M, et al. Prevalence of chronic energy deficiency in rural-dwelling older Indian adults during a period of severe drought. J Nutr Elder. (2009) 28:301–12. doi: 10.1080/01639360903140338
41. Wang, B, Yan, X, Cai, J, Wang, Y, and Liu, P. Nutritional assessment with different tools in leukemia patients after hematopoietic stem cell transplantation. Chin J Cancer Res. (2013) 25:762–9. doi: 10.3978/j.issn.1000-9604.2013.12.09
Keywords: comprehensive geriatric assessment, nutritional status, Mini nutritional assessment, real-world, multivariable logistic regression analysis
Citation: Ju Y, Lin X, Zhang K, Yang D, Cao M, Jin H and Leng J (2024) The role of comprehensive geriatric assessment in the identification of different nutritional status in geriatric patients: a real-world, cross-sectional study. Front. Nutr. 10:1166361. doi: 10.3389/fnut.2023.1166361
Edited by:
Gabriela Salim de Castro, University of São Paulo, BrazilReviewed by:
Lina Ma, Xuanwu Hospital, Capital Medical University, ChinaShibao Lu, Capital Medical University, China
Kenji Nagao, Ajinomoto (Japan), Japan
Copyright © 2024 Ju, Lin, Zhang, Yang, Cao, Jin and Leng. This is an open-access article distributed under the terms of the Creative Commons Attribution License (CC BY). The use, distribution or reproduction in other forums is permitted, provided the original author(s) and the copyright owner(s) are credited and that the original publication in this journal is cited, in accordance with accepted academic practice. No use, distribution or reproduction is permitted which does not comply with these terms.
*Correspondence: Jiyan Leng, bGVuZ2p5QGpsdS5lZHUuY24=