- 1Graduate School, Beijing University of Chinese Medicine, Beijing, China
- 2Medical Oncology, Wangjing Hospital of China Academy of Chinese Medical Sciences, Beijing, China
Purpose: Shiliao Decoction (SLD) was developed for treatment and prevention of cancer-associated malnutrition (CAM) in China. In this study, we aim to discover SLD’s active compounds and demonstrate the mechanisms of SLD that combat CAM through network pharmacology and molecular docking techniques.
Methods: All components of SLD were retrieved from the pharmacology database of Traditional Chinese Medicine Systems Pharmacology (TCMSP). The GeneCards database and the Online Mendelian Inheritance in Man database (OMIM) were used to identify gene encoding target compounds, and Cytoscape was used to construct the drug compound–target network. The network of target protein-protein interactions (PPI) was constructed using the STRING database, while gene ontology (GO) functional terms and the Kyoto Encyclopaedia of Genes and Genomes (KEGG) pathways associated with potential targets were analyzed using a program in R language (version 4.2.0). Core genes linked with survival and the tumor microenvironment were analyzed using the Kaplan–Meier plotter and TIMER 2.0 databases, respectively. Protein expression and transcriptome expression levels of core gene were viewed using the Human Protein Atlas (HPA) and the Cancer Genome Atlas (TCGA). A component-target-pathway (C-T-P) network was created using Cytoscape, and Autodock Vina software was used to verify the molecular docking of SLD components and key targets.
Results: The assembled compound–target network primarily contained 134 compounds and 147 targets of the SLD associated with JUN, TP53, MAPK3, MAPK1, MAPK14, STAT3, AKT1, HSP90AA1, FOS, and MYC, which were identified as core targets by the PPI network. KEGG pathway analysis revealed pathways involved in lipid and atherosclerosis, the PI3K/Akt signaling pathway, and immune-related pathways among others. JUN is expressed at different levels in normal and cancerous tissues, it is closely associated with the recruitment of different immune cells and has been shown to have a significant impact on prognosis. The C-T-P network suggests that the active component of SLD is capable of regulating target genes affecting these related pathways. Finally, the reliability of the core targets was evaluated using molecular docking technology.
Conclusion: This study revealed insights into SLD’s active components, potential targets, and possible molecular mechanisms, thereby demonstrating a potential method for examining the scientific basis and therapeutic mechanisms of TCM formulae.
Introduction
Many malnourished cancer patients exhibit associated pathophysiological changes such as inflammation and immunosuppression, and a complex interplay exists between these two factors and cancer-associated malnutrition (CAM). Localized tumor cells and immune cells in the microenvironment interact to release various types of cytokines that promote systemic inflammation, contributing to malnutrition and poor outcomes by causing anorexia, altering metabolism, and increasing resting energy expenditure and muscle breakdown (1). According to the results of the largest global survey to date (2), the overall prevalence of malnutrition among inpatients with cancer in China is 80.4%, and the prevalence of moderate and severe malnutrition is as high as 58.2% (3). Malnutrition, as the most common clinically neglected comorbidity in tumor patients, is a major prognostic predictor of poor clinical outcomes for cancer patients.
Nutrition and health have been incorporated into China’s national strategy in “Health China 2030” and the “National Nutrition Plan (2017-2030),” which place particular focus on rapidly improving the nutrition of cancer patients. Although there is a great need for effective therapeutic options to address CAM, there is a lack of pharmacological agents that can be safely administered as part of long-term treatment. Traditional Chinese medicine (TCM) is a major component of complementary alternative medicine and can play important roles in the entire cancer treatment process, up to and including palliative care in advanced stages. Our team has long been concerned about the metabolic and immune disturbances in tumor patients and have previously designed a therapy formula named Shiliao Decoction (SLD) aimed at improving nutrition levels, drawing on the ideas behind the Wu Zhi An Zhong and Da Jian Zhong decoctions (4). Previous single-armed clinical studies have shown that SLD has outstanding efficacy in improving patients’ appetite and relieving pain, as well as synergistic effects with anti-tumor therapy.
Network pharmacology can create a relationship prediction model between drugs and disease targets, integrate an interaction network to analyze drug interactions with specific nodes in each network module, and investigate the interaction relationship between drugs and potential targets from a systematic perspective (5, 6). Network pharmacology is highly suited for evaluating the numerous components, targets, and pathways of TCM due to its complex composition and multitarget therapeutic characteristics (7, 8). Computer simulation technology is used in molecular docking (9). In this study, we sought to use network pharmacology to understand the active compounds of SLD and predict their potential targets and signaling pathways for the treatment of CAM. Molecular docking analysis techniques were used to validate previously obtained targets. In addition, the structural docking of related proteins and compounds has the potential to provide a theoretical basis for the development of new drugs containing the active alkaloids of botanical drugs (Figure 1).
Materials and methods
Composition of shiliao decoction
SLD is a decoction of 10 botanicals extracted by water, including Codonopsis pilosula (Franch.) Nannf (Campanulaceae; Codonopsis radix), Zanthoxylum bungeanum Maxim (Rutaceae; Zanthoxylum bungeanum pericarp), Citrus reticulata Blanco (Rutaceae;Citri reticulatae pericarpium), Coix lacryma-jobi L.var.ma-yuen (Roman.) Stapf (Gramineae; Coicis semen), Dioscorea opposite Thunb (Dioscoreaceae; Dioscoreae rhizoma), Typha angustifolia L.(Typhaceae; Typhae pollen), Amomum villosum Lour (Zingiberaceae; Amomi fructus), Zingiber officinale Rosc (Zingiberaceae; Zingiberis rhizome recens), Ziziphus jujuba Mill. (Rhamnaceae; Jujubae fructus), Glycyrrhiza uralensis Fisch (Leguminosae; Glycyrrhiza radix et rhizoma), all of which are plants known to have medicinal properties. Details on the composition of SLD can be found in Table 1. The plant taxonomy was confirmed using the MPNS database1 and drug names were confirmed using the Chinese Pharmacopeia 2020 edition.
Database establishment
Active components of SLD were identified in the Traditional Chinese Medicine Systems Pharmacology (TCMSP) Database and Analysis Platform (TCMSP)2 (10). Oral bioavailability (OB) refers to the rate and extent at which a drug is absorbed into the body’s circulatory system. Drug-like (DL) properties reflect the nature of a drug that has a specific functional group or contains the same or similar physical characteristics. Compounds with higher activity were further screened for compounds with both high OB and DL, using OB > 30% and DL > 0.18 as thresholds (11). Target proteins corresponding to each component were then obtained in the TCMSP Database and converted to a unified gene name using the protein database UniProt3 (12).
Identification of putative target genes for cancer-associated malnutrition
The GeneCards4 (13) and OMIM5 (14) databases were searched using the keyword “cancer malnutrition” and the species “Homo sapiens.” Retrieved gene data were merged and disease-related target data were obtained by filtering out duplicate values.
Shiliao decoction and cancer-associated malnutrition target screening and network construction
A Venn diagram was built by mapping active ingredient targets to disease targets using the “Venn” function package in R software (15), and overlapping targets were identified as core targets of SLD for CAM therapy. A common target network was constructed using Cytoscape (version 3.8.2) (16).
Construction of the protein-protein interaction network
Shared target data about drugs and diseases were imported into the STRING 11.0 database6 (17) to construct protein-protein interaction (PPI) networks. To ensure the robustness of the analysis, the screening threshold of the STRING database was set to an interaction score ≥ 0.9, and free proteins were removed. PPI networks were then visualized and analyzed using Cytoscape (16). To identify the central nodes and key proteins in the PPI network, topological parameters were calculated using NetworkAnalyzer. CytoNCA (18) was used to calculate degree, betweenness, and closeness in order to describe the topological importance of proteins in the network.
Biological information: Gene ontology and genome encyclopedia pathway enrichment analysis
Target gene analysis was performed using the “cluster profile” and “pathview” function package in R, a well-known R package with dynamically updated data for KEGG and GO analysis. Results were screened using a threshold p-value of ≤ 0.05. Results were then visualized using the “ggplot2 package” in R software (version 4.2.0) to obtain a bubble map of the results of the GO and KEGG enrichment analyses. Biological process (BP), cellular component (CC), and molecular function (MF) are all included in the GO functional analysis.
Prognostic values of hub genes and analysis of tumor-infiltrating immune cells
The Kaplan-Meier Plotter (19) is a powerful online tool for assessing the impact of 54,000 genes on survival in 21 different cancer types. We focused on the relationship between the expression of the height-degree value gene and survival, including overall survival (OS) and progression-free survival (PFS). Log-rank P-values with 95% confidence intervals (CIs) and hazard ratios (HRs) were calculated.
The TIMER database7 (20) contains 10,897 TCGA samples from 32 cancer types and is a suitable resource for the systematic study of immune infiltration in different cancer types. Key targets of SLD components were analyzed using the TIMER database to explore their relationship with immune cell infiltration (B cells, CD4+T cells, CD8+T cells, neutrophils, macrophages, and dendritic cells) and the association with prognosis.
Differential expression analyses
Immunohistochemistry (IHC) can reveal the relative distribution and abundance of proteins by utilizing the high specificity of antibody-antigen binding. Immunohistochemical data were obtained from the Human Protein Atlas (HPA),8 and the expression of target genes in normal and cancer tissues was compared. Transcriptome data of cancer-related genes were obtained from The Cancer Genome Atlas (TCGA).9
Construction and analysis of the component-target-pathway network
An appropriate component-target-pathway (C-T-P) network was constructed and visualized via Cytoscape (16) to analyze the association between SLD, candidate targets, and CAM-related pathways.
Molecular docking analysis
2D structures of the 10 compounds with the highest degree of active ingredients were downloaded from the Pub Chem database10 (21) and saved in “SDF” format. Chem 3D was used to convert the “SDF” format into mol2 structures as small molecule ligands. The 3D structures of the top 6 proteins in terms of degree value were retrieved from the PDB database11 (22) and saved as protein receptors in “PDB” format. Water molecules were removed using PyMOL software (version 2.3.6), and the original ligands were isolated from core target proteins. The processed protein targets were imported into AutoDock software (version 4.2.0) (23) for hydrogenation, calculation of total charge, and setting of the atom type. Ligands and protein receptors were recorded in PDBQT format. Molecular docking was performed with AutoDock-Vina software (version 1.1.2) (24) to evaluate the affinity of the receptor-ligand complexes with a comprehensive score. Molecular docking was visualized using Discovery Studio and docking patterns were displayed in 2D and 3D structures. Docking effects were evaluated based on their affinity value, with affinity values < –5 kcal/mol interpreted as representing good binding interaction between compound and target (25).
Results
The main active ingredients of shiliao decoction and putative target genes for cancer-associated malnutrition
Target prediction of SLD ingredients using the TCMSP database and screening (for OB ≥ 30% and DL ≥ 0.18 as described in methods) identified 172 compounds with sufficiently high OB and DL. They consisted of 5 compounds from Zanthoxylum bungeanum Maxim., 4 compounds from Zingiber officinale Rosc., 5 compounds from Citrus reticulata Blanco., 6 compounds from Typha angustifolia L., 17 compounds from Codonopsis pilosula (Franch.) Nannf., 88 compounds from Glycyrrhiza uralensis Fisch., 6 compounds from Coix lacryma-jobi L.var.ma-yuen (Roman.) Stapf., 19 compounds from Ziziphus jujuba Mill., 9 compounds from Amomum villosum Lour., 12 compounds from Dioscorea opposite Thunb.
The UniProt database was used to search for information on potential targets of the active ingredients identified by the TCMSP database. Target gene names were standardized, and 261 target genes were obtained after removal of duplicates.
2,581 CAM-related genes were identified by searching the GeneCards database, and 168 CAM-related genes were separately identified in the OMIM database. Following combination of these two sets and removal of duplicates, a total of 2,706 CAM-related genes were selected for further analysis.
Shiliao decoction and cancer-associated malnutrition target gene screening and network construction
In order to identify potential gene targets of SLD with an effect on CAM, the set of 2,706 CAM-related genes selected earlier was compared with the set of 261 target genes of SLD components. A total of 147 potential target genes were found to be members of both these sets.
Cytoscape software was used to visualize the compound-target relationships and rank the components of SLD in descending order of degree. The top 12 key compounds are listed in Table 2, and the “compound-target” network consisting of 281 nodes and 1,360 edges, including 134 active components and 147 targets, is illustrated in Figure 2.
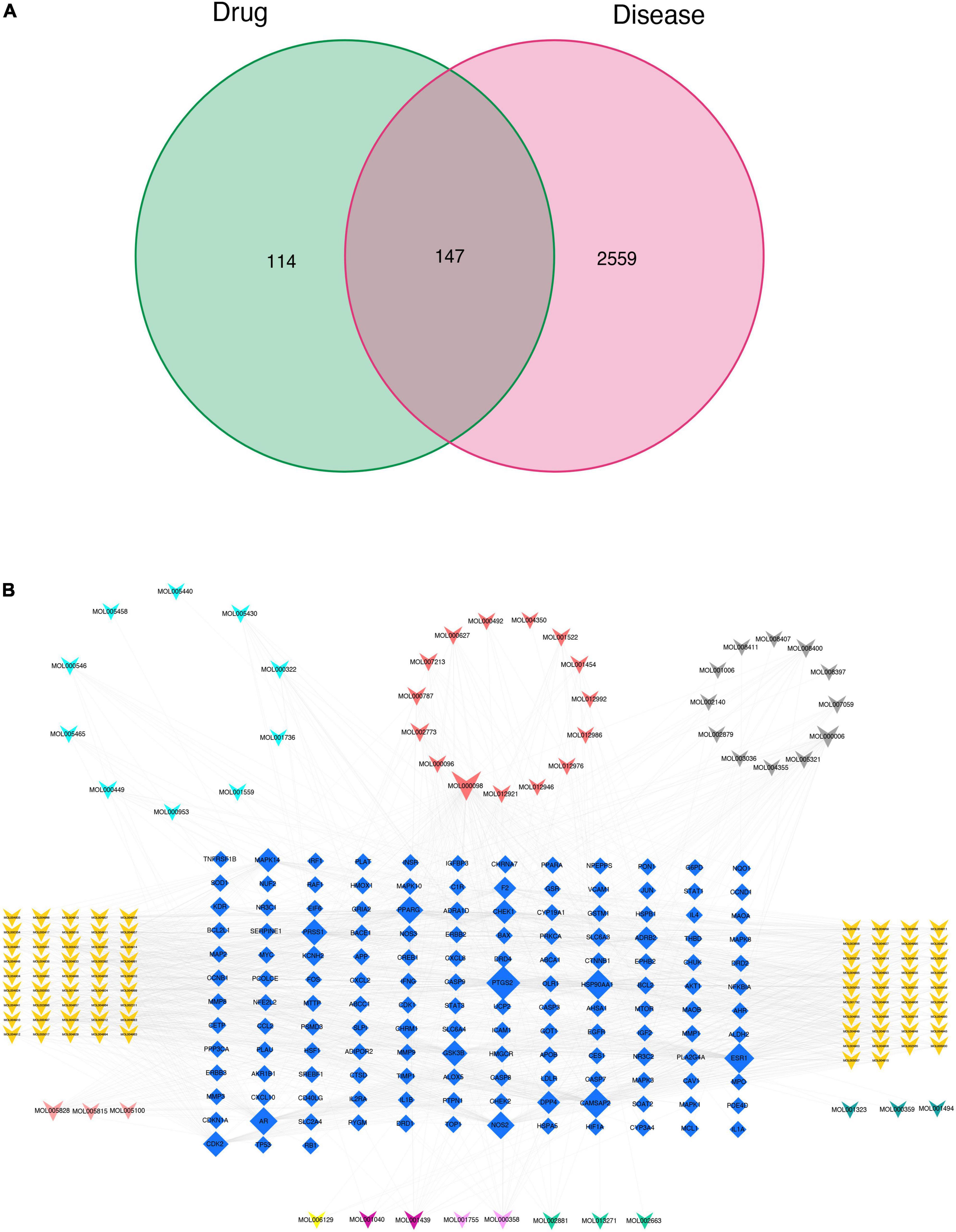
Figure 2. Construction of the target genes set (A) and compound-target network (B). Blue quadrilateral nodes indicate targets, while differentially colored V nodes denote compounds. The dark yellow, light purple, light red, light blue, dark green, gray, dark red, dark purple, light yellow, and light green V nodes represent active compounds of Glycyrrhiza uralensis Fisch., Amomum villosum Lour., Citrus reticulata Blanco., Dioscorea opposite Thunb., Coix lacryma-jobi L.var.ma-yuen (Roman.) Stapf., Codonopsis pilosula (Franch.) Nannf., Ziziphus jujuba Mill., Typha angustifolia L., Zingiber officinale Rosc., Zanthoxylum bungeanum Maxim., respectively.
Protein-protein interactions network construction and key target screening
We created a PPI network by importing the 147 target genes selected earlier into the STRING database. Cytoscape software was used to visualize the PPI network. Screening was carried out using a threshold score value of 0.9 to provide a high level of confidence for protein interactions, and unconnected nodes in the network were concealed, as seen in Figure 3A. Topological analysis was carried out using the CytoNCA plug-in. To identify core target genes, “intermediate centrality (BC), closeness centrality (CC), and degree centrality (DC) greater than the median” were utilized as screening criteria. This process is shown in Figure 3B, and specific information on the 10 core target genes is listed in Table 3.
Gene ontology and genome encyclopedia enrichment analysis
GO enrichment analysis found that BP mainly included pathways related to cellular metal ion response, response to drugs, and response to radiation. CC included the endocytotic activation pathway of membrane rafts and membrane microfilms among other pathways. MF mainly included pathways related to DNA binding transcription factor and ubiquitinated protein ligase (Figure 4A). Pathways identified by KEGG analysis included those involved in lipid and atherosclerosis, the PI3K-Akt signaling pathway, MAPK signaling pathway, TNF signaling pathway, cancer-related signaling pathways, and numerous immune-related signaling pathways (Figure 4B). Among these enriched pathways, we found that lipid and atherosclerosis signaling pathways play an important role in CAM (Figure 4C).
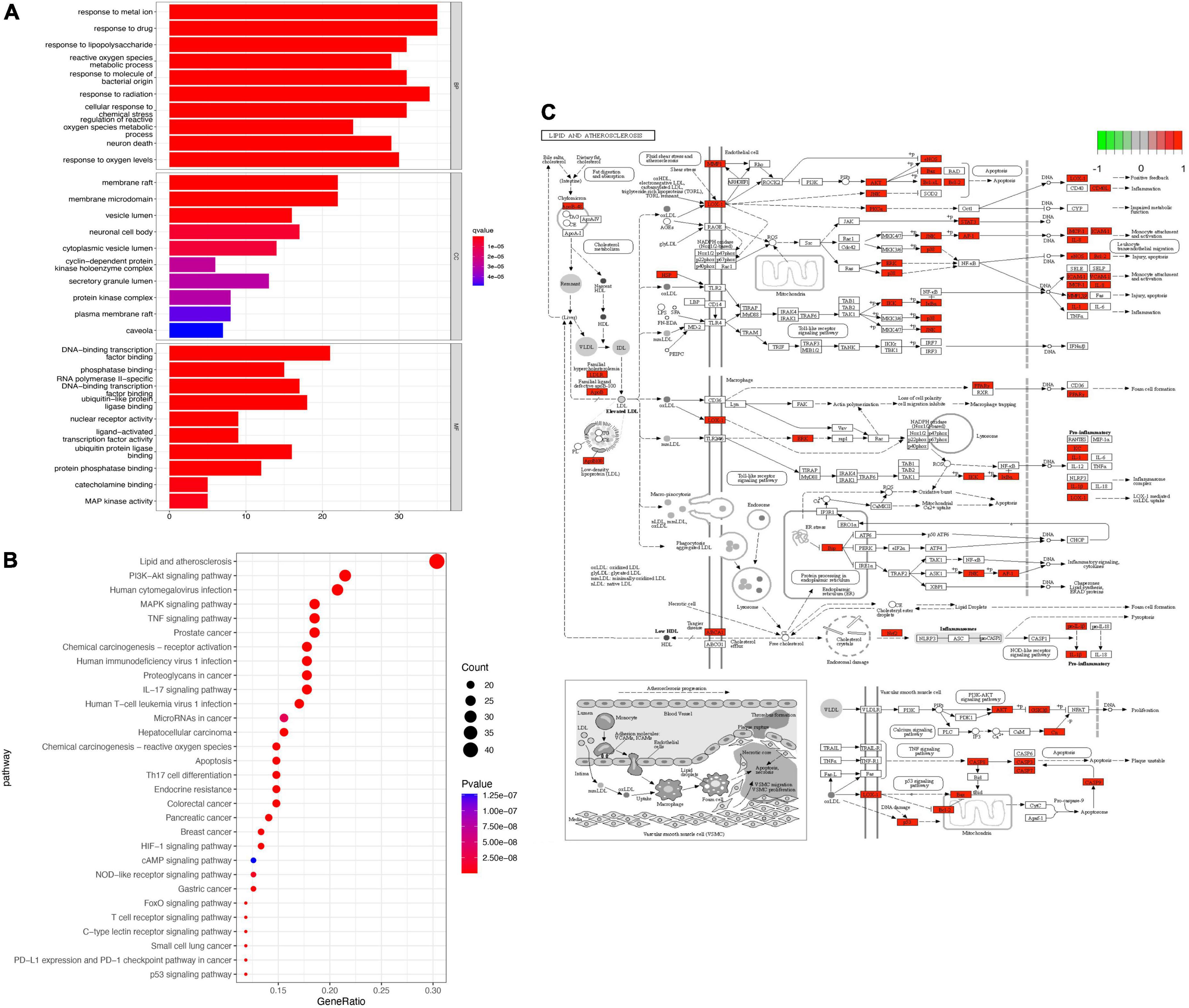
Figure 4. Enrichment analysis. (A) GO enrichment analysis. (B) KEGG enrichment analysis. (C) The most enriched KEGG pathway. Red genes in the network is the SLD target genes.
Prognostic values and immune infiltration
Based on these results, we selected JUN as a representative gene and focused on cancer types with co-occurring malnutrition to explore the association between them. Significant correlations (P ≤ 0.05) were found between JUN expression levels and survival in patients with liver hepatocellular carcinoma (LIHC), esophageal squamous carcinoma (ESCA) and rectal adenocarcinoma (READ), as shown in Figures 5A–D.
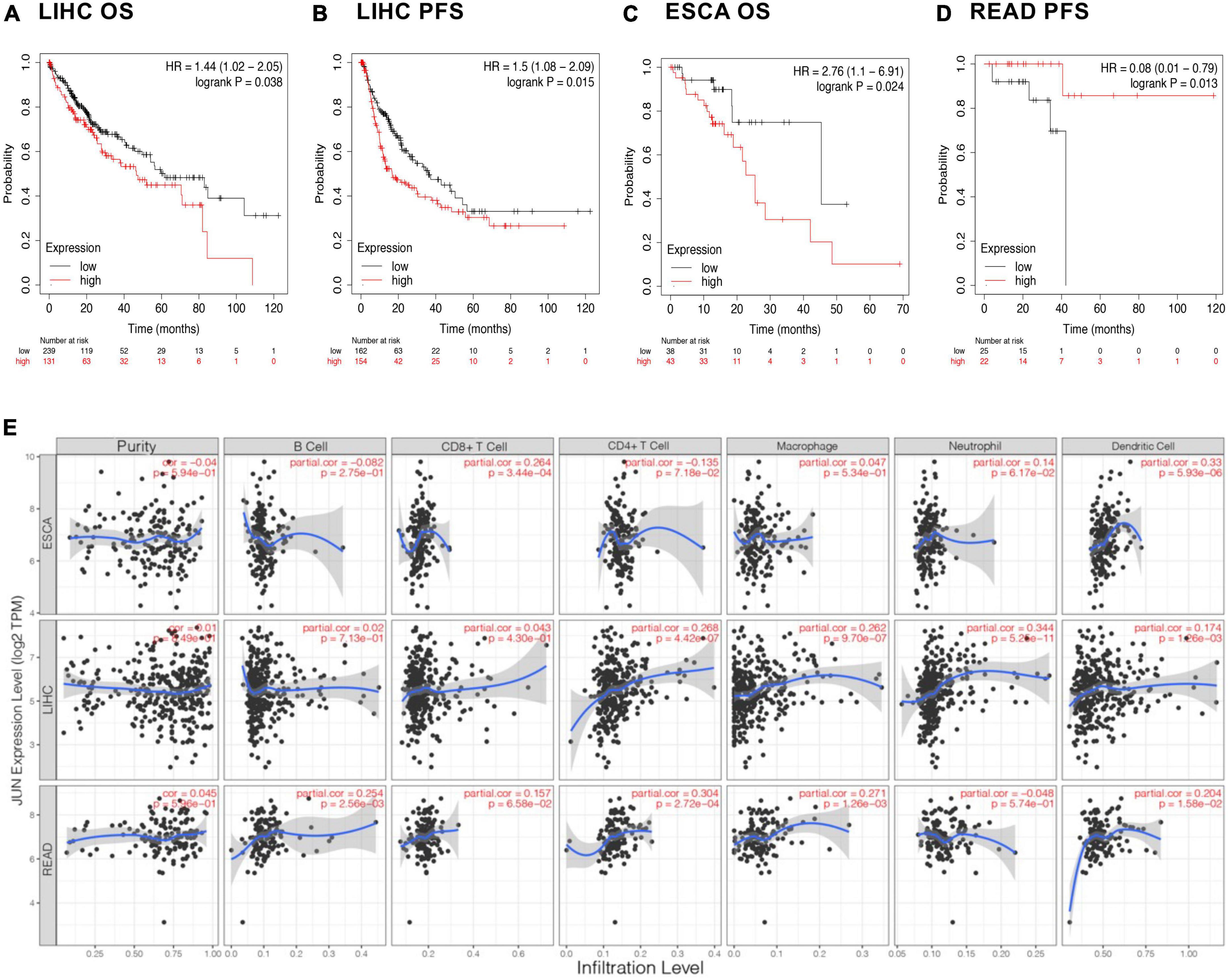
Figure 5. Immune infiltration and prognosis. (A–D) Association of JUN with the prognosis of different cancers. (E) Correlation of JUN expression with immune cells.
To further explore possible immunological mechanisms, we looked at the relationship between gene expression, tumor tissue purity, and the abundance of immune infiltrating cells in the microenvironment using the TIMER database. Tumor tissue purity is the proportion of cancer cells in a tumor sample, which is critical to selecting genes related to deconvolving immune cells in the tumor tissue. This was inferred from copy number alteration data using the R package CHAT (26). As seen in Figure 5E, it was observed that JUN expression levels did not significantly correlate with tumor purity, but more likely affected prognosis by regulating the tumor microenvironment. For example, in ESCA, JUN expression was positively correlated with infiltration of CD8+ T cells and dendritic cells. In LIHC, JUN expression was positively correlated with infiltration of CD4+ T cells, macrophages, and neutrophils while in READ, JUN expression was positively correlated with CD4+ T cell, macrophage and dendritic cell infiltration.
Differential expression of JUN in cancers
To verify the potential of the identified proteins as therapeutic targets, we analyzed the differential expression of proteins in cancer and cancer-adjacent tissues. IHC results from the HPA database showed that JUN expression in cancer tissues was higher than that in normal liver and rectum tissues. On the other hand, antibody staining levels of JUN in ESCA cancer tissues were lower than those in adjacent tissues (Figure 6A).
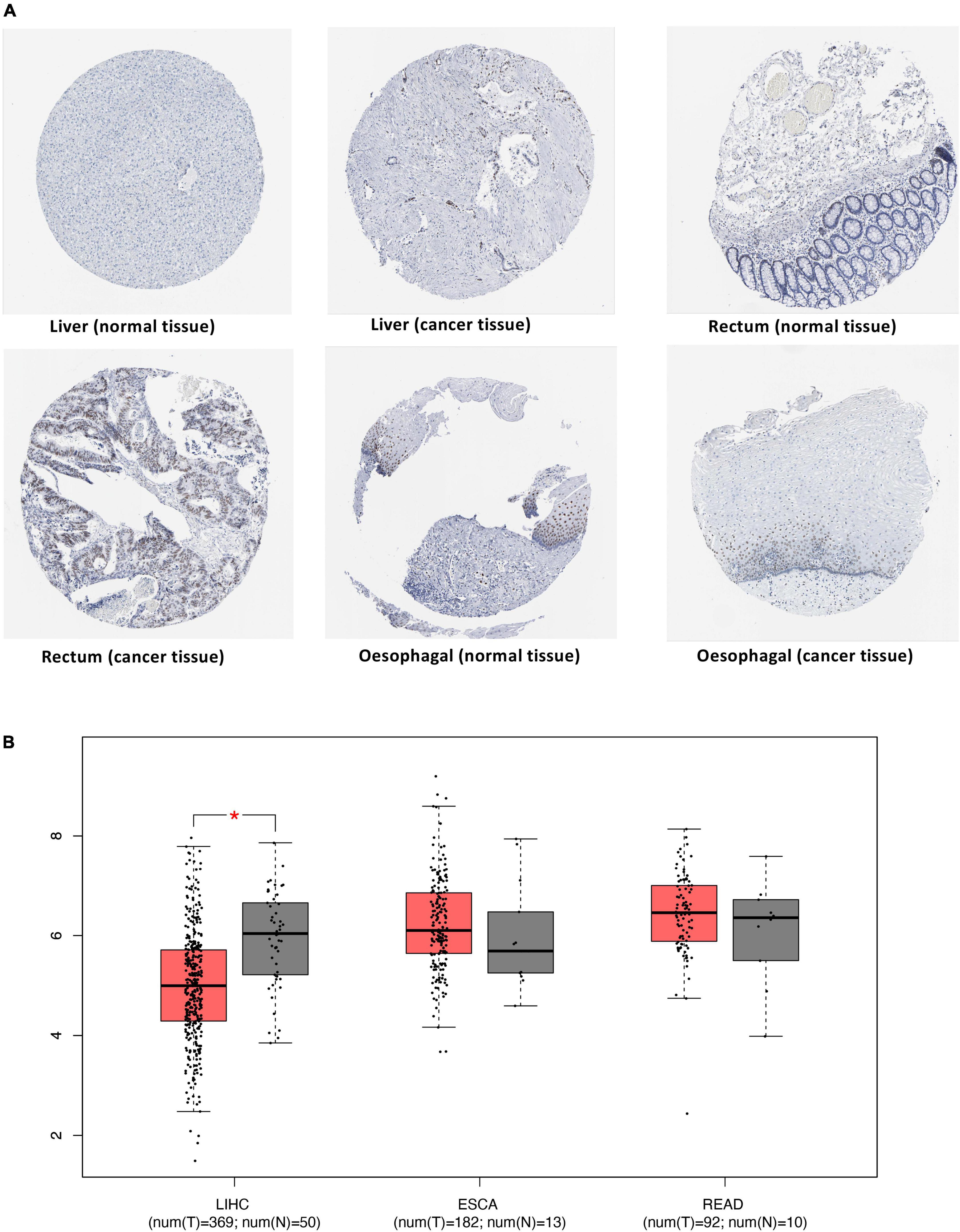
Figure 6. Differential expression of JUN in cancers. (A) Validation of the expression of JUN at the protein level using the HPA database. (B) Validation of the expression of JUN at the transcriptome level using the TCGA database.
The TCGA database was used to expand the sample size for validation and obtain expression data at the transcriptome level for this gene. The box plot of differential expression analysis (Figure 6B) showed that JUN was significantly overexpressed in normal tissues than in liver cancer tissues.
Component-target-pathway network and molecular docking
A “C-T-P” network was constructed based on the 30 most relevant signaling pathways obtained in Figure 4B. Quercetin, luteolin, kaempferol, nobiletin, naringenin, 7-Methoxy-2-methyl isoflavone, formononetin, licochalconea, and isorhamnetin were the elements with the most targets in this network, indicating that these substances may be the foundation of how SLD affects CAM. JUN (PDB ID:1A02), TP53 (PDB ID: 6wqx), MAPK3 (PDB ID: 6GES), MAPK1 (PDB ID: 7nr9), MAPK14 (PDB ID:6QE1), and STAT3 (PDB ID: 6tlc) were protein targets that connected with the most active components and pathways, suggesting that they may be key targets for treatment of CAM with SLD. It is likely that active components of SLD act through targets to jointly regulate signaling pathways involved in lipid and atherosclerosis, the PI3K-Akt signaling pathway, immune-related signaling pathways, and pathways in cancer to improve patient outcomes (Figure 7A).
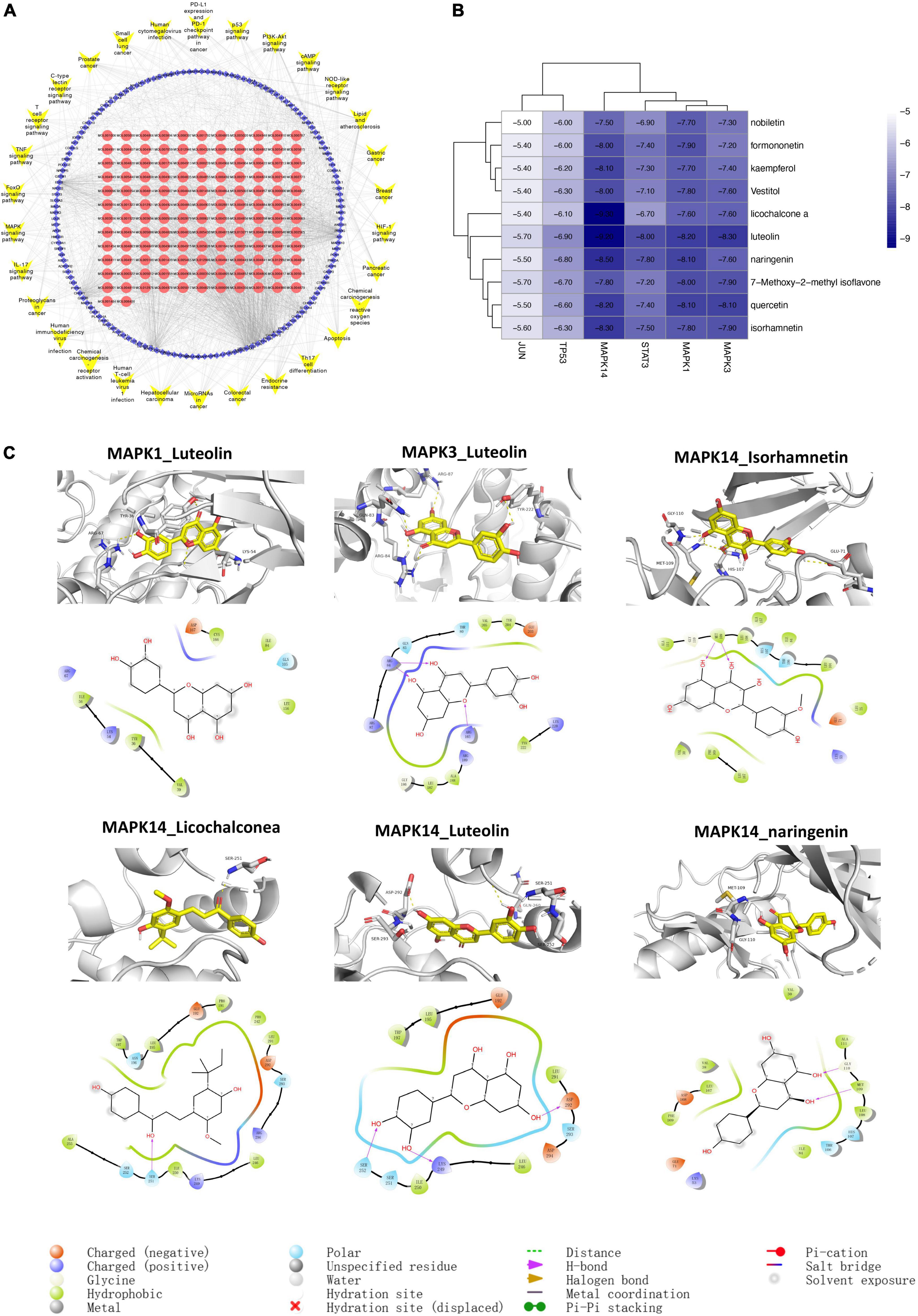
Figure 7. Component-target-pathway network (A) and molecular docking (B,C). (A) The blue diamonds represent genes. The red circle nodes denote components of SLD, and the yellow V nodes represent CAM-related signaling pathways. (B) Molecular docking results of core targets and active compounds. The color indicates an affinity score. Dark blue represents the lowest affinity score and the highest affinity between receptor and ligand. White represents the highest affinity score and the lowest affinity between receptor and ligand. (C) Optimal complex structure of components and targets.
We performed molecular docking between the top 6 protein targets in the “active ingredient-target-disease” network and the top 10 active ingredients in the SLD using Autodock vina. Based on similar experiments, binding energy < –4.25 kJ-mol-1 was interpreted as indicating that the active ingredient had some binding ability for the target, binding energy < –5.0 kJ/mol indicated good binding activity, and binding energy < –7.0 kJ/mol indicated strong binding activity (27). Analysis of molecular docking found that the binding energies of the above target proteins and compounds were less than –5 kcal/mol, indicating good binding activity. Detailed results are shown in Figures 7B,C.
Discussion
Increased catabolism of fat, muscle, and other tissues is a clinical characteristic shared by malnutrition and cancer cachexia, a specific kind of CAM characterized by persistent inflammation (28). Notably, typical nutrition therapy does not completely reverse cancer cachexia. Refractory cachexia is an advanced stage of disease fundamentally arising from the combination of uncorrected nutritional impairment and lack of response to anticancer treatment. Nutritional issues of patients should be thought of as existing along a continuum, stretching from the first signs and symptoms of anorexia to precachexia, cachexia, and refractory cachexia (29). It is well known that the efficacy and the impact of nutritional interventions are linked to the timing of such support, with the best results obtained with early intervention. However, there are no effective medical interventions in Western medicine that can completely reverse the conditions of CAM and cachexia. By contrast, TCM containing phytonutrients has good efficacy and safety and should be explored in greater depth.
The TCM expert and one of the pioneers of Chinese medical oncology Professor Zhou Daihan proposed that Chinese medical oncology food therapy is a discipline based on the theory of the doctrine of TCM organs and meridians, combined with modern oncology and nutrition to guide people to cure tumors through dietary changes (30). According to the latest document “National Health Food Convenience Letter (2018) No. 8” issued by the National Health Commission of China in 2018, 110 Chinese botanical drugs are recognized as medicinal and food ingredients and promoted for appropriate medical use (31). Based on the basic system theory of Chinese medicine, our team selected ten botanical drugs to make up SLD. Through network pharmacological research, the primary active components of SLD for CAM treatment were identified as quercetin, luteolin, kaempferol, nobiletin, naringenin, 7-Methoxy-2-methyl isoflavone, formononetin, licochalconea, and isorhamnetin. Quercetin, luteolin, kaempferol, nobiletin, naringenin and isorhamnetin are all flavonoids, which possess strong anti-inflammatory and anti-tumor effects both in vitro and in vivo. Flavonoids have been shown to lower triglyceride and cholesterol levels and reduce inflammatory mediators (32), as well as activate the monocyte/macrophage pathway. This suggests that flavonoids may contribute to treatment of CAM by regulating inflammation, immunity and lipid metabolism (33). For example, luteolin has been found to reduce cancer-induced skeletal muscle atrophy in a Lewis lung cancer mouse model by a mechanism that inhibits TNF-α and IL-6 directly and can suppress inflammation levels to treat cachexia by reducing NF-κB activation at the transcriptional and translational level (34). A 2021 study using mice with C26 cells found that naringenin prevented the loss of muscle strength and decreased levels of insulin resistance and inflammation (35). Many reports have demonstrated that kaempferol is a safe and effective natural dietary anti-inflammatory agent, and the poor bioavailability of kaempferol has also been addressed by nanotechnology (36). Kaempferol has been shown to protect against chemotherapeutic drug-induced cardiotoxicity and improve advanced tumor cardiac cachexia by inhibiting p53-mediated mitochondria-dependent apoptotic signaling and regulating in ERK-dependent mitogen-activated protein kinase pathway in in vitro and in vivo assays (37).
In this study, JUN, TP53, MAPK3, MAPK1, MAPK14, STAT3, AKT1, HSP90AA1, FOS, and MYC were finally selected based on the PPI network and major targets of the C-T-P network map. Molecular docking studies have shown that SLD has a good affinity for the products of many of these gene targets. JUN, a prominent member of the activator protein 1 (AP-1) family, plays an important role in processes including cell proliferation, differentiation, and tumor transformation (38). A study using an animal model of prolonged fasting malnutrition showed that metabolic control in metabolically active organs is exerted by transcription factors, including JUN, activated by nutritional signaling (39). Consistent with this, the presence of anti-c-Jun antibodies has been shown to reduce the nuclear activator protein AP-1 binding activity in protein energy-deficient dystrophic rats (40). A clinical study also found oxidative modifications and ubiquitination of Jun-D in skeletal muscle of patients with cancer cachexia, suggesting that this factor is also associated with cachectic muscle atrophy (41). JUN is also a key target of c-Jun N-terminal kinase (JNK), a member of the mitogen-activated protein kinase superfamily (MAPK), which activates JNK, translocates it from the nucleus to the cytoplasm and phosphorylates Jun, thereby increasing its activation potential. Following cancer-induced activation of Toll-like receptor 4 in skeletal muscle, p38β MAPK phosphorylates Ser-12 on p300 to stimulate C/EBPβ acetylation. p38β MAPK has been shown to cause skeletal muscle atrophy by inducing autophagy in skeletal muscle cells (42). As such, it may be a central mediator and potential therapeutic target in cancer-induced muscle atrophy. Because nilotinib selectively inhibits p38β MAPK, systemic administration of nilotinib at low doses (0.5 mg/kg/day, ip) in tumor-bearing mice not only attenuates muscle atrophy but also prolongs survival (43). The cachexia phenotype is causally associated with the cytokine-activated transcription factor STAT3, which contributes to symptoms including skeletal muscle atrophy, cardiac dysfunction, and hypothalamic inflammation (44). Related studies have shown that STAT3 is associated with fat wasting and the acute phase response to cancer cachexia. STAT3 also contributes to cancer cachexia by enhancing tumorigenesis, metastasis and immunosuppression, particularly in tumor types associated with a high risk of cachexia (45).
GO gene enrichment and KEGG pathway analysis identified pathways affecting lipid metabolism and atherosclerosis, in addition to many immune-related pathways. Brown catabolism of fat is necessary for muscle catabolism in CAM, linking fat metabolism to CAM and indicating that it may be a prime target process for early therapy intervention (46). Animal studies have confirmed that quercetin inhibits cisplatin-induced fat loss by regulating the expression of genes involved in fat metabolism and synthesis as well as plasma TNF-α levels (47). Citrus flavonoids, including naringenin and nobiletin, can blunt the inflammatory response in metabolically important tissues including adipose tissue, and have emerged as promising therapeutic agents for the treatment of metabolic dysregulation (48). The above results suggest that SLD has the potential to intervene in CAM and curb the progression of cachexia at an early stage by reducing pathological inflammation and improving lipid metabolism. On the other hand, Luteolin activates the PI3K-Akt pathways in antigen presenting cells (APCs), induces the activation of APCs, enhances cytotoxic T lymphocyte responses, and inhibits tolerogenic T cells (49). An in silico docking simulation determines the detailed mode of binding of kaempferol to PD-1/PD-L1 and these results suggest that kaempferol can potentially be developed as a potent small molecule inhibitor for PD-1/PD-L1 blockade (50). Isorhamnetin has been identified as a negative regulator of pro-inflammatory cytokine release from CD4+ T lymphocytes and has also been shown to reduce the release of pro-inflammatory cytokines from primary human lymphocytes (51). Therefore, we hypothesize that SLD may improve the prognosis of tumor patients by influencing the immune microenvironment, a proposal that is supported by the K-M and TIMER databases.
However, this study had several limitations. First, since the data from online databases were based on evaluated, we may not have included undocumented or unverified chemicals or targets in our study. Second, the metabolic forms, effective components, and absorption mechanisms of bioactive components of SLD would ideally be studied in greater depth. Furthermore, it must also be noted that this decoction interacts with the gut microbiota following oral ingestion, and this process may be different in subjects with different cancers or microbiome populations. Future microbiota-based multi-omics studies in Chinese medicine are likely to provide richer insights (52).
Conclusion
In conclusion, we identified luteolin, kaempferol, isorhamnetin, and several other compounds to be the main active components of SLD. Among their targets that may mediate anti-CAM effects are JUN, TP53, MAPK, STAT3, etc. The effect of SLD appears to be primarily mediated by control of the inflammatory response through lipid metabolism pathways, immune-related pathways, and reduction in tissue and organ depletion that translate into improved prognoses for patients with CAM. The results of our study provide recommendations for experimental validation and studies on clinical application.
Data availability statement
The datasets presented in this study can be found in online repositories. The names of the repository/repositories and accession number(s) can be found in the article.
Author contributions
SL and SJ wrote the manuscript, performed GO, KEGG. immune infiltration, survival analysis, expression differences, and molecular docking. HX modified the figures. PX edited and improved the manuscript. SL compiled the TCM database and target capture. SJ collated the disease target database. SZ directed the research and proposed changes to the manuscript. All authors reviewed the manuscript and approved the final version of the manuscript.
Funding
This work was financially supported by the Traditional Chinese Medicine Innovation Team Project of the National Administration of Traditional Chinese Medicine (ZYYCXTD-C-202205).
Conflict of interest
The authors declare that the research was conducted in the absence of any commercial or financial relationships that could be construed as a potential conflict of interest.
Publisher’s note
All claims expressed in this article are solely those of the authors and do not necessarily represent those of their affiliated organizations, or those of the publisher, the editors and the reviewers. Any product that may be evaluated in this article, or claim that may be made by its manufacturer, is not guaranteed or endorsed by the publisher.
Footnotes
- ^ http://mpns.kew.org
- ^ http://tcmspw.com/tcmsp
- ^ http://www.uniprot.org/uploadlists/
- ^ https://www.genecards.org/
- ^ https://www.omim.org/
- ^ https://string-db.org/
- ^ http://cistrome.org/TIMER/
- ^ https://www.proteinatlas.org/
- ^ https://portal.gdc.cancer.gov/
- ^ https://pubchem.ncbi.nlm.nih.gov/
- ^ https://www.rcsb.org/
References
1. Arends J, Baracos V, Bertz H, Bozzetti F, Calder PC, Deutz NEP, et al. Espen expert group recommendations for action against cancer-related malnutrition. Clin Nutr. (2017) 36:1187–96. doi: 10.1016/j.clnu.2017.06.017
2. Song CH, Wang KH, Guo ZQ. Survey on the nutritional status of patients with common malignant tumors in China. Sci China Life Sci. (2020) 50:1437–52.
3. Ryan AM, Power DG, Daly L, Cushen SJ, Ní Bhuachalla Ç, Prado CM. Cancer-associated malnutrition, cachexia and sarcopenia: the skeleton in the hospital closet 40 years later. Proc Nutr Soc. (2016) 75:199–211. doi: 10.1017/s002966511500419x
4. Sun Y, Zhang J, Chen Y. Efficacy and safety of Jianzhong decoction in treating peptic ulcers: a meta-analysis of 58 randomised controlled trials with 5192 patients. BMC Complement Altern Med. (2017) 17:215. doi: 10.1186/s12906-017-1723-2
5. Sun S, Wang M, Yuan Y, Wang S, Ding H, Liang C, et al. A new strategy for the rapid identification and validation of direct toxicity targets of psoralen-induced hepatotoxicity. Toxicol Lett. (2022) 363:11–26. doi: 10.1016/j.toxlet.2022.05.002
6. Hopkins AL. Network pharmacology: the next paradigm in drug discovery. Nat Chem Biol. (2008) 4:682–90.
7. Liu S, Zhao F, Deng Y, Zeng Y, Yan B, Guo J, et al. Investigating the multi-target therapeutic mechanism of Guihuang formula on chronic prostatitis. J Ethnopharmacol. (2022) 294:115386. doi: 10.1016/j.jep.2022.115386
8. Li S, Zhang B. Traditional Chinese medicine network pharmacology: theory, methodology and application. Chin J Nat Med. (2013) 11:110–20.
9. Kumar S, Bhardwaj VK, Singh R, Das P, Purohit RA-O. Identification of acridinedione scaffolds as potential inhibitor of denv-2 c protein: an in Silico strategy to combat dengue. J Cell Biochem. (2022) 123:935–46. doi: 10.1002/jcb.30237
10. Ru J, Li P, Wang J, Zhou W, Li B, Huang C, et al. Tcmsp: a database of systems pharmacology for drug discovery from herbal medicines. J Cheminform. (2014) 6:13. doi: 10.1186/1758-2946-6-13
11. Liu H, Wang J, Zhou W, Zhou W, Wang Y, Yang L. Systems approaches and polypharmacology for drug discovery from herbal medicines: an example using licorice. J Ethnopharmacol. (2013) 146:773–93. doi: 10.1016/j.jep.2013.02.004
12. The UniProt Consortium. Uniprot: the universal protein knowledgebase in 2021. Nucleic Acids Res. (2021) 49:D480–9. doi: 10.1093/nar/gkaa1100
13. Safran M, Dalah I, Alexander J, Rosen N, Iny Stein T, Shmoish M, et al. Genecards version 3: the human gene integrator. Database (Oxford). (2010) 2010:baq020. doi: 10.1093/database/baq020
14. Amberger JS, Bocchini CA, Schiettecatte F, Scott AF, Hamosh A. Omim.org: online mendelian inheritance in man (Omim®), an online catalog of human genes and genetic disorders. Nucleic Acids Res. (2015) 43:D789–98. doi: 10.1093/nar/gku1205
15. Jia A, Xu L, Wang Y. Venn diagrams in bioinformatics. Brief Bioinform. (2021) 22:bbab108. doi: 10.1093/bib/bbab108
16. Shannon P, Markiel A, Ozier O, Baliga NS, Wang JT, Ramage D, et al. Cytoscape: a software environment for integrated models of biomolecular interaction networks. Genome Res. (2003) 13:2498–504. doi: 10.1101/gr.1239303
17. Szklarczyk D, Gable AL, Lyon D, Junge A, Wyder S, Huerta-Cepas J, et al. String V11: protein-protein association networks with increased coverage, supporting functional discovery in genome-wide experimental datasets. Nucleic Acids Res. (2019) 47:D607–13. doi: 10.1093/nar/gky1131
18. Tang Y, Li M, Wang J, Pan Y, Wu FX. Cytonca: a cytoscape plugin for centrality analysis and evaluation of protein interaction networks. Biosystems. (2015) 127:67–72. doi: 10.1016/j.biosystems.2014.11.005
19. Lánczky A, Nagy Á, Bottai G, Munkácsy G, Szabó A, Santarpia L, et al. Mirpower: a web-tool to validate survival-associated mirnas utilizing expression data from 2178 breast cancer patients. Breast Cancer Res Treat. (2016) 160:439–46. doi: 10.1007/s10549-016-4013-7
20. Li T, Fan J, Wang B, Traugh N, Chen Q, Liu JS, et al. Timer: a web server for comprehensive analysis of tumor-infiltrating immune cells. Cancer Res. (2017) 77:e108–10. doi: 10.1158/0008-5472.Can-17-0307
21. Falaguera MJ, Mestres J. Identification of the core chemical structure in surechembl patents. J Chem Inf Model. (2021) 61:2241–7. doi: 10.1021/acs.jcim.1c00151
22. Berman HM, Westbrook J, Feng Z, Gilliland G, Bhat TN, Weissig H, et al. The protein data bank. Nucleic Acids Res. (2000) 28:235–42. doi: 10.1093/nar/28.1.235
23. Morris GM, Huey R, Lindstrom W, Sanner MF, Belew RK, Goodsell DS, et al. Autodock4 and autodocktools4: automated docking with selective receptor flexibility. J Comput Chem. (2009) 30:2785–91. doi: 10.1002/jcc.21256
24. Trott O, Olson AJ. Autodock vina: improving the speed and accuracy of docking with a new scoring function, efficient optimization, and multithreading. Comput Chem. (2010) 31:455-61. doi: 10.1002/jcc.21334
25. Gaillard T. Evaluation of autodock and autodock vina on the casf-2013 benchmark. J Chem Inf Model. (2018) 58:1697–706. doi: 10.1021/acs.jcim.8b00312
26. Li B, Severson E, Pignon JC, Zhao H, Li T, Novak J, et al. Comprehensive analyses of tumor immunity: implications for cancer immunotherapy. Genome Biol. (2016) 17:1–16.
27. Yuan C, Wang MH, Wang F, Chen PY, Ke XG, Yu B, et al. Network pharmacology and molecular docking reveal the mechanism of scopoletin against non-small cell lung cancer. Life Sci. (2021) 270:119105. doi: 10.1016/j.lfs.2021.119105
28. Meza-Valderrama DA-O, Marco E, Dávalos-Yerovi VA-O, Muns MD, Tejero-Sánchez M, Duarte E, et al. Sarcopenia, malnutrition, and cachexia: adapting definitions and terminology of nutritional disorders in older people with cancer. Nutrients. (2021) 13:761. doi: 10.3390/nu13030761
29. Bossi PA-O, Delrio P, Mascheroni A, Zanetti M. The spectrum of malnutrition/cachexia/sarcopenia in oncology according to different cancer types and settings: a narrative review. Nutrients. (2021) 13:1980. doi: 10.3390/nu13061980
30. Li HJ, Zhou RS, Shen M. Professor Zhou Daihan’s contribution to TCM tumor therapeutics. J Tradit Chin Med Oncol. (2019) 1:66–8.
31. Yin Q, Zeng GF, Jiang LS. Analysis of the application characteristics of TCM food therapy for health. Chin J Tradit Chin Med. (2021) 36:2378–80.
32. Barnaba C, Medina-Meza IG. Flavonoids ability to disrupt inflammation mediated by lipid and cholesterol oxidation. Adv Exp Med Biol. (2019) 1161:243–53. doi: 10.1007/978-3-030-21735-8_19
33. Fardoun MM, Maaliki D, Halabi N, Iratni R, Bitto A, Baydoun E, et al. Flavonoids in adipose tissue inflammation and atherosclerosis: one arrow, two targets. Clin Sci. (2020) 134:1403–32. doi: 10.1042/CS20200356
34. Chen T, Li B, Xu Y, Meng S, Wang Y, Jiang Y. Luteolin reduces cancer-induced skeletal and cardiac muscle atrophy in a lewis lung cancer mouse model. Oncol Rep. (2018) 40:1129–37. doi: 10.3892/or.2018.6453
35. Snoke DB, Nishikawa Y, Cole RM, Ni A, Angelotti A, Vodovotz Y, et al. Dietary naringenin preserves insulin sensitivity and grip strength and attenuates inflammation but accelerates weight loss in a mouse model of cancer cachexia. Mol Nutr Food Res. (2021) 65:e2100268. doi: 10.1002/mnfr.202100268
36. Alam W, Khan H, Shah MA, Cauli O, Saso L. Kaempferol as a dietary anti-inflammatory agent: current therapeutic standing. Molecules. (2020) 25:4073. doi: 10.3390/molecules25184073
37. Xiao J, Sun GB, Sun B, Wu Y, He L, Wang X, et al. Kaempferol protects against doxorubicin-induced cardiotoxicity in vivo and in vitro. Toxicology. (2012) 292:53–62. doi: 10.1016/j.tox.2011.11.018
38. Shaulian E, Karin M. Ap-1 as a regulator of cell life and death. Nat Cell Biol. (2002) 4:E131–6.
39. Hakvoort TB, Moerland PD, Frijters R, Sokolović A, Labruyère WT, Vermeulen JL, et al. Interorgan coordination of the murine adaptive response to fasting. J Biol Chem. (2011) 286:16332–43. doi: 10.1074/jbc.M110.216986
40. Cho MK, Kim YG, Lee MG, Kim SG. Prevention of C-jun/activator protein-1 activation and microsomal epoxide hydrolase induction in the rat liver by cysteine during protein-calorie malnutrition. Biochem Pharmacol. (2001) 61:15–24. doi: 10.1016/s0006-2952(00)00518-9
41. Ramamoorthy S, Donohue M, Buck M. Decreased jun-D and myogenin expression in muscle wasting of human cachexia. Am J Physiol Endocrinol Metab. (2009) 297:E392–401. doi: 10.1152/ajpendo.90529.2008
42. Liu Z, Sin KWT, Ding H, Doan HA, Gao S, Miao H, et al. P38β mapk mediates Ulk1-dependent induction of autophagy in skeletal muscle of tumor-bearing mice. Cell Stress. (2018) 2:311–24. doi: 10.15698/cst2018.11.163
43. Sin TK, Zhang G, Zhang Z, Zhu JZ, Zuo Y, Frost JA, et al. Cancer-induced muscle wasting requires P38β Mapk activation of P300. Cancer Res. (2021) 81:885–97. doi: 10.1158/0008-5472.Can-19-3219
44. Baracos VE, Martin L, Korc M, Guttridge DC, Fearon KCH. Cancer-associated cachexia. Nat Rev Dis Primers. (2018) 4:17105. doi: 10.1038/nrdp.2017.105
45. Zimmers TA, Fishel ML, Bonetto A. Stat3 in the systemic inflammation of cancer cachexia. Semin Cell Dev Biol. (2016) 54:28–41. doi: 10.1016/j.semcdb.2016.02.009
46. Wu T, Li X, Ma DF. The role of adipose tissue browning in cachexia fat consumption. Chin J Pathophysiol. (2021) 37:2279–85.
47. Lin YC, Chen LW, Chen YC, Chan ST, Liao JW, Yeh SA-O. Quercetin attenuates cisplatin-induced fat loss. Eur J Nutr. (2021) 60:1781–93. doi: 10.1007/s00394-020-02371-5
48. Assini JM, Mulvihill EF, Huff MW. Citrus flavonoids and lipid metabolism. Curr Opin Lipidol. (2013) 24:34–40.
49. Tian L, Wang S, Jiang S, Liu Z, Wan X, Yang C, et al. Luteolin as an adjuvant effectively enhances Ctl anti-tumor response in B16f10 mouse model. Int Immunopharmacol. (2021) 94:107441. doi: 10.1016/j.intimp.2021.107441
50. Kim JH, Kim YS, Choi JG, Li W, Lee EJ, Park JW, et al. Kaempferol and its glycoside, kaempferol 7-O-rhamnoside, inhibit Pd-1/Pd-L1 interaction in vitro. Int J Mol Sci. (2020) 21:3239. doi: 10.3390/ijms21093239
51. Ford CT, Richardson S, McArdle F, Lotito SB, Crozier A, McArdle A, et al. Identification of (Poly)phenol treatments that modulate the release of pro-inflammatory cytokines by human lymphocytes. Br J Nutr. (2016) 115:1699–710. doi: 10.1017/S0007114516000805
Keywords: shiliao decoction, cancer-associated malnutrition, network pharmacology, molecular docking, immunity, prognosis
Citation: Long S, Ji S, Xue P, Xie H, Ma Y and Zhu S (2022) Network pharmacology and molecular docking analysis reveal insights into the molecular mechanism of shiliao decoction in the treatment of cancer-associated malnutrition. Front. Nutr. 9:985991. doi: 10.3389/fnut.2022.985991
Received: 04 July 2022; Accepted: 08 August 2022;
Published: 25 August 2022.
Edited by:
Qinghua Yao, Cancer Research Institute, ChinaReviewed by:
Hiu Yee Kwan, Hong Kong Baptist University, Hong Kong SAR, ChinaChanggang Sun, Affiliated Hospital of Weifang Medical University, China
Copyright © 2022 Long, Ji, Xue, Xie, Ma and Zhu. This is an open-access article distributed under the terms of the Creative Commons Attribution License (CC BY). The use, distribution or reproduction in other forums is permitted, provided the original author(s) and the copyright owner(s) are credited and that the original publication in this journal is cited, in accordance with accepted academic practice. No use, distribution or reproduction is permitted which does not comply with these terms.
*Correspondence: Yinjie Ma, ZW1tYTU0NTdAMTI2LmNvbQ==; Shijie Zhu, MjAxODA5NDEyMzRAYnVjbS5lZHUuY24=
†These authors share first authorship