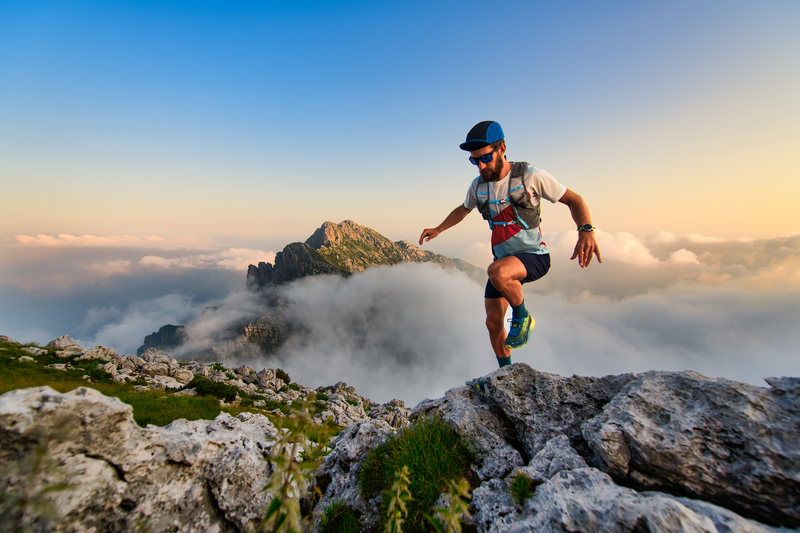
94% of researchers rate our articles as excellent or good
Learn more about the work of our research integrity team to safeguard the quality of each article we publish.
Find out more
ORIGINAL RESEARCH article
Front. Nutr. , 05 October 2022
Sec. Clinical Nutrition
Volume 9 - 2022 | https://doi.org/10.3389/fnut.2022.981338
This article is part of the Research Topic Nutritional Status in Disease: Development, Evaluation, Prognosis and Individualized Treatment in Clinical Practice View all 23 articles
Objective: The prognostic nutritional index (PNI) is an important prognostic factor for survival outcomes in various hematological malignancies. The current study focused on exploring the predictive value of the PNI in newly diagnosed follicular lymphoma (FL) in China.
Materials and methods: The clinical indicators and follow-up data of 176 patients who received chemotherapy or immunotherapy combined with chemotherapy with FL in our hospital from January 2016 to March 2022 were retrospectively analyzed. Cox proportional hazard model was used for univariate and multivariate analyses. Kaplan–Meier curves were used to calculate survival rates and draw survival curves. The log-rank test was applied to compare differences between groups.
Results: The optimal cut-off value of PNI was 44.3. All patients were divided into a high PNI group (>44.3) and a low PNI group (≤44.3). The low PNI group had a low CR rate and a high risk of death, with a tendency toward POD24, and Both OS and PFS were worse than those in the high PNI group. PNI was able to predict OS and PFS in FL patients and was the only independent predictor of OS (P = 0.014 HR 5.024; 95%CI 1.388∼18.178) in multivariate analysis. PNI could re-stratify patients into groups of high FLIPI score, high FLIPI2 score, no POD24, and rituximab combined with chemotherapy. Moreover, integrating PNI into the FLIPI and FLIPI2 models improved the area under the curve (AUC) for more accurate survival prediction and prognosis.
Conclusion: PNI is a significant prognostic indicator for newly diagnosed FL in China that can early identify patients with poor prognosis and guide clinical treatment decisions.
Follicular lymphoma (FL) is a malignant tumor originating from B cells in the follicular center, it manifests mainly as painless enlargement of lymph nodes at an early stage and may include extrasensory organs at an advanced stage (1, 2). The first-line treatment regimen recommended by guidelines is mainly rituximab (R) combined with chemotherapy, followed by R maintenance therapy after induction therapy, with significant improvement in prognosis (3, 4). The course of follicular lymphoma is usually indolent, and most patients can achieve long-term survival. However, FL has high clinical heterogeneity (5, 6), some patients have a poor response to immunochemotherapy, such as early recurrence and progression, histological transformation (7–9). Identifying high risk patients in a timely manner and improving prognosis have become a current problem and hot research topic (10).
The PNI based on serum albumin and lymphocyte counts is an indicator of nutritional status and systemic inflammation, which can assess nutritional status and surgical risk before surgery (11). Currently, the PNI is widely considered to be related to survival outcomes in malignancy (12, 13). A study showed the PNI to be an independent predictor of OS in elderly patients of FL, with low PNI having poor prognosis (14). There are few relevant studies on PNI in the prognosis of patients with FL, and evidence for its prognostic value is still limited.
In this study, we conducted a single-center retrospective analysis to evaluate the prognostic ability of the PNI in newly diagnosed FL in China. We incorporated it into existing clinical outcome models to improve risk stratification and provide a rationale for achieving precise prognostic stratification and individualized treatment of FL.
A retrospective analysis of 176 newly diagnosed FL patients admitted to the First Affiliated Hospital of Zhengzhou University from January 2016 to March 2022 was performed. Inclusion criteria: (1) grade 1-3a FL confirmed by pathology and immunohistochemistry (15); (2) not receiving antitumor therapy before diagnosis; (3) complete medical records and follow-up information. Exclusion criteria: (1) diseases with varying effects on lymphocyte levels and albumin levels, such as chronic kidney disease; (2) mixed components or histological transformation in the pathological tissue at diagnosis (16); (3) other tumor-related diseases. This study was approved by The Ethics Committee of Scientific Research and Clinical Trial the First Affiliated Hospital of Zhengzhou University and followed the Declaration of Helsinki.
Clinical data of FL patients were collected, such as age, sex, Eastern Cooperative Oncology Group Performance Status (ECOG PS) score, Ann Arbor stage, histological grade, B symptoms, serum albumin, lymphocyte count, hemoglobin (Hb), lactate dehydrogenase (LDH), β2-microglobulin (β2-MG) and bone marrow involvement, Ki-67 expression etc. We analyzed the prognostic PNI in patients with FL. The PNI was calculated as follows: PNI = albumin (g/L) + 5 × lymphocyte count (×109/L) (17).
Follow-up was conducted by consulting hospitalization records and telephone interviews until March 2022, from initial diagnosis to follow-up deadline or date of death. Overall survival (OS) was defined as the time from the date of diagnosis to death or last observation for any cause, Progression-free survival (PFS) was defined as that from the date of diagnosis to first relapse, progression or death and date of the last follow-up (18, 19). Progression of disease within 24 months was defined as POD24 (20). Response assessment was defined as Complete response (CR), Partial Response (PR), Stable disease (SD), and Progressive disease (PD) (21).
SPSS 26.0 software was used for statistical analysis. The Kaplan–Meier method was used to draw survival curves, and the log-rank test was performed in parallel. The optimal cut-off value for stratifying PNI was determined by Receiver operating characteristic curve (ROC) analysis (22, 23). Categorical variables were presented as frequencies and percentages. The chi-square test was used for categorical variables. Cox proportional hazard model was used to analyze univariate association between prognostic factors and OS/PFS (24). All variables with P < 0.05 in univariate analysis were retained in multivariate analysis by using forward selection for the best predictor set, and Akaike Information Criteria (AIC) was used to evaluate the model. The PNI was incorporated into the FLIPI and FLIPI2 models, risk grouping was performed again, and the AUC value was used to evaluate the prognostic model. P < 0.05 was considered statistically significant.
We used ROC curves to analyze the PNI and survival of FL patients at initial diagnosis (Figure 1). The AUC was 0.742, and the 95%CI was 0.577∼0.907. The PNI had some accuracy in predicting FL prognosis, and the Jordan index was the largest at a PNI of 44.3, with a sensitivity of 63.6% and specificity of 81.8%. All patients were divided according to the PNI cut-off value into a high PNI group (PNI > 44.3) and a low PNI group (PNI ≤ 44.3).
Among 176 patients, the median age was approximately 50 (19–85) years old, 49 (27.8%) were >60 years old. Of the patients, 75 (42.6%) were males and 101 (57.4%) females. Most patients were diagnosed with advanced disease. Details were as follows: Ann Arbor Stage III-IV in 138 cases (78.4%); grade 3a in 85 cases (48.3%); ECOG PS ≥ 2 in 30 cases (17.0%); 15 cases (8.5%) with B-cell symptoms; bone marrow participation in 84 cases (47.7%); 47 (26.7%) and 32 (18.2%) cases with LDH and β2-MG exceeding the upper limit of normal; 67 cases (38.1%) with Hb <120 g/L; 123 cases (69.9%) with Ki-67 ≥30%; low, intermediate, and high FLIPI score in 49 (27.8%), 50 (28.4%), and 77 (43.8%) cases; low, intermediate, and high FLIPI2 score in 99 (56.3%), 50 (28.4%) and 27 (15.3%) cases. The high PNI group and the low PNI group had different baseline characteristic distributions in age, ECOG PS score, FLIPI score, FLIPI2 score, Hb, and LDH, and the difference was statistically significant (P < 0.05) (Table 1).
Treatment initiation was guided by Groupe d’Etude des Lymphomes Folliculaires (GELF) or National Comprehensive Cancer Network (NCCN) criteria. 155 patients (88.1%) received chemotherapy containing rituximab (R), including RCHOP and RCHOP-like regimens and the RCVP, RFC, and BR. 21 patients (11.9%) received chemotherapy alone, and there was no significant difference in the treatment methods between PNI stratifications (P > 0.05). The difference in first-line treatment response between the two groups was statistically significant (P = 0.017). The high PNI group was more likely to achieve CR than the low PNI group, CR rates were 55.8% vs. 34.2%,and the difference in CR rates was statistically significant (P = 0.018). As of the date of follow-up, 38 patients (21.6%) developed POD24, which was more likely to occur in the low PNI group, with an incidence rate of 34.2% vs. 18.1% in the high PNI group (P < 0.05). Six patients underwent re-biopsy at the time of relapse, and pathological findings involved transformation into diffuse large B-cell lymphoma (DLBCL). Histological transformation (HT) was not significantly different between the two groups (Table 2).
Cox regression was used to analyze FL patient results. The results showed that the PNI, ECOG PS score, B symptoms, FLIPI score, and LDH were risk factors for OS, and the PNI, ECOG PS score, and FLIPI score were risk factors for PFS, with a statistically significant difference (P < 0.05). Univariate analysis of meaningful indicators (P < 0.05) was carried out with a stepwise forward method for multivariate analysis. The PNI was the only independent prognostic risk factor for OS in FL patients, and the ECOG PS score was the only independent prognostic risk factor for PFS (Tables 3, 4).
Among the 176 newly treated FL patients, the median follow-up time was 27 (7–74) months, and 5-year OS and PFS rates were 92.1% and 44.8%, respectively (Figures 2A,B). Kaplan–Meier results showed that OS and PFS were significantly worse in the low PNI group than in the high PNI group (P < 0.05). The 5-year OS rates in the two groups were 73.8% and 97.0%, 5-year PFS in the low PNI group was not reached and the high PNI group was 47.1% (Figures 2C,D). 11 patients (6.3%) died during follow-up with 7 (63.6%) in the low PNI group and 4 (36.4%) in the high PNI group. All died of lymphoma-related comorbidities, with a mortality rate of 18.4% vs. 2.9% in the two groups. Patients with a low PNI had a higher risk of death (P = 0.002).
Figure 2. Overall survival and progression-free survival curves for all patients (A,B) and stratified by the prognostic nutritional index (C,D).
This study showed that PNI was an independent prognostic risk factor for OS in FL patients. Considering the distribution differences between the low and high PNI groups in terms of age, occurrence of POD24, FLIPI score, and first-line treatment regimen, we performed subgroup analysis separately. The results showed that in patients aged ≤60 years and >60 years old, OS of the low PNI group was worse than that of the high PNI group (Figures 3A,B). The PNI was able to stratify the prognosis of patients with high FLIPI scores, and that of patients with low PNI was worse (Figures 3C,D). In the no POD24 group and the R combined with chemotherapy group, patients with low PNI were more likely to have poor prognosis, and there was no difference between the POD24 group and the chemotherapy alone group (Figures 3E–H).
Figure 3. Overall survival according to the prognostic nutritional index and age (A,B), High FLIPI score (C,D), presence or absence of POD24 (E,F) and treatment regimen (G,H).
To evaluate the prognostic ability of the PNI for FL patients, we combined it with the FLIPI score and FLIPI2 score, scoring 1 point for PNI ≤44.3, and then regrouped risk, dividing into three risk categories: low (0–1 points), intermediate (2–3 points), and high (4–6 points) (Table 5). FLIPI-PNI scores were 26.7%, 48.9%, and 24.4% for low, intermediate, and high risks, and FLIPI2-PNI scores were 52.8%, 36.9%, and 10.2%, respectively. Kaplan-Meier curves were generated according to the four scoring systems to estimate the influence of different risk groups on OS (Figures 4A–D). The results showed that in addition to FLIPI2, the prognosis of different risk groups could be distinguished. AUC values for FLIPI-PNI and FLIPI2-PNI were higher than those for FLIPI and FLIPI2 (Figure 5). Incorporating the PNI into the FLIPI score improved AUC and predictive power.
Figure 4. Overall survival for risk groups defined by four scoring systems. (A) FLIPI, (B) FLIPI2, (C) FLIPI-PNI, (D) FLIPI2-PNI.
Follicular lymphoma (FL) is the most common non-Hodgkin’s lymphoma (NHL) in Western countries, accounting for 22 to 25% of NHL (25, 26), with a median OS of nearly 20 years. However, early progression and HT lead to poor prognosis (27), and there is an urgent clinical need for prognostic indicators that can identify high risk patients. It is well known that the nutritional status of the body can lead to dysfunctional immune function, promoting tumor proliferation and progression. The PNI calculated from the serum albumin level and peripheral blood lymphocyte count is now widely considered to be associated with the prognosis of patients with some solid malignant tumors and hematological malignancies (28–30).
In studies on DLBCL, a low PNI was found to be associated with poor baseline characteristics, lower CR rates, and shorter PFS and OS (31). A study in China showed that the PNI was a reliable prognostic factor for NK/T-cell lymphoma and could improve the performance of commonly used scoring models (32). According to a study in HongKong, PNI was shown to be the independent prognostic factor of PFS in FL and was a cheap and widely available biomarker (19). Some studies have also reported that both the serum albumin level and decreased lymphocyte count were associated with poor OS in FL (33). In this study, we used the peripheral blood index obtained before primary treatment of the disease to calculate the PNI and analyze its prognostic value for FL in primary treatment in China.
The PNI cut-off value in this study was 44.3, compared with the broad cut-off value of 45, which was more accurate for predicting survival, with a sensitivity of 63.6% and specificity of 81.8%. Most patients in the low PNI group had risk factors, such as age >60 years, ECOG PS ≥2, high FLIPI score, LDH higher than normal, and Hb < 120 g/L. There was no significant difference in the treatment regimens between the two groups, but response to first-line treatment was comparable. The high PNI group was more likely to achieve CR, the CR rate was 55.8% vs. 34.2% for the low PNI group. This was consistent with previous studies and indicated that better nutritional status at initial treatment was helpful for achieving CR and providing a theoretical basis for improving nutritional status as soon as possible in FL patients prior to treatment (19).
Studies have shown that approximately 20% of FL patients will experience POD24 with poor survival outcomes, and this was considered a significant adverse prognostic factor for FL (34). A summary analysis of data from 13 randomized clinical trials indicated (35) that male sex [odds ratio (OR), 1.30], PS ≥ 2 (OR,1.63), β2-MG (≥ 3 mg/L; OR,1.43), and high risk FLIPI score (3–5; OR,3.14) were associated with an increased risk of POD24, though no clinical and genetic predictive models were available to accurately predict which patients will experience POD24. In this study, we found a correlation between the PNI and POD24, with a total of 38 patients (21.6%) developing POD24 and a higher incidence in the low PNI group (34.2% vs. 18.1%), showing value for early identification and helping clinicians to develop individual treatment strategies for patients with FL to improve survival.
Multivariate analysis showed that PNI was the only independent factor influencing OS in FL patients. OS and PFS in the low PNI group were significantly worse than those in the high PNI group, and patients with a low PNI had a higher risk of death, which was similar to the results of a retrospective analysis (14). Older FL patients may have lower lymphocyte counts and serum albumin concentrations, leading to a low PNI (36, 37). To avoid attributing the poor prognostic effect in the low PNI group to age factors, we performed stratified survival analysis of patients aged ≤60 years old and >60 years old, and the PNI showed prognostic value for OS in both groups. Moreover, the PNI was able to re-stratify the prognostic risk of the highrisk FLIPI score group, no POD24 group, and R combined with chemotherapy group, with patients with a lower PNI usually having poorer prognosis and shorter survival.
Many prognostic indicators based on clinical data, the tumor microenvironment and gene expression could be used to clinically guide FL-related treatment, such as FLIPI and FLIPI2, which predicted OS and PFS in previously untreated FL patients (38, 39). Nevertheless, early progression could not be accurately predicted. Clinical genomic models such as m7-FLIPI and 23-gene signatures were still difficult to implement clinically due to their cost and complexity (40, 41). Our results showed that the PNI had certain predictive value for FL patients in terms of first-line treatment response, early recurrence and progression risk, and survival prognosis. Adding the PNI to the FLIPI scoring model could improve AUC, with better predictive ability. In the era of immunotherapy, PNI may become an important factor for FL prognosis and risk stratification and has broad clinical application prospects.
The advantage of this study was that patients with diseases affecting nutritional status and other tumors were excluded, all patients were treated in the same treatment institution, and follow-up data were complete, which avoided the influence of confounding factors on prognosis to a certain extent. However, the sample size was relatively limited and was a retrospective study. Conclusions still need to be verified using large-sample prospective data.
In conclusion, the PNI calculation method was simple and easy and could be used as a reliable clinical prognostic index for FL patients. Adding it to the well-established clinical risk model FLIPI improved predictive ability and identify high risk patients early. A large number of prospective and multicenter studies are still needed to confirm this conclusion and provide more useful guidance for individualized treatment of FL.
The original contributions presented in this study are included in the article/supplementary material, further inquiries can be directed to the corresponding author.
The studies involving human participants were reviewed and approved by the Clinical and Research Ethics Committee of the First Affiliated Hospital of Zhengzhou University. Written informed consent for participation was not required for this study in accordance with the national legislation and the institutional requirements. Written informed consent was not obtained from the individual(s) for the publication of any potentially identifiable images or data included in this article.
JG, YL, and XZ designed the research. QW, YZ, XK, WW, SQ, HH, ZW, SW, MD, MJD, XW, XF, LZ, MZ, and QC collected and analyzed research data. JG and YL wrote and edited the manuscript. All authors and their respective research teams recruited and followed up the patient, were involved at each stage of manuscript preparation, contributed to the article, and approved the submitted version.
The study was supported by National Natural Science Foundation of China (No. 82070210) and Major Medical Scientific and Technological Project of Henan Province (No. SBGJ202001008).
The authors declare that the research was conducted in the absence of any commercial or financial relationships that could be construed as a potential conflict of interest.
All claims expressed in this article are solely those of the authors and do not necessarily represent those of their affiliated organizations, or those of the publisher, the editors and the reviewers. Any product that may be evaluated in this article, or claim that may be made by its manufacturer, is not guaranteed or endorsed by the publisher.
1. Relander T, Johnson NA, Farinha P, Connors JM, Sehn LH, Gascoyne RD. Prognostic factors in follicular lymphoma. J Clin Oncol. (2010) 28:2902–13. doi: 10.1200/JCO.2009.26.1693
2. Hiddemann W, Cheson BD. How we manage follicular lymphoma. Leukemia. (2014) 28:1388–95. doi: 10.1038/leu.2014.91
3. Dreyling M, Ghielmini M, Rule S, Salles G, Ladetto M, Tonino SH, et al. Newly diagnosed and relapsed follicular lymphoma: ESMO clinical practice guidelines for diagnosis, treatment and follow-up. Ann Oncol. (2021) 32:298–308. doi: 10.1016/j.annonc.2020.11.008
4. Bachy E, Seymour JF, Feugier P, Offner F, Lopez-Guillermo A, Belada D, et al. Sustained progression-free survival benefit of rituximab maintenance in patients with follicular lymphoma: long-term results of the PRIMA study. J Clin Oncol. (2019) 37:2815–24. doi: 10.1200/JCO.19.01073
5. Kumar E, Pickard L, Okosun J. Pathogenesis of follicular lymphoma: genetics to the microenvironment to clinical translation. Br J Haematol. (2021) 194:810–21. doi: 10.1111/bjh.17383
6. Freedman A, Jacobsen E. Follicular lymphoma: 2020 update on diagnosis and management. Am J Hematol. (2020) 95:316–27. doi: 10.1002/ajh.25696
7. Matasar MJ, Luminari S, Barr PM, Barta SK, Danilov AV, Hill BT, et al. Follicular lymphoma: recent and emerging therapies, treatment strategies, and remaining unmet needs. Oncologist. (2019) 24:e1236–50. doi: 10.1634/theoncologist.2019-0138
8. Milpied P, Gandhi AK, Cartron G, Pasqualucci L, Tarte K, Nadel B, et al. Follicular lymphoma dynamics. Adv Immunol. (2021) 150:43–103. doi: 10.1016/bs.ai.2021.05.002
9. Smith S. Transformed lymphoma: what should I do now? Hematol Am Soc Hematol Educ Program. (2020) 2020:306–11. doi: 10.1182/hematology.2020000115
10. Kahl B. High-risk follicular lymphoma: treatment options. Hematol Oncol. (2021) 39(Suppl. 1):94–9. doi: 10.1002/hon.2853
11. Bailon-Cuadrado M, Perez-Saborido B, Sanchez-Gonzalez J, Rodriguez-Lopez M, Velasco-Lopez R, Sarmentero-Prieto CJ, et al. Prognostic nutritional index predicts morbidity after curative surgery for colorectal cancer. Cir Esp. (2019) 97:71–80. doi: 10.1016/j.ciresp.2018.08.015
12. Okadome K, Baba Y, Yagi T, Kiyozumi Y, Ishimoto T, Iwatsuki M, et al. Prognostic nutritional index, tumor-infiltrating lymphocytes, and prognosis in patients with esophageal cancer. Ann Surg. (2020) 271:693–700. doi: 10.1097/SLA.0000000000002985
13. Luan C, Wang F, Wei N, Chen B. Prognostic nutritional index and the prognosis of diffuse large b-cell lymphoma: a meta-analysis. Cancer Cell Int. (2020) 20:455. doi: 10.1186/s12935-020-01535-x
14. Mozas P, Rivero A, Rivas-Delgado A, Nadeu F, Gine E, Delgado J, et al. The prognostic nutritional index (PNI) is an independent predictor of overall survival in older patients with follicular lymphoma. Leuk Lymphoma. (2022) 63:903–10. doi: 10.1080/10428194.2021.2010064
15. Randall C, Fedoriw Y. Pathology and diagnosis of follicular lymphoma and related entities. Pathology. (2020) 52:30–9. doi: 10.1016/j.pathol.2019.09.010
16. Maeshima AM, Taniguchi H, Ida H, Hosoba R, Fujino T, Saito Y, et al. Non-diffuse large B-cell lymphoma transformation from follicular lymphoma: a single-institution study of 19 cases. Hum Pathol. (2020) 102:33–43. doi: 10.1016/j.humpath.2020.06.001
17. Onodera T, Goseki N, Kosaki G. [Prognostic nutritional index in gastrointestinal surgery of malnourished cancer patients]. Nihon Geka Gakkai Zasshi. (1984) 85:1001–5.
18. Gebauer N, Mengler B, Kopelke S, Frydrychowicz A, Furschke A, Hackenbroch C, et al. Prognostic impact of nutritional and inflammation-based risk scores in follicular lymphoma in the era of anti-CD20 targeted treatment strategies. J Cancer Res Clin Oncol. (2022) 148:1789–801. doi: 10.1007/s00432-021-03758-5
19. Lee SF, Ng TY, Wong FCS. The value of prognostic nutritional index in follicular lymphoma. Am J Clin Oncol. (2019) 42:202–7. doi: 10.1097/COC.0000000000000493
20. Moccia AA, Schar S, Hayoz S, Pirosa MC, Taverna C, Novak U, et al. Prognostic value of POD24 validation in follicular lymphoma patients initially treated with chemotherapy-free regimens in a pooled analysis of three randomized trials of the Swiss Group for Clinical Cancer Research (SAKK). Br J Haematol. (2021) 192:1031–4. doi: 10.1111/bjh.17045
21. Cheson BD, Fisher RI, Barrington SF, Cavalli F, Schwartz LH, Zucca E, et al. Recommendations for initial evaluation, staging, and response assessment of Hodgkin and non-Hodgkin lymphoma: the Lugano classification. J Clin Oncol. (2014) 32:3059–68. doi: 10.1200/JCO.2013.54.8800
22. Obuchowski NA, Bullen JA. Receiver operating characteristic (ROC) curves: review of methods with applications in diagnostic medicine. Phys Med Biol. (2018) 63:07TR1. doi: 10.1088/1361-6560/aab4b1
23. Youden WJ. Index for rating diagnostic tests. Cancer. (1950) 3:32–5. doi: 10.1002/1097-014219503:13.0.co;2-3
24. In J, Lee DK. Survival analysis: part II – Applied clinical data analysis. Korean J Anesthesiol. (2019) 72:441–57. doi: 10.4097/kja.19183
25. Dada R. Diagnosis and management of follicular lymphoma: a comprehensive review. Eur J Haematol. (2019) 103:152–63. doi: 10.1111/ejh.13271
26. Horn H, Kohler C, Witzig R, Kreuz M, Leich E, Klapper W, et al. Gene expression profiling reveals a close relationship between follicular lymphoma grade 3A and 3B, but distinct profiles of follicular lymphoma grade 1 and 2. Haematologica. (2018) 103:1182–90. doi: 10.3324/haematol.2017.181024
27. Mozas P, Nadeu F, Rivas-Delgado A, Rivero A, Garrote M, Balague O, et al. Patterns of change in treatment, response, and outcome in patients with follicular lymphoma over the last four decades: a single-center experience. Blood Cancer J. (2020) 10:31. doi: 10.1038/s41408-020-0299-0
28. Soh J, Suzawa K, Shien K, Otani S, Yamamoto H, Okazaki M, et al. Prognostic nutrition index affects the prognosis of patients undergoing trimodality therapy for locally advanced non-small cell lung cancer. Surg Today. (2020) 50:1610–8. doi: 10.1007/s00595-020-02067-7
29. Paydas S, Lacin S, Dogan M, Barista I, Yildiz B, Seydaoglu G, et al. Easier and more explanatory indices by integrating leukocyte lymphocyte ratio (LLR) and prognostic nutritional index (PNI) to IPS systems in cases with classical Hodgkin lymphoma. Leuk Res. (2021) 107:106586. doi: 10.1016/j.leukres.2021.106586
30. Perisa V, Zibar L, Knezovic A, Perisa I, Sincic-Petricevic J, Aurer I. Prognostic nutritional index as a predictor of prognosis in patients with diffuse large B cell lymphoma. Wien Klin Wochenschr. (2017) 129:411–9. doi: 10.1007/s00508-016-1077-7
31. Go SI, Park S, Kang MH, Kim HG, Kim HR, Lee GW. Clinical impact of prognostic nutritional index in diffuse large B cell lymphoma. Ann Hematol. (2019) 98:401–11. doi: 10.1007/s00277-018-3540-1
32. Yao N, Hou Q, Zhang S, Xiao H, Liang Y, Xu X, et al. Prognostic nutritional index, another prognostic factor for extranodal natural killer/T cell lymphoma, nasal type. Front Oncol. (2020) 10:877. doi: 10.3389/fonc.2020.00877
33. Siddiqui M, Ristow K, Markovic SN, Witzig TE, Habermann TM, Colgan JP, et al. Absolute lymphocyte count predicts overall survival in follicular lymphomas. Br J Haematol. (2006) 134:596–601. doi: 10.1111/j.1365-2141.2006.06232.x
34. Casulo C, Byrtek M, Dawson KL, Zhou X, Farber CM, Flowers CR, et al. Early relapse of follicular lymphoma after rituximab plus cyclophosphamide, doxorubicin, vincristine, and prednisone defines patients at high risk for death: an analysis from the National Lymphocare Study. J Clin Oncol. (2015) 33:2516–22. doi: 10.1200/JCO.2014.59.7534
35. Casulo C, Dixon JG, Le-Rademacher J, Hoster E, Hochster HS, Hiddemann W, et al. Validation of POD24 as a robust early clinical end point of poor survival in FL from 5225 patients on 13 clinical trials. Blood. (2022) 139:1684–93. doi: 10.1182/blood.2020010263
36. Valiathan R, Ashman M, Asthana D. Effects of ageing on the immune system: infants to elderly. Scand J Immunol. (2016) 83:255–66. doi: 10.1111/sji.12413
37. Gom I, Fukushima H, Shiraki M, Miwa Y, Ando T, Takai K, et al. Relationship between serum albumin level and aging in community-dwelling self-supported elderly population. J Nutr Sci Vitaminol. (2007) 53:37–42. doi: 10.3177/jnsv.53.37
38. Solal-Celigny P, Roy P, Colombat P, White J, Armitage JO, Arranz-Saez R, et al. Follicular lymphoma international prognostic index. Blood. (2004) 104:1258–65. doi: 10.1182/blood-2003-12-4434
39. Federico M, Bellei M, Marcheselli L, Luminari S, Lopez-Guillermo A, Vitolo U, et al. Follicular lymphoma international prognostic index 2: a new prognostic index for follicular lymphoma developed by the international follicular lymphoma prognostic factor project. J Clin Oncol. (2009) 27:4555–62. doi: 10.1200/JCO.2008.21.3991
40. Pastore A, Jurinovic V, Kridel R, Hoster E, Staiger AM, Szczepanowski M, et al. Integration of gene mutations in risk prognostication for patients receiving first-line immunochemotherapy for follicular lymphoma: a retrospective analysis of a prospective clinical trial and validation in a population-based registry. Lancet Oncol. (2015) 16:1111–22. doi: 10.1016/S1470-2045(15)00169-2
41. Huet S, Tesson B, Jais J-P, Feldman AL, Magnano L, Thomas E, et al. A gene-expression profiling score for prediction of outcome in patients with follicular lymphoma: a retrospective training and validation analysis in three international cohorts. Lancet Oncol. (2018) 19:549–61. doi: 10.1016/S1470-2045(18)30102-5
Keywords: follicular lymphoma, prognostic nutritional index, lymphocyte, albumin, prognosis
Citation: Ge J, Lei Y, Wen Q, Zhang Y, Kong X, Wang W, Qian S, Hou H, Wang Z, Wu S, Dong M, Ding M, Wu X, Feng X, Zhu L, Zhang M, Chen Q and Zhang X (2022) The prognostic nutritional index, an independent predictor of overall survival for newly diagnosed follicular lymphoma in China. Front. Nutr. 9:981338. doi: 10.3389/fnut.2022.981338
Received: 29 June 2022; Accepted: 24 August 2022;
Published: 05 October 2022.
Edited by:
Guangxun Gao, Fourth Military Medical University, ChinaReviewed by:
Jieqiong Liu, Sun Yat-sen Memorial Hospital, ChinaCopyright © 2022 Ge, Lei, Wen, Zhang, Kong, Wang, Qian, Hou, Wang, Wu, Dong, Ding, Wu, Feng, Zhu, Zhang, Chen and Zhang. This is an open-access article distributed under the terms of the Creative Commons Attribution License (CC BY). The use, distribution or reproduction in other forums is permitted, provided the original author(s) and the copyright owner(s) are credited and that the original publication in this journal is cited, in accordance with accepted academic practice. No use, distribution or reproduction is permitted which does not comply with these terms.
*Correspondence: Xudong Zhang, ZmNjemhhbmd4ZEAgenp1LmVkdS5jbg==
†These authors have contributed equally to this work and share first authorship
Disclaimer: All claims expressed in this article are solely those of the authors and do not necessarily represent those of their affiliated organizations, or those of the publisher, the editors and the reviewers. Any product that may be evaluated in this article or claim that may be made by its manufacturer is not guaranteed or endorsed by the publisher.
Research integrity at Frontiers
Learn more about the work of our research integrity team to safeguard the quality of each article we publish.