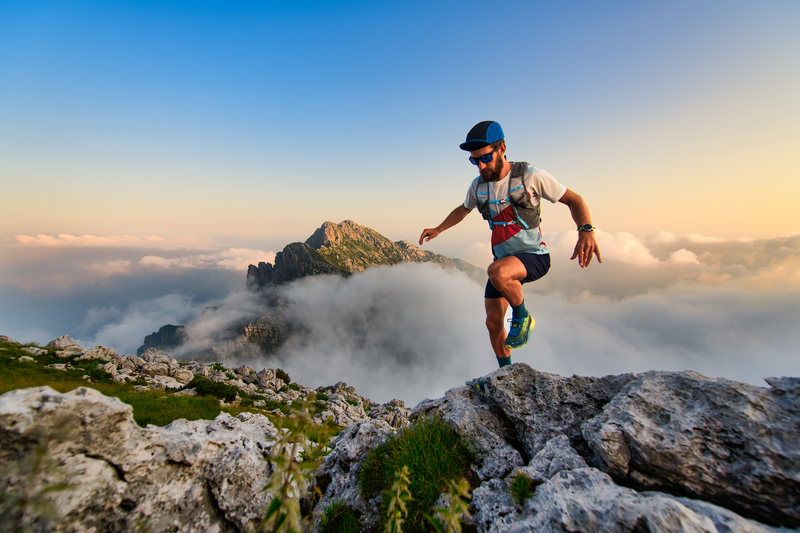
95% of researchers rate our articles as excellent or good
Learn more about the work of our research integrity team to safeguard the quality of each article we publish.
Find out more
ORIGINAL RESEARCH article
Front. Nutr. , 10 November 2022
Sec. Clinical Nutrition
Volume 9 - 2022 | https://doi.org/10.3389/fnut.2022.966518
Background: This study aimed to establish and validate a novel predictive model named integrative inflammatory and nutritional score (IINS) for prognostic prediction in esophageal squamous cell carcinoma (ESCC).
Materials and methods: We retrospectively recruited 494 pathologically confirmed ESCC patients with surgery and randomized them into training (n = 346) or validation group (n = 148). The least absolute shrinkage and selection operator (LASSO) Cox proportional hazards (PH) regression analysis was initially used to construct a novel predictive model of IINS. The clinical features and prognostic factors with hazard ratio (HRs) and 95% confidence intervals (CIs) grouped by IINS were analyzed. Nomogram was also established to verify the prognostic value of IINS.
Results: According to the LASSO Cox PH regression analysis, a novel score of IINS was initially constructed based on 10 inflammatory and nutritional indicators with the optimal cut-off level of 2.35. The areas under the curve (AUCs) of IINS regarding prognostic ability in 1-year, 3-years, and 5-years prediction were 0.814 (95% CI: 0.769–0.854), 0.748 (95% CI: 0.698–0.793), and 0.792 (95% CI: 0.745–0.833) in the training cohort and 0.802 (95% CI: 0.733–0.866), 0.702 (95% CI: 0.621–0.774), and 0.748 (95% CI: 0.670–0.816) in the validation cohort, respectively. IINS had the largest AUCs in the two cohorts compared with other prognostic indicators, indicating a higher predictive ability. A better 5-years cancer-specific survival (CSS) was found in patients with IINS ≤ 2.35 compared with those with IINS > 2.35 in both training cohort (54.3% vs. 11.1%, P < 0.001) and validation cohort (53.7% vs. 18.2%, P < 0.001). The IINS was then confirmed as a useful independent factor (training cohort: HR: 3.000, 95% CI: 2.254–3.992, P < 0.001; validation cohort: HR: 2.609, 95% CI: 1.693–4.020, P < 0.001). Finally, an IINS-based predictive nomogram model was established and validated the CSS prediction (training set: C-index = 0.71 and validation set: C-index = 0.69, respectively).
Conclusion: Preoperative IINS is an independent predictor of CSS in ESCC. The nomogram based on IINS may be used as a potential risk stratification to predict individual CSS and guide treatment in ESCC with radical resection.
As one of the most frequent cancers, esophageal cancer (EC) ranks 10th in cancer incidence (0.60 million new diagnosed cases) and 6th in cancer death (0.54 million died cases) in 2020 based on the global cancer statistics (1). EC [mostly esophageal squamous cell carcinoma, (ESCC)] is one of the most common and aggressive cancers in China, with approximately 0.32 million new cases (6th in incidence) and 0.30 million deaths (4th in mortality) in 2020 (2). Therefore, more than half of world’s new cases and deaths from EC occur in China. Recently, despite great efforts in therapeutic measures, the treatment outcomes for patients with EC remain unsatisfactory (3, 4). The main reasons for poor prognosis are late diagnosis, regional and distant metastasis, treatment resistance and frequent recurrence (5–7). Thus, it is urgent to identify more effective clinical indicators to predict prognosis and improve survival prior to treatment in patients with EC.
Accumulating studies have indicated that nutritional and/or inflammatory status was closely correlated to prognosis in a variety of cancers (8, 9). Recently, preoperative hematological inflammation and nutrition based indicators, such as albumin (ALB), C-reactive protein (CRP), platelet (PLT) to lymphocyte (LYM) ratio (PLR), neutrophil (NEU) to LYM ratio (NLR), and LYM to monocyte (MON) ratio (LMR), have demonstrated their prognostic roles in various cancers (10–13). Moreover, researchers were not satisfied with the single indicator and sought to more and more integrative indicators. A variety of studies published in recent years also revealed that several integrative indicators, such as systemic immune-inflammation index (SII), systemic inflammation response index (SIRI), and prognostic nutritional index (PNI) were prognostic factors for cancer prognosis (14–16). However, the results for these above indexes are still controversial in cancers. Moreover, the hematological inflammation and nutrition based indicators mentioned above may be affected by various other factors.
It is indicated that the combination of these indicators may provide more accurate prognostic values than individual indicators. Moreover, the influence of confounding factors may be reduced by the combination of these indicators. In addition, accurate prognosis prediction may help clinician choose appropriate therapeutic treatments based on risk stratification. Therefore, we initially developed and verified an integrative inflammatory and nutritional score (IINS) by using least absolute shrinkage and selection operator (LASSO) to predict clinical outcomes in ESCC patients.
A series of 672 pathologically confirmed EC patients undergoing radical resection in our institute were retrospectively collected from 2012 to 2014. The inclusion criteria were (1) ESCC histologically confirmed; (2) patients with radical resection (R0 resection); (3) patients with full medical records and follow-up; (4) patients with no preoperative therapy; (5) patients with no acute or chronic infection, autoimmune disease or hematologic disease; and (6) patients with no other previous or synchronous malignancy. As a retrospective study with anonymous data, informed consent was waived. Study approval was obtained from the ethics committee of Zhejiang Cancer Hospital (Number: 2021-5). The study was performed in line with the Declaration of Helsinki.
The main surgical procedure in the present study included McKeown or Ivor Lewis with two or three-field lymphadenectomy (17). The stage in the current study was in accordance with the 8th AJCC/UICC tumor node metastasis (TNM) staging system (18). Neoadjuvant treatments were recommend by the NCCN guidelines for locally advanced ESCC, however, a large number of ESCC patients in China tended to choose surgery as initial treatment (19, 20). Adjuvant therapy was mainly carried out based on the post-operative pathological results (21). Generally speaking, if the post-operative pathology result indicated stage T3 or higher and/or lymph node metastasis, cisplatin-based chemotherapy and/or radiotherapy would be administered (22, 23). Patients were then followed up including physical examinations, tumor markers tests, and contrast CT examinations with regular checks (first 2 years: quarterly; next 2–5 years: semiannually; after 5 years: annually). The last follow-up date for the patients was Dec. 2019.
The clinical data including preoperative hematological indexes were retrospectively collated. The hematological indicators, such as CRP, ALB, NEU, LYM, PLT, NLR, PLR, LMR, hemoglobin (HB), PLT to HB ratio (PHR), NEU to HB ratio (NHR), CRP to HB ratio (CHR), CRP to prealbumin (PALB) ratio (CPR), total protein (TP), globulin (GLO), and so on, were tested within 1 week before the surgery. The other variables (SII, SIRI, and PNI) were calculated by the following formula based on published studies: PNI = ALB (g/L) + 5 × LYM (109/L), SII = PLT × NEU/LYM, and SIRI = MON × NEU/LYM (14–16).
All data were analyzed in the current study by using R software (version 3.6.1), SPSS 20.0 and Medcalc 17.6. The LASSO Cox PH regression analysis was used to select useful indicators. The “maxstat” package of R software was used to classify various inflammatory and nutritional indicators based on the cutoff point determined by the maximally selected rank statistics. The “glmnet” package of R software was performed to identify the most valuable prognostic factors among all candidate hematological inflammatory and nutritional indicators by LASSO Cox PH regression analysis. In order to shrink some regression coefficients to exactly zero, an L1 penalty was set in the LASSO model. Then 10-fold cross-validation with minimum criteria was carried out to find the optimal log (λ). These above hematological inflammatory and nutritional indicators with non-zero coefficients were incorporated to construct the novel score of IINS, which was calculated as follows: IINS = sum (score of every indicator × relevant regression coefficients from LASSO model). The optimum cut-off value for IINS in our study was calculated by the Cutoff Finder1 with the method of survival: significance (log-rank test), which was a straightforward and comprehensive application enabling rapid cutoff optimization (24). According to the Medcalc 17.6 statistical software with DeLong’s methods, the areas under the curve (AUCs) and the 95% confidence intervals (CIs) between IINS and SIRI, SII, and PNI were compared by the receiver operating characteristic (ROC) curves (25, 26). Independent prognostic factors for cancer-specific survival (CSS) were identified by Cox PH regression analyses with hazard ratio (HRs) and 95% CIs. The curves of CSS were calculated by using Kaplan–Meier method. The CSS was defined as the time between surgery and death resulting from the primary cancer according to previous published study (27). Finally, a novel nomogram model was also established to verify the prognostic value of independent indicators. Subsequently, the predictive accuracy and discriminative ability were assessed by the calibration curves, ROC curves and decision curve analyses (DCA). A two-side P-value < 0.05 was considered to be statistically significant.
A total of 494 patients were enrolled in the current study (training set: n = 346 and validation set: n = 148) (Figure 1). There was no statistically significant difference in mean age between the two groups (training cohort: 59.1 ± 7.5 years and validation cohort: 60.0 ± 6.6 years; P = 0.178). However, there was a sex difference between the two groups (P = 0.033). Regarding to the inflammatory and nutritional indexes, the values of TP were significant higher in the training set than those in validation set (7.21 ± 0.53 g/dL vs. 7.09 ± 0.52 g/dL, P = 0.019), while the values of PALB were significant lower in training group than those in the validation group (256.9 ± 64.2 mg/L vs. 270.5 ± 64.1 mg/L, P = 0.031). The baseline clinicopathologic features between these two groups were displayed in Table 1.
Figure 1. The flow diagram of selection esophageal squamous cell carcinoma (ESCC) patients with radical resection. Based on the inclusion and exclusion criteria, a total of 494 patients were randomly included.
The process diagram of IINS was shown in Figure 2A. The correlation of heat map for 23 inflammatory and nutritional indicators was shown in Figure 2B. According to the LASSO Cox PH regression model, 10 indicators including HB, PHR, NHR, CHR, PLR, NLR, LMR, CRP, CPR, and GLO were selected out of 23 inflammatory and nutritional indexes (Figures 2C,D). Finally, the IINS = –0.0053 × HB + 0.0895 × PHR + 2.4830 × NHR + 0.4486 × CHR + 0.0027 × PLR + 0.0569 × NLR + 0.1849 × LMR + 0.0094 × CRP + 4.4222 × CPR + 0.4335 × GLO.
Figure 2. Process diagram for integrative inflammatory and nutritional score (IINS) construction and risk stratification. IINS constructed with 10 indicators out of 23 variables by least absolute shrinkage and selection operator (LASSO) Cox proportional hazards (PH) regression model (A). A correlation matrix is represented regarding 23 indicators (B). LASSO coefficient profiles of the 23 indicators (C). 10-fold cross–validation for tuning parameter selection in the LASSO model (D).
To better understand the predictive ability between IINS and other conventional established prognostic scores, AUC comparisons in different time points (1-year, 3-years, and 5-years) were compared between IINS and other conventional indexes (SIRI, SII, and PNI) (Figure 3). The AUCs of IINS regarding prognostic ability in 1-year, 3-years, and 5-years prediction were 0.814 (95% CI: 0.769–0.854), 0.748 (95% CI: 0.698–0.793), and 0.792 (95% CI: 0.745–0.833) in the training cohort and 0.802 (95% CI: 0.733–0.866), 0.702 (95% CI: 0.621–0.774), and 0.748 (95% CI: 0.670–0.816) in the validation cohort, respectively. Therefore, IINS had the largest AUCs in the training cohort and validation cohort compared with other prognostic indicators (SIRI, PNI, and SII), indicating a higher predictive ability.
Figure 3. Areas under the curve (AUC) comparisons in different time points between integrative inflammatory and nutritional score (IINS) and other variables. AUC comparisons in different time points were compared between IINS and other conventional indexes [systemic inflammation response index (SIRI), systemic immune-inflammation index (SII), and prognostic nutritional index (PNI)]. The AUCs of IINS regarding prognostic ability in 1-year, 3-years, and 5-years prediction were 0.814 (A), 0.748 (B), and 0.792 (C) in the training cohort and 0.802 (D), 0.702 (E), and 0.748 (F) in the validation cohort, respectively.
According to the cutoff finder and ROC curve, the cut-off level of IINS in the training set was 2.35 (Figure 4). Patients were divided into two groups for further analysis with the cut-off value of 2.35. IINS was significantly associated with vessel and perineural invasion, TNM stage, SIRI, PNI, and SII in both training cohort and validation cohort. Tumor length was significantly associated with IINS in the training set (P < 0.001), but not in the validation set (P = 0.095) (Table 2). In addition, the values regarding IINS grouped by TNM stage, SIRI, PNI, and SII in these two groups were also displayed in Figure 5.
Figure 4. The optimal cutoff value achieved for integrative inflammatory and nutritional score (IINS). Distribution for IINS based cutoff optimization (A). A score of 2.35 was chosen as the optimal cutoff point by receiver operating characteristic (ROC) (B). Cutoff optimization by correlation with cancer-specific survival (CSS) prediction (C). Waterfall plot for IINS (D).
Table 2. Comparison of baseline characteristics based on integrative inflammatory and nutritional score (IINS) in training and validation sets.
Figure 5. The violin plots of integrative inflammatory and nutritional score (IINS) values. The violin plots of IINS grouped by tumor node metastasis (TNM), prognostic nutritional index (PNI), systemic immune-inflammation index (SII), and systemic inflammation response index (SIRI) in both training cohort (A–D) and validation cohort (E–H).
A better 5-years CSS was found in patients with IINS ≤ 2.35 compared with those with IINS > 2.35 in both training cohort (54.3% vs. 11.1%, P < 0.001) and validation cohort (53.7% vs. 18.2%, P < 0.001) (Figure 6). Subgroup analyses suggested that IINS (training or validation cohort) had reliable abilities to predict prognosis in resected ESCC patients in any TNM stages (Figure 7). The IINS was confirmed as a useful independent factor [training set: (HR 3.000, 95% CI 2.254–3.992, P < 0.001); validation set: HR 2.609, 95% CI 1.693–4.020, P < 0.001] (Tables 3, 4).
Figure 6. Cancer-specific survival (CSS) analyses grouped by integrative inflammatory and nutritional score (IINS). Kaplan–Meier curves regarding 5-years CSS in the training cohort (A) and validation cohort (B).
Figure 7. Cancer-specific survival (CSS) analyses grouped by tumor node metastasis (TNM) stages. Subgroup analyses based on TNM stage in the training cohort (A–C) and validation cohort (D–F).
Table 3. Univariate and multivariate Cox analyses of cancer-specific survival (CSS) in training set.
Table 4. Univariate and multivariate Cox analyses of cancer-specific survival (CSS) in validation set.
A nomogram model (training set: C-index = 0.71 and validation set: C-index = 0.69) based on IINS and TNM stage, two independent significant variables in multivariate analyses, was established to predict individual CSS in ESCC (Figure 8A). An acceptable agreement was represented in calibration curves for 5-years CSS in both two cohorts (Figures 8B,C). Compared with traditional TNM stages, the predictive accuracy and discriminative ability for ROC (Figures 8D,E) and DCA (Figures 8F,G) was much better in both two cohorts. All these results in our study indicated that the current nomogram model based on IINS showed excellent risk stratification and prognostic accuracy.
Figure 8. Nomogram established and validated. Nomogram established based on integrative inflammatory and nutritional score (IINS) and tumor node metastasis (TNM) (A). Calibration curves for 5-years cancer-specific survival (CSS) prediction in the training cohort (B) and validation cohort (C). Receiver operating characteristic (ROC) analyses of CSS prediction in the training cohort (D) and validation cohort (E). Decision curve analyses (DCA) revealed nomogram model in the training cohort (F) and validation cohort (G). TNM 1/2/3, stage I/II/III; IINS 0/1, ≤2.35/>2.35.
In this study, we initial developed and verified a novel prognostic score of IINS based on various preoperative hematological indexes in patients with ESCC. Our results confirmed the prognostic effect of IINS as a novel indicator in patients with ESCC. IINS had the largest AUCs both in the training cohort and validation cohort compared with other indicators, indicating a higher predictive ability for prognostic prediction in ESCC. Subsequently, a novel predictive nomogram based on two variables (IINS and TNM) was firstly established and validated, showing excellent risk stratification and prognostic accuracy.
To date, determining the prognosis of ESCC patients before treatment has been a challenge. Therefore, it is very important to identify the prognostic value of the clinical characteristics as well as various hematological indicators in cancers. Based on these prognostic analyses, clinicians may make their further risk stratification and formulate individualized therapeutic strategy. It is indicated in several studies that inflammation and nutrition is associated with cancer prognosis (10–16). Inflammatory and nutritional status is closely associated with carcinogenesis, cancer growth and progression (8, 9). Moreover, prognostic values of several preoperative inflammatory and nutritional indicators have been explored in ESCC patients in the past few years (13, 14, 16). A meta-analysis conducted by Ishibashi et al. (13) aimed to explore the correlations between PLT associated markers and overall survival (OS) in ESCC. Based on 14 retrospective studies, they demonstrated that low PLR level was significantly associated with well OS. Fujiwara et al. (14) analyzed several indexes based on inflammation and nutrition in 111 ESCC patients and the results revealed low PNI was associated with shorter recurrence-free survival (RFS). Geng et al. (16) revealed that SIRI was significantly related to OS and served as an independent prognostic score in 916 ESCC patients with radical resection.
In fact, a single inflammatory and nutritional indicator has some limitations and can’t fully reflect the overall status of inflammation and nutrition (28). Moreover, most of published studies incorporated these inflammatory and nutritional indicators in multivariate analyses to explore independent prognostic factors (14–16), but ignore the strong collinearity and correlation between these indicators, causing variables interference and some statistical problems. In addition, an integrated prognostic indicator consisting of multiple dimensions may help to reflect the real and complicated inflammatory and nutritional status (29). In the face of so many features, it is very important to eliminate over-fitting in feature selection (30, 31). Published studies revealed that over-fitting might be solved by applying bootstrapping technique and LASSO Cox PH regression analysis (32). Recently, several studies established some prognostic models with a variety of hematological indicators by LASSO regression. Wang et al. (33) established an inflammatory and nutritional prognostic score (INPS) based on 15 hematological indexes in 513 stage III gastric cancer. They confirmed that INPS was a prognostic factor. They also conducted a nomogram based on INPS for survival prediction in resected stage III gastric cancer with adjuvant chemotherapy. Hua et al. (34) also validated the prognostic value of INPS by LASSO regression analysis in 1,259 patients with early stage breast cancer. In another study including 334 patients with limited-stage small cell lung cancer, the authors also indicated that several hematological nutritional and inflammatory indexes were associated with OS (35). This study conducted an integrated score by LASSO Cox PH regression model to effectively select valuable variables, reducing the influence of multi-collinearity to some extent.
There were several possible mechanisms to illustrate the relationships between cancer and inflammation-nutrition. Most published studies revealed that various inflammatory markers, such as NEU, MON, LYM, PLT, and CRP, play a very important role in promoting metastasis of cancer cells, increasing vascular proliferation and permeability, regulating cancer progression and metastasis, promoting immune surveillance, and antitumor immune response (36–39). The mechanism regarding nutritional markers, such as HB, ALB, GLO, and PALB, in cancer include regulating hypoxia of cancer cells, stimulating cancer growth and progression, activating a variety of cytokines, such as tumor necrosis factor-α and interleukin-1, and increasing the resistance to chemotherapy and/or radiotherapy (40–42).
Nomogram is considered to be a reliable tool for integrating and quantifying significant risk factors for cancer prognosis (43). Nomogram, as a prognostic statistical model, can not only visually display the relevant indicators affecting the outcome in Cox PH regression analyses but also predict the survival probability through a simple graphical representation, which makes the prediction more simple and convenient (44). Wang et al. (33) and Hua et al. (34) both established a nomogram based on INPS to predict prognosis. They also revealed that the INPS-based prognostic nomogram showed a good prognostic stratification. Mao et al. (45) developed and verified a nomogram in hepatocellular carcinoma patients after surgical resection. They indicated that nomogram model may guide clinical prediction, personalized treatment approach and prognosis. In the current study, a novel predictive nomogram based on IINS and TNM was firstly performed and validated in ESCC, which showed excellent risk stratification and prognostic accuracy.
Our study had some limitations that should be noticed. Firstly, this was a single-center retrospective study. So there may be potential data collection bias. As a retrospective design, all data in the current study were observational statistics and were subject to selection bias inherent in non-randomized retrospective designs (46, 47). It is possible that some cases (particularly for those without full medical records and/or follow-up time) could have been missed. Moreover, although we have excluded immune diseases, hematological diseases, and inflammatory diseases, other diseases such as essential hypertension and diabetes mellitus might also affect these blood indicators (48, 49). Secondly, other factors, such as genomics and tumor biomarkers, and coagulation indicators, influencing the ESCC prognosis were not included in the construction of IINS. Thirdly, hematological inflammatory and nutritional variables may be affected by other factors and the results may be changed. Fourthly, the results may be biased due to the lack of additional independent external validation cohort. Fifthly, the exact mechanisms regarding inflammation and nutrition in cancer prognosis require further explore. Sixthly, it would be more convincing if the follow-up time could be updated to the present. However, patient follow-up is a long-term and onerous task, which requires a lot of manpower and material resources, so we can’t get the latest follow-up data in a short time. Last but not least, the prognostic value of IINS needs to be confirmed in various other cancers. Although the above limitations existed, our developed IINS-based nomogram may be used as a potential risk stratification to predict individual CSS in ESCC.
In summary, the preoperative IINS is an independent predictor of CSS in ESCC patients with radical resection. The nomogram based on IINS may be used as a potential risk stratification to predict individual CSS and guide treatment in ESCC with radical resection.
The original contributions presented in the study are included in the article/supplementary material, further inquiries can be directed to the corresponding authors.
The studies involving human participants were reviewed and approved by the study was performed in line with the Declaration of Helsinki. As a retrospective study with anonymous data, informed consent was waived. Study approval was obtained from the Ethics Committee of Zhejiang Cancer Hospital (Number: 2021-5). Written informed consent for participation was not required for this study in accordance with the national legislation and the institutional requirements.
JF, QC, and XC contributed to the study design and prepared for the manuscript. LW and XY contributed to the data collect. JF, LW, and XY contributed to the statistical analysis. All authors approved the final manuscript as submitted and agreed to be accountable for all aspects of the work.
This study was supported by the grants from Zhejiang Medical and Health Science and Technology Project (2017KY237, 2018KY022, and 2019RC129). This study was also supported by the Zhejiang TCM Science and Technology Project (2020ZB036, 2021ZB034, and 2022ZB051).
The authors declare that the research was conducted in the absence of any commercial or financial relationships that could be construed as a potential conflict of interest.
All claims expressed in this article are solely those of the authors and do not necessarily represent those of their affiliated organizations, or those of the publisher, the editors and the reviewers. Any product that may be evaluated in this article, or claim that may be made by its manufacturer, is not guaranteed or endorsed by the publisher.
1. Sung H, Ferlay J, Siegel RL, Laversanne M, Soerjomataram I, Jemal A, et al. Global cancer statistics 2020: GLOBOCAN estimates of incidence and mortality worldwide for 36 cancers in 185 countries. CA Cancer J Clin. (2021) 71:209–49. doi: 10.3322/caac.21660
2. Cao W, Chen HD, Yu YW, Li N, Chen WQ. Changing profiles of cancer burden worldwide and in China: a secondary analysis of the global cancer statistics 2020. Chin Med J. (2021) 134:783–91. doi: 10.1097/CM9.0000000000001474
3. Liu X, Guo W, Shi X, Ke Y, Li Y, Pan S, et al. Construction and verification of prognostic nomogram for early onset esophageal cancer. Bosn J Basic Med Sci. (2021) 21:760–72. doi: 10.17305/bjbms.2021.5533
4. Wang S, Pan D, Chen Z, Song G, Han R, Sun G, et al. Trends in incidence and mortality of esophageal cancer in Huai’an district, a high-risk area in northern Jiangsu province China. Cancer Control. (2022) 29:10732748221076824. doi: 10.1177/10732748221076824
5. Reichenbach ZW, Murray MG, Saxena R, Farkas D, Karassik EG, Klochkova A, et al. Clinical and translational advances in esophageal squamous cell carcinoma. Adv Cancer Res. (2019) 144:95–135. doi: 10.1016/bs.acr.2019.05.004
6. Huang FL, Yu SJ. Esophageal cancer: risk factors, genetic association, and treatment. Asian J Surg. (2018) 41:210–5. doi: 10.1016/j.asjsur.2016.10.005
7. Domper Arnal MJ, Ferrández Arenas Á, Lanas Arbeloa Á. Esophageal cancer: risk factors, screening and endoscopic treatment in Western and Eastern countries. World J Gastroenterol. (2015) 21:7933–43. doi: 10.3748/wjg.v21.i26.7933
8. Michels N, van Aart C, Morisse J, Mullee A, Huybrechts I. Chronic inflammation toward cancer incidence: a systematic review and meta-analysis of epidemiological studies. Crit Rev Oncol Hematol. (2021) 157:103177. doi: 10.1016/j.critrevonc.2020.103177
9. Ryan AM, Sullivan ES. Impact of musculoskeletal degradation on cancer outcomes and strategies for management in clinical practice. Proc Nutr Soc. (2021) 80:73–91. doi: 10.1017/S0029665120007855
10. Komura N, Mabuchi S, Shimura K, Kawano M, Matsumoto Y, Kimura T. Significance of pretreatment C-reactive protein, albumin, and C-reactive protein to albumin ratio in predicting poor prognosis in epithelial ovarian cancer patients. Nutr Cancer. (2021) 73:1357–64. doi: 10.1080/01635581.2020.1798479
11. Yamamoto T, Kawada K, Obama K. Inflammation-related biomarkers for the prediction of prognosis in colorectal cancer patients. Int J Mol Sci. (2021) 22:8002. doi: 10.3390/ijms22158002
12. Stefaniuk P, Szymczyk A, Podhorecka M. The neutrophil to lymphocyte and lymphocyte to monocyte ratios as new prognostic factors in hematological malignancies – a narrative review. Cancer Manag Res. (2020) 12:2961–77. doi: 10.2147/CMAR.S245928
13. Ishibashi Y, Tsujimoto H, Sugasawa H, Kouzu K, Itazaki Y, Sugihara T, et al. Prognostic value of platelet-related measures for overall survival in esophageal squamous cell carcinoma: a systematic review and meta-analysis. Crit Rev Oncol Hematol. (2021) 164:103427. doi: 10.1016/j.critrevonc.2021.103427
14. Fujiwara Y, Higashida M, Kubota H, Okamoto Y, Mineta S, Endo S, et al. Perioperative predictive markers for recurrence of esophageal cancer after esophagectomy. Gastrointest Tumors. (2021) 8:87–95. doi: 10.1159/000513961
15. Zhang W, Wang R, Ma W, Wu Y, Maskey N, Guo Y, et al. Systemic immune-inflammation index predicts prognosis of bladder cancer patients after radical cystectomy. Ann Transl Med. (2019) 7:431. doi: 10.21037/atm.2019.09.02
16. Geng Y, Zhu D, Wu C, Wu J, Wang Q, Li R, et al. A novel systemic inflammation response index (SIRI) for predicting post-operative survival of patients with esophageal squamous cell carcinoma. Int Immunopharmacol. (2018) 65:503–10. doi: 10.1016/j.intimp.2018.10.002
17. Chen D, Hu Y, Chen Y, Hu J, Wen Z. Comparison of outcomes between McKeown and Sweet esophagectomy in the elderly patients for esophageal squamous cell carcinoma: a propensity score-matched analysis. Cancer Control. (2020) 27:1073274820904700. doi: 10.1177/1073274820904700
18. Rice TW, Ishwaran H, Hofstetter WL, Kelsen DP, Apperson-Hansen C, Blackstone EH, et al. Recommendations for pathologic staging (pTNM) of cancer of the esophagus and esophagogastric junction for the 8th edition AJCC/UICC staging manuals. Dis Esophagus. (2016) 29:897–905. doi: 10.1111/dote.12533
19. Qiu ML, Lin JB, Li X, Luo RG, Liu B, Lin JW. Current state of esophageal cancer surgery in China: a national database analysis. BMC Cancer. (2019) 19:1064. doi: 10.1186/s12885-019-6191-2
20. Xu QL, Li H, Zhu YJ, Xu G. The treatments and post-operative complications of esophageal cancer: a review. J Cardiothorac Surg. (2020) 15:163. doi: 10.1186/s13019-020-01202-2
21. Lee Y, Samarasinghe Y, Lee MH, Thiru L, Shargall Y, Finley C, et al. Role of adjuvant therapy in esophageal cancer patients after neoadjuvant therapy and esophagectomy: a systematic review and Meta-analysis. Ann Surg. (2022) 275:91–8. doi: 10.1097/SLA.0000000000005227
22. Semenkovich TR, Subramanian M, Yan Y, Hofstetter WL, Correa AM, Cassivi SD, et al. Adjuvant therapy for node-positive esophageal cancer after induction and surgery: a multisite study. Ann Thorac Surg. (2019) 108:828–36. doi: 10.1016/j.athoracsur.2019.04.099
23. Li L, Zhao L, Lin B, Su H, Su M, Xie D, et al. Adjuvant therapeutic modalities following three-field lymph node dissection for stage II/III esophageal squamous cell carcinoma. J Cancer. (2017) 8:2051–9. doi: 10.7150/jca.18981
24. Budczies J, Klauschen F, Sinn BV, Gyõrffy B, Schmitt WD, Darb-Esfahani S, et al. Cutoff finder: a comprehensive and straightforward Web application enabling rapid biomarker cutoff optimization. PLoS One. (2012) 7:e51862. doi: 10.1371/journal.pone.0051862
25. DeLong ER, DeLong DM, Clarke-Pearson DL. Comparing the areas under two or more correlated receiver operating characteristic curves: a nonparametric approach. Biometrics. (1988) 44:837–45. doi: 10.2307/2531595
26. Cho H, Matthews GJ, Harel O. Confidence intervals for the area under the receiver operating characteristic curve in the presence of ignorable missing data. Int Stat Rev. (2019) 87:152–77. doi: 10.1111/insr.12277
27. Huang Y, Feng JF. Low preoperative lymphocyte to monocyte ratio predicts poor cancer-specific survival in patients with esophageal squamous cell carcinoma. Onco Targets Ther. (2015) 8:137–45. doi: 10.2147/OTT.S73794
28. Feng JF, Wang L, Yang X. The preoperative hemoglobin, albumin, lymphocyte and platelet (HALP) score is a useful predictor in patients with resectable esophageal squamous cell carcinoma. Bosn J Basic Med Sci. (2021) 21:773–81. doi: 10.17305/bjbms.2021.5666
29. Pang H, Zhang W, Liang X, Zhang Z, Chen X, Zhao L, et al. Prognostic score system using preoperative inflammatory, nutritional and tumor markers to predict prognosis for gastric cancer: a two-center cohort study. Adv Ther. (2021) 38:4917–34. doi: 10.1007/s12325-021-01870-z
30. Piles M, Bergsma R, Gianola D, Gilbert H, Tusell L. Feature selection stability and accuracy of prediction models for genomic prediction of residual feed intake in pigs using machine learning. Front Genet. (2021) 12:611506. doi: 10.3389/fgene.2021.611506
31. Barry A, Li W, Becerra JA, Gilabert PL. Comparison of feature selection techniques for power amplifier behavioral modeling and digital predistortion linearization. Sensors. (2021) 21:5772. doi: 10.3390/s21175772
32. Collignon O, Han J, An H, Oh S, Lee Y. Comparison of the modified unbounded penalty and the LASSO to select predictive genes of response to chemotherapy in breast cancer. PLoS One. (2018) 13:e0204897. doi: 10.1371/journal.pone.0204897
33. Wang N, Xi W, Lu S, Jiang J, Wang C, Zhu Z, et al. A novel inflammatory-nutritional prognostic scoring system for stage III gastric cancer patients with radical gastrectomy followed by adjuvant chemotherapy. Front Oncol. (2021) 11:650562. doi: 10.3389/fonc.2021.650562
34. Hua X, Duan F, Zhai W, Song C, Jiang C, Wang L, et al. A novel inflammatory-nutritional prognostic scoring system for patients with early stage breast cancer. J Inflamm Res. (2022) 15:381–94. doi: 10.2147/JIR.S338421
35. Qi J, Zhang J, Ge X, Wang X, Xu L, Liu N, et al. The addition of peripheral blood inflammatory indexes to nomogram improves the predictive accuracy of survival in limited-stage small cell lung cancer patients. Front Oncol. (2021) 11:713014. doi: 10.3389/fonc.2021.713014
36. Kim SH, Lee HW, Go SI, Lee SI, Lee GW. Clinical significance of the preoperative platelet count and platelet-to-lymphocyte ratio (PLT-PLR) in patients with surgically resected non-small cell lung cancer. Oncotarget. (2016) 7:36198–206. doi: 10.18632/oncotarget.8809
37. Bambace NM, Holmes CE. The platelet contribution to cancer progression. J Thomb Haemost. (2011) 9:237–49. doi: 10.1111/j.1538-7836.2010.04131.x
38. Germano G, Allavena P, Mantovani A. Cytokines as a key component of cancer-related inflammation. Cytokine. (2008) 43:374–9. doi: 10.1016/j.cyto.2008.07.014
39. Tsai CY, Hsieh SC, Liu CW, Lu CS, Wu CH, Liao HT, et al. Cross-talk among polymorphonuclear neutrophils, immune, and non-immune cells via released cytokines, granule proteins, microvesicles, and neutrophil extracellular trap formation: a novel concept of biology and pathobiology for neutrophils. Int J Mol Sci. (2021) 22:3119. doi: 10.3390/ijms22063119
40. Chojkier M. Inhibition of albumin synthesis in chronic diseases: molecular mechanisms. J Clin Gastroenterol. (2005) 39:S143–6. doi: 10.1097/01.mcg.0000155514.17715.39
41. Wu W, Yang Q, Li T, Zhang P, Zhou R, Yang C. Hemoglobin-based oxygen carriers combined with anticancer drugs may enhance sensitivity of radiotherapy and chemotherapy to solid tumors. Artif Cells Blood Substit Immobil Biotechnol. (2009) 37:163–5. doi: 10.1080/10731190903043218
42. Jin W, Zhu M, Zheng Y, Wu Y, Ding X, Wu H, et al. Perineural invasion, lactate dehydrogenase, globulin, and serum sodium predicting occult metastasis in oral cancer. Oral Dis. (2022) 28:132–41. doi: 10.1111/odi.13750
43. Li S, Zhao Z, Yang H, Wang D, Sun W, Li S, et al. Construction and validation of a nomogram for the preoperative prediction of lymph node metastasis in gastric cancer. Cancer Control. (2021) 28:10732748211027160. doi: 10.1177/10732748211027160
44. Balachandran VP, Gonen M, Smith JJ, DeMatteo RP. Nomograms in oncology: more than meets the eye. Lancet Oncol. (2015) 16:e173–80. doi: 10.1016/S1470-2045(14)71116-7
45. Mao S, Yu X, Sun J, Yang Y, Shan Y, Sun J, et al. Development of nomogram models of inflammatory markers based on clinical database to predict prognosis for hepatocellular carcinoma after surgical resection. BMC Cancer. (2022) 22:249. doi: 10.1186/s12885-022-09345-2
46. Tripepi G, Jager KJ, Dekker FW, Zoccali C. Selection bias and information bias in clinical research. Nephron Clin Pract. (2010) 115:c94–9. doi: 10.1159/000312871
47. Geneletti S, Richardson S, Best N. Adjusting for selection bias in retrospective, case-control studies. Biostatistics. (2009) 10:17–31. doi: 10.1093/biostatistics/kxn010
48. Zhang C, Chen H, Cui S, Lin Y, Liang Y, Zhao P, et al. Platelet-lymphocyte ratio, neutrophil-lymphocyte ratio and their dynamic changes with type 2 diabetes mellitus: a cohort study in China. Endocr Res. (2022) 47:138–52. doi: 10.1080/07435800.2022.2127757
Keywords: least absolute shrinkage and selection operator (LASSO), cancer-specific survival (CSS), esophageal squamous cell carcinoma (ESCC), prognosis, integrative inflammatory and nutritional score
Citation: Feng J, Wang L, Yang X, Chen Q and Cheng X (2022) Prognostic prediction by a novel integrative inflammatory and nutritional score based on least absolute shrinkage and selection operator in esophageal squamous cell carcinoma. Front. Nutr. 9:966518. doi: 10.3389/fnut.2022.966518
Received: 11 June 2022; Accepted: 25 October 2022;
Published: 10 November 2022.
Edited by:
Clelia Madeddu, University of Cagliari, ItalyCopyright © 2022 Feng, Wang, Yang, Chen and Cheng. This is an open-access article distributed under the terms of the Creative Commons Attribution License (CC BY). The use, distribution or reproduction in other forums is permitted, provided the original author(s) and the copyright owner(s) are credited and that the original publication in this journal is cited, in accordance with accepted academic practice. No use, distribution or reproduction is permitted which does not comply with these terms.
*Correspondence: Qixun Chen, Y2hlbnFpeEB5ZWFoLm5ldA==; Xiangdong Cheng, Y2hlbmd4ZDUxNkAxMjYuY29t
Disclaimer: All claims expressed in this article are solely those of the authors and do not necessarily represent those of their affiliated organizations, or those of the publisher, the editors and the reviewers. Any product that may be evaluated in this article or claim that may be made by its manufacturer is not guaranteed or endorsed by the publisher.
Research integrity at Frontiers
Learn more about the work of our research integrity team to safeguard the quality of each article we publish.