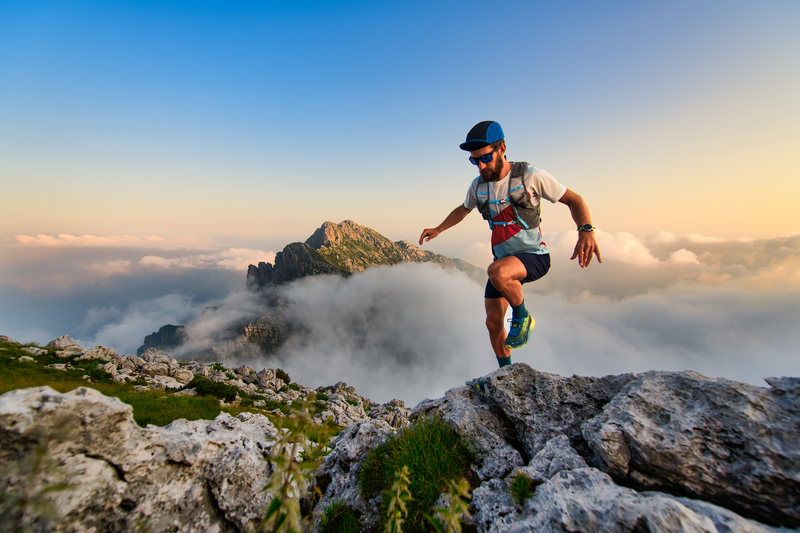
94% of researchers rate our articles as excellent or good
Learn more about the work of our research integrity team to safeguard the quality of each article we publish.
Find out more
ORIGINAL RESEARCH article
Front. Nutr. , 08 September 2022
Sec. Nutritional Epidemiology
Volume 9 - 2022 | https://doi.org/10.3389/fnut.2022.950338
This article is part of the Research Topic Dietary and Nutritional Indices and Chronic Diseases View all 22 articles
Background: Heart failure (HF) is associated with generalized insulin resistance (IR). Recent studies demonstrated that triglyceride glucose (TyG) is an effective alternative index of IR. However, the relationship between the TyG index and in-hospital mortality in patients with HF is unclear. In the present study, we aimed to clarify the association between the TyG index and in-hospital mortality in patients with HF.
Methods: A retrospective study consisting of 4,411 patients diagnosed with HF from 2015 to 2018 was conducted. All-cause mortality during hospitalization was the primary endpoint. The association between the TyG index and in-hospital mortality was assessed using the logistic regression analysis.
Results: The risk of in-hospital mortality was significantly associated with increased TyG index (OR: 1.886, 95% CI: 1.421–2.501, p < 0.001) under logistic regression with multivariable adjustment. When divided into three groups based on the TyG index, Tertile 3 demonstrated significantly higher in-hospital mortality than the other two Tertiles (OR: 2.076, 95% CI: 1.284–3.354, p = 0.001). Moreover, the TyG index improved the prediction efficiency of the Get with the Guidelines-Heart Failure (GWTG-HF) score (absolute integrated discrimination improvement = 0.006, p < 0.001; category-free net reclassification improvement = 0.075, p = 0.005). In subgroup analysis, the TyG index exhibited similar predictive performance of in-hospital mortality when groups were stratified based on type 2 diabetes mellitus (T2DM) and coronary artery disease (CAD).
Conclusion: TyG is a potential index for predicting in-hospital mortality in patients with HF, independent of T2DM or CAD status. The TyG index may be combined with the GWTG-HF score to further improve its predictive efficacy.
Heart failure (HF) is the end stage of many cardiovascular diseases, with high morbidity and mortality (1, 2). Due to the continuous development of oral drugs, the poor prognosis of HF has improved to some extent in recent years. However, poor prognosis remains the main cause of mortality in cardiovascular disease (3). Therefore, early risk stratification and accurate identification of high-risk patients with HF are essential for their effective management and treatment.
Insulin resistance (IR) is common in cardiovascular disease and has been established as a predictor of outcomes independent of type 2 diabetes mellitus (T2DM) status (4–7). As research has progressed, IR has been found to play a crucial role in the development of HF because of associated reductions in cardiac insulin metabolic signaling (8–10). To date, the closest approach to the gold diagnostic standard in IR is hyperinsulinemic–euglycemic clamp (11). However, the complexity of the test process limits its clinical applications. Based on the features of IR, hyperglycemia, and dyslipidemia, the triglyceride glucose (TyG) index was developed. TyG is calculated using fasting plasma glucose (FPG) and triglycerides, which are easily obtained by blood tests (12, 13). Numerous clinical studies indicated that TyG is significantly associated with IR obtained by the hyperinsulinemic–euglycemic clamp and even shows a better performance than the homeostasis model assessment of IR (HOMA-IR) in patients with and without diabetes (12, 13).
Previous studies primarily focused on the correlation between the TyG index and coronary artery disease (CAD; 6, 7). However, no study analyzed the predictive value of TyG for adverse events in HF patients with or without T2DM. Therefore, in this study, we aimed to explore the relationship between TyG levels and in-hospital mortality in patients with HF. Furthermore, we evaluated whether TyG could enhance the prediction efficiency of the Get with the Guidelines-Heart Failure (GWTG-HF) score.
The current study population was based on a retrospective observational cohort study, which enrolled 5,126 consecutive patients with HF at the Shengjing Hospital of China Medical University (from January 2015 to December 2018). The diagnosis of HF was confirmed by symptoms (such as breathlessness, ankle swelling, and fatigue), signs (such as elevated jugular venous pressure, pulmonary crackles, and peripheral edema), echocardiography, and the results of laboratory tests as recommended by the modified Framingham criteria (14), including de novo HF or decompensation of chronic HF. HF with reduced ejection fraction was defined as left ventricular ejection fraction (LVEF) ≤ 40%, HF with mildly reduced ejection fraction as 41% ≤ LVEF ≤ 49%, and HF with preserved ejection fraction (HFpEF) as LVEF ≥ 50% (15). Patient data were recorded in a database set up specifically for this study. The TyG index was calculated according to the formula:
The GWTG-HF risk score was calculated based on seven previously reported indicators: systolic blood pressure, heart rate, age, sodium, blood urea nitrogen (BUN), history of chronic obstructive pulmonary disease, and race (16). Within 24 h of admission, fasting venous blood samples were collected from all the patients. FPG and triglyceride levels were measured by an enhanced immunonephelometric assay using an automated analyzer (AU5800; Beckman Coulter, Inc., Carlsbad, CA, United States) in the Shengjing Hospital core laboratory. All-cause in-hospital mortality was the primary endpoint.
The exclusion criteria were as follows: no availability of fasting triglyceride or FPG data, cardiogenic shock, chronic kidney failure with dialysis on admission and/or diagnosed liver disease, and hormone therapy before admission. The present study included 4411 patients with HF.
Patient selection is shown in the flow diagram in Figure 1. The participants were divided into two groups according to their in-hospital mortality. This study complied with the Helsinki Declaration and was approved by the Research Ethics Committee of Shengjing Hospital of China Medical University (IRB number: 2019PS594K).
All patients were divided into two groups according to in-hospital mortality. Continuous variables are expressed as mean ± standard deviation, or median and interquartile range, depending on whether or not they were normally distributed. The differences in continuous variables between groups were analyzed using Student’s t-test or the Kruskal–Wallis H test. Categorical variables are expressed as numbers and percentages (%). The differences in categorical variables between the groups were analyzed using the chi-square test or Fisher’s exact test. Predictors associated with the primary endpoint were analyzed using univariate and multivariate logistic regression analyses. TyG levels were considered to be a continuous variable. To further explore the association between TyG and in-hospital mortality, TyG was also analyzed as a categorical variable, with Tertile 1 as the reference group. The relationship between the TyG index and primary endpoint was given as an odds ratio (OR) with associated 95% confidence intervals (CIs). The predictive potential of the TyG index and TyG + GWTG-HF score was analyzed. In brief, the area under the curve associated with the primary endpoint through the receiver operating characteristic curve was calculated by the statistical software MedCalc (version 18.1.1; MedCalc Software, Ostend, Belgium; 17). Absolute integrated discrimination improvement (IDI) and category-free net reclassification improvement (NRI) were used to evaluate the improvement in predictive efficiency of the TyG + GWTG-HF score compared to the GWTG-HF score (18). The interaction was tested with a likelihood ratio test, and the ORs with associated 95% CIs were represented by a forest plot. Statistical analyses were performed using SAS (version 9.4; Statistical Analysis Software Institute, Inc., Cary, NC, United States), and statistical significance was set at a p-value < 0.05.
A total of 4,411 patients with HF (mean age: 70.6 ± 12.6) were enrolled in our study, and 48.4% of patients were men. The mean length of hospitalization was 10.0 ± 6.2 days, and 187 (4.2%) patients died during hospitalization. The proportions of patients with HF with reduced ejection fraction, HF with mildly reduced ejection fraction, and HFpEF were 18.9, 40.1, and 41%, respectively. The baseline characteristics of the patients are summarized in Table 1. Patients with in-hospital mortality were likely to have a poor New York Heart Association (NYHA) grade, older age, high systolic blood pressure on admission, higher TyG index, higher GWTG-HF score, and a history of CAD. The patients who met in-hospital mortality also had higher creatinine, BUN, uric acid, N-terminal brain natriuretic peptide and cardiac troponin I levels, and lower hemoglobin, serum sodium, and albumin levels on admission than surviving patients. In terms of medications, the in-hospital mortality group had lower treatment rates with angiotensin-converting enzyme inhibitors (ACEI)/angiotensin receptor blockers (ARB)/angiotensin receptor-neprilysin inhibitor (ARNI), diuretics, and aldosterone antagonists (Table 1).
Table 1. Characteristics of subjects divided by in-hospital mortality, median (IQR), or N (%), or means ± SD.
Indicators of the primary endpoint identified by univariate analysis were also analyzed using multivariate analysis. Supplementary File 1 shows the univariate logistic analysis and the indicators related to in-hospital mortality: TyG index, age, sex, NYHA grade, systolic blood pressure, albumin, total bilirubin, BUN, creatinine, uric acid, hemoglobin, serum sodium, N-terminal brain natriuretic peptide, cardiac troponin I, LVEF, history of CAD, atrial fibrillation, smoking, and the use of ACEI/ARB/ARNI, beta-blockers, diuretics, or aldosterone antagonists.
Moreover, the logistic univariate analysis demonstrated that TyG, as a continuous variable, was associated with in-hospital mortality (OR: 1.266, 95% CI: 1.031–1.551, p = 0.024). Multiple adjustments for confounders did not attenuate this prediction (OR: 1.886, 95% CI: 1.421–2.501, p < 0.001) (Table 2). When patients were divided into three groups based on their TyG index, TyG remained a strong predictor of the primary endpoint. Using Tertile 1 (TyG index < 8.25) as a reference, multivariate analysis revealed that the TyG index in Tertiles 2 (8.25 ≤ TyG index < 8.78) and 3 (TyG index ≥ 8.78) increased the OR for in-hospital mortality in patients with HF (Tertile 2: OR 1.536, 95% CI 1.002–2.354, p = 0.049; Tertile 3: OR 2.076, 95% CI 1.284–3.354, p = 0.003) (Table 2) (The details of the multivariate analysis are shown in Supplementary Files 2, 3).
Table 2. Effects of multiple variables on clinical outcomes in univariate and multivariate analyses.
Combining the TyG index and GWTG-HF score enhanced the predictive efficiency of the new model compared to that with the GWTG-HF score only (IDI = 0.006, p < 0.001; NRI = 0.075, p = 0.005) (Table 3).
Table 3. Comparisons of the predictive performance of GWTG-HF and TYG+GWTG-HF for the prognosis prediction.
Relevant clinical indicators for in-hospital mortality such as age (< 65 vs. ≥ 65 years), ejection fraction (< 50% vs. ≥ 50%), sex (men vs. women), CAD (yes vs. no), and T2DM (yes vs. no) were evaluated using post hoc subgroup analysis. In subgroup analysis, we observed that a higher TyG index was significantly associated with an increased risk of in-hospital mortality after stratification by age, sex, CAD, and T2DM. However, the TyG index was less effective for predicting the endpoint in patients with HFpEF (Figure 2).
The TyG level was found to be a potential independent index for predicting in-hospital mortality. Furthermore, when combined with the GWTG-HF score, the TyG index produced better prognostic efficiency than the GWTG-HF score alone. Additionally, a similar predictive performance was found when patients were stratified by CAD and T2DM, which indicates that the TyG index plays an important role in prediction, independent of T2DM or CAD status. Therefore, our study reveals a relationship between TyG and HF in the general population for the first time.
Insulin resistance is classically defined as the failure of insulin to promote its metabolic activity in organs and tissues, such as the liver, the skeletal muscle, and the adipose tissue (8, 9). In 2005, Ingelsson et al. reported that IR could predict HF incidence even when adjusted for established risk factors for HF (19). In their study, insulin sensitivity was measured using the euglycemic insulin clamp (20). Despite the improvements in accuracy from euglycemic insulin and hyperinsulinemic–euglycemic clamps, these complex procedures remain difficult to use in the clinic. In the ARIC study, Vardeny et al. utilized HOMA-IR, which was calculated using FPG and fasting insulin, to explore the relationship between IR and HF. They observed that lower levels of HOMA-IR (1.0–2.0) than previously considered were associated with an increased risk of HF (21). Other than FPG and insulin, the symptoms of IR may manifest as dyslipidemia due to its influence on the liver (5, 22). Based on glucotoxicity and lipotoxicity, TyG was developed as a potential index to represent IR. In previous studies, TyG has shown a good correlation with IR, better than HOMA-IR (12, 13). Wenqin et al. studied 546 HF patients with T2DM and demonstrated a positive correlation between the TyG index and prognosis (23). However, the predictive effect of the TyG index in patients with HF who are non-diabetic remains unclear. To the best of our knowledge, our study is the first to explore the prognostic value of the TyG index in patients with HF, independent of T2DM status. We observed that TyG is a potential independent index for predicting in-hospital mortality in patients with HF. Moreover, the TyG index showed good performance in patients who are non-diabetic with HF (interaction p = 0.283, Figure 2). This observation suggests that IR affects the prognosis of patients with HF independent of diabetes. To further explore the clinical conditions of patients, we performed a subgroup analysis. A similar predictive performance was found for the TyG index under CAD stratification (interaction p = 0.055, Figure 2). This result was supported by the ARIC study, indicating that the relationship between IR and HF is not mediated by an increased risk of CAD (21). Notably, the results of our subgroup analysis of LVEF differ from those of previous studies (24, 25). In a prospective study of HF patients with LVEF > 40%, Tamariz et al. reported that patients with metabolic syndrome had a worse prognosis at 2.6 years of follow-up than those without metabolic syndrome (26). In contrast, Zhou et al. studied 1,548 patients with HFpEF and found no differences in cardiovascular and all-cause mortality between groups with and without metabolic syndrome. This is consistent with the results of our subgroup analyses. The predictive efficiency of the TyG index was impaired in patients with HFpEF (Figure 2). This result may be related to the heterogeneous nature of HFpEF (27). Age, female sex, diabetes, hypertension, obesity, metabolic syndrome, and atrial fibrillation have been identified as classical risk factors for the development of HFpEF (28). Thus, interactions between these comorbidities may make it challenging to evaluate specific indicators of in-hospital mortality. In addition, the 95% CI for the TyG index in HFpEF was wide and included a potentially important effect size; therefore, further understanding of the pathophysiology of HFpEF and long-term follow-up studies may be required to assess the TyG index for patients with HFpEF.
In 2010, Peterson et al. set up the GWTG-HF score to predict adverse events in hospitals, and the points-scoring system has been widely utilized since the publishing of studies by Peterson et al., Suzuki et al., and Shiraishi et al. (16, 29, 30). We further explored whether TyG can enhance the predictive efficiency of the GWTG-HF score. Because the receiver operating characteristic analysis could not provide pertinent information about whether a combination of GWTG-HF score with a new index would enhance the accuracy of the model for predicting the risk of in-hospital mortality, we also conducted IDI and NRI to measure the incremental value (31, 32). In our study, both IDI and NRI demonstrated a significant improvement in the reclassification of in-hospital mortality by TyG + GWTG-HF vs. GWTG-HF alone (IDI = 0.006, p < 0.001; NRI = 0.075, p = 0.005) (Table 3).
Although the pathophysiology of IR in HF requires further research, the effect of the TyG index on patient prognosis may be related to known mechanisms. In conditions of IR, the myocardium utilizes free fatty acids and decreases glucose uptake and oxidation (33). Because glucose is a more efficient substrate than fatty acids, converting cardiac metabolism from glucose metabolism to fatty acid oxidation reduces cardiac efficiency. This metabolic disorder increases the predisposition to stress overload and ischemia. In addition to glucotoxicity and lipotoxicity, the dysregulation of neurohumoral factors, cytokines, and oxidative stress is the main cause of cardiac IR and impaired cardiac function (34). Furthermore, IR can lead to hyperinsulinemia, which is recognized as a factor that accelerates cardiac remodeling (35, 36). Hyperinsulinemia can also lead to the retention of sodium and the activation of the sympathetic nervous system, which contributes to HF (37, 38). Consequently, IR influences glucose and lipid metabolism, leading to a mismatch in energy needs, affecting neurohumoral factors, and causing HF progression.
The TyG index is simple to calculate and easy to obtain at the bedside. Therefore, it improves upon other IR prediction indicators and must be popularized. Monitoring the TyG index can better identify patients with a high risk of in-hospital mortality from HF, independent of T2DM and CAD status. Additionally, a new model consisting of the TyG index and GWTG-HF score can further enhance the predictive efficacy of GWTG-HF, potentially benefiting clinical practice.
The present study has some limitations. First, this was a retrospective study, and the causal relationship of the association between the TyG index and in-hospital mortality requires further confirmation through prospective studies. Second, because the TyG index was assessed only at admission without dynamic monitoring, we could not assess whether lowering the TyG index might improve patient prognosis. Finally, although we included as many clinically relevant variables as possible in the multivariate analysis, potential confounders likely remained.
In summary, TyG was a potential independent index for predicting in-hospital mortality in patients with HF, independent of T2DM or CAD status. The TyG index can further improve the predictive efficacy of the GWTG-HF score.
The raw data supporting the conclusions of this article will be made available by the authors, without undue reservation.
Ethical review and approval was not required for the study on human participants in accordance with the local legislation and institutional requirements. Written informed consent from the patients was not required to participate in this study in accordance with the national legislation and the institutional requirements.
All authors were involved in the conception and design of the study, as well as in the collection, analysis and interpretation of data, reviewed, and approved the final manuscript.
The authors declare that the research was conducted in the absence of any commercial or financial relationships that could be construed as a potential conflict of interest.
All claims expressed in this article are solely those of the authors and do not necessarily represent those of their affiliated organizations, or those of the publisher, the editors and the reviewers. Any product that may be evaluated in this article, or claim that may be made by its manufacturer, is not guaranteed or endorsed by the publisher.
The Supplementary Material for this article can be found online at: https://www.frontiersin.org/articles/10.3389/fnut.2022.950338/full#supplementary-material
1. Chandra A, Vaduganathan M, Lewis E, Claggett BL, Rizkala AR, Wang W, et al. Health-related quality of life in heart failure with preserved ejection fraction: The PARAGON-HF trial. JACC Heart Fail. (2019) 7:862–74. doi: 10.1016/j.jchf.2019.05.015
2. Ma L, Chen W, Gao R, Liu LS, Zhu ML, Wang YJ, et al. China cardiovascular diseases report 2018: An updated summary. J Geriatr Cardiol. (2020) 17:1–8. doi: 10.11909/j.issn.1671-5411.2020.01.001
3. Gager G, Gelbenegger G, Jilma B, von Lewinski D, Sourij H, Eyileten C, et al. Cardiovascular outcome in patients treated with SGLT2 inhibitors for heart failure: A meta-analysis. Front Cardiovasc Med. (2021) 8:691907. doi: 10.3389/fcvm.2021.691907
4. Rask-Madsen C, Kahn CR. Tissue-specifific insulin signaling, metabolic syndrome, and cardiovascular disease. Arterioscler Thromb Vasc Biol. (2012) 32:2052–9.
5. Ormazabal V, Nair S, Elfeky O, Aguayo C, Salomon C, Zu?iga FA, et al. Association between insulin resistance and the development of cardiovascular disease. Cardiovasc Diabetol. (2018) 17:122.
6. Ma X, Dong L, Shao Q, Cheng Y, Lv S, Sun Y, et al. Triglyceride glucose index for predicting cardiovascular outcomes after percutaneous coronary intervention in patients with type 2 diabetes mellitus and acute coronary syndrome. Cardiovasc Diabetol. (2020) 19:31. doi: 10.1186/s12933-020-01006-7
7. Luo E, Wang D, Yan G, Qiao Y, Liu B, Hou J, et al. High triglyceride-glucose index is associated with poor prognosis in patients with acute ST-elevation myocardial infarction after percutaneous coronary intervention. Cardiovasc Diabetol. (2019) 18:150. doi: 10.1186/s12933-019-0957-3
8. Aroor A, Mandavia C, Sowers J. Insulin resistance and heart failure: Molecular mechanisms. Heart Fail Clin. (2012) 8:609–17.
10. Velez M, Kohli S, Sabbah HN. Animal models of insulin resistance and heart failure. Heart Fail Rev. (2014) 19:1–13.
11. American Diabetes Association [ADA]. Consensus development conference on insulin resistance. 5–6 November 1997. American Diabetes Association. Diabetes Care. (1998) 21:310–4. doi: 10.2337/diacare.21.2.310
12. Guerrero-Romero F, Simental-Mendia LE, Gonzalez-Ortiz M, Martínez-Abundis E, Ramos-Zavala MG, Hernández-González SO, et al. The product of triglycerides and glucose, a simple measure of insulin sensitivity comparison with the euglycemic–hyperinsulinemic clamp. J Clin Endocrinol Metab. (2010) 95:3347–51. doi: 10.1210/jc.2010-0288
13. Vasques AC, Novaes FS, de Oliveira MDS, Souza JRM, Yamanaka A, Pareja JC, et al. TyG index performs better than HOMA in a Brazilian population: A hyperglycemic clamp validated study. Diabetes Res Clin Pract. (2011) 93:e98–100. doi: 10.1016/j.diabres.2011.05.030
14. McKee P, Castelli W, McNamara P, Kannel WB. The natural history of congestive heart failure: The Framingham study. New Engl J Med. (1971) 285:1441–6.
15. McDonagh T, Metra M, Adamo M, Gardner RS, Baumbach A, Bohm M, et al. 2021 ESC Guidelines for the diagnosis and treatment of acute and chronic heart failure: Developed by the Task Force for the diagnosis and treatment of acute and chronic heart failure of the European Society of Cardiology (ESC). With the special contribution of the Heart Failure Association (HFA) of the ESC. Eur J Heart Fail. (2022) 24:4–131.
16. Peterson P, Rumsfeld J, Liang L, Albert NM, Hernandez AF, Peterson ED, et al. A validated risk score for in-hospital mortality in patients with heart failure from the American Heart Association Get With the Guidelines program. Circ Cardiovasc Qual. (2010) 3:25–32.
17. DeLong E, DeLong D, Clarke-Pearson D. Comparing the areas under two or more correlated receiver operating characteristic curves: A nonparametric approach. Biometrics. (1988) 44:837–45.
18. Redelmeier D, Bloch D, Hickam D. Assessing predictive accuracy: How to compare Brier scores. J Clin Epidemiol. (1991) 44:1141–6.
19. Ingelsson E, Sundström J, Arnlöv J. Insulin resistance and congestive heart failure. JAMA. (2005) 294:334–41.
20. DeFronzo RA, Tobin JD, Andres R. Glucose clamp technique: A method for quantifying insulin secretion and resistance. Am J Physiol. (1979) 237:E214–23.
21. Vardeny O, Gupta D, Claggett B, Burke S, Shah A, Loehr L, et al. Insulin resistance and incident heart failure the ARIC study (Atherosclerosis Risk in Communities). JACC Heart Fail. (2013) 1:531–6.
22. Klop B, Elte J, Cabezas M. Dyslipidemia in obesity: Mechanisms and potential targets. Nutrients. (2013) 5:1218–40.
23. Guo W, Zhao L, Mo F, Peng C, Li L, Xu Y, et al. The prognostic value of the triglyceride glucose index in patients with chronic heart failure and type 2 diabetes: A retrospective cohort study. Diabetes Res Clin Pract. (2021) 177:108786. doi: 10.1016/j.diabres.2021.108786
24. Arcopinto M, Schiavo A, Salzano A, Bossone E, D’Assante R, Marsico F, et al. Metabolic syndrome in heart failure: Friend or foe? Heart Fail Clin. (2019) 15:349–58.
25. Tamariz L, Hassan B, Palacio A, Arcement L, Horswell R, Hebert K, et al. Metabolic syndrome increases mortality in heart failure. Clin Cardiol. (2009) 32:327–31.
26. Zhou Y, Fu L, Sun J, Zhu Z, Xing Z, Zhou S, et al. Association between metabolic syndrome and an increased risk of hospitalization for heart failure in population of HFpEF. Front Cardiovasc Med. (2021) 8:698117. doi: 10.3389/fcvm.2021.698117
27. Zheng S, Chan F, Nabeebaccus A, Shah AM, McDonagh T, Okonko DO, et al. Drug treatment effects on outcomes in heart failure with preserved ejection fraction: A systematic review and meta-analysis. Heart. (2018) 104:407–15.
28. Ferrari R, Bohm M, Cleland JG, Paulus WJS, Pieske B, Rapezzi C, et al. Heart failure with preserved ejection fraction: Uncertainties and dilemmas. Eur J Heart Fail. (2015) 17:665–71.
29. Suzuki S, Yoshihisa A, Sato Y, Kanno Y, Watanabe S, Abe S, et al. Clinical significance of get with the guidelines-heart failure risk score in patients with chronic heart failure after hospitalization. J Am Heart Assoc. (2018) 7:e008316. doi: 10.1161/JAHA.117.008316
30. Shiraishi Y, Kohsaka S, Abe T, Mizuno A, Goda A, Izumi Y, et al. Validation of the Get With The Guideline-Heart Failure risk score in Japanese patients and the potential improvement of its discrimination ability by the inclusion of B-type natriuretic peptide level. Am Heart J. (2016) 171:33–9. doi: 10.1016/j.ahj.2015.10.008
31. Cook NR. Use and misuse of the receiver operating characteristic curve in risk prediction. Circulation. (2007) 115:928–35.
32. Pickering JW, Endre ZH. New metrics for assessing diagnostic potential of candidate biomarkers. Clin J Am Soc Nephrol. (2012) 7:1355–64.
33. Witteles RM, Fowler MB. Insulin-resistant cardiomyopathy clinical evidence, mechanisms, and treatment options. J Am Coll Cardiol. (2008) 51:93–102.
34. Hattori Y. Insulin resistance and heart failure during treatment with sodium glucose cotransporter 2 inhibitors: Proposed role of ketone utilization. Heart Fail Rev. (2020) 25:403–8. doi: 10.1007/s10741-020-09921-3
35. Rutter MK, Parise H, Benjamin EJ, Levy D, Larson MG, Meigs JB, et al. Impact of glucose intolerance and insulin resistance on cardiac structure and function: Sex-related differences in the Framingham Heart Study. Circulation. (2003) 107:448–54. doi: 10.1161/01.cir.0000045671.62860.98
36. Sundstrom J, Lind L, Nystrom N, Zethelius B, Andrén B, Hales CN, et al. Left ventricular concentric remodeling rather than left ventricular hypertrophy is related to the insulin resistance syndrome in elderly men. Circulation. (2000) 101:2595–600. doi: 10.1161/01.cir.101.22.2595
37. DeFronzo RA, Cooke CR, Andres R, Faloona GR, Davis PJ. The effect of insulin on renal handling of sodium, potassium, calcium, and phosphate in man. J Clin Invest. (1975) 55:845–55. doi: 10.1172/JCI107996
Keywords: heart failure, triglyceride glucose index, type 2 diabetes, prognosis, GWTG-HF risk score
Citation: Han S, Wang C, Tong F, Li Y, Li Z, Sun Z and Sun Z (2022) Triglyceride glucose index and its combination with the Get with the Guidelines-Heart Failure score in predicting the prognosis in patients with heart failure. Front. Nutr. 9:950338. doi: 10.3389/fnut.2022.950338
Received: 01 June 2022; Accepted: 12 August 2022;
Published: 08 September 2022.
Edited by:
Mohammad Alizadeh, Tabriz University of Medical Sciences, IranReviewed by:
Jun Gu, Shanghai Jiao Tong University School of Medicine, ChinaCopyright © 2022 Han, Wang, Tong, Li, Li, Sun and Sun. This is an open-access article distributed under the terms of the Creative Commons Attribution License (CC BY). The use, distribution or reproduction in other forums is permitted, provided the original author(s) and the copyright owner(s) are credited and that the original publication in this journal is cited, in accordance with accepted academic practice. No use, distribution or reproduction is permitted which does not comply with these terms.
*Correspondence: Zhijun Sun, c3VuempfOTlAMTYzLmNvbQ==
Disclaimer: All claims expressed in this article are solely those of the authors and do not necessarily represent those of their affiliated organizations, or those of the publisher, the editors and the reviewers. Any product that may be evaluated in this article or claim that may be made by its manufacturer is not guaranteed or endorsed by the publisher.
Research integrity at Frontiers
Learn more about the work of our research integrity team to safeguard the quality of each article we publish.