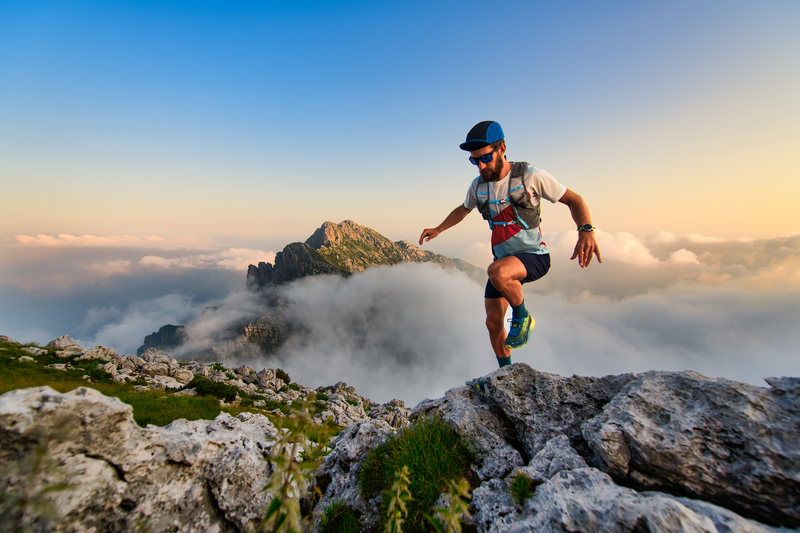
94% of researchers rate our articles as excellent or good
Learn more about the work of our research integrity team to safeguard the quality of each article we publish.
Find out more
ORIGINAL RESEARCH article
Front. Nutr. , 22 June 2022
Sec. Nutrition and Food Science Technology
Volume 9 - 2022 | https://doi.org/10.3389/fnut.2022.919882
This article is part of the Research Topic Recent Advances and Product Opportunities in the Technology of Proteins, Probiotics and Prebiotics View all 21 articles
Background: Overweight and obesity increase the risk of noncommunicable diseases (NCDs). Personalized nutrition (PN) approaches may provide tailored nutritional advice/service by focusing on individual's unique characteristics to prevent against NCDs.
Objective: We aimed to compare the effect of PN intervention with the traditional “one size fits all” intervention on health status in overweight/obese Chinese adults.
Methods: In this 12-week randomized controlled trial, 400 adults with BMI ≥24 kg/m2 were randomized to control group (CG, n = 200) and PN group (PNG, n = 200). The CG received conventional health guidance according to the Dietary Guidelines for Chinese Residents and Chinese DRIs Handbook, whereas the PNG experienced PN intervention that was developed by using decision trees based on the subjects' anthropometric measurements, blood samples (phenotype), buccal cells (genotype), and dietary and physical activity (PA) assessments (baseline and updated).
Results: Compared with the conventional intervention, PN intervention significantly improved clinical outcomes of anthropometric (e.g., body mass index (BMI), body fat percentage, waist circumference) and blood biomarkers (e.g., blood lipids, uric acid, homocysteine). The improvement in clinical outcomes was achieved through behavior change in diet and PA. The subjects in the PNG had higher China dietary guidelines index values and PA levels. Personalized recommendations of “lose weight,” “increase fiber” and “take multivitamin/mineral supplements” were the major contributors to the decrease of BMI and improvement of lipid profile.
Conclusion: We provided the first evidence that PN intervention was more beneficial than conventional nutrition intervention to improve health status in overweight/obese Chinese adults. This study provides a model of framework for developing personalized advice in Chinese population.
Chictr.org.cn (ChiCTR1900026226).
Overweight and obesity increase the risk of non-communicable diseases (NCDs), including diabetes, cardiovascular diseases, non-alcohol fatty liver disease, and even certain cancers (1, 2). The rising trends in overweight and obesity have plateaued in many high-income countries, but have accelerated in most Asian countries (1). Effective prevention strategies are required to reduce the immense global burden of nutrition-related NCDs, especially those primarily related to poor lifestyle choices, including unhealthy dietary patterns and insufficient physical activity (PA) (3, 4). Most population strategies to reduce the burden of NCDs have used conventional ‘one size fits all' public health recommendations throughout the past decades, but with limited efficacy (5).
Personalized nutrition (PN) approaches, which provide nutrition advice, products, and services tailored for an individual according to his/her unique biological characteristics, may provide extra motivations to the individual, and therefore, be more efficacious in preventing against NCDs (6). Zeevi et al. created personalized diets using a machine learning algorithm that integrated parameters including dietary habits, PA, and gut microbiota, to successfully lower postprandial blood glucose and its metabolic consequences (7). Mathers et al. carried out the Food4Me randomized controlled trial (RCT) in seven European countries and showed that decision tree-based PN intervention produced larger and more appropriate changes in dietary behavior compared with the conventional approaches. These studies collectively triggered enthusiasm on PN research in recent years (5, 8).
PN at the individual level requires not only the comprehensive collection of information, including anthropometric data, dietary intake, PA, blood biomarkers, and genotypes—which is both costly and demanding—but also models that are capable of accurately generating personalized advice for each individual (9, 10). Food4Me decision trees have been validated in several PN trials (5, 8, 11, 12). In the current study, the principal investigator and registered dietitians certificated by the China Nutrition Society revised the decision trees and accompanying advice messages to keep them in accordance with the Chinese dietary habits and technical standards. Using the localized decision trees, along with the PN report prepared by the registered dietitians, we carried out the first RCT on PN in China (Diet2Me study) to investigate whether PN intervention would show greater benefits to health status in overweight/obese adults compared with the conventional intervention.
This randomized, controlled, multicenter clinical trial was conducted in Aier Hospital and Songnan Hospital, Shanghai, China. The participants were identified from the hospital databases. A total of 400 participants were initially enrolled in the study at baseline and randomized with a 1:1 ratio into two groups: control group (CG) and personalized nutrition group (PNG) (Figure 1). For both groups, questionnaires assessing diet and PA, anthropometric measurements, blood samples, buccal cells, and fecal samples were collected at baseline. During the intervention, 24-h dietary recall was collected every 2 weeks, and portable devices were worn every day. At the end point, the questionnaires, anthropometric measurements, blood, and fecal samples were collected again from both groups for analysis (Figure 2A). The primary outcome was body mass index (BMI). The secondary outcomes were other clinical outcomes of anthropometrics and blood biomarkers. No changes to trial outcomes after the trial commenced. Informed consent was obtained from all the patients. This trial was approved by the Institutional Review Board (IRB) of the Shanghai Nutrition Society and registered at chictr.org.cn (ChiCTR1900026226).
Figure 2. Overview of the study design. (A) Questionnaires, anthropometric measurements, blood, and fecal samples were collected at baseline and at end point. Buccal cells were collected at baseline for genotyping. During the intervention, 24-h recall was collected every 2 weeks, and a portable device was worn every day. (B) The subjects in the control group (CG) received non-personalized, conventional health guidance on diet, physical activity (PA), and nutritional supplements intake based on the Dietary Guidelines for Chinese Residents and Chinese DRIs Handbook. A brochure summarizing the health guidance was delivered to each CG subject at baseline, and follow-up notifications on nutrition education were provided biweekly to these subjects using WeChat. (C) The subjects in the personalized nutrition group (PNG) received personalized nutrition solution on diet, physical activity (PA), and nutritional supplements intake from the registered dietitians based on the decision trees. The personalized solution was updated biweekly based on the subjects' dietary and PA changes.
The inclusion criteria encompassed either of the sexes, age 25 to 50 years, and overweight/obesity with BMI ≥24 kg/m2. Subjects were excluded if they had coronary heart disease, cerebrovascular disease, liver disease, kidney disease, inflammatory bowel disease, or hematologic disease; were pregnant or lactating women; were currently taking any drugs or dietary supplements in recent days, which could interfere with the study product; participated in another clinical trial in the past 3 months; or were not willing to comply with the study procedures.
The subjects in CG received a non-personalized, conventional health guidance on diet, PA, and nutritional supplements intake based on Dietary Guidelines for Chinese Residents and Chinese DRIs Handbook (Figure 2B). The guidelines embody the recommended proportion of the different food groups in the diet, recommendations to drink plenty of water, PA, dietary supplement intake, and dietary recommendations for specific population groups. A brochure summarizing the health guidance was delivered to each CG subject at baseline. Differed from the conventional nutrition intervention, a personalized solution integrating recommendations on diet, PA, and dietary supplements (Nutrilite, Guangzhou, China) was generated by registered dietitians based on decision trees, and was biweekly updated according to the dietary recall and PA assessments during the study (Figure 2C). The interventions to the CG and PNG were delivered through sending notifications via a localized messaging and calling application (WeChat) with the same frequency (biweekly) to ensure that both groups underwent the same intensity of intervention throughout the study. Neither CG nor PNG had actual interactions with dieticians to minimize bias between the groups. More details on the personalized intervention were described in the following sections. The list of dietary supplements is shown in Supplementary Table 1.
The decision trees used herein originated from the Food4Me study decision trees, which were kindly provided by Prof. John C. Mathers (5, 8). Considering the differences in dietary habits and technical standards between Western and Eastern countries, the decision trees and corresponding feedback messages were revised by Prof. Hongwei Guo, the principal investigator of the current study, in addition to five other registered dietitians certificated by the Chinese Nutrition Society. There were 16 decision trees in the current study, covering the majority of macro- and micronutrients.
The PN report included a summary of the individual's anthropometric, nutrient, blood, and genetic (nutrition-related) profiles, an overall goal established by the registered dietitian, as well as personalized recommendations on lifestyle modifications. All the PN reports were prepared manually by registered dietitians using the revised decision trees. In brief, the numerical data collected at baseline (anthropometric characteristics, blood biomarkers, food intake, PA) were categorized into three levels: green (normal), yellow (higher or lower in moderation), and red (severely higher or lower), based on the Chinese standards published by the Chinese Nutrition Society and Chinese Medical Association (13, 14). Then, the categorized parameters were ranked, and 3–5 targeted goals were identified by registered dietitians. Specific messages related to each goal were developed according to the decision trees to advise changes in dietary habits and PA (5, 15). A nutritional supplement was suggested when the calculated intake of a certain nutrient was lower than 80% of the estimated average requirement (EAR). The complete report was delivered to each subject at baseline. In the follow-up period, the subjects received updated report sent by the dietitian every 2 weeks. This updated report was prepared based on the most recently collected 24 h-recall and wearable device recording, and just included the main goals and some follow-up comments on the behavior changes regarding to the individual goal.
Body weight (BW) and body height (BH) were measured using a standardized digital weight scale (Seca, Hamburg, Germany) and height meter (Seca). BMI is defined as weight in kilograms divided by the square of height in meters (kg/m2). Waist circumference (WC) and hip circumference (HC) was measured using a non-stretch tape, and waist-to-hip ratio (WHR) was calculated accordingly. Body composition was assessed utilizing an InBody (Biospace Co., Ltd., Seoul, Korea), in which body fat percentage (BFP) was recorded. Systolic blood pressure (SBP) and diastolic blood pressure (DBP) were measured by an electronic sphygmomanometer (Omron, Kyoto, Japan). All measures were taken twice at least. A third measure was taken when substantial discrepancies (difference ≥ 1%) were observed in the first two attempts.
Intensity (vigorous, moderate, or walking), frequency, and duration of PA were recorded using the short version of the International Physical Activity Questionnaire (IPAQ) to measure the PA level (16). The minutes/week of each PA intensity was calculated. According to the IPAQ scoring protocol, the metabolic equivalents of task (METs)-min/week were obtained by multiplying the average energy expenditure by min/week for each PA intensity (8.0 MET for vigorous intensity, 4.0 MET for moderate intensity, and 3.3 MET for walking) (17). Total PA score in MET-min/week was obtained by summing the results of each intensity of PA. The continuous scores were then categorized into three levels (low, moderate, and high) of PA to classify populations. In addition, step count, consumed energy, and sleep condition were recorded by Mi Band (Xiaomi, Beijing, China), a wearable device, in real time.
A semiquantitative food frequency questionnaire (FFQ) approved by the Chinese Center for Disease Control and Prevention was slightly revised in the current study to assess the dietary intake of the subjects (18). The questionnaire contained 77 food items grouped into 13 categories: staple food, bean, vegetable, fungi/algae, fruit, dairy, meat, fish, egg, snack, drink, oil/spices, and smoke/alcohol. For each food item, the frequency and amount of consumption over the past week were recorded. China dietary guidelines index (CDGI) was calculated accordingly to evaluate the overall diet quality of the subjects. The details on the CDGI calculation were previously published (19).
The participants also used 24-h dietary recalls to record the name and amount of each food consumed for breakfast, lunch, dinner, and snacks. Food composition analysis was performed using commercially available software (Feihua, Beijing, China).
Blood samples were collected after over 12-h fasting. The serum levels of triglycerides (TG), total cholesterol (TC), low-density lipoprotein (LDL), high-density lipoprotein (HDL), glucose (GLU), uric acid (UA), homocysteine (HCY), aspartate aminotransferase (AST), and alanine aminotransferase (ALT) were determined by colorimetry. The levels of vitamin A (VA), 25(OH)D3 (VD), docosahexaenoic acid (DHA), and eicosapentaenoic acid (EPA) were determined by tandem mass spectrometry. The levels of insulin (INS), vitamin B9 (folic acid, VB9) and vitamin B12 (VB12) were determined by chemiluminescence. The levels of calcium (Ca), magnesium (Mg), iron (Fe), zinc (Zn), and copper (Cu) were determined by atomic absorption spectrometry.
Buccal cells were collected with oral swab (DNA Genotek, Ottawa, ON, Canada). DNA was extracted and analyzed by WuXi NextCode (Shanghai, China) using an Asian Single Nucleotide Polymorphism (SNP) Screening Chip (Illumina, San Diego, California, USA). The list of nine nutrition-related genes, including BCO1, GC, MTHFR, FTO, APOE, FADS1, TCF7L2, CaSR, and TMPRSS6, is shown in Supplementary Table 2. The gene variants were selected based on the documented evidence of gene-diet interactions (20–28). A systematic review of the rationale for selection of genes will be published separately soon.
It was estimated that a total sample size of 286 participants (143 per group) would provide 80% power to detect a more than 5% change in BMI (29) between the two groups, with a significance level of 0.05. Allowing for a potential dropout rate of 25% during the intervention, we recruited 400 subjects (200 for each group) at baseline.
Statistical analyses were completed using SAS 9.4 (SAS Institute Inc., Cary, NC, USA). All statistical tests were two-sided and performed at the 0.05 significance level. Means and standard deviations (SD) were summarized for continuous variables with a normal distribution; medians and quartiles were provided for non-normally distributed variables; and frequencies and percentages were provided for categorical variables. Differences between the groups at baseline were assessed using analysis of variance (ANOVA) for continuous variables, and chi-square test for nominal categorical variables. Evaluations of the intervention effect were performed using analysis of covariance (ANCOVA) for normally distributed continuous variables, adjusted for baseline values. Log transformation was applied to non-normal continuous variables. Wilcoxon–Mann–Whitney test was used for highly skewed variables. The connections of longitudinal correlation network were calculated by Spearman or Point-Biserial and then plotted as a network by Cytoscape 3.8.2 (Cytoscape Consortium, San Diego, CA, USA).
A total of 2,718 participants were screened, of whom 400 eligible subjects were randomized into two groups: control group (CG) and personalized nutrition group (PNG). During 12-week intervention, 82 subjects withdrew, resulting in a total dropout rate of 20.5% (Figure 1). The subjects in PNG received a personalized nutrition solution on diet, PA, and nutritional supplements intake, which was provided by registered dietitians using decision trees. The subjects in CG received non-personalized, conventional health guidance (Figure 2). The baseline characteristics of the subjects, including demographics and anthropometrics, were comparable between the two groups (Table 1).
After 12 weeks of intervention, the subjects in both CG and PNG experienced significant improvement in weight, BMI, body fat percentage, waist circumference, and waist-to-hip ratio (Supplementary Table 3). The effects of PN intervention on decreasing BMI, body fat percentage, waist circumference, and waist-to-hip ratio were significantly more potent than those in CG (Figure 3). In addition, PN intervention was more efficient than the conventional intervention in decreasing blood levels of TG, TC, LDL, uric acid, and homocysteine (Table 2, Supplementary Table 4).
Figure 3. Changes in anthropometric measurements between CG and PNG. Intergroup differences in changes in anthropometric measurements (A–D) were evaluated using Wilcoxon–Mann–Whitney test. *P < 0.05, **P < 0.01, ***P < 0.001.
PN intervention significantly improved the overall diet quality of the subjects, as reflected in the CDGI (Table 3). Compared with the subjects in CG, the subjects receiving PN intervention consumed more whole grains, vegetables, especially dark green vegetables, fruits, dairy products, and nuts. Within-intervention comparison showed the improvement of diet quality in both groups, but PNG showed greater improvement (Supplementary Table 5). Although the energy intake was not signficantly differed, compared with the CG, the PNG had substantially reduced fat consumption and increased dietary intake of beneficial vitamin and minerals (Supplementary Table 6). The dietary modification partially explained why we observed significantly higher circulating EPA, folic acid and calcium levels in the PNG than CG (Table 2). Dietary supplement intake was beneficial in improving blood biomarkers, and Multi-Vitamin and Minerals (MVM) was the only supplement that led to significant BMI decrease (Figure 4).
Figure 4. Spearman correlation with clustered clinical outcomes (anthropometric measurements and blood biomarkers) and supplement usage. Blue color indicates strong reverse correlation; red color indicates strong positive correlation. *P < 0.05, **P < 0.01, ***P < 0.001. ALT, alanine aminotransferase; AST, aspartate aminotransferase; BFP, body fat percentage; BMI, body mass index; Ca, calcium; Cu, copper; DBP, diastolic blood pressure; DHA, docosahexaenoic acid; EPA, eicosapentaenoic acid; Fe, iron; GLU, glucose; HCY, homocysteine; HDL, high density lipoprotein; INS, insulin; LDL, low density lipoprotein; Mg, magnesium; MVM, multiple vitamin mineral; SBP, systolic blood pressure; TC, total cholesterol; TG, triglycerides; SBP, systolic blood pressure; Suppl, supplement; UA, uric acid; VA, vitamin A; VB, vitamin B; VC, vitamin C; VD, vitamin D; VE, vitamin E; WC, waist circumference; WHR, waist-to-hip ratio; Zn, zinc.
Although both groups expereinced improvement in PA with the interventions, the PNG had significantly higher vigorous, moderate, and total metabolic equivalent of task (MET), as compared to the CG (Table 3, Supplementary Table 7). In addition, the sitting duration was shortened to a greater extent in the PNG than the CG, whereas the sleeping duration change in both groups was similar. The PA was categorized into three levels (low, moderate, and high); a significantly higher level of PA was observed in PNG after the intervention compared with CG (Supplementary Table 8). Subjects with higher PA level after the intervention experienced greater reduction in body weight, BMI, and waist circumference (Supplementary Figure 1). Based on the wearable device, the PNG had signficantly more step counts and consumed more energy than the CG (Supplementary Table 9).
Data collected at the baseline showed significant correlations between the risk of MTHFR and blood level of folic acid; the risk of FTO and the levels of HDL and uric acid; the risk of APOE and the levels of TG, TC and LDL; the risk of FADS1 and the level of HDL; and the risk of TCF7L2 and the level of uric acid (Supplementary Table 10). The effects of PN intervention on the change from baseline in anthropometric characteristics, including body weight, BMI, waist circumference, and body fat percent, as well as on the changes in blood levels of TG, TC, HDL, and LDL, appeared to bring more benefits to the subjects carrying risk alleles of FTO, APOE, FADS1, and TCF7L2 genes than to those with normal alleles, though none of the interactions between the genotype and intervention group were significant (Supplementary Tables 11, 12).
We analyzed the longitudinal correlation network among the advice in the PN report, changes in lifestyle, and changes in clinical outcomes. Following the given advice, the subjects in PNG increased their PA and optimized nutrient intake, which led to the increase of beneficial nutrients in the blood and to the decrease of “bad” blood biomarkers and anthropometrics (Figure 5). In the PNG, the decrease of BMI was mostly related to the personalized recommendations of “increase fiber,” “lose weight”, and “use MVM supplements,” the increase of moderate MET, and the intake of dietary vitamin B2, B6, and B12. In addition, TMPRSS6, FTO and MTHFR genotypes mediated the efficacy of nutritional recommendations and lifestyle modifications on BMI decrease (Figure 5, sub-network).
Figure 5. Longitudinal correlation network of advice, genotype, lifestyles, and clinical outcomes. Connections were calculated by Spearman or Point-Biserial with Benjamini-Hochberg-corrected P < 0.05 and then plotted as a network by Cytoscape. Nodes are colored according to (1) group of advice, including genetic risk notification (e.g., FTO), lifestyle guidance (e.g., lose weight), and nutritional supplements (e.g., Suppl MVM), (2) change in lifestyles (diet and PA), and (3) change in clinical outcomes (anthropometric measurements and blood biomarkers). Node sizes are proportional to betweenness centrality. Edge weights are proportional to the correlation strength, and colors correspond to the direction of association (positive: red; negative: blue). Sub-network in top right shows an example of how “BMI” change be associated with the advice, genotype, lifestyles, and clinical outcomes. ALT, alanine aminotransferase; AST, aspartate aminotransferase; BFP, body fat percentage; BMI, body mass index; Ca, calcium; Cu, copper; DBP, diastolic blood pressure; DHA, docosahexaenoic acid; EPA, eicosapentaenoic acid; Fe, iron; GLU, glucose; HCY, homocysteine; HDL, high density lipoprotein; I, iodine; LDL, low density lipoprotein; MET, metabolic equivalent of task; Mg, magnesium; MVM, multiple vitamin mineral; Na, sodium; SBP, systolic blood pressure; SFA, saturated fatty acid; TC, total cholesterol; TG, triglycerides; UA, uric acid; VA, vitamin A; VB, vitamin B; VC, vitamin C; VD, vitamin D; VE, vitamin E; WC, waist circumference; WHR, waist-to-hip ratio; Zn, zinc.
Health benefits achieved by PN should be measured using validated clinical outcomes, such as anthropometric parameters (e.g., BMI, body fat percentage, and waist circumference) and blood biomarkers (e.g., blood lipids, blood glucose, and blood nutrients) (10). Anthropometric measurements and blood biomarkers are suitable for PN assessment and monitoring as they provide real-time information that reflects an individual's current metabolic or physiological state (6). More importantly, the information is readily actionable and easily trackable, as these parameters change over time in response to diet, PA, and other lifestyle factors (6). In this study, we observed improved clinical outcomes (weight, BMI, body fat percentage, waist circumference, waist-to-hip ratio, blood TG, TC, LDL, uric acid, homocysteine, folic acid, EPA, and calcium) in the PNG compared with those in the CG. This was the first RCT to show the health benefits of PN intervention in overweight/obese Chinese adults. In the Food4Me study, there was no significant difference in clinical outcomes between PN and control groups, though a trend was observed in weight, BMI, waist circumference, and blood TC (5). The reason might be the lack of inclusion criteria on BMI in the Food4Me study, while in this study we included overweight/obese adults because there is evidence that people are more open to health-promoting information when the individuals recognize themselves as being highly susceptible to preventable diseases (9, 30).
Diet is among the most important modifiable lifestyle factors contributing to NCDs risk (9). Diets with high intake of energy-dense and highly refined carbohydrate foods are associated with obesity and type 2 diabetes (31). Changes in diet, especially a personalized diet, can prevent the onset of disease (9). Zeevi et al. continuously monitored glucose levels in an 800-person cohort, measured responses to 46,898 meals, and found high variability in the response to identical meals, suggesting that universal dietary recommendations may have limited utility (7). In the PREDICT1 study, a machine-learning model was created to predict both triglyceride and glycemic responses to food intake for developing personalized diet strategies (4). These leading-edge studies shed new light on the personalized diet intervention. In a previous RCT on PN, changing dietary intake to better align with the MedDiet—widely recognized as a healthy eating pattern—produced substantial health benefits (8). The participants who received PN intervention consumed less red meat, salt, and saturated fat, and had higher healthy eating index score (5). In our study, we observed similar dietary behavior changes in PNG, including increased consumption of whole grains, vegetables, especially dark green vegetables, fruits, dairy products, and nuts, and an improved CDGI, which again, validated that PN intervention is more efficacious than the conventional nutrition intervention. One of the novelties of the current study is the application of dietary supplements, especially among the subjects with high risk of deficiencies. Among all the supplements, MVM displayed the most potent efficacy by modulating serum TG, TC, homocysteine, and folic acid, and facilitating the decrease in BMI, body fat percentage and waist circumference. This was within expectation since MVM offered a wide range of nutrients, which might generate synergistic effects in promoting health outcomes. MVM would be an optimal choice for the overweight/obese adults who aim to lose weight.
In addition to diet, PA is another important modifiable lifestyle factor included in PN intervention (32). Sedentary behavior and physical inactivity are among the leading modifiable risk factors worldwide for cardiovascular disease and all-cause mortality (33). For all-cause mortality, spending > 3–4 h/day in watching television and > 6–8 h/day in any sitting activity have been suggested as detrimental (34). Although the health benefits of PA and exercise are evident, the research on personalized PA intervention is still limited. The Food4Me study showed that PA attenuated the effect of the FTO genotype on obesity traits in European adults (12). It is important to note that, subjects in the CG and PNG recieved the exercise notifications at the same frequency, so the differences in MET were not due to the subjects' awareness. Previous RCTs have shown that tailored training, or personal training, are superior to untailored trainging in promoting PA (35, 36), potentially through changing the participants' attitudes in PA. In this case, the subjects in the PNG might feel more motivated when they read the tailored notifications, which led to promoted PA duration and intensity. These findings suggested the important contribution of individualized PA advice to PN intervention.
PN advice promotes changes in individual dietary and PA behaviors, which may result in health or function improvement (10). In the P100 study, specific recommendations based on personal data were customized by a coach to help participants modify their behaviors, which potentially improved their clinical biomarkers (37). The Food4Me study successfully led to health-related behavioral changes but failed to bring significant improvement in clinical outcomes (5). In our study, following PN advice, the subjects improved their PA and nutrient intake, which was associated with the increase of beneficial nutrients in the blood and the decrease of “bad” blood biomarkers and anthropometrics. For instance, BMI decrease was associated with the increase of moderate MET, and the intake of dietary vitamin B2, B6, and B12. The results suggested that our PN intervention improved the clinical outcomes by changing the subjects' behavior. An important element to consider relating to the efficacy of PN intervention is the sustainability of behavior change, or longer-term adherence to personalized diet and lifestyle recommendations (10). That would require a precise and validated algorithm, professional and trustworthy guidance from registered dietitian, and compassionate and sustainable communication with a service team.
According to several publications, the PN intervention strategies that include genetic information have greater potential than the ones based on the phenotype alone for improving health (38–40). Adding a genetic component and disclosure of genetic information may bring crisis awareness and improve motivation and compliance (38). In addition, people who carry risk alleles may develop certain nutrient deficiency even when their intake meets recommended levels (41, 42). Since the efficacy of diet could be modulated or impaired by the SNPs of certain enzymes (43), it is critical to apply dietary supplements among the population that are genetically at risk of deficiencies. Although the PN intervention effects on the changes in anthropometric characteristics and blood biomarkers were similar for subjects with risk or non-risk allele of FTO, APOE, FADS1, and TCF7L2 genes, we still observed that subjects with a risk allele experienced greater improvement in certain clinical outcomes. Follow up studies are warranted in the future to further explore the association between genotype and PN intervention effect.
As expected, the decrease of BMI was significantly associated with the “lose weight” advice, since a series of suggestions were provided under the “lose weight” node, which included dietary changes and PA modifications, the most critical modifiable lifestyle factors that are related to weight loss (44). The B complex vitamins play an important role in maintaining energy homeostasis. Vitamin B2, for example, is a vital cofactor within the electron transport chain, Krebs cycle, and beta-oxidation (fat burning) (45), which explained why the increased vitamin B2 intake is a major contributor of BMI reduction. Vitamin B6 and vitamin B12 are involved in one-carbon metabolism, in which MTHFR plays a critical role (46). Although there is a lack of clear evidence of how promoted one-carbon metabolism is related to weight loss, it is reported that vitamin B intake is positively associated with fat-free mass in overweight/obese females (47), and according to a meta-analysis with 9,075 participants, higher serum vitamin B12 levels are inversely associated with obesity (48). Expectedly, as the first identified obesity-related gene, FTO polymorphisms affected weight loss. As an RNA N6-methyladenosine (m6A) demethylase, the group of FTO proteins are described as a regulator of m6A level of hormones, such as ghrelin, to modify energy intake and adipogenesis (23, 49). In addition to FTO and MTHFR, TMPRSS6 polymorphism is another key genetic factor in the longitudinal correlation network. This is interesting since no evidence has shown that TMPRSS6 affects energy metabolism. As reported, TMPRSS6 genotype influences iron metabolism, and the mutations in TMPRSS6 may lead to iron deficiency (27, 50). Previous studies have shown that a low iron level may result in exercise intolerance, weakness, and impaired muscle strength (51, 52). Iron supplementation in the iron-depleted females significantly improved their progressive fatigue resistance during exercise (53). Considering that the increase in moderate MET is one of the major contributors to BMI decrease, it is possible that the subjects with TMPRSS6 risk alleles may have experienced more fatigue during exercises, which potentially affected their PA duration and intensity, and subsequently sabotaged their plan on weight loss.
Admittedly, there are several limitations in this study. PN intervention is a holistic and integrated solution, in which continuous follow-up action and motivation are part of the whole PN intervention strategy to help the subjects achieve the goal (54). In this study, we cautiously designed the intervention of CG and PNG to minimize bias between the groups. Nevertheless, we admit that the intensity of intervention cannot be completely consistent between the groups, which is due to the nature of PN intervention (15, 54). Another limitation is the lack of objective biomarkers for assessing adherence. Unlike non-personalized dietary intervention trials, it is difficult to identify certain objective biomarker(s) to fully indicate the adherence in the personalized setting. As alternatives to objective biomarkers, CDGI, step count, and pill count were used in our study to show the compliance of diet, PA, and nutritional supplements, respectively. Previous studies have shown that microbiota phenotypes might modulate physiological responses to diet, especially postprandial TG, glucose, and insulin (4, 7). The analysis of “omics” data, such as metagenomics and metabolomics data, may provide a broad perspective on the changes of large sets of biomarkers (4, 55). In the near future, we will add more parameters to the PN report by including the multi-omics data.
Our current study has advanced our knowledge from previously published PN studies. It is important to note that this is the first RCT in China that showed the health benefits of PN intervention in overweight/obese adults, which provided a model of framework for developing the personalized advice that leads to modification of lifestyle and improvement of clinical outcomes. In previous studies, nutritional supplements were usually neglected by investigators, even though taking supplements is suggested when the residents are limiting dietary intake (56), which leaves a major gap of knowledge in the study of PN intervention. In contrast, we provided a holistic and integrated solution, containing both diet (supplements were used as a tool to fill the gap) and PA, to each individual in the PNG. Moreover, we combined two critical elements in the delivery of PN report: the validated decision trees as an algorithm to develop a PN report and the registered dietitians certificated to provide the solution. Currently, we are coding the decision trees to develop a mini application to carry out a real-world study, which may expand our data sources and facilitate our understanding on the efficacy of PN intervention in everyday environments.
In conclusion, we demonstrated that PN intervention showed greater benefits to health status in overweight/obese Chinese adults compared with the benefits from conventional intervention. PN intervention improved the clinical outcomes of anthropometric characteristics and blood biomarkers by changing the dietary and PA behaviors of subjects. The approach and the associated outcomes presented here open up the possibility for positive health outcomes in a general population should a similar program become widely available.
The original contributions presented in the study are included in the article/Supplementary Material, further inquiries can be directed to the corresponding author/s.
The studies involving human participants were reviewed and approved by the Institutional Review Board (IRB) of the Shanghai Nutrition Society. The participants provided their written informed consent to participate in this study.
JK, HG, and JD designed the research. JK, KX, and PW conducted the research. JN, FW, JC, and JZ analyzed the data. JK and JC wrote the manuscript. JK, JC, MR, JN, FW, and JZ revised the manuscript. JD and HG had primary responsibility for final content. All authors read and approved the final manuscript.
The authors declare that this study received funding from Amway. The funder was not involved in the study design, collection, analysis, interpretation of data, the writing of this article or the decision to submit it for publication.
All claims expressed in this article are solely those of the authors and do not necessarily represent those of their affiliated organizations, or those of the publisher, the editors and the reviewers. Any product that may be evaluated in this article, or claim that may be made by its manufacturer, is not guaranteed or endorsed by the publisher.
We would like to thank Dr. Li Zhang and his colleagues from SPRIM China for their excellent work in coordinating the clinical trial. We also appreciate the kind help from Prof. John Mathers for providing Food4Me decision trees and Shanghai Nutrition Society for providing support of registered dietitians. We thank LetPub (www.letpub.com) for its linguistic assistance during the preparation of this manuscript.
The Supplementary Material for this article can be found online at: https://www.frontiersin.org/articles/10.3389/fnut.2022.919882/full#supplementary-material
1. Collaboration NRF. Worldwide trends in body-mass index, underweight, overweight, and obesity from 1975 to 2016: a pooled analysis of 2,416 population-based measurement studies in 128·9 million children, adolescents, and adults. Lancet. (2017) 390:2627–42. doi: 10.1016/s0140-6736(17)32129-3
2. Nyberg ST, Batty GD, Pentti J, Virtanen M, Alfredsson L, Fransson EI, et al. Obesity and loss of disease-free years owing to major non-communicable diseases: a multicohort study. Lancet Public Health. (2018) 10:e490–7. doi: 10.1016/s2468-2667(18)30139-7
3. Hill JO, Wyatt HR, Peters JC. Energy balance and obesity. Circulation. (2012) 126:126–32. doi: 10.1161/circulationaha.111.087213
4. Berry SE, Valdes AM, Drew DA, Asnicar F, Mazidi M, Wolf J, et al. Human postprandial responses to food and potential for precision nutrition. Nat Med. (2020) 6:964–73. doi: 10.1038/s41591-020-0934-0
5. Celis-Morales C, Livingstone KM, Marsaux CF, Macready AL, Fallaize R, O'Donovan CB, et al. Effect of personalized nutrition on health-related behaviour change: evidence from the Food4Me European randomized controlled trial. Int J Epidemiol. (2017) 46:578–88. doi: 10.1093/ije/dyw186
6. Westerman K, Reaver A, Roy C, Ploch M, Sharoni E, Nogal B, et al. Longitudinal analysis of biomarker data from a personalized nutrition platform in healthy subjects. Sci Rep. (2018) 8:14685. doi: 10.1038/s41598-018-33008-7
7. Zeevi D, Korem T, Zmora N, Israeli D, Rothschild D, Weinberger A, et al. Personalized Nutrition by Prediction of Glycemic Responses. Cell. (2015) 163:1079–94. doi: 10.1016/j.cell.2015.11.001
8. Livingstone KM, Celis-Morales C, Navas-Carretero S, San-Cristobal R, Macready AL, Fallaize R, et al. Effect of an Internet-based, personalized nutrition randomized trial on dietary changes associated with the Mediterranean diet: the Food4Me study. Am J Clin Nutr. (2016) 104:288–97. doi: 10.3945/ajcn.115.129049
9. Palmnäs M, Brunius C, Shi L, Rostgaard-Hansen A, Torres NE, González-Domínguez R, et al. Perspective: metabotyping-A potential personalized nutrition strategy for precision prevention of cardiometabolic disease. Adv Nutr. (2020) 11:524–32. doi: 10.1093/advances/nmz121
10. Adams SH, Anthony JC, Carvajal R, Chae L, Khoo CSH, Latulippe ME, et al. Perspective: guiding principles for the implementation of personalized nutrition approaches that benefit health and function. Adv Nutr. (2020) 11:25–34. doi: 10.1093/advances/nmz086
11. Celis-Morales C, Marsaux CF, Livingstone KM, Navas-Carretero S, San-Cristobal R, Fallaize R, et al. Can genetic-based advice help you lose weight? Findings from the Food4Me European randomized controlled trial. Am J Clin Nutr. (2017) 105(5):1204–13. doi: 10.3945/ajcn.116.145680
12. Celis-Morales C, Marsaux CF, Livingstone KM, Navas-Carretero S, San-Cristobal R, O'Donovan C B, et al. Physical activity attenuates the effect of the FTO genotype on obesity traits in European adults: the Food4Me study. Obesity. (2016):962–9. doi: 10.1002/oby.21422
13. Yang Y, Yang X, Zhai F, Guo J, Su Y. Chinese Dietary Reference Intakes. Beijing: Science Press (2013). p. 649–660 (Society CN, editor.).
14. Zhu J, Gao R, Zhao S, Lu G, Zhao D, Jianjun L. Guidelines for the prevention and treatment of dyslipidemia in Chinese adults (revised in 2016). Chin Circ J. (2016) 31:937–53. doi: 10.3969/j.issn.1000-3614.2016.10.001
15. Celis-Morales C, Livingstone KM, Marsaux CF, Forster H, O'Donovan CB, Woolhead C, et al. Design and baseline characteristics of the Food4Me study: a web-based randomised controlled trial of personalised nutrition in seven European countries. Genes Nutr. (2015) 2:450. doi: 10.1007/s12263-014-0450-2
16. Arija V, Villalobos F, Pedret R, Vinuesa A, Jovani D, Pascual G, Basora J. Physical activity, cardiovascular health, quality of life and blood pressure control in hypertensive subjects: randomized clinical trial. Health Qual Life Outcomes. (2018) 16:184. doi: 10.1186/s12955-018-1008-6
17. Patterson E. Guidelines for Data Processing Analysis of the International Physical Activity Questionnaire (IPAQ)-Short Long Forms (2005). Available online at: https://biobank.ndph.ox.ac.uk/showcase/ukb/docs/ipaq_analysis.pdf
18. Huang L, Wang H, Wang Z, Zhang J, Zhang B, Ding G. Regional disparities in the association between cereal consumption and metabolic syndrome: results from the china health and nutrition survey. Nutrients. (2019) 11:764. doi: 10.3390/nu11040764
19. Huang F, Wang Z, Wang L, Wang H, Zhang J, Du W, et al. Evaluating adherence to recommended diets in adults 1991–2015: revised China dietary guidelines index. Nutr J. (2019) 18:70. doi: 10.1186/s12937-019-0498-3
20. Lietz G, Oxley A, Leung W, Hesketh J. Single nucleotide polymorphisms upstream from the β-carotene 15,15'-monoxygenase gene influence provitamin A conversion efficiency in female volunteers. J Nutr. (2012) 142:161s–5s. doi: 10.3945/jn.111.140756
21. Steluti J, Carvalho AM, Carioca AAF, Miranda A, Gattás GJF, Fisberg RM, Marchioni DM. Genetic Variants involved in one-carbon metabolism: polymorphism frequencies and differences in homocysteine concentrations in the folic acid fortification era. Nutrients. (2017) 9:539. doi: 10.3390/nu9060539
22. Roke K, Mutch DM. The role of FADS1/2 polymorphisms on cardiometabolic markers and fatty acid profiles in young adults consuming fish oil supplements. Nutrients. (2014) 6:2290–304. doi: 10.3390/nu6062290
23. Huang T, Qi Q, Li Y, Hu FB, Bray GA, Sacks FM, Williamson DA, Qi L. FTO genotype, dietary protein, and change in appetite: the preventing overweight using novel dietary strategies trial. Am J Clin Nutr. (2014) 99:1126–30. doi: 10.3945/ajcn.113.082164
24. Fallaize R, Celis-Morales C, Macready AL, Marsaux CF, Forster H, O'Donovan C, et al. The effect of the apolipoprotein E genotype on response to personalized dietary advice intervention: findings from the Food4Me randomized controlled trial. Am J Clin Nutr. (2016) 104:827–36. doi: 10.3945/ajcn.116.135012
25. Li L, Wang J, Ping Z, Li Y, Wang C, Shi Y, Zhou W, Zhang L. Interaction analysis of gene variants of TCF7L2 and body mass index and waist circumference on type 2 diabetes. Clin Nutr. (2020) 39:192–7. doi: 10.1016/j.clnu.2019.01.014
26. Kapur K, Johnson T, Beckmann ND, Sehmi J, Tanaka T, Kutalik Z, et al. Genome-wide meta-analysis for serum calcium identifies significantly associated SNPs near the calcium-sensing receptor (CASR) gene. PLoS Genet. (2010) 6:e1001035. doi: 10.1371/journal.pgen.1001035
27. Gan W, Guan Y, Wu Q, An P, Zhu J, Lu L, et al. Association of TMPRSS6 polymorphisms with ferritin, hemoglobin, and type 2 diabetes risk in a Chinese Han population. Am J Clin Nutr. (2012) 95:626–32. doi: 10.3945/ajcn.111.025684
28. Nissen J, Vogel U, Ravn-Haren G, Andersen EW, Madsen KH, Nexø BA, et al. Common variants in CYP2R1 and GC genes are both determinants of serum 25-hydroxyvitamin D concentrations after UVB irradiation and after consumption of vitamin D3-fortified bread and milk during winter in Denmark. Am J Clin Nutr. (2015) 101:218–27. doi: 10.3945/ajcn.114.092148
29. Nutrition and Health Status Report for Chinese Residents (2010–2013). Metabolic Syndrome. Gangqiang D, Yuna H, editors.: Beijing: People's Medical Publishing House. (2018).
30. de Roos B. Personalised nutrition: ready for practice? Proc Nutr Soc. (2013) 72:48–52. doi: 10.1017/s0029665112002844
31. Cordain L, Eaton SB, Sebastian A, Mann N, Lindeberg S, Watkins BA, et al. Origins and evolution of the Western diet: health implications for the 21st century. Am J Clin Nutr. (2005) 81:341–54. doi: 10.1093/ajcn.81.2.341
32. Ezzati M, Riboli E. Behavioral and dietary risk factors for noncommunicable diseases. N Engl J Med. (2013) 369:954–64. doi: 10.1056/NEJMra1203528
33. Lavie CJ, Ozemek C, Carbone S, Katzmarzyk PT, Blair SN. Sedentary Behavior, Exercise, and Cardiovascular Health. Circ Res. (2019) 124:799–15. doi: 10.1161/circresaha.118.312669
34. Patterson R, McNamara E, Tainio M, de Sá TH, Smith AD, Sharp SJ, et al. Sedentary behaviour and risk of all-cause, cardiovascular and cancer mortality, and incident type 2 diabetes: a systematic review and dose response meta-analysis. Eur J Epidemiol. (2018) 33:811–29. doi: 10.1007/s10654-018-0380-1
35. McClaran SR. The effectiveness of personal training on changing attitudes towards physical activity. J Sports Sci Med. (2003) 2:10–4.
36. Marcus BH, Emmons KM, Simkin-Silverman LR, Linnan LA, Taylor ER, Bock BC, et al. Evaluation of motivationally tailored vs. standard self-help physical activity interventions at the workplace. Am J Health Promot. (1998) 12:246–53. doi: 10.4278/0890-1171-12.4.246
37. Price ND, Magis AT, Earls JC, Glusman G, Levy R, Lausted C, et al. A wellness study of 108 individuals using personal, dense, dynamic data clouds. Nat Biotechnol. (2017) 35:747–56. doi: 10.1038/nbt.3870
38. Arkadianos I, Valdes AM, Marinos E, Florou A, Gill RD, Grimaldi KA. Improved weight management using genetic information to personalize a calorie controlled diet. Nutr J. (2007) 6:29. doi: 10.1186/1475-2891-6-29
39. Nielsen DE, El-Sohemy A. Disclosure of genetic information and change in dietary intake: a randomized controlled trial. PLoS ONE. (2014) 9:e112665. doi: 10.1371/journal.pone.0112665
40. Hietaranta-Luoma HL, Tahvonen R, Iso-Touru T, Puolijoki H, Hopia A. An intervention study of individual, apoE genotype-based dietary and physical-activity advice: impact on health behavior. J Nutrigenet Nutrigenomics. (2014) 7:161–74. doi: 10.1159/000371743
41. Rivera-Paredez B, Macías N, Martínez-Aguilar MM, Hidalgo-Bravo A, Flores M, Quezada-Sánchez AD, et al. Association between Vitamin D deficiency and single nucleotide polymorphisms in the Vitamin D receptor and GC genes and analysis of their distribution in mexican postmenopausal women. Nutrients. (2018) 10:1175. doi: 10.3390/nu10091175
42. Liu CS, Chen CH, Chiang HC, Kuo CL, Huang CS, Cheng WL, et al. B-group vitamins, MTHFR C677T polymorphism and carotid intima-media thickness in clinically healthy subjects. Eur J Clin Nutr. (2007) 61:996–1003. doi: 10.1038/sj.ejcn.1602606
43. Moran NE, Thomas-Ahner JM, Fleming JL, McElroy JP, Mehl R, Grainger EM, et al. Single nucleotide polymorphisms in β-Carotene Oxygenase 1 are associated with plasma lycopene responses to a tomato-soy juice intervention in men with prostate cancer. J Nutr. (2019) 149:381–97. doi: 10.1093/jn/nxy304
44. Management. IoMUSoMW. Weight Management: State of the Science and Opportunities for Military Programs. Washington (DC): National Academies Press (US); 2004. (4, Weight-Loss and Maintenance Strategies.). Available online at: https://www.ncbi.nlm.nih.gov/books/NBK221839/
45. LeMond G, Hom M. 5 - Mitochondrial Supplements. In: LeMond G, Hom M, editors. The Science of Fitness. Boston: Academic Press (2015). p. 65–70.
46. Niforou A, Konstantinidou V, Naska A. Genetic variants shaping inter-individual differences in response to dietary intakes-a narrative review of the case of vitamins. Front Nutr. (2020) 7:558598. doi: 10.3389/fnut.2020.558598
47. Rodríguez-Rodríguez E, López-Sobaler AM, Navarro AR, Bermejo LM, Ortega RM, Andrés P. Vitamin B6 status improves in overweight/obese women following a hypocaloric diet rich in breakfast cereals, and may help in maintaining fat-free mass. Int J Obes. (2008) 32:1552–8. doi: 10.1038/ijo.2008.131
48. Sun Y, Sun M, Liu B, Du Y, Rong S, Xu G, Snetselaar LG, Bao W. Inverse association between serum vitamin B12 concentration and obesity among adults in the United States. Front Endocrinol. (2019) 10:414–14. doi: 10.3389/fendo.2019.00414
49. Karra E, O'Daly OG, Choudhury AI, Yousseif A, Millership S, Neary MT, et al. A link between FTO, ghrelin, and impaired brain food-cue responsivity. J Clin Invest. (2013) 123:3539–51. doi: 10.1172/JCI44403
50. Finberg KE, Heeney MM, Campagna DR, Aydinok Y, Pearson HA, Hartman KR, et al. Mutations in TMPRSS6 cause iron-refractory iron deficiency anemia (IRIDA). Nat Genet. (2008) 40:569–71. doi: 10.1038/ng.130
51. Wouthuyzen-Bakker M, van Assen S. Exercise-induced anaemia: a forgotten cause of iron deficiency anaemia in young adults. Br J Gen Pract. (2015) 65:268–9. doi: 10.3399/bjgp15X685069
52. Neidlein S, Wirth R, Pourhassan M. Iron deficiency, fatigue and muscle strength and function in older hospitalized patients. Eur J Clin Nutr. (2021) 75:456–63. doi: 10.1038/s41430-020-00742-z
53. Brutsaert TD, Hernandez-Cordero S, Rivera J, Viola T, Hughes G, Haas JD. Iron supplementation improves progressive fatigue resistance during dynamic knee extensor exercise in iron-depleted, non-anemic women. Am J Clin Nutr. (2003) 77:441–8. doi: 10.1093/ajcn/77.2.441
54. Contento IR. Nutrition education: linking research, theory, and practice. Asia Pac J Clin Nutr. (2008) 17(Suppl 1):176–9.
55. Chen R, Mias GI, Li-Pook-Than J, Jiang L, Lam HY, Chen R, et al. Personal omics profiling reveals dynamic molecular and medical phenotypes. Cell. (2012) 148:1293–307. doi: 10.1016/j.cell.2012.02.009
56. U.S. Department of Agriculture and U.S. Department of Health and Human Services. Dietary Guidelines for Americans, 2020–2025. 9th ed. (2020). Available online at: https://www.dietaryguidelines.gov/resources/2020-2025-dietary-guidelines-online-materials
Keywords: personalized nutrition, diet, physical activity, genotype, phenotype, clinical trial
Citation: Kan J, Ni J, Xue K, Wang F, Zheng J, Cheng J, Wu P, Runyon MK, Guo H and Du J (2022) Personalized Nutrition Intervention Improves Health Status in Overweight/Obese Chinese Adults: A Randomized Controlled Trial. Front. Nutr. 9:919882. doi: 10.3389/fnut.2022.919882
Received: 14 April 2022; Accepted: 20 May 2022;
Published: 22 June 2022.
Edited by:
Kirsty Pourshahidi, Ulster University, United KingdomCopyright © 2022 Kan, Ni, Xue, Wang, Zheng, Cheng, Wu, Runyon, Guo and Du. This is an open-access article distributed under the terms of the Creative Commons Attribution License (CC BY). The use, distribution or reproduction in other forums is permitted, provided the original author(s) and the copyright owner(s) are credited and that the original publication in this journal is cited, in accordance with accepted academic practice. No use, distribution or reproduction is permitted which does not comply with these terms.
*Correspondence: Jun Du, RXJpYy5kdUBhbXdheS5jb20=; Hongwei Guo, aHdndW9Ac2htdS5lZHUuY24=
†These authors have contributed equally to this work and share first authorship
Disclaimer: All claims expressed in this article are solely those of the authors and do not necessarily represent those of their affiliated organizations, or those of the publisher, the editors and the reviewers. Any product that may be evaluated in this article or claim that may be made by its manufacturer is not guaranteed or endorsed by the publisher.
Research integrity at Frontiers
Learn more about the work of our research integrity team to safeguard the quality of each article we publish.