- 1Department of Epidemiology and Biostatistics, School of Public Health, Jilin University, Changchun, China
- 2Department of Orthopedics, The Second Clinical Hospital, Jilin University, Changchun, China
- 3Department of Nutrition and Food Hygiene, School of Public Health, Jilin University, Changchun, China
Objective: We aimed to investigate the association between different eating habit patterns and mental health problems among Chinese middle and high school students, and further to estimate the interaction effect of different grouping variables on eating habits.
Methods: One thousand three hundred and forty-eight adolescents from Jilin Province in China were involved in this cross-sectional study. Mental health and eating habits were assessed using General Health Questionnaire and questions on Nutrition Knowledge, Attitude and Practice, respectively. Latent class analysis (LCA) was performed to identify eating habit patterns. Binary logistic regression and generalized linear models were used to explore the association between eating habit patterns, energy-adjusted nutrient intakes and mental health problems. Interaction analysis was performed to analyze the association between eating habits and mental health in different groups.
Results: Based on the LCA results, a 3-class parallel model was identified: 648 adolescents (48.1%) were classified in class-1 “Healthy Eating Behavior/Eating at Home,” 452 adolescents (33.5%) in class-2 “Healthy Eating Behavior/Eating at School” and 248 adolescents (18.4%) in class-3 “Unhealthy Eating Behavior/Random Place.” Compared with class-1, participants in class-2 and class-3 were at higher risk of mental health problems, especially for class-3 (p < 0.05). The energy and nutrient intakes by different latent classes showed that adolescents who ate unhealthy had lower daily intake of energy, protein, carbohydrate, fiber, Vitamins and minerals (p < 0.05). The interaction between age, sleep duration and different eating habits was statistically significant (p for interaction < 0.1).
Conclusion: “Unhealthy eating behavior/random place pattern” was positively correlated with mental health problems of adolescents. The adolescents with health diet were accompanied by fewer mental health problems, especially for that eating at home. And there were interactions between eating habits and age, sleep duration on the mental health problems.
Introduction
Mental health problems affect 10–20% of young people around the world (1). Adolescence is a period of great and rapid changes in students' physiology and habit (2). Many health-related bad behaviors would appear in this period, which increased the risk of mental health problems (3). Mental health status was closely related to health-related problems of young people, especially the decline of academic performance, poor interpersonal relationship, violence and drug abuse. Mental disorders also placed a heavy burden on young people's mortality, mainly due to significantly higher suicide rates among youth with mental health problems. And suicide was the leading cause of death among people aged 15–24 (1). The factors that affected mental health problems were complex and diverse, such as physical health, unhealthy lifestyle and family factors (3–5).
Although the determinants of mental health were complex, more and more studies showed that unhealthy eating habits were related to mental health (6–8). Teenagers were often exposed to unhealthy foods, such as takeout and high calorie snacks, and their meals were irregular. Research showed that people who regularly ate snacks at midnight (4) and skipped breakfast (9) were more likely to have psychological problems such as depression and increased psychological stress. And some studies pointed out that there were significant differences in dietary nutrition intake between students who ate at home and those who ate at school (10). However, the relationship between dietary patterns including eating locations and mental health status was unclear among adolescents (11–14).
Previous studies on mental health and nutrition of adolescents mainly focused on specific nutrients and foods or specific eating habits. The high correlation between various foods or eating habits made it difficult to attribute the impact to a single independent component, which limited the interpretation and application of the results (15). Due to the presence of dietary patterns, the association between diet and mental health outcomes was simplified and robust. The influence of overall diet on mental health may be more likely to translate into dietary advice and public health information (16). Moreover, most diet studies focus on the food types of “dietary patterns.” For example, a study in Norway divided the eating patterns into three types: varied Norwegian/junk or convenient/snacking (17). However, few studies integrated adolescent eating habit and eating environment into different patterns.
Although various methods were used to cluster different eating patterns, there were still great differences in accuracy. Recently, latent class analysis (LCA) has been applied to nutritional epidemiology (18). Compared with factor analysis, LCA is a human-centered analysis method, which classifies samples into several mutually exclusive categories to explore the latent variables behind the categorical explicit variables with statistical correlation (19). And a Chinese study showed that LCA was more suitable for the study of dietary structure and disease risk than factor analysis (20).
Therefore, this study used LCA to analyze the eating habit patterns of adolescents in Jilin Province of China, to explore the association between different potential categories of eating habits and mental health problems, and further to estimate the interaction effect of different grouping variables on eating habits, so as to provide a scientific basis for the development of targeted health intervention measures.
Methods
Data source and study population
This cross-sectional study was questionnaire-based, which was conducted from August 2019 to December 2020 in Jilin Province, China, funded by the Department of Science and Technology of Jilin Province, China. A total of 1,875 adolescents were investigated in this survey. We excluded participants who had missing data on GHQ score or covariates, those who reported extreme total energy intake (outside the mean ± 3 standard deviations [SD]). Finally, a total of 1,348 adolescents were involved in our study, with an average age of 14.49 ± 1.99 years. This study covered students from 6 high schools and 6 middle schools in 9 cities in Jilin Province, and adopted the principle of multi-stage stratified random sampling and the form of face-to-face interview.
The study protocol was approved by the Ethics Committee of Jilin University (batch number: 20180515). All participants provided written informed consent. Research was conducted in accordance with the Helsinki Declaration as revised 1989.
Data collection
Data collection occurred in two steps. The first step was to measure anthropometric parameters. Body composition analyzer of Tsinghua Tongfang BAC-2A was used to measure body weight and body mass index (BMI). Height measurements (without shoes) were taken with a height ruler. In our study, IOTF criteria for Asian children and adolescents were selected to determine BMI cut-off values of 23 and 27 kg/m2 for overweight and obesity (21).
The second step was the questionnaire. Students were asked to complete a nutrition status questionnaire, which was divided into four main parts referred to as follows: Section A was the basic information questionnaire, including gender, age, grade, nation and other information. Section B was the General Health Questionnaire (GHQ), which was originally designed by Goldberg in 1972 to examine the mental health status of respondents in community settings and non-psychiatric clinical settings (22). In this study, the Chinese version of GHQ-20 revised by Li was used to reflect the mental health status of subjects in the past month, including 20 items from three dimensions of self-identity, depression and anxiety, which was a useful scale to measure the mental health of students (23). Each item has a score of 0 or 1, and the sum of self-affirmation, depression, and anxiety sub-score is the GHQ total score, ranging from 0 to 20. The higher the total GHQ score, the worse the individual's mental health. The cut-off point for “case” recognition in GHQ-20 is between 3 and 4, with sensitivity and specificity of 78 and 85%, respectively, compared to the DSM-IL criteria (24). Section C included questions on nutrition knowledge, attitude and practices (KAP). The data we used on eating habits was from the nutritional practices section of the KAP questionnaire, which contained 5 questions, as shown in Table 1. The contents of the questionnaire were formulated on the basis of previous studies (25). Section D was the food frequency questionnaire (FFQ), which was a validated semi-quantitative food questionnaire and included more than 170 kinds of food in 11 categories, including cereals, beans, fungi and algae, vegetables and fruits. Both the reproducibility (r value = 0.5–0.8) and validity (r value = 0.3–0.6) of the instrument were acceptable, as reported elsewhere (26, 27). The intake frequency of each food should be filled in according to times/day, times/week, times/month, or times/year. Meanwhile, the consumption of each food should be recalled and recorded to obtain the daily intake of each food, and the food intake level and nutrients of the subjects were estimated based on the results of dietary frequency questionnaire. Under the guidance of nutrition experts from the School of Public Health, Jilin University, China, before the widespread use of this questionnaire, quality investigators conducted pre-experiments to verify the feasibility and comprehensiveness of the questionnaire and survey process.
Statistical analyses
This study clustered eating behaviors by LCA to form different eating habits patterns of adolescents. LCA was conducted using MPlus 8.3 as indicators for each item of eating habits. LCA is a finite mixed model that identifies meaningful homogeneous groups of individuals in a population given a set of measurement variables. We compared LCA for 2–5 categories, these criteria were used to determine the best model: (1) The lower Bayesian Information Criterion (BIC) or the lower Akaike Information Criterion (AIC) values indicate that the model fits well. (2) The p-values corresponding to the Lo-Mendell-Rubin adjusted likelihood ratio test (LMR) and bootstrapped likelihood ratio test (BLRT) indicators reach a significant level (p < 0.05), indicating that the models of the k classes are significantly superior to the models of the (k−1) classes. (3) The Entropy value ranges from 0 to 1. When Entropy ≥ 0.80 indicates that the classification accuracy reaches 90%. The higher the Entropy value, the higher the classification accuracy (28).
Continuous variables were presented as mean and standard deviation (SD) or Median (25–75th Percentile). Wilcoxon signed rank test was used for comparison between the two groups. Categorical variables were presented as case and percentage and were compared using Pearson's Chi-square test. We took the total score of GHQ-20 from 3 to 4 as the cut-off point to form a binary variable. Next, we used binary logistic regression to evaluate the association between eating habit patterns and mental health problems. Model 2 was adjusted for age and gender; Model 3 was adjusted for variables in model 2 plus physical activity, sleep duration and passive smoking. Generalized linear models were applied to investigate the comparison of energy and nutrient intakes by latent classes with post-hoc pairwise comparisons conducted using the Bonferroni correction. Generalized linear models were controlled for covariates including age, gender physical activity, sleep duration and passive smoking. When interactions were significant (p for interaction < 0.1), stratified analyses were performed. P < 0.05 was considered statistically significant. The analysis was performed using SPSS24.0.
Results
The demographic characteristics of the participants were presented in Table 2. In total, 704 (52.2%) adolescents had mental health problems and 644 (47.8%) adolescents did not, of whom 47.8% were male and 52.2% were female. The average age of the sample was 14.49 years old, of whom 59.1% were junior middle school students. And most of the students were Han nationality (83.4%). The proportion of female with mental health problems was higher than that of male adolescents (p = 0.006). In addition, compared with the adolescents without mental health problems, most of the adolescents with mental health problems had fewer physical activities, shorter sleep duration and were passive smokers (p < 0.05).
The association between eating habits and GHQ scores and their subscales has shown in Table 3. The total score of GHQ, as well as the scores of self-affirmation, depression and anxiety sub-score had statistical significance in different breakfast frequency, preference for roadside stall and frequency of night snack (p < 0.05). Regular breakfast eating was positively associated with mental health. And the mental health status of people who ate take out and fast food for lunch and dinner was poor (p < 0.05). In addition, people who liked to eat roadside stalls and often ate night snack had higher GHQ scores (p < 0.001).
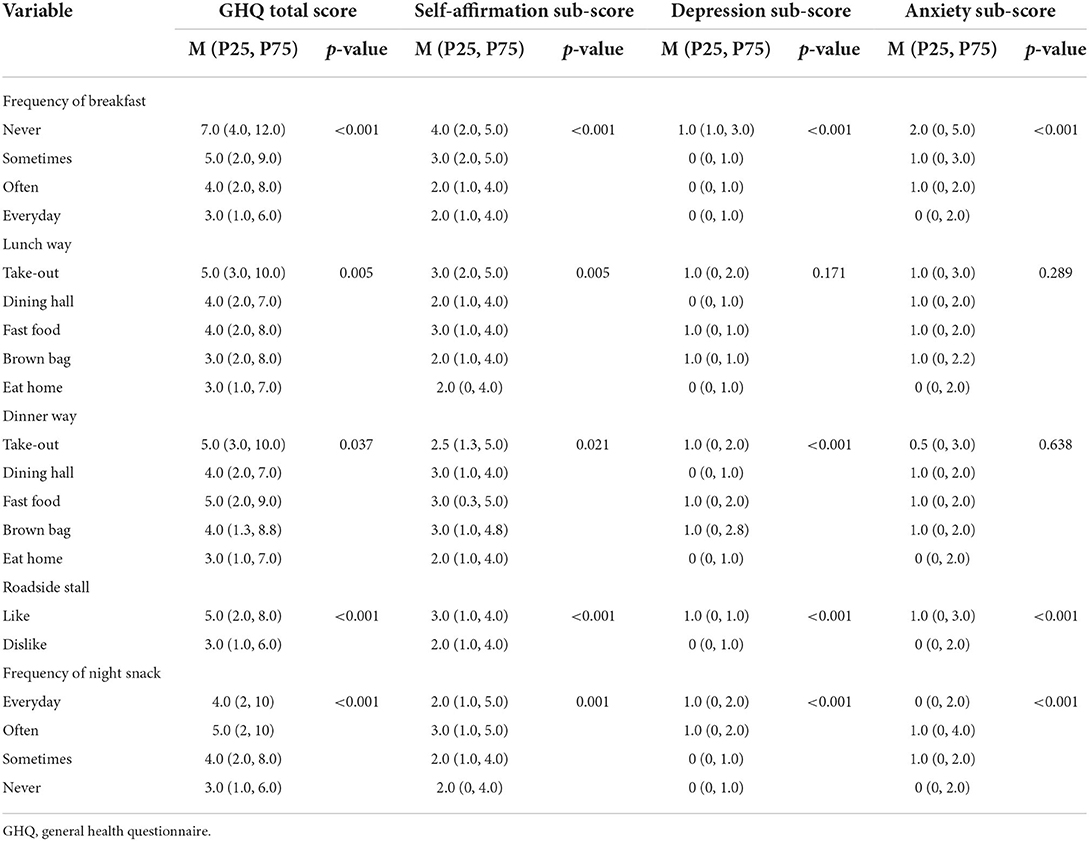
Table 3. Nutritional behavior based on GHQ total score, self-affirmation sub-score, anxiety sub-score, and depression sub-score of the sample.
Model fit indicators for solutions with 2 through 5 classes were summarized in Table 4, and three eating habit pattern classes were chosen. To determine the most optimal classification, we first assumed that there were only two classes for all subjects, and gradually increased the number of classes until we found the best model to fit the data. BLRT significance test showed that increasing the number of classifications might improve the model, but LMR showed no statistical significance for 4-class model, indicating that there was no significant improvement in model fitting between 3-class model and 4-class model. When the model class was 3, LMR and BLRT had p < 0.05, and the entropy was 0.745. For these reasons, the 3-class model was selected as the optimal models in terms of suitability, simplicity and interpretability for further analysis. This solution created 3 mutually exclusive and collectively exhaustive groups into which an individual was assigned to one class based on their greatest likelihood. The different eating habits conditional probability from the selected eating habit patterns for the three classes was presented in Table 5.
Figure 1 showed the project probabilities for each of the three categories of solutions, and we provided subjective labels for each category. Class 1, accounting for 48.1% of the sample (n = 648), was termed “healthy eating behavior/eating at home,” because adolescents in this class had regular breakfast, mostly ate at home for lunch and dinner, and did not often eat roadside stalls and night snacks. The class 2 accounted for 33.5% of the sample (n = 452), and were termed “healthy eating behavior/eating at school.” Adolescents in this class had regular breakfast, and most of them had lunch and dinner in the school canteen. These people did not eat night snacks very often, and nearly half of them didn't like to eat roadside stalls. Class 3, accounting for 18.4% of the sample (n = 248), was termed as “unhealthy eating behavior/random place,” because the teenagers in this class did not have regular eating habits and eating places, most of them skipped breakfast and school meals, preferred roadside stalls, and had a higher rate (34.1%) of eating late-night snacks than the other two classes. This pattern was deemed unhealthy.
Table 6 summarized binary logistic regression analysis using class-1 (healthy eating behavior/eating at home) as the reference. Univariate analysis showed that class-2 (healthy eating behavior/eating at school) and class-3 (unhealthy eating behavior/ random place) were associated with a higher mental health risk (p < 0.05), and the harmful effect of class-3 [adjusted Odds Ratio (OR) = 2.15, 95% Confidence Interval (CI) =1.59, 2.91] on mental health was higher than that of class-2 (adjusted OR = 1.59, 95% CI = 1.25, 2.02). After further adjusting for age, gender, physical activity, sleep duration, and passive smoking in model 3, the ORs (95% CIs) of class-2 (healthy eating behavior/eating at school) and class-3 (unhealthy eating behavior/random place) for mental health problems comparing with class-1 (healthy eating behavior/eating at home) were 1.73 (1.27, 2.38) and 2.23 (1.62, 3.08). The correlation between the two variables did not change (p < 0.05).
Table 7 presented the comparison of energy and nutrient intakes by different latent classes. After adjustment for multiple covariates, adolescents with an “unhealthy eating behavior/random place pattern” had lower daily intakes of energy, protein, dietary fiber, vitamins and minerals, and higher intakes of total fat (p < 0.05). Compared with “healthy eating behavior/eating at home” adolescents, adolescents who ate at school had higher intakes of minerals (p < 0.05), but there was no statistically significant difference in nutrient intakes (p ≥ 0.05). The results of pairwise comparisons showed that there was no statistically significant difference in the percentage (%) of energy provided by carbohydrates among the three groups (p ≥ 0.05).
Table 8 showed the interaction between eating habits and adolescents' mental health problems in different subgroups, and we performed subgroup analyses by different variables. The results showed that adolescents aged <14 years old had a better correlation between eating habits and mental health problems (p for interaction = 0.003). The ORs (95% CIs) of class-3 (unhealthy eating behavior/random place pattern) for mental health problems comparing with class-1 (healthy eating behavior/eating at home pattern) were 2.37 (1.52, 3.69). There was also a significant difference in the association between eating habit patterns and mental health problems among different sleep duration groups (p for interaction = 0.001). In the group with sleep duration of 6–8 h, unhealthy eating behavior was strongly associated with mental health problems (adjusted OR = 2.91, 95% CI = 1.88, 4.51). And we did not find an association between eating habit patterns and mental health in groups with adequate sleep duration (≥8 h) and short sleep duration (<6 h). Furthermore, gender (p for interaction = 0.387) and passive smoking (p for interaction = 0.290) did not significantly alter the association between eating habits and mental health problems.
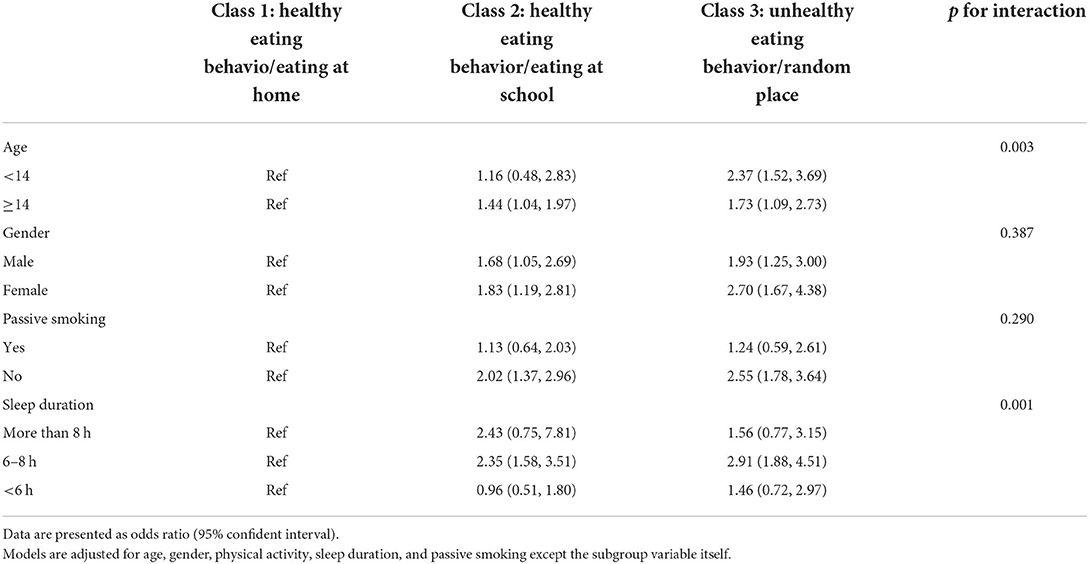
Table 8. Multivariable-adjusted odds ratios for the association between eating habits and mental health problems by subgroups.
Discussion
In this study, we used LCA to analyze the association between eating habits and mental health problems among adolescents in Jilin Province. Our study identified three patterns, “healthy eating behavior/eating at home,” “healthy eating behavior/eating at school“ and ”unhealthy eating behavior/random place.” Participants in the “unhealthy eating behavior/random place pattern” had lower daily intake of energy, protein, carbohydrate, fiber, Vitamins and minerals. Unhealthy eating habits were associated with higher mental health risks. The adolescents with health diet were accompanied by fewer mental health problems, especially for that eating at home. Subgroup analysis showed that the association still persisted in age <14 years and participants with less sleep duration (6–8 h).
The incidence of mental health problems among Chinese adolescents in our study was high (52.2%), which was consistent with recent reports (29, 30). There were several factors that may lead to changes in mental health, such as the increase of academic pressure, the change of relationship with classmates or parents, bad behavior (5). Previous reports have pointed out that many people choose to relieve stress by overeating or eating unhealthy food (31). However, studies pointed out that unhealthy diet had no benefit in reducing psychological stress (32). The conclusion was controversial. But study examining eating habit patterns in relation to mental health was lacking. In line with these findings, our study explored the impact of eating habits on adolescents' mental health. We observed that the “unhealthy eating behavior/random place pattern” observed in the present study was associated with higher mental health risk, compared with the “healthy eating behavior/eating at home pattern” and “healthy eating behavior/eating at school pattern,” after adjusting for sociodemographic and lifestyle factors. This finding was consistent with literature reporting a positive association between unhealthy eating behavior and mental health problems.
Epidemiological studies suggested adolescents who didn't eat breakfast were more likely to have elevated stress, depression and emotional distress (7). An earlier study among Chinese teenagers found that college students who ate breakfast almost every day tended to choose healthier food all day (33). A study showed that skipping breakfast was associated with increased cortisol (34), which in turn increased the risk of mental health problems such as anxiety and depression (35). A survey of four provinces in China pointed out that the increased consumption of fast food and sugary drinks had a great impact on teenagers' psychological symptoms (36). The possible explanation was that takeout, fast food and roadside stalls were related to pro-inflammatory diet. Studies pointed out that there was a significant positive correlation between pro-inflammatory diet and the risk of depression (37). In addition, compared with the food at home, fast food and roadside stalls were often less healthy, because these foods contained higher fat, salt and sugar and less nutrients, which were related to various negative health outcomes (38, 39).
Previous studies formed different eating patterns based on dietary intake and eating habits (15–17), but these studies did not consider the impact of eating place on adolescents' mental health. We incorporated eating location into eating patterns, which emphasized that direct food environment might have an impact on adolescents' mental health. Our research results indicated that eating at home had a higher protective effect on adolescents' mental health than eating at school. Children who ate at home had higher intake of core foods (grains, vegetables, fruits, fish, meat, nuts and dairy products) (10). Studies on nutritional intake of children and adolescents in Europe showed that eating at home was associated with higher nutritional intake and lower dietary energy density (40). A study pointed out that regular family meals might promote healthier eating habits, improve parent-child relationship and reduce the risk of depressive symptoms in adolescents (5). Children eating with their parents helped to improve their life satisfaction and emotional stability, and reduced high-risk behaviors such as suicide attempts, antisocial behavior, violence and extreme dieting (14).
Previous research pointed out that limited food and vegetable variety in school cafeterias may lead to inadequate vitamin and mineral intake among teens, and adolescents preferred to buy energy-dense foods in the cafeteria (41, 42). But we did not find similar results in our study. Our results found no significant differences in energy and nutrient intake between home and school meals among adolescents eating healthy eating behaviors. School diets and mental health often involve the interaction of multiple influences and factors. The reason for the poor mental health of adolescents who ate at school may be that most of these students live in dormitories, and they were more likely to encounter problems such as peer influence, school atmosphere pressure, and interpersonal relationships (43, 44). This prompted us to pay more attention to the mental health of students who ate at school.
In the subgroup analysis of this study, we found that the association between unhealthy eating habits and mental health problems was present in younger participants, which was consistent with previous research (11). A potential reason for the age difference may be that younger children were not as good at dealing with mental health issues as older children (45). Furthermore, the relationship was also related to sleep duration. Eating habits had a significant effect on the mental health of participants with a sleep duration of 6–8 h, but there was no correlation when the duration was <6 h. This may be because shorter sleep had a greater impact on mental health status (46), but the relationship between the three need to be further studied. In the findings of this study, girls were more likely to experience mental health problems, and gender differences in mental health risks during adolescence were widely recognized (12). However, we did not find an interaction between gender and eating habits. This was also consistent with results from previous studies showing no signs of gender differences between eating habits and mental health (12, 13).
Our findings have clinical and policy implications. First of all, schools should pay attention to the assessment of students' mental health, and suggest to carry out mental health promotion activities for parents. Educators should promptly identify high-risk groups for early psychological intervention. In addition, we promote strategies for families to eat together. More importantly, schools should strengthen supervision over the sale of unhealthy foods in the surrounding environment, strengthen nutrition and health education for youth schools and families, and cultivate students to form healthy eating habits.
The advantages of this study included the use of LCA as a novel method to obtain three different eating habit patterns according to teenagers' eating places and eating characteristics. The dietary patterns of adolescents were assessed from the overall level, which was more conducive to macro-regulation of diets and more easily translated into dietary recommendations and public health information. And the sample size of the study was relatively large and representative, which can well-reflect the dietary situation of middle school students in Northeast China. Finally, our study explored the association between eating habits and mental health problems stratified by age, sex, and sleep duration, which was conducive to the timely detection of the adverse mental state and take measures.
There were some limitations that should be considered in interpreting the study results. First of all, this study was a cross-sectional study, we cannot judge the causal association between eating habits and mental health status. However, a longitudinal research results did not support the hypothesis of reverse causality (47). Another limitation was that the mental health scale used in this study was not a clinical diagnostic standard scale, so the interpretation of the results should be more cautious. Finally, study outcomes were assessed using self-reported questionnaires and were susceptible to recall bias and misreporting.
Conclusion
Our results supported the hypothesis that eating habit affected adolescents' mental health. “Unhealthy eating behavior/random place pattern” was positively correlated with mental health problems of adolescents. The protective effect of healthy eating behavior/eating at home on mental health was higher than that of healthy eating behavior/eating at school. And there were interactions between eating habits and age, sleep duration.
Data availability statement
The original contributions presented in the study are included in the article/supplementary material, further inquiries can be directed to the corresponding author/s.
Author contributions
YL and XL made the study design. BL, NY, and JL conducted the study. XL, MS, and RG analyzed the data and wrote the manuscript. LW, WH, and YY attended the manuscript revision. All authors agreed with the final manuscript.
Funding
This work was supported by the Department of Science and Technology of Jilin Province, China (No. 20180623001TC).
Acknowledgments
We sincerely thank all the adolescents and their schools for their support.
Conflict of interest
The authors declare that the research was conducted in the absence of any commercial or financial relationships that could be construed as a potential conflict of interest.
Publisher's note
All claims expressed in this article are solely those of the authors and do not necessarily represent those of their affiliated organizations, or those of the publisher, the editors and the reviewers. Any product that may be evaluated in this article, or claim that may be made by its manufacturer, is not guaranteed or endorsed by the publisher.
References
1. Jurewicz I. Mental health in young adults and adolescents: supporting general physicians to provide holistic care. Clin Med. (2015) 15:151–4. doi: 10.7861/clinmedicine.15-2-151
2. Louis-Jacques J, Samples C. Caring for teens with chronic illness: risky business? Curr Opin Pediatr. (2011) 23:367–72. doi: 10.1097/MOP.0b013e3283481101
3. Ng P, Chan K-F, Ho W-C. A study on mental health of secondary school students in three metropolitan cities in China: Hong Kong, Shanghai, and Beijing. Int J Adolesc Med Health. (2008) 20:53–62. doi: 10.1515/IJAMH.2008.20.1.53
4. Colles SL, Dixon JB, O'Brien PE. Night eating syndrome and nocturnal snacking: association with obesity, binge eating and psychological distress. Int J Obes. (2007) 31:1722–30. doi: 10.1038/sj.ijo.0803664
5. Moitra P, Madan J, Shaikh NI. Eating habits and sleep patterns of adolescents with depression symptoms in Mumbai, India. Maternal Child Nutr. (2020) 16:e12998. doi: 10.1111/mcn.12998
6. Lee KS, Vaillancourt T. Longitudinal associations among bullying by peers, disordered eating behavior, and symptoms of depression during adolescence. JAMA Psychiatry. (2018) 75:605–12. doi: 10.1001/jamapsychiatry.2018.0284
7. Pengpid S, Peltzer K. Skipping breakfast and its association with health risk behaviour and mental health among university students in 28 countries. Diab Metabol Syndrome Obesity Targets Ther. (2020) 13:2889–97. doi: 10.2147/DMSO.S241670
8. Muscaritoli M. The impact of nutrients on mental health and well-being: insights from the literature. Front Nutr. (2021) 8:97. doi: 10.3389/fnut.2021.656290
9. Overby N, Hoigaard R. Diet and behavioral problems at school in norwegian adolescents. Food Nutr Res. (2012) 56:17231. doi: 10.3402/fnr.v56i0.17231
10. Ziauddeen N, Page P, Penney TL, Nicholson S, Kirk SFL, Almiron-Roig E. Eating at food outlets and leisure places and “on the Go” is associated with less-healthy food choices than eating at home and in school in children: cross-sectional data from the UK national diet and nutrition survey rolling program (2008–2014). Am J Clin Nutr. (2018) 107:992–1003. doi: 10.1093/ajcn/nqy057
11. Li S, Chen K, Liu C, Bi J, He Z, Luo R, et al. Dietary diversity and mental health in preschoolers in rural China. Public Health Nutr. (2021) 24:1869–76. doi: 10.1017/S1368980020003237
12. Sinclair R, Millar L, Allender S, Snowdon W, Waqa G, Jacka F, et al. The cross-sectional association between diet quality and depressive symptomology amongst fijian adolescents. PLoS ONE. (2016) 11:e0161709. doi: 10.1371/journal.pone.0161709
13. Huang P, O'Keeffe M, Elia C, Karamanos A, Goff LM, Maynard M, et al. Fruit and vegetable consumption and mental health across adolescence: evidence from a diverse urban British cohort study. Int J Behav Nutr Phys Act. (2019) 16:19. doi: 10.1186/s12966-019-0780-y
14. Fulkerson JA, Story M, Mellin A, Leffert N, Neumark-Sztainer D, French SA. Family dinner meal frequency and adolescent development: relationships with developmental assets and high-risk behaviors. J Adolescent Health. (2006) 39:337–45. doi: 10.1016/j.jadohealth.2005.12.026
15. Tapsell LC. Foods and food components in the mediterranean diet: supporting overall effects. BMC Med. (2014) 12:100. doi: 10.1186/1741-7015-12-100
16. Hu FB. Dietary pattern analysis: a new direction in nutritional epidemiology. Curr Opin Lipidol. (2002) 13:3–9. doi: 10.1097/00041433-200202000-00002
17. Oellingrath IM, Svendsen MV, Hestetun I. Eating patterns and mental health problems in early adolescence: a cross-sectional study of 12-13-year-old Norwegian schoolchildren. Public Health Nutr. (2014) 17:2554–62. doi: 10.1017/S1368980013002747
18. Berlin KS, Parra GR, Williams NA. An introduction to latent variable mixture modeling (part 2): longitudinal latent class growth analysis and growth mixture models. J Pediatr Psychol. (2014) 39:188–203. doi: 10.1093/jpepsy/jst085
19. Lim K-S, Wong CH, McIntyre RS, Wang J, Zhang Z, Tran BX, et al. Global lifetime and 12-month prevalence of suicidal behavior, deliberate self-harm and non-suicidal self-injury in children and adolescents between 1989 and 2018: a meta-analysis. Int J Environ Res Public Health. (2019) 16:4581. doi: 10.3390/ijerph16224581
20. Cao S, Liu L, Zhu Q, Zhu Z, Zhou J, Wei P, et al. Association between dietary patterns and plasma lipid biomarker and female breast cancer risk: comparison of latent class analysis (Lca) and factor analysis (Fa). Front Nutr. (2021) 8:PMC8761950. doi: 10.3389/fnut.2021.645398
21. Cole TJ, Lobstein T. Extended international (Iotf) body mass index cut-offs for thinness, overweight and obesity. Pediatr Obes. (2012) 7:284–94. doi: 10.1111/j.2047-6310.2012.00064.x
22. Goldberg D. Mental health priorities in a primary care setting. Ann N Y Acad Sci. (1978) 310:65–8. doi: 10.1111/j.1749-6632.1978.tb22054.x
23. Li H, Boey KW. Assessing psychological well-being of college student: psychometric properties of Ghq-20. Psychol Develop Educ. (2002) 18:75–9. doi: 10.16187/j.cnki.issn1001-4918.2002.01.016
24. Bjordal K, Kaasa S. Psychological distress in head-and-neck-cancer patients 7–11 years after curative treatment. Br J Cancer. (1995) 71:592–7. doi: 10.1038/bjc.1995.115
25. Gautreau S, Monsen ER. Priorities of nutritional concepts assigned by health-professionals and students. J Med Educ. (1979) 54:607–12. doi: 10.1097/00001888-197908000-00001
26. Chung J, Kwon SO, Ahn H, Hwang H, Hong SJ, Oh SY. Association between dietary patterns and atopic dermatitis in relation to Gstm1 and Gstt1 polymorphisms in young children. Nutrients. (2015) 7:9440–52. doi: 10.3390/nu7115473
27. Lee HA, Hwang HJ, Oh SY, Park EA, Cho SJ, Kim HS, et al. Which diet-related behaviors in childhood influence a healthier dietary pattern? From the Ewha birth and growth cohort. Nutrients. (2016) 9:4. doi: 10.3390/nu9010004
28. Nylund KL, Asparoutiov T, Muthen BO. Deciding on the number of classes in latent class analysis and growth mixture modeling: a monte carlo simulation study. Struct Equ Model Multidiscip J. (2007) 14:535–69. doi: 10.1080/10705510701575396
29. Zhou S-J, Zhang L-G, Wang L-L, Guo Z-C, Wang J-Q, Chen J-C, et al. Prevalence and socio-demographic correlates of psychological health problems in Chinese adolescents during the outbreak of Covid-19. Eur Child Adolesc Psychiatry. (2020) 29:749–58. doi: 10.1007/s00787-020-01541-4
30. Luo Y, Cui Z, Zou P, Wang K, Lin Z, He J, et al. Mental health problems and associated factors in chinese high school students in henan province: a cross-sectional study. Int J Environ Res Public Health. (2020) 17:5944. doi: 10.3390/ijerph17165944
31. Rutters F, Nieuwenhuizen AG, Lemmens SG, Born JM, Westerterp-Plantenga MS. Acute stress-related changes in eating in the absence of hunger. Obesity. (2009) 17:72–7. doi: 10.1038/oby.2008.493
32. Finch LE, Cummings JR, Tomiyama AJ. Cookie or clementine? Psychophysiological stress reactivity and recovery after eating healthy and unhealthy comfort foods. Psychoneuroendocrinology. (2019) 107:26–36. doi: 10.1016/j.psyneuen.2019.04.022
33. Wang M, Zhong J-M, Wang H, Zhao M, Gong W-W, Pan J, et al. Breakfast consumption and its associations with health-related behaviors among school-aged adolescents: a cross-sectional study in Zhejiang Province, China. Int J Environ Res Public Health. (2016) 13:761. doi: 10.3390/ijerph13080761
34. Witbracht M, Keim NL, Forester S, Widaman A, Laugero K. Female breakfast skippers display a disrupted cortisol rhythm and elevated blood pressure. Physiol Behav. (2015) 140:215–21. doi: 10.1016/j.physbeh.2014.12.044
35. de Azeredo LA, Viola TW, Rothmann LM, Trentin R, Arteche AX, Kristensen CH, et al. Hair cortisol levels and mental health problems in children and adolescents exposed to victimization. Stress Int J Biol Stress. (2020) 23:546–55. doi: 10.1080/10253890.2019.1690448
36. Xu H, Wu X, Wan Y, Zhang S, Yang R, Wang W, et al. Interaction effects of co-consumption of fast food and sugar-sweetened beverages on psychological symptoms: evidence from a nationwide survey among chinese adolescents. J Affect Disord. (2020) 276:104–11. doi: 10.1016/j.jad.2020.07.030
37. Oddy WH, Allen KL, Trapp GSA, Ambrosini GL, Black LJ, Huang R-C, et al. Dietary patterns, body mass index and inflammation: pathways to depression and mental health problems in adolescents. Brain Behav Immun. (2018) 69:428–39. doi: 10.1016/j.bbi.2018.01.002
38. Janssen HG, Davies IG, Richardson LD, Stevenson L. Determinants of takeaway and fast food consumption: a narrative review. Nutr Res Rev. (2018) 31:16–34. doi: 10.1017/S0954422417000178
39. Jaworowska A, Blackham T, Stevenson L, Davies IG. Determination of salt content in hot takeaway meals in the United Kingdom. Appetite. (2012) 59:517–22. doi: 10.1016/j.appet.2012.06.018
40. Tyrrell RL, Greenhalgh F, Hodgson S, Wills WJ, Mathers JC, Adamson AJ, et al. Food environments of young people: linking individual behaviour to environmental context. J Public Health. (2017) 39:95–104. doi: 10.1093/pubmed/fdw019
41. Teo CH, Chin YS, Lim PY, Masrom SAH, Shariff ZM. School-based intervention that integrates nutrition education and supportive healthy school food environment among malaysian primary school children: a study protocol. BMC Public Health. (2019) 19:1427. doi: 10.1186/s12889-019-7708-y
42. Mohammadi S, Su TT, Papadaki A, Jalaludin MY, Dahlui M, Mohamed MNA, et al. Perceptions of eating practices and physical activity among malaysian adolescents in secondary schools: a qualitative study with multi-stakeholders. Public Health Nutr. (2021) 24:2273–85. doi: 10.1017/S1368980020002293
43. László KD, Andersson F, Galanti MR. School climate and mental health among swedish adolescents: a multilevel longitudinal study. BMC Public Health. (2019) 19:1695. doi: 10.1186/s12889-019-8018-0
44. Oberle E, Guhn M, Gadermann AM, Thomson K, Schonert-Reichl KA. Positive mental health and supportive school environments: a population-level longitudinal study of dispositional optimism and school relationships in early adolescence. Soc Sci Med. (2018) 214:154–61. doi: 10.1016/j.socscimed.2018.06.041
45. Hu H, Lu S, Huang C-C. The psychological and behavioral outcomes of migrant and left-behind children in China. Child Youth Serv Rev. (2014) 46:1–10. doi: 10.1016/j.childyouth.2014.07.021
46. Xie J, Li X, Luo H, He L, Bai Y, Zheng F, et al. Depressive symptoms, sleep quality and diet during the 2019 novel coronavirus epidemic in China: a survey of medical students. Front Public Health. (2021) 8:588578. doi: 10.3389/fpubh.2020.588578
Keywords: eating habit, mental health, adolescent, latent class analysis, energy intake
Citation: Li X, Sun M, Yao N, Liu J, Wang L, Hu W, Yang Y, Guo R, Li B and Liu Y (2022) Association between patterns of eating habits and mental health problems in Chinese adolescents: A latent class analysis. Front. Nutr. 9:906883. doi: 10.3389/fnut.2022.906883
Received: 29 March 2022; Accepted: 08 July 2022;
Published: 05 August 2022.
Edited by:
Fenghua Sun, The Education University of Hong Kong, Hong Kong SAR, ChinaReviewed by:
Zhaowei Kong, University of Macau, Macao SAR, ChinaEric Tsz-Chun Poon, The Education University of Hong Kong, Hong Kong SAR, China
Copyright © 2022 Li, Sun, Yao, Liu, Wang, Hu, Yang, Guo, Li and Liu. This is an open-access article distributed under the terms of the Creative Commons Attribution License (CC BY). The use, distribution or reproduction in other forums is permitted, provided the original author(s) and the copyright owner(s) are credited and that the original publication in this journal is cited, in accordance with accepted academic practice. No use, distribution or reproduction is permitted which does not comply with these terms.
*Correspondence: Yajuan Liu, eWpsaXUmI3gwMDA0MDtqbHUuZWR1LmNu
Bo Li bGlfYm8mI3gwMDA0MDtqbHUuZWR1LmNu